- 1Fujian Maternity and Child Health Hospital, Affiliated to Fujian Medical University, Fuzhou, China
- 2Fujian Obstetrics and Gynecology Hospital, Affiliated to Fujian Medical University, Fuzhou, China
- 3School of Nursing, Fujian University of Traditional Chinese Medicine, Fuzhou, China
The roles of gut microbiota and metabolomics in women with gestational diabetes mellitus (GDM) are not well understood. This study investigated the gut metabolomic profiling of GDM rats and GDM rats treated with probiotic supplements. Associations between gut metabolites and microbiota were also studied in GDM rats. Liquid chromatography–mass spectrometry was used to detect gut metabolites in GDM rats and GDM rats treated with probiotic supplements of 0.5 g (low-dose group) or 1 g (high-dose group) for 15 days. Each gram of probiotic supplement contained 5 × 107 colony-forming units (CFU) of Lactobacillus rhamnosus LGG and 1 × 108 CFU of Bifidobacterium animalis subspecies lactis Bb12. The association between gut metabolites and microbiota in GDM rats was investigated using Spearman’s correlation. Finally, 10 rats in the normal pregnant group, eight rats in the GDM model group, eight GDM rats in the low-dose probiotics group, and nine GDM rats in the high-dose probiotics group were further studied. Serum parameters and pancreatic and colon histology were significantly changed in GDM rats, and these were restored using probiotic supplements. In total, 999 gut metabolites were detected in the feces, and GDM rats were distinguished from normal rats. The levels of 44 metabolites were increased in GDM rats, and they were alleviated using probiotic supplements. Changes in metabolites in GDM rats were associated with amino acids and bile acids metabolism signaling pathways. Furthermore, changes in metabolites after probiotic supplementation were associated with porphyrin and chlorophyll metabolism pathways. We found that the Allobaculum genus displayed strong positive correlations, whereas the Bryobacter and Gemmatimonas genera displayed strong negative correlations with metabolisms of amino acids and bile acids in GDM rats. The Lactobacillus and Bifidobacterium genera were positively correlated with gut metabolites. Overall, our results showed that metabolism signaling pathways of amino acids and bile acids are associated with the development of GDM. Probiotic supplements alleviate the pathology of GDM through the metabolism pathways of amino acids, bile acids, porphyrin, and chlorophyll.
Introduction
Gestational diabetes mellitus (GDM) is the most common type of obstetric complication (Veeraswamy et al., 2012) and affects 14.8% of pregnant women in China (Gao et al., 2019). The incidence of GDM has increased globally due to poor dietary structure, unhealthy lifestyle changes, and environmental pollution (Ovesen et al., 2018; Rasmussen et al., 2020). GDM is not only associated with the adverse maternal-infant outcomes in the short term (Kurtzhals et al., 2018) but is also related to metabolic diseases in the woman and offspring in the long term, such as childhood obesity and type 2 diabetes (Kurtzhals et al., 2018; Ponnusamy et al., 2020). Therefore, effective measures to prevent and treat GDM are required.
The gut microbiome plays important roles in modulating host metabolism and immune cell development (He et al., 2020). Dysregulated gut microbiota is associated with diabetes, obesity, and other metabolic diseases (Qin et al., 2012). Metabolites also play important roles in revealing the gut microbiome and maintaining the health status of the host (Liu et al., 2017; Olson et al., 2018; Canfora et al., 2019; He et al., 2020). Abnormalities in gut microbiota and metabolomics in women are important for pathogenesis of GDM. GDM-related differential metabolites are mainly involved in metabolic pathways such as steroid hormone biosynthesis, metabolisms of amino acids, fatty acids, arachidonic acid, and butyric acid, and bile secretion (Li, 2019); however, gut metabolomics of GDM remain unclear.
Probiotics have positive effects on preventing and alleviating diabetes by regulating the intestinal microenvironment and immunity (Liu et al., 2017). Probiotic therapy may be a promising approach to improve glucose control and insulin resistance among pregnant women (de Brito Alves et al., 2019; Pan et al., 2021). Previously, our results showed that Lactobacillus and Bifidobacterium probiotic supplements could reduce the fasting blood glucose level of GDM rats by restoring the diversity of gut microbiota (Zheng et al., 2021). However, the effects of probiotic supplements on gut metabolomics in GDM are unclear. The associations between gut metabolites and microbiota in GDM rats are also unclear. Therefore, in this study, we analyzed gut metabolomic profiles of GDM rats and GDM rats treated with Lactobacillus and Bifidobacterium probiotic supplements. Associations between gut metabolites and microbiota in GDM rats were also studied.
Materials and Methods
Gestational Diabetes Mellitus Rats Mode and Probiotics Supplement
The procedures of GDM model construction and probiotics supplement can be accessed from our previous study (Zheng et al., 2021). Briefly, after adaptive feeding, 48-week-old female Sprague–Dawley rats were randomly divided into normal pregnant group that fed with ordinary feed and GDM model group that received high-fat and high-sugar feed for 6 weeks. Then, two female rats were paired with one male rat overnight. Pregnant rats were validated by vaginal secretions and recorded as embryonic day 1. The pregnant rats were further injected with streptozocin (25 mg/kg) to induce the development of GDM. Fasting blood glucose values higher than 7.8 mmol/L at embryonic day 4 were considered indicative of GDM rats. The GDM rats were intragastrically administrated with or without probiotic supplements of 0.5 g (low-dose group) or 1 g (high-dose group) for 15 days. Each gram of probiotic supplement contained 5 × 107 colony-forming units (CFU) of Lactobacillus rhamnosus LGG and 1 × 108 CFU of Bifidobacterium animalis subspecies lactis Bb12 (Life-space®, Australia). Finally, 10 rats in the normal pregnant group, eight rats in the GDM model group, eight GDM rats in the low-dose probiotics group, and nine GDM rats in the high-dose probiotics group were further studied. All animal procedures were approved by the Fujian University of Traditional Chinese Medicine (certificate number: SYXK 2019-0007; ethics approval number: FJTCM IACUC 2020020).
Enzyme-Linked Immunosorbent Assay of Serum Parameters
Abdominal aortic blood was collected on embryonic day 19. Before blood extraction, a dose of 5 ml/kg of 20% uratan solution was injected intraperitoneally for anesthesia. Abdominal aortic blood was placed at 25°C room temperature for 2 h, centrifuged at a low temperature to obtain the serum sample, and finally stored at −80°C. Concentrations of interleukin-6 (IL-6), tumor necrosis factor–α (TNF-α), nitric oxide (NO), insulin, and low-density lipoprotein cholesterol (LDL-C), high-density lipoprotein cholesterol (HDL-C), and lipid factors including total cholesterol (TC) and triglyceride (TG) were measured using enzyme-linked immunosorbent assay (ELISA) kits (Boster Biological Technology Co., Ltd., Wuhan, Hubei, China for IL-6; ProteinTech® Group, Chicago, IL, United States, for insulin and TNF-α; and Nanjing Jiancheng Bioengineering Institute Co., Ltd., Nanjing, Jiangsu, China, for NO, LDL-C, HDL-C, TC, and TG). The value of homeostasis model assessment–estimated insulin resistance (HOMA-IR) was also calculated.
Histopathological Analysis
The pancreatic and colon tissues of rats were quickly collected and placed on ice. The tissues were fixed, embedded in paraffin, and stained with hematoxylin and eosin for histopathological analysis.
Analysis of 16S rRNA Sequencing Data
The procedures and analysis method for 16S rRNA sequencing have been described in a previous study (Zheng et al., 2021). Raw sequence data are available in the Sequence Read Archive under the BioProject accession number PRJNA770477. The 16S rRNA sequencing was performed using Illumina HiSeq platform (Illumina, California, United States). Raw data were cleared using FLASH, TrimMomatic, and UCHIME software. In addition, operational taxonomic units (OTUs) of 16S rRNA sequencing data were clustered using the UCLUST software based on 97% similarity. The obtained OTUs were then matched with the SILVA database for taxonomic assignment.
Fecal Samples Processing
At embryonic day 19, fecal samples were collected from each rat and stored in a −80°C refrigerator until metabolomic analysis. For fecal sample pretreatment, 50 mg of sample was weighed into an Eppendorf tube, and 1,000 μl of extract solution (acetonitrile:methanol:water = 2:2:1, with an isotopically labeled internal standard mixture) was added. After subjecting to a 30-s vortex, the samples were homogenized at 35 Hz for 4 min and sonicated for 5 min in an ice-water bath. The homogenization and sonication cycles were repeated three times. The samples were then incubated for 1 h at −40°C and centrifuged at 12,000 rpm for 15 min at 4°C. The resulting supernatant was transferred to a fresh glass vial for further analysis. The quality control sample was prepared by mixing an equal aliquot of the supernatant from all samples.
Liquid Chromatography With Tandem Mass Spectrometry Analysis
LC-MS/MS analyses were performed using an ultrahigh-performance liquid chromatography system (Vanquish, Thermo Fisher Scientific) with a UPLC BEH amide column (2.1 × 100 mm, 1.7 μm) coupled to a Q Exactive HF-X mass spectrometer (Orbitrap MS, Thermo Fisher Scientific). The mobile phase consisted of 25 mmol/L of ammonium acetate and 25 mmol/L of ammonium hydroxide in water (pH = 9.75) and acetonitrile. The analysis was carried out with elution gradients as follows: 0–0.5 min, 95% B; 0.5–7.0 min, 95–65% B; 7.0–8.0 min, 65–40% B; 8.0–9.0 min, 40% B; 9.0–9.1 min, 40–95% B; and 9.1–12.0 min, 95% B. The column temperature was maintained at 30°C. The auto-sampler temperature was 4°C, and the injection volume was 3 μl.
The Q Exactive HF-X mass spectrometer was used for its ability to acquire MS/MS spectra in the information-dependent acquisition mode in the control of the acquisition software (Xcalibur, Thermo Fisher Scientific). In this mode, the acquisition software continuously evaluates the full-scan MS spectrum. Electrospray ionization source conditions were set as follows: sheath gas flow rate of 50 arbitrary units, aux gas flow rate of 10 arbitrary units, capillary temperature at 320°C, a full MS resolution of 60,000, MS/MS resolution of 7,500, collision energy of 10/30/60 in the normalized collision energy mode, and spray voltage of 3.5 kV (positive) or −3.2 kV (negative). Meanwhile, raw data were converted to the mzXML format using the ProteoWizard application and processed with an in-house program, which was developed using R and based on the XCMS platform, for peak detection, extraction, alignment, and integration. Then, an in-house MS2 database (BiotreeDB) was used for metabolite annotation. The cutoff for annotation was set at 0.3.
Hierarchical Clustering Analysis
The hierarchical clustering analysis of the gut metabolites was performed using the pheatmap function in R software.
Orthogonal Partial Least-Squares Discrimination Analysis
The Orthogonal Partial Least-Squares Discrimination Analysis (OPLS-DA) was used to analyze the metabolic patterns. The OPLS-DA permutation test was used to test the effect of the model fit. The R2Y (cum) index of the OPLS-DA permutation test was used to determine the accuracy of the model fit. The Q2 (cum) index refers to the predictive power of the model, and it is an evaluation parameter that is model-validated to prevent random fitting or overfitting. When the R2Y (cum) and Q2 (cum) indexes are closer to 1, the model fits well. In addition, if they are greater than 0.5, then the model is acceptable. On the basis of the threshold criteria of the projection of the first principal component of OPLS-DA > 1, an absolute fold change of < 0.5 or > 2 and a P-value of < 0.05 indicated a significant difference.
Metabolic Signaling Pathways Analysis
Metabolic signaling pathways of gut metabolites were obtained from the Kyoto Encyclopedia of Genes and Genomes (KEGG) pathway database.1 The signaling pathways included more than four metabolites.
Correlation Analysis and Co-occurrence Network of Target Metabolites and Gut Microbiota
The correlations of metabolites and the relative abundance of gut microbiota were analyzed using the Spearman’s correlation test through the psych package in the R software. The co-occurrence network of metabolites and gut microbiota was constructed using the Cytoscape software (version 3.1.1).
Data Analysis
Statistical analysis and plotting of figures were conducted using GraphPad Prism (version 7.0), SPSS (version 25.0), and R programming language software (version 4.4.1). A normality test was performed to determine whether the data obeyed a normal distribution. If data obeyed a normal distribution, then a t-test or post-hoc test was performed to analyze the data. Otherwise, a non-parametric test was conducted. Statistical significance was set at P < 0.05, and data were presented as mean ± standard deviation (mean ± SD).
Results
Probiotic Supplements Restored Normal Serum Parameters, and Pancreatic and Colon Histology Are Influenced by Gestational Diabetes Mellitus
Previously, we successfully constructed a GDM rat model and demonstrated that Lactobacillus and Bifidobacterium probiotic supplements can reduce the fasting blood glucose level of GDM rats by restoring the diversity of gut microbiota (Zheng et al., 2021). Furthermore, using the same GDM model, we tested the effects of Lactobacillus and Bifidobacterium probiotic supplements on the concentrations of IL-6, TNF-α, NO, insulin, LDL-C, HDL-C, TC, and TG and estimated the value of HOMA-IR in the serum of GDM rats. In total, 10 normal pregnant rats, eight GDM rats, eight GDM rats with low-dosage probiotic supplements, and nine GDM rats with high-dosage probiotic supplements were tested. Compared to the normal pregnant rats, the concentrations of TNF-α, NO, insulin, LDL-C, TC, and TG (P = 0.002, P = 0.003, P = 0.041, P = 0.000, P = 0.000, and P = 0.000, respectively) and the value of HOMA-IR were increased in GDM rats (P = 0.000). Moreover, high-dose Lactobacillus and Bifidobacterium probiotic supplements significantly alleviated the concentrations of TNF-α, insulin, and LDL-C (P = 0.009, P = 0.011, and P = 0.007, respectively) and the value of HOMA-IR in GDM rats (P = 0.000). However, high-dose Lactobacillus and Bifidobacterium probiotic supplements did not alleviate the concentrations of NO, TC, and TG in GDM rats (P = 0.653, P = 0.046, and P = 0.002, respectively) (Figure 1A). In contrast, the concentrations of HDL-C were decreased in GDM rats (P = 0.000) and could not be further increased by the Lactobacillus and Bifidobacterium probiotic supplements (P = 0.794 and P = 0.358, respectively) (Figure 1A). Furthermore, the concentration of IL-6 was not significantly different among normal pregnant rats, GDM rats, and GDM rats with probiotic supplements (P < 0.05) (Figure 1A).
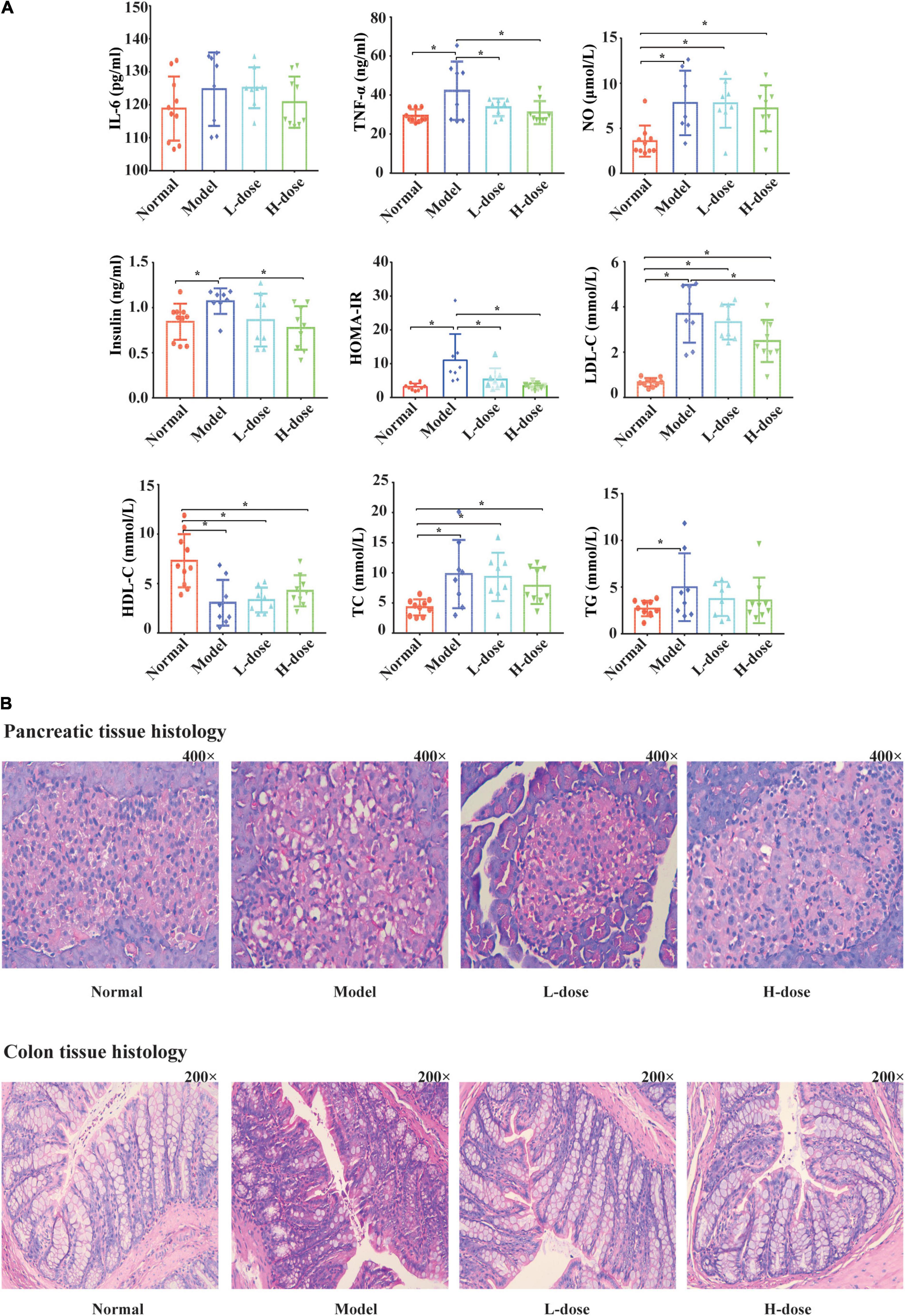
Figure 1. Probiotics supplements restore the normal serum parameters and pancreatic and colon histology influenced by GDM. (A) The concentrations of IL-6, TNF-α, NO, insulin, HOMA-IR, LDL-C, HDL, TC, and TG in GDM rats and GDM rats treated with Lactobacillus and Bifidobacterium probiotics supplements were tested. (B) The hematoxylin-eosin staining sections of the pancreas tissue and the colon tissue in GDM rats and GDM rats treated with probiotics supplements. *P < 0.05.
Lactobacillus and Bifidobacterium probiotic supplements could also influence the affected morphological and structural characteristics of pancreatic tissues in GDM rats. Compared to normal pregnant rats, the pancreatic islands of GDM rats were irregular and cyto-reduced and exhibited vacuolar degeneration. In addition, the capillary vessels of the pancreatic islands had undergone congestive expansion. After probiotic supplementation, the pancreatic islands of the rats were clearer, had less vacuolar degeneration, and showed cellular increase compared to these parameters in GDM rats (Figure 1B).
The morphological and structural characteristics of the colon tissues of GDM rats were also analyzed (Figure 1B). The colon mucous membrane and cell–cell junction of the epithelial cells of GDM rats were significantly damaged. The cryptostructures of colon tissues partially disappeared, and the beaker cells were reduced. After probiotic supplementation, the colon mucous membrane of rats was more complete and displayed queuing discipline compared to that in the GDM model group, and the numbers of cryptostructures and beaker cells were further increased.
Altered Metabolites in Gestational Diabetes Mellitus Rats
One possible mechanism of Lactobacillus and Bifidobacterium probiotic supplements restoring the normal serum parameters and pancreatic and colon histology influenced by GDM is the regulation of gut metabolites. Fecal metabolomic studies facilitate the interpretations of the metabolic interactions between the host, diet, and gut microbiota and provide functional data on the microbiome. Therefore, in this study, we further explored the influence of probiotic supplements on gut metabolites in the fecal samples of GDM rats using LC-MS/MS.
In total, 999 gut metabolites were detected. Normal rats were clearly distinguished from the GDM rats based on the levels of 999 gut metabolites by using the unsupervised clustering heatmap (Figure 2A). In addition, in the OPLS-DA score plots, normal pregnant rats and GDM rats were clearly separated from each other (Figure 2B). Similarly, the OPLS-DA permutation test indicated that the classifications of normal and GDM rats were robust and exhibited good fitness and prediction [(R2Y (cum) = (0, 0.71), Q2 (cum) = (0, −1.01)] (Figure 2C). Moreover, compared to normal rats, the levels of most gut metabolites were increased in GDM rats (Figure 2A).
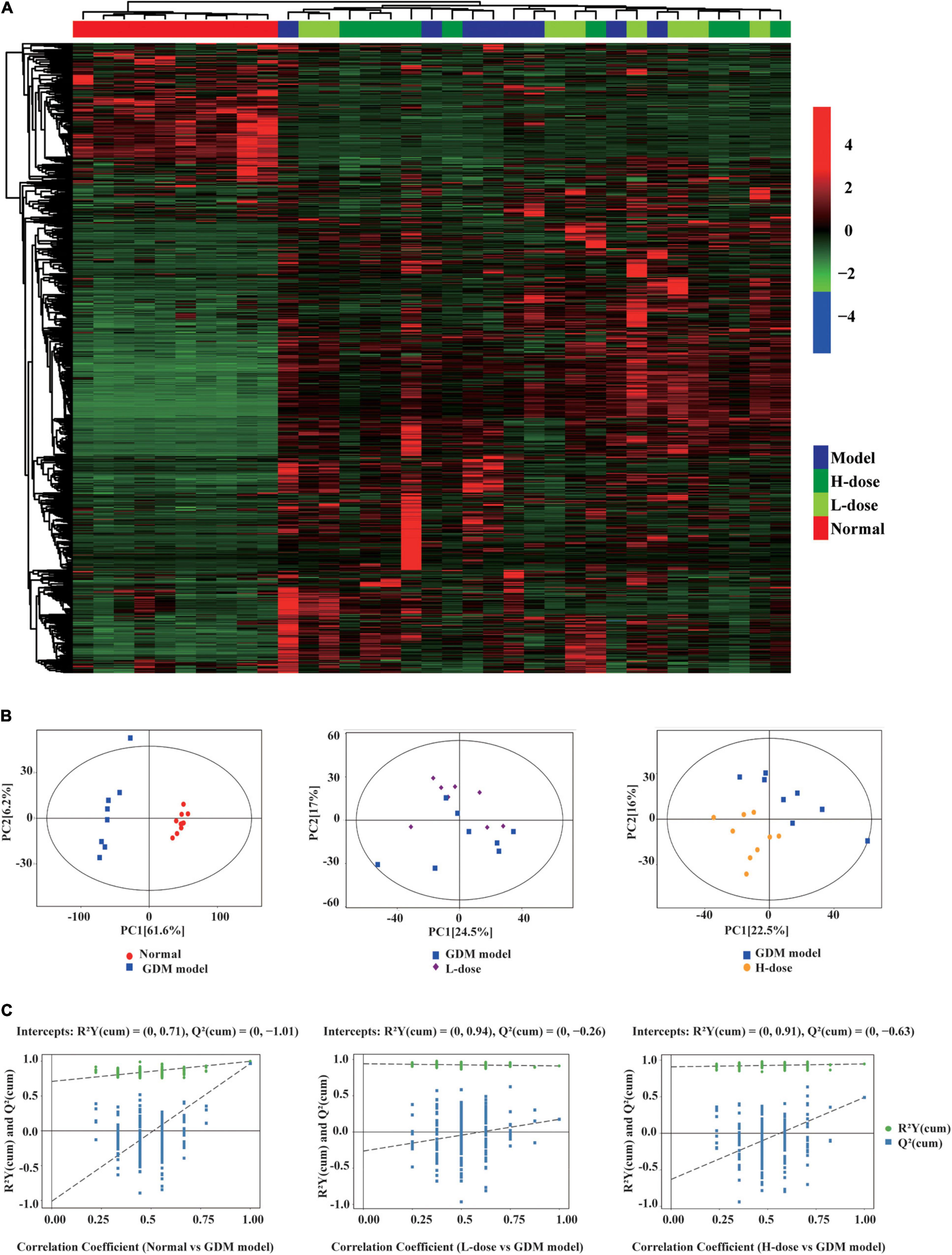
Figure 2. The gut metabolites feature of GDM rats and GDM rats treated with probiotics. (A) Hierarchical clustering analysis of 999 metabolites in GDM rats and GDM rats treated with Lactobacillus and Bifidobacterium probiotics supplements. The OPLS-DA analysis (B) and the OPLS-DA permutation test (C) of the gut metabolites in GDM rats and GDM rats treated with probiotics supplements.
However, in the unsupervised clustering heatmap, the GDM rats were indistinguishable from the GDM rats treated with low- or high-dose Lactobacillus and Bifidobacterium probiotic supplements (Figure 2A). In addition, in the OPLS-DA score plots and in the OPLS-DA permutation test, GDM rats and GDM rats with low probiotic supplements could not be distinctly separated from each other (Figures 2B,C). In contrast, GDM rats with high probiotic supplements represented a separate group in the OPLS-DA score plots and in the OPLS-DA permutation test (Figures 2B,C).
Altered Metabolites in Gestational Diabetes Mellitus Rats Treated With Probiotics
Although most of the altered metabolites in GDM rats could not be restored by Lactobacillus and Bifidobacterium probiotic supplements, partial metabolites were significantly influenced by probiotic supplements. We detected 44 metabolites that were increased in GDM rats, and their levels were alleviated by high-dose Lactobacillus and Bifidobacterium probiotic supplements (Figure 3A).
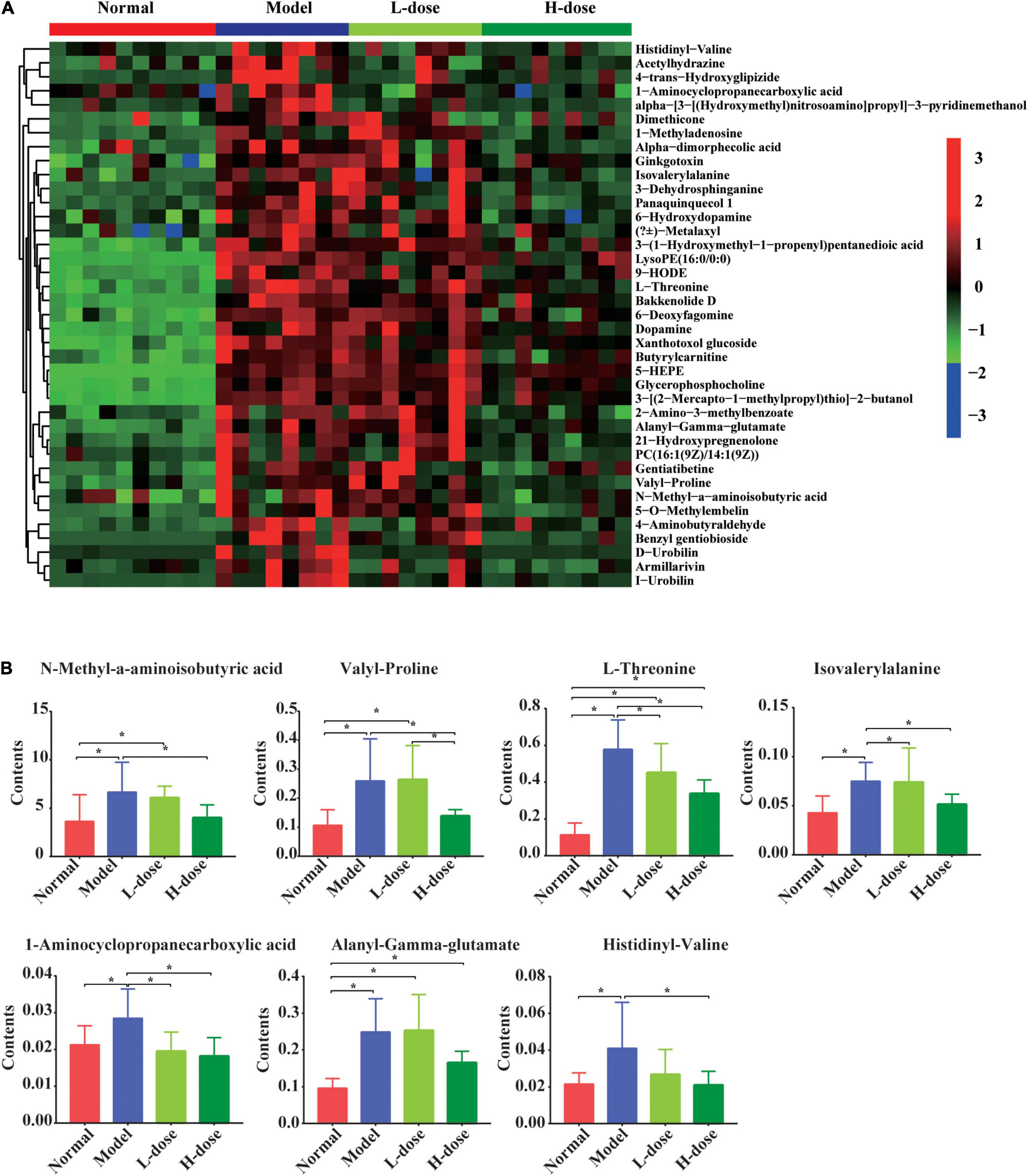
Figure 3. The influenced gut metabolites after Lactobacillus and Bifidobacterium probiotics supplements in GDM rats. (A) Hierarchical clustering analysis of 44 metabolites in GDM rats and GDM rats treated with Lactobacillus and Bifidobacterium probiotics supplements. (B) The comparison of seven amino acids by the ANOVA/Kruskal–Wallis and post-hoc test. *P < 0.05.
The levels of N-methyl-α-aminoisobutyric acid, L-proline, L-threonine, isovalerylalanine, 1-aminocyclopropanecarboxylic acid, alanyl-gamma-glutamate, and histidinyl-valine were shown in the box plots. The concentrations of N-methyl-α-aminoisobutyric acid, valyl-proline, L-threonine, isovalerylalanine, 1-aminocyclopropanecarboxylic acid, alanyl-gamma-glutamate, and histidinyl-valine concentrations were increased in GDM rats (P = 0.008, P = 0.002, P = 0.000, P = 0.004, P = 0.015, P = 0.000, and P = 0.008, respectively). Moreover, high-dose Lactobacillus and Bifidobacterium probiotic supplements significantly reduced the concentrations of these metabolites in GDM rats (P < 0.05) (Figure 3B), suggesting that probiotic supplements restored normal serum parameters and pancreatic and colon histology influenced by GDM through the regulation of these metabolites.
Changes in Metabolism Signaling Pathways of Amino Acids and Bile Acids in Gestational Diabetes Mellitus Rats
The changes in metabolites in the GDM rats were further studied. There were 499 metabolites that significantly changed in GDM rats compared to normal pregnant rats. Furthermore, the top 20 most significantly changed metabolites in GDM rats vs. normal pregnant rats were shown in Table 1. Those significantly changed metabolites in GDM rats was dihydroisoalantolactone, followed by (E)-2-Butyl-2-octenal and 4-Hydroxy-4-(3-pyridyl)-butanoic acid.
Next, the KEGG signaling pathways associated with the significantly altered metabolites in GDM rats vs. normal pregnant rats were studied. Although these metabolites were diverse, they were mainly concentrated in signaling pathways of amino acids, organic acids, and bile acids (Figure 4A). Metabolites including amino acids, organic acids, and bile acids and glucuronide metabolites were further depicted in the heatmap (Figure 4B). The concentrations of most of the metabolites were increased in GDM rats. For example, pipecolic acid, l-lysine, 5-aminopentanoic acid, L-phenylalanine, L-glutamic acid, and cholic acid from porphyrin and chlorophyll metabolism, biosynthesis of amino acids, and bile secretion signaling pathways were all significantly increased in GDM rats compared to their levels in normal pregnant rats (P = 0.000, P = 0.006, P = 0.006, P = 0.000, P = 0.003, and P = 0.015, respectively) (Figure 4C).
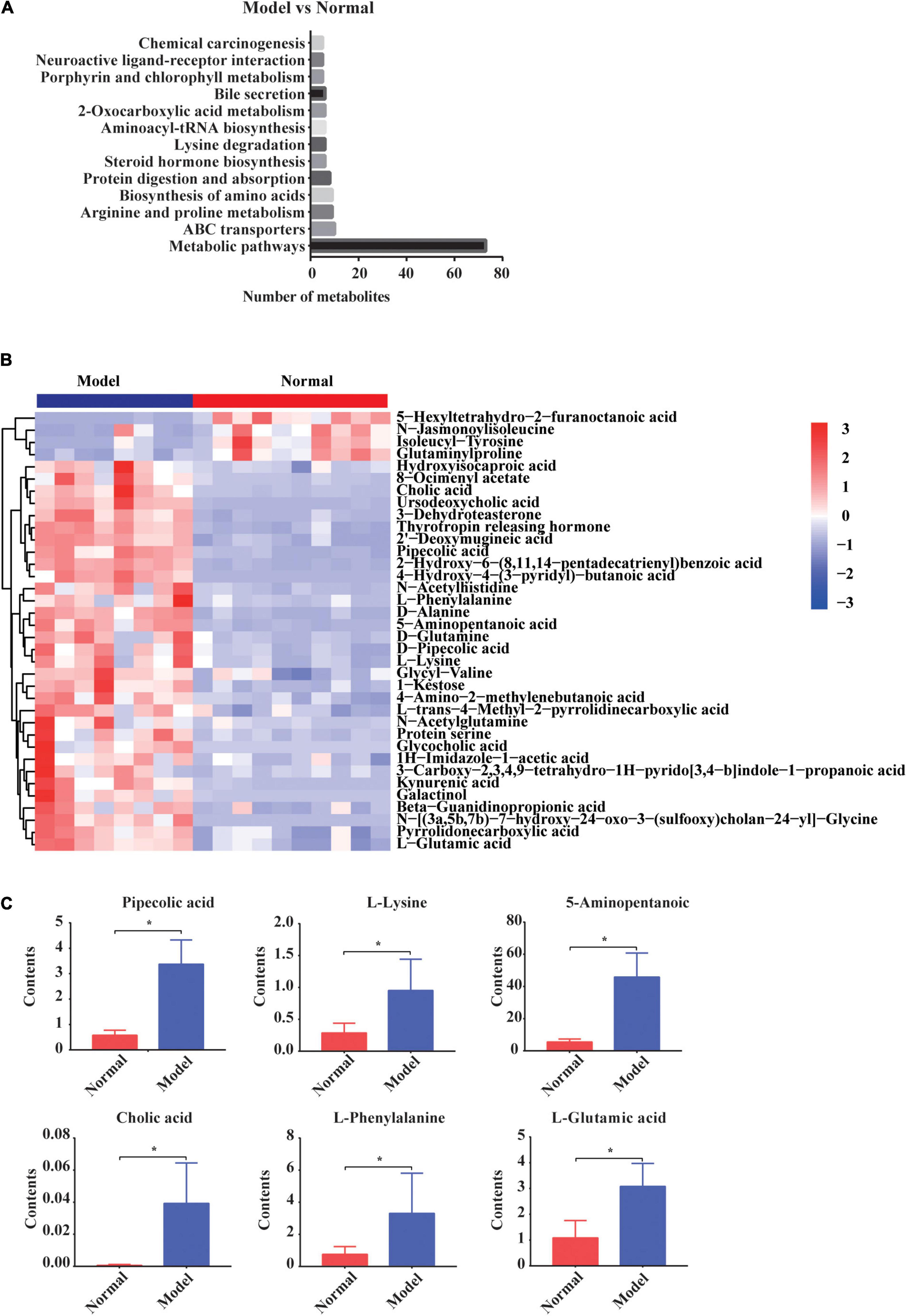
Figure 4. The changed gut metabolites and signaling pathways in normal pregnant rats vs. GDM rats. (A) Gut metabolites’ signaling pathways in KEGG Database. (B) Hierarchical clustering analysis of 36 metabolites in GDM rats. (C) The comparison of amino acids and bile acids that participated in gut metabolites’ signaling pathways by independent samples t-test or non-parametric test. *P < 0.05.
Metabolism Pathways of Porphyrin and Chlorophyll Were Associated With the Altered Metabolites Following Probiotic Supplementation
The changes in metabolites in GDM rats treated with Lactobacillus and Bifidobacterium probiotics were further studied. Compared to the metabolites in the GDM rats, low-dosage probiotic supplements induced the changes of 56 metabolites and high-dosage probiotic supplements induced the changes of 102 metabolites. Moreover, the top 20 most significantly changed metabolites in the GDM rats vs. GDM rats with low-dosage probiotic supplementation and GDM rats vs. GDM rats with high-dose probiotic supplementation are shown in Tables 2, 3, respectively. LysoPE and 2-aminoacrylic acid were significantly changed after low-dose probiotic supplementation, whereas [4]-gingerdiol 3,5-diacetate and propionylcarnitine were significantly changed after high-dose probiotic supplementation.
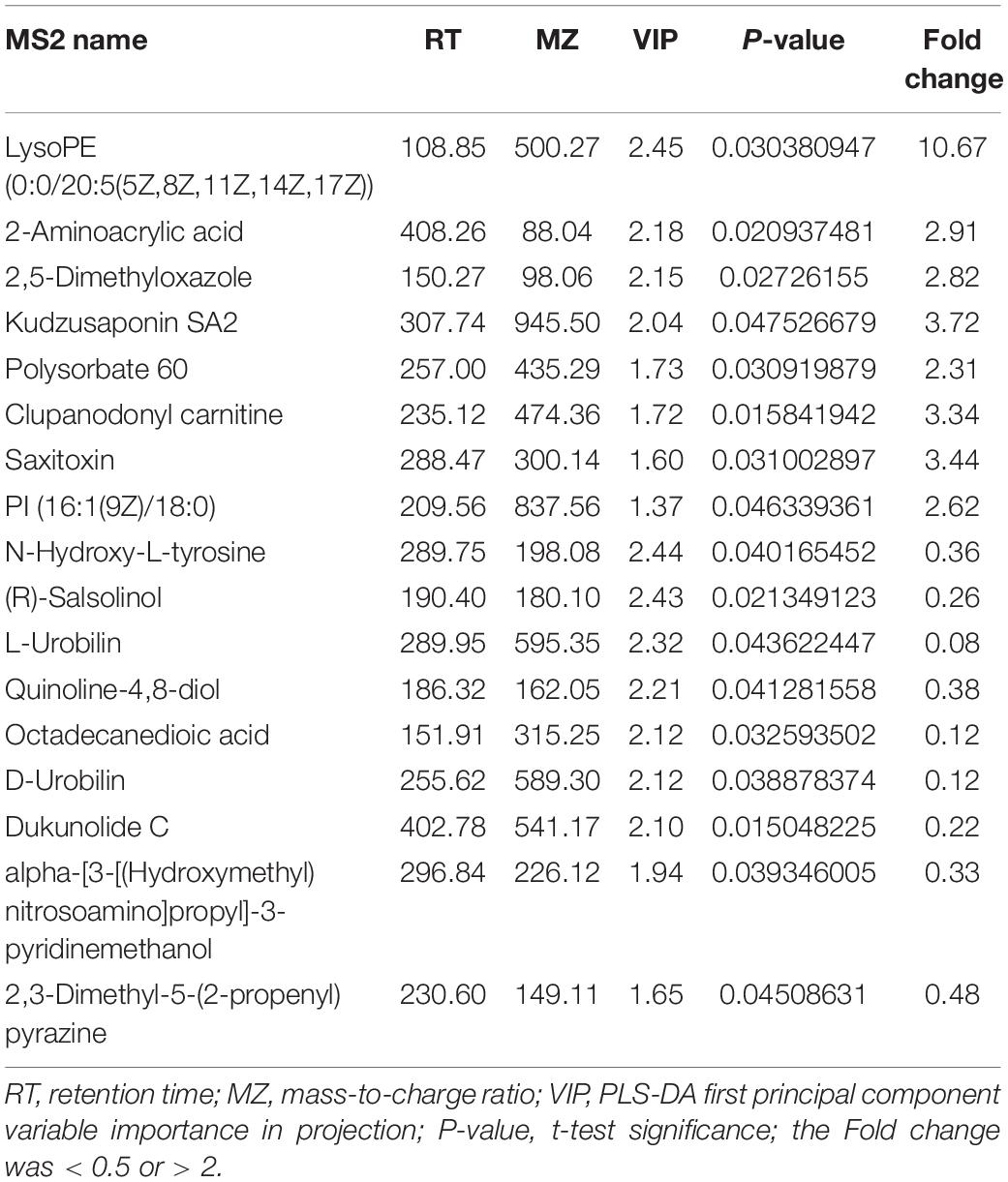
Table 2. The top 20 most significantly changed metabolites in GDM rats treated with lose-dose probiotics supplements vs. GDM rats.
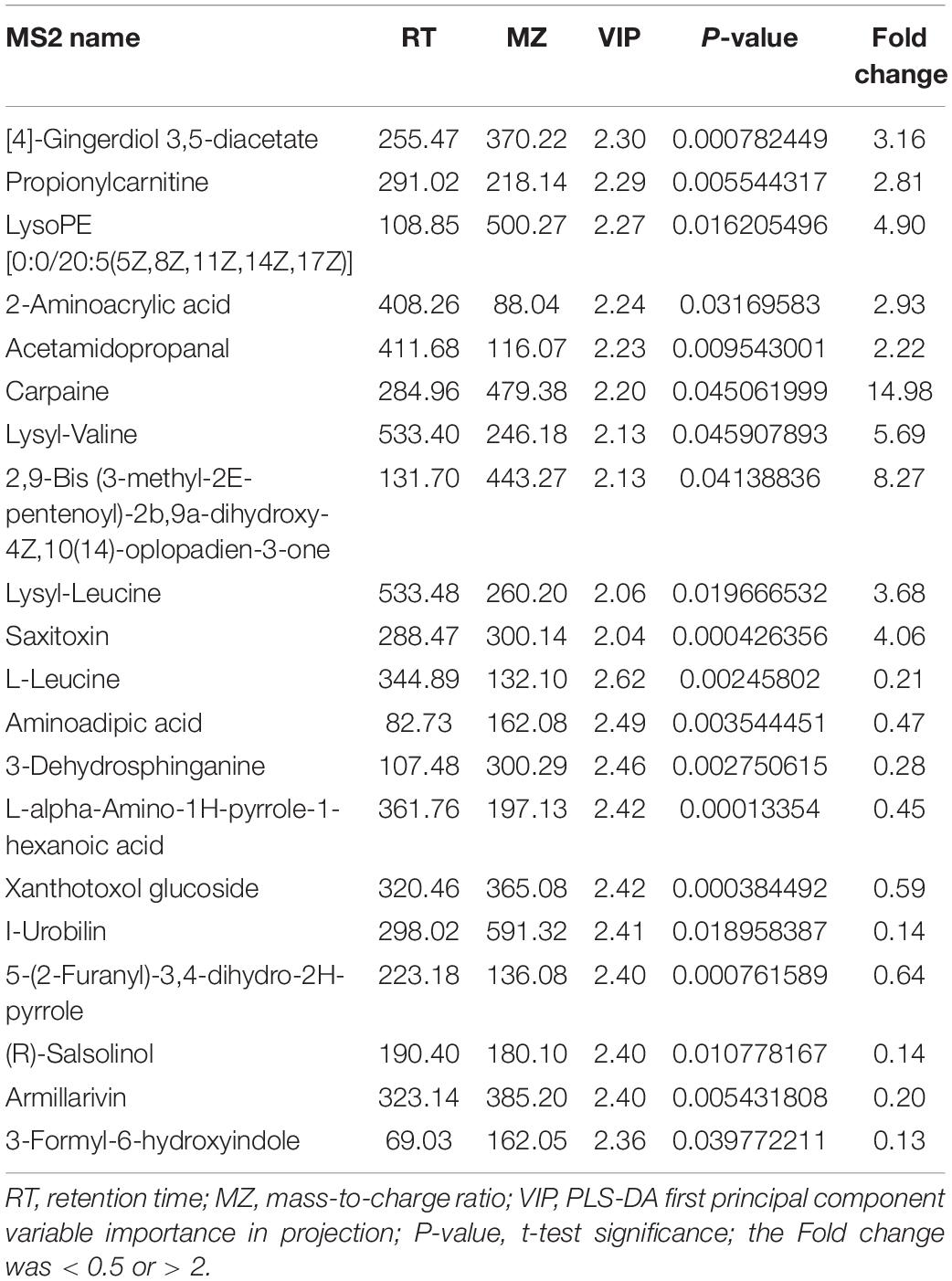
Table 3. The top 20 most significantly changed metabolites in GDM rats treated with high-dose probiotics supplements vs. GDM rats.
Next, the KEGG signaling pathways associated with the significantly changed metabolites in GDM rats vs. GDM rats with low-dose probiotic supplementation and GDM rats vs. GDM rats with high-dose probiotic supplementation were analyzed. Compared to GDM rats, the metabolites induced by low- or high-dose probiotic supplementation were concentrated on the metabolism pathways of porphyrin and chlorophyll (Figures 5A,B). Interestingly, the metabolism pathways of porphyrin and chlorophyll were also associated with the altered metabolites in GDM rats vs. normal pregnant rats (Figure 4A). We further showed that the levels of the metabolites L-threonine, I-urobilin, D-urobilin, and L-urobilin from the metabolism pathways of porphyrin and chlorophyll were decreased by high-dose probiotic supplementation in GDM rats (P = 0.001, P = 0.002, P = 0.002, and P = 0.005, respectively) (Figure 5C). In addition, D-urobilin and L-urobilin levels were also decreased by low-dose probiotic supplementation in GDM rats (P = 0.005 and P = 0.006, respectively) (Figure 5C), whereas L-glutamic acid level was decreased by low-dosage probiotic administration in GDM rats (P = 0.026) (Figure 5C).
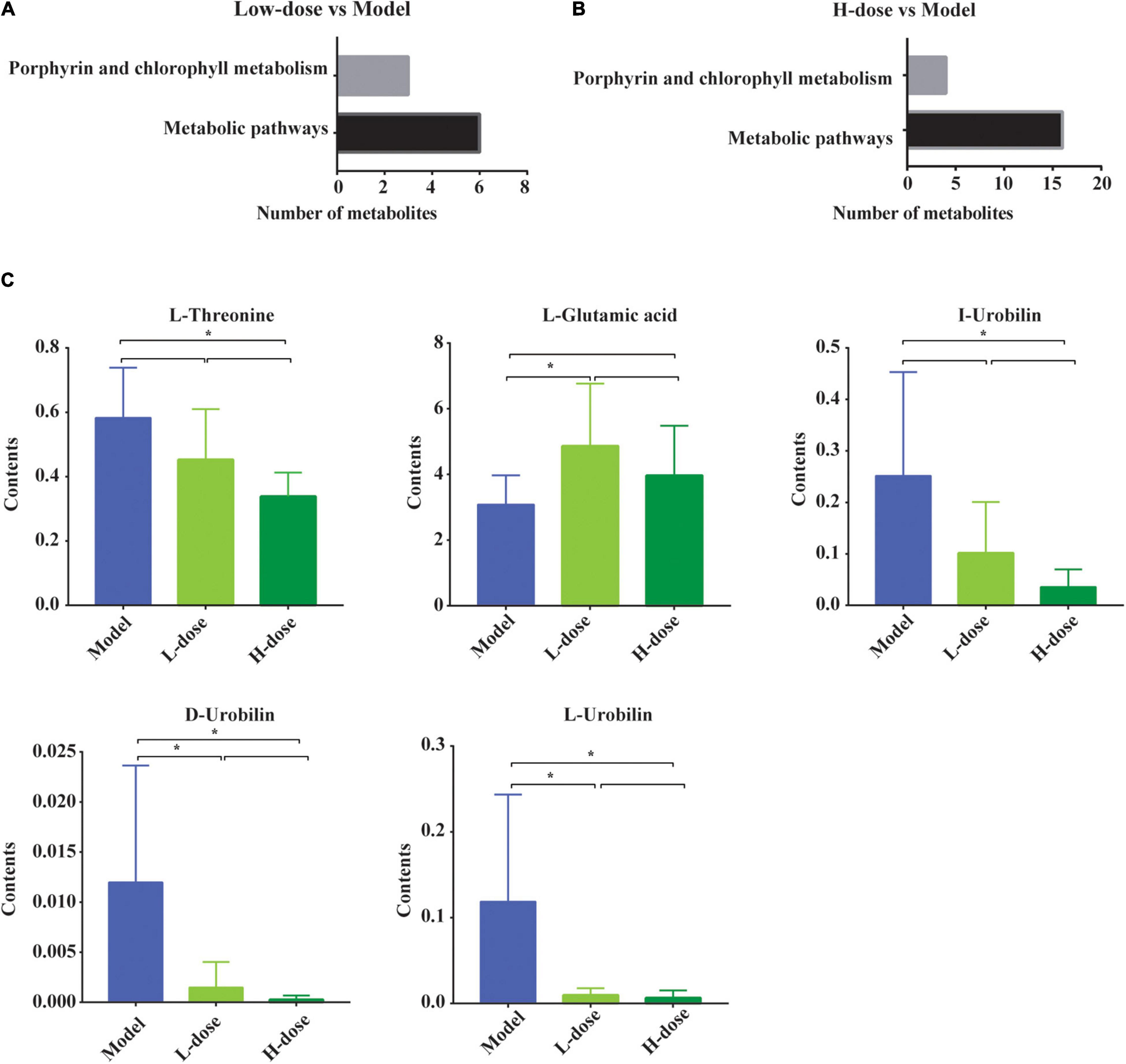
Figure 5. The changed gut metabolites and pathways in GDM rats treated with probiotics. (A,B) The porphyrin and chlorophyll metabolism pathway and metabolic pathway in KEGG Database. (C) The comparison of gut metabolites which participated in porphyrin and chlorophyll metabolism pathway and metabolic pathway by the post-hoc test or non-parametric test. *P < 0.05.
Changed Metabolites From Metabolism Signaling Pathways of Amino Acids and Bile Acids After Probiotic Supplementation
Although the significantly altered metabolites in GDM rats vs. GDM rats with Lactobacillus and Bifidobacterium probiotic supplementation were not enriched with metabolism signaling pathways of amino acids and bile acids, some metabolites from these pathways were still influenced by probiotic supplements. We showed that the concentration of 2-aminoacrylic acid was increased in GDM rats following supplementation with low or high dosages of Lactobacillus and Bifidobacterium probiotics, whereas that of 1-aminocyclopropanecarboxylic acid was decreased in GDM rats following supplementation with low or high dosages of probiotics (Figures 6A,B). Moreover, the concentrations of valyllysine, L-glutamic acid, serylvaline, thyrotropin-releasing hormone, clupanodonyl carnitine, and falcarindiol were significantly increased after the administration of low-dose Lactobacillus and Bifidobacterium probiotic supplements (Figure 6A). In contrast, the concentrations of 1-aminocyclopropanecarboxylic acid, benzyl-gentiobioside, L-threonine, isovalerylalanine, aminoadipic acid, alanyl-gamma-glutamate, phenylacetylglycine, L-leucine, and norvaline were significantly reduced by high-dose probiotic supplements (Figure 6B).
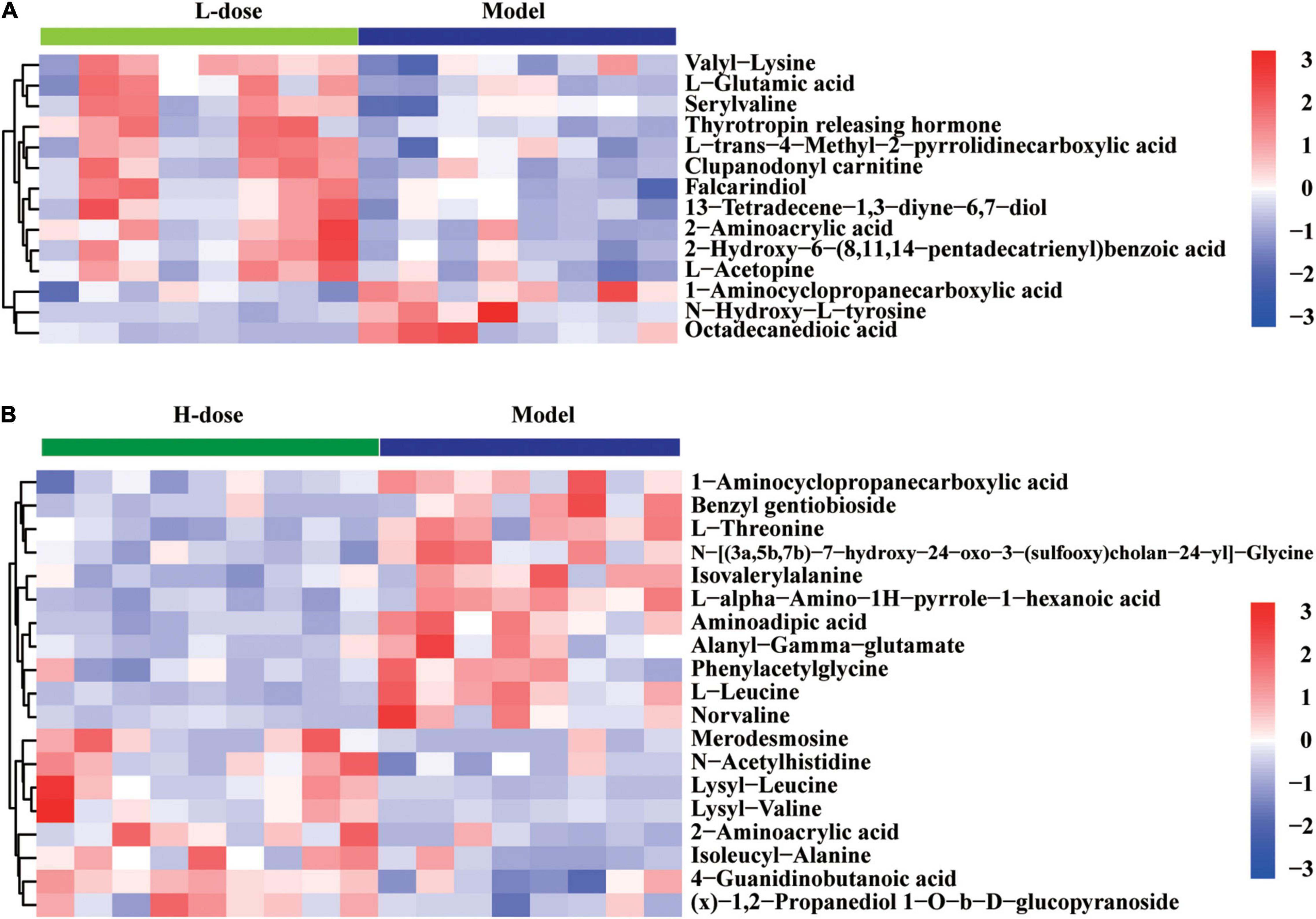
Figure 6. The changed amino acids and bile acids in GDM rats treated with probiotics supplements. (A) Hierarchical clustering analysis of 14 amino acids and bile acids in GDM rats treated with low-dose probiotics supplements vs. GDM rats. (B) Hierarchical clustering analysis of 19 amino acids and bile acids in GDM rats treated with high-dose probiotics supplements vs. GDM rats.
Correlations of Gestational Diabetes Mellitus-Related Gut Microbiota and Metabolites
Fecal metabolomics may reflect the functions of the gut microbiome. The altered metabolites in GDM rats may be perturbed by the gut microbiome during GDM pathogenesis. Previously, we studied gut microbiota in GDM rats and GDM rats with Lactobacillus and Bifidobacterium probiotic supplements (Zheng et al., 2021). Next, we explored the functional correlations between gut microbiota perturbations and metabolite changes. We observed strong correlations between the gut bacterial families and metabolites assigned to the metabolism of amino acids and bile acids in GDM rats, as determined by the Spearman’s correlation coefficient. The gut bacterial OTUs belonging to the genus Allobaculum displayed strong positive correlations (P < 0.05), whereas the genera Bryobacter and Gemmatimonas displayed strong negative correlations with the metabolism of amino acids and bile acids, such as L-glutamic acid, pipecolic acid, alanyl-gamma glutamate, isovalerylalanine, L-phenylalanine, 5-aminopentanoic acid, L-threonine, glycocholic acid, and lysine (P < 0.05) (Figure 7). Moreover, the metabolites pipecolic acid, 5-aminopentanoic acid, and cholic acid were associated with most of the gut bacterial genera in GDM rats (P < 0.05) (Supplementary Figure 1A).
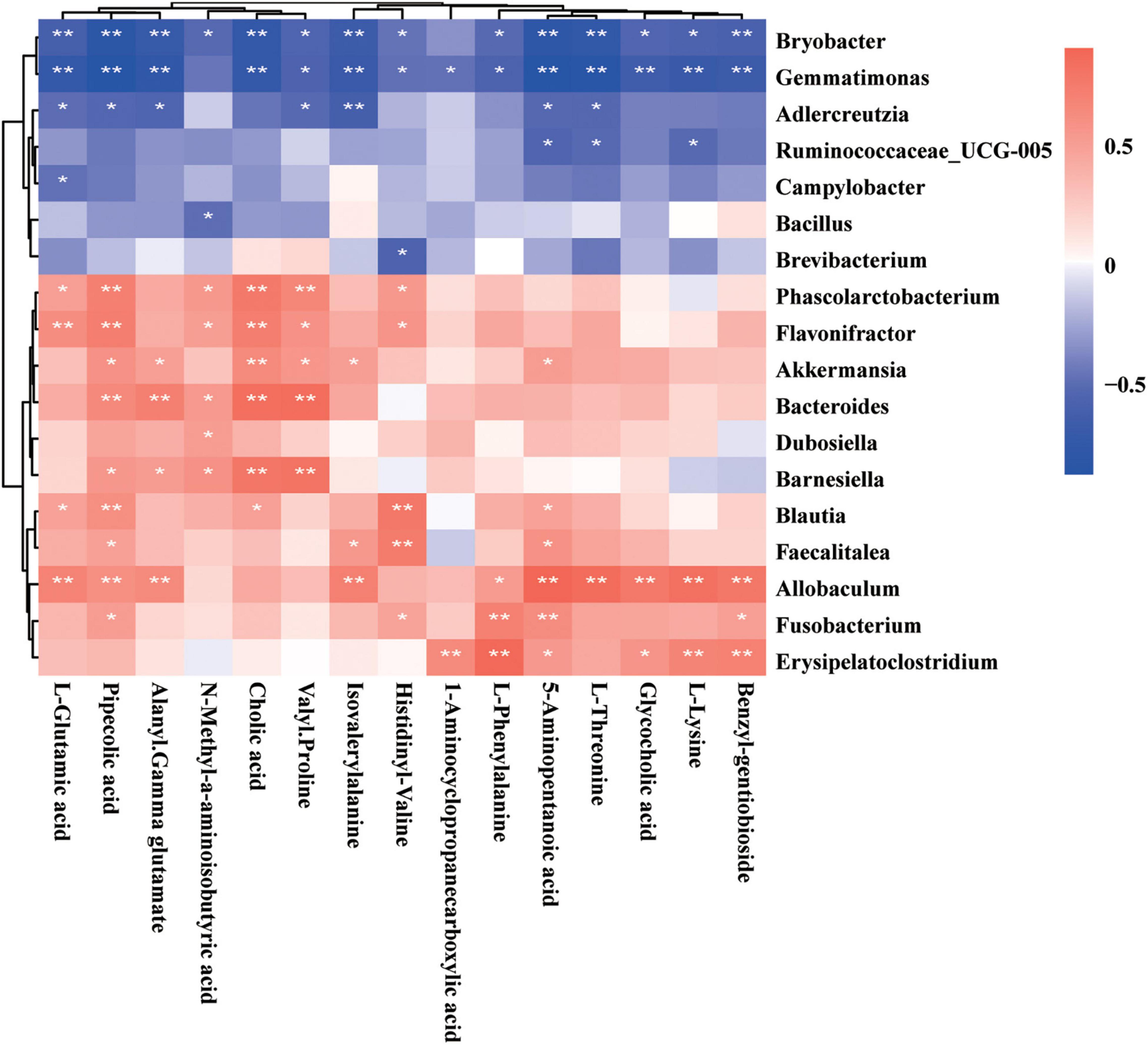
Figure 7. Spearman’s correlation analysis of metabolites and gut microbiota. The r-values were denoted with graduated colors, and red and blue grids indicated positive and negative correlations, respectively (*P < 0.05 and ** P < 0.01).
To further explore the reciprocal synergistic relationships between gut microbiota and metabolites, we constructed a co-occurrence network based on the correlations of the gut bacterial genus and its metabolites in GDM rats (Figure 7). We found that metabolites 5-aminopentanoic acid, alanyl-gamma glutamate, benzyl-gentiobioside L-phenylalanine, and the gut bacterial genera Phascolarctobacterium, Barnesiella, and Blautia were at the hub of the co-occurrence network, whereas other gut bacterial genera and their metabolites were connected with those hub factors (Supplementary Figure 1A).
Lactobacillus and Bifidobacterium Were Positively Correlated With the Gut Metabolites
Lactobacillus rhamnosus LGG and Bifidobacterium lactis Bb12 were used as probiotic supplements in this study. Therefore, we tested the correlations of the Lactobacillus and Bifidobacterium genera with gut metabolites. The Spearman’s correlation coefficient of the OTU of Lactobacillus and Bifidobacterium genera and concentrations of 999 detected gut metabolites were determined. On the basis of r-values of > 0.6 and P-values of < 0.01, the Lactobacillus genus was positively correlated with 90 gut metabolites and the Bifidobacterium genus was positively correlated with 71 gut metabolites. None of the gut metabolite showed a negative correlation with the OTUs of Lactobacillus or Bifidobacterium genera. The correlations of 26 gut metabolites with Lactobacillus and Bifidobacterium genera are shown in a heatmap (Figure 8); these include the amino acid metabolites arginyl-phenylalanine, L-phenylalanine, D-proline, lysyl-valine, valyl-lysine, lysyl-leucine, L-tyrosine, N-α-acetyl-L-arginine, and tryptophyl-arginine. The co-occurrence network also revealed the connections of Lactobacillus and Bifidobacterium probiotic supplements with amino acid metabolism (Supplementary Figure 1B). In addition, other metabolites, such as histamine, oleamide, fenpropimorph, dhurrin, and tyramine, were also correlated with the OTUs of the Lactobacillus or Bifidobacterium genera (Supplementary Figure 1B).
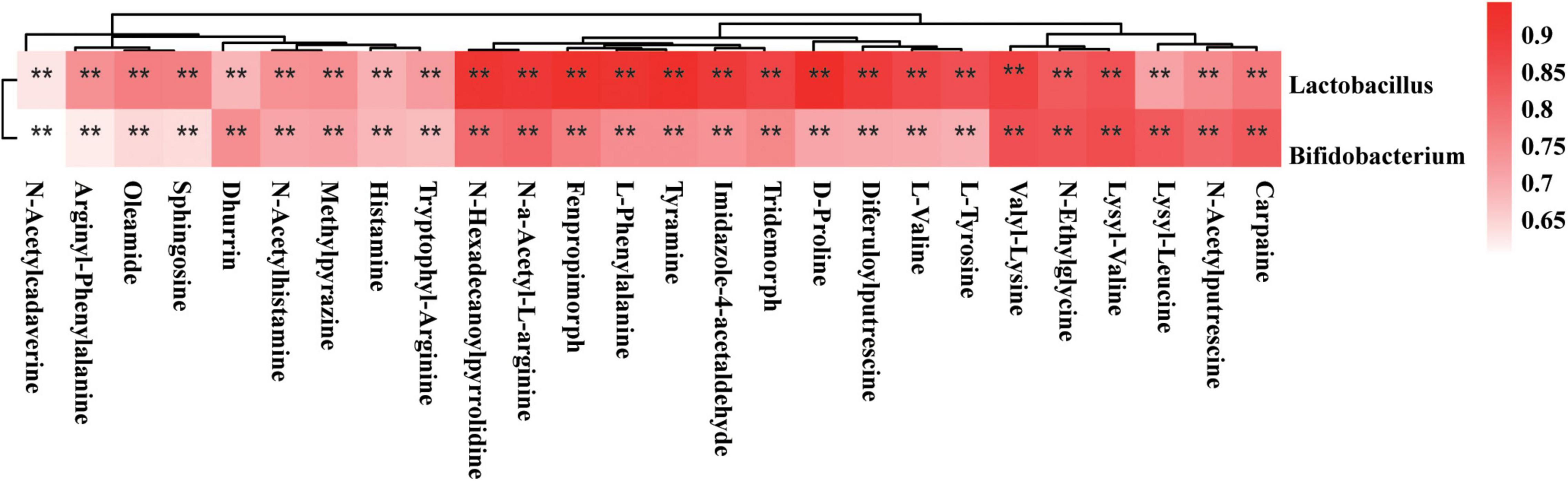
Figure 8. Spearman’s correlation analysis of Lactobacillus and Bifidobacterium genus and metabolites. The r-values were denoted with graduated colors, and red grids indicated positive correlations (*P < 0.05 and **P < 0.01).
Discussion
Probiotics have a mild, stable, and effective influence on controlling blood glucose (Peng et al., 2018; Zhang et al., 2019; Masulli et al., 2020). However, the effects of probiotics on gut metabolites in GDM rats are not clear (Yao et al., 2017). Our results showed that high-dose Lactobacillus and Bifidobacterium probiotic supplements can decrease the concentrations of TNF-α, insulin, and LDL-C and the value of HOMA-IR in GDM rats. After Lactobacillus and Bifidobacterium probiotic intervention, the morphological and structural characteristics of pancreatic and colon tissues were similar to those in the normal pregnant group. In addition, after probiotic supplementation, GDM model rats showed significant changes in gut microbiome composition and gut metabolic profiles. Moreover, the perturbed gut microbiota was strongly associated with changes in gut microbiota-related metabolites, suggesting that probiotic supplements can substantially alter the metabolomic profile of the gut microbiome and adjust host metabolite homeostasis.
Our results from a previous study (Zheng et al., 2021) and this study further verified that L. rhamnosus LGG and B. lactis Bb12 used as probiotic supplements can reduce blood glucose and insulin resistance in GDM rats and also improve the morphological and structural characteristics of their pancreatic and colon tissues. Potential mechanisms of probiotic supplements regulating blood glucose were mainly manifested in regulating the structure of gut microbiota and gut metabolites and then reducing the level of fasting blood glucose and insulin resistance. In addition, insulin resistance has been regarded as a cause of endothelial dysfunction in diabetes (Zhang et al., 2012).
Changes in gut metabolomics are associated with intestinal dysbacteriosis in the development of obesity and metabolic syndrome (Qin et al., 2012; Karlsson et al., 2013). Moreover, metabolic alterations can further aid in the exploration of the pathological mechanisms of GDM. We found that the fecal metabolic profile of GDM rats was significantly different from that of normal pregnant rats. In total, 999 gut metabolites were detected in the feces. Forty-four metabolites in GDM rats had increased concentrations, which were reduced by probiotic supplements, including amino acids, bile acids, and glucuronide. Changes in metabolites in GDM rats were associated with the metabolism signaling pathways of amino acids and bile acids. Metabolites from metabolism signaling pathways of amino acids and bile acids were influenced by probiotic supplements. Changes in metabolites after probiotic supplementation were associated with the metabolism pathways of porphyrin and chlorophyll.
The fecal metabolic profiles of GDM rats were significantly different from those of normal pregnant rats in the metabolism pathways of amino acids and bile acids. The identified amino acids participating in the development of GDM rats included 1-pyrroline, creatinine, cytosine, 2-hydroxypyridine, choline, 2,6-dimethylpyridine, and quinoline. These results are similar to those of previous studies (Wang et al., 2020; Zhao et al., 2020; Lu et al., 2021) that suggest that fecal gut metabolomics of women with GDM was distinct from that of healthy pregnant women, and the levels of amino acids were disrupted in the former. Moreover, the levels of amino acids in early pregnancy are potential biomarkers for predicting subsequent GDM (Jiang et al., 2020). Consistent with previous reports (Lu et al., 2021), we found that, compared to the metabolic profiles of normal pregnant rats, the bile secretion signaling pathways were significantly increased in GDM rats. Both our results and previous studies suggest that bile acids participate in the development of GDM. Serum bile acids in early pregnancy like deoxycholic acid ≤ 0.28 nmol/ml or glycoursodeoxycholic acid ≤ 0.07 nmol/ml were significantly associated with increased risk of GDM (Li et al., 2018). Bile acids play important roles in glucose and lipid metabolism (Vítek and Haluzik, 2016). Furthermore, the relationship between bile acid metabolism and the gut microbiome is mutual (Vítek and Haluzik, 2016). On the one hand, gut microbiota can metabolize bile acids. On the other hand, bile acids can affect the composition of gut microbiota (Vítek and Haluzik, 2016). Our results indicated the relationship between gut microbiota and bile acid and the potential influences of their interaction on host glucose and lipid metabolism in GDM.
A balanced structure of gut microbiota and its metabolites are beneficial to the health of the host; however, the poor interaction between the host and its gut microbiota can lead to metabolic dysfunction (Hills et al., 2019). Disturbances in gut microbiota might contribute to GDM pathogenesis by modulating the metabolism signaling pathways of amino acids and bile acids (Wang et al., 2020). For example, gut microbiota perturbations of the Bacteroides and Bifidobacterium genera displayed strong correlations with amino acid metabolism, and the Bifidobacterium genus was also positively associated with bile acid metabolism. Allobaculum genus displayed strong positive correlations, whereas the Bryobacter and Gemmatimonas genera displayed strong negative correlations with amino acid and bile acid metabolism in GDM rats. Lactobacillus, Bifidobacterium, and Akkermansia are widely used to aid in weight loss and ameliorate metabolic diseases such as obesity and diabetes (Park et al., 2019; Yan et al., 2019; Li et al., 2020). L. rhamnosus LGG and B. lactis Bb12 were used as probiotic supplements to treat GDM model rats in this study. Consistent with the findings from our previous study (Zheng et al., 2021) as well as the present study, a previous randomized controlled trial found that L. rhamnosus HN001 supplementation in early pregnancy decreased GDM prevalence and fasting conjugated bile acids, and these bile acids were positively correlated with fasting glucose and fasting insulin, suggesting that they play important roles in improving the glucose metabolism in pregnant women (Chen et al., 2021).
In summary, 16S RNA sequencing and metabolomic analysis conducted in this study revealed the effect of probiotic supplements (Lactobacillus and Bifidobacterium) on the gut microbiota and gut microbiome of GDM rats and the metabolic status of normal pregnant rats and GDM rats. In addition, this work extended our insights into the relationship among gut microbiota, host metabolism, and GDM rats, pointing to possible future modalities to treat GDM by targeting gut microbiota or gut metabolites. More research is needed to further identify the bacterial species that play a key role in the metabolism of GDM. Overall, this study indicated that probiotic supplementation might be a novel approach to reduce blood glucose and insulin resistance and regulate gut microbiota or gut metabolites in GDM rats. Furthermore, this study provides insights into the applicability of probiotic supplementation in the treatment of GDM.
Data Availability Statement
The raw data supporting the conclusions of this article will be made available by the authors, without undue reservation.
Ethics Statement
All animal procedures were approved by the Fujian University of Traditional Chinese Medicine (Certificate number: SYXK 2019-0007; Ethics approval number: FJTCM IACUC 2020020).
Author Contributions
Q-XZ and H-WW analyzed data, plotted figures, drafted, and revised the manuscript. Q-XZ and X-MJ designed this project and performed coordination. LG revised important scholarly content of our manuscript. Y-TL, X-YJ, P-PH, FC, and X-QC performed the rat experiments, collected, and analyzed samples. All authors contributed to the article and approved the submitted version.
Funding
This work was supported by the Fujian Maternity and Child Health Hospital (YCXMH 20-08) and Joint Funds for the Innovation of Science and Technology, Fujian Province (grant no. 2020Y9133).
Conflict of Interest
The authors declare that the research was conducted in the absence of any commercial or financial relationships that could be construed as a potential conflict of interest.
Publisher’s Note
All claims expressed in this article are solely those of the authors and do not necessarily represent those of their affiliated organizations, or those of the publisher, the editors and the reviewers. Any product that may be evaluated in this article, or claim that may be made by its manufacturer, is not guaranteed or endorsed by the publisher.
Supplementary Material
The Supplementary Material for this article can be found online at: https://www.frontiersin.org/articles/10.3389/fmicb.2022.779314/full#supplementary-material
Supplementary Figure 1 | Reciprocal synergistic relationships of metabolites and gut microbiota in GDM rats. (A) The co-occurrence network of metabolites and gut microbiota. Yellow points represented gut metabolites, and green points represented gut microbiota. Red lines represented positive correlations, and blue lines represented negative correlations. (B) The co-occurrence network of Lactobacillus and Bifidobacterium genus and metabolites. Yellow points represented gut metabolites, and green points represented gut microbiota. Red lines represented positive correlations.
Footnotes
References
Canfora, E. E., Meex, R. C. R., Venema, K., and Blaak, E. E. (2019). Gut microbial metabolites in obesity, NAFLD and T2DM. Nat. Rev. Endocrinol. 15, 261–273. doi: 10.1038/s41574-019-0156-z
Chen, Y., Lu, J., Wickens, K., Stanley, T., Maude, R., Stone, P., et al. (2021). Effect of Lactobacillus rhamnosus Probiotic in Early Pregnancy on Plasma Conjugated Bile Acids in a Randomised Controlled Trial. Nutrients 13:209. doi: 10.3390/nu13010209
de Brito Alves, J. L., de Oliveira, Y., Carvalho, N. N. C., Cavalcante, R. G. S., Pereira Lira, M. M., Nascimento, L. C. P. D., et al. (2019). Gut microbiota and probiotic intervention as a promising therapeutic for pregnant women with cardiometabolic disorders: Present and future directions. Pharmacol Res 145:104252. doi: 10.1016/j.phrs.2019.104252
Gao, C., Sun, X., Lu, L., Liu, F., and Yuan, J. (2019). Prevalence of gestational diabetes mellitus in mainland China: A systematic review and meta-analysis. J. Diabetes Investig. 10, 154–162. doi: 10.1111/jdi.12854
He, J., Chan, T., Hong, X., Zheng, F., Zhu, C., Yin, L., et al. (2020). Microbiome and Metabolome Analyses Reveal the Disruption of Lipid Metabolism in Systemic Lupus Erythematosus. Front. Immunol. 11:1703. doi: 10.3389/fimmu.2020.01703
Hills, R. D. Jr., Pontefract, A. B., Mishcon, R. H., Black, A. C., Sutton, C. S., and Theberge, R. C. (2019). Gut Microbiome: Profound Implications for Diet and Disease. Nutrients 11:1613. doi: 10.3390/nu11071613
Jiang, R., Wu, S., Fang, C., Wang, C., Yang, Y., Liu, C., et al. (2020). Amino acids levels in early pregnancy predict subsequent gestational diabetes. J. Diabetes 12, 503–511. doi: 10.1111/1753-0407.13018
Karlsson, F. H., Tremaroli, V., Nookaew, I., Bergström, G., Behre, C. J., Fagerberg, B., et al. (2013). Gut metagenome in European women with normal, impaired and diabetic glucose control. Nature 498, 99–103. doi: 10.1038/nature12198
Kurtzhals, L. L., Nørgaard, S. K., Secher, A. L., Nichum, V. L., Ronneby, H., Tabor, A., et al. (2018). The impact of restricted gestational weight gain by dietary intervention on fetal growth in women with gestational diabetes mellitus. Diabetologia 61, 2528–2538. doi: 10.1007/s00125-018-4736-6
Li, G. (2019). Preliminary Study on Intestinal Microflora and Metabolomics in Gestational Diabetes Mellitus. [Ph.D thesis]. (Zhengzhou, IL: Zhengzhou University).
Li, J., Huo, X., Cao, Y. F., Li, S. N., Du, Z., Shao, P., et al. (2018). Bile acid metabolites in early pregnancy and risk of gestational diabetes in Chinese women: A nested case-control study. EBioMedicine 35, 317–324. doi: 10.1016/j.ebiom.2018.08.015
Li, X. P., Huang, Y. M., Song, L. Q., Xiao, Y. C., Lu, S., Xu, J. G., et al. (2020). Lactobacillus plantarum prevents obesity via modulation of gut microbiota and metabolites in high-fat feeding mice. J. Funct. Foods 73:104103. doi: 10.1016/j.jff.2020.104103
Liu, R., Hong, J., Xu, X., Feng, Q., Zhang, D., Gu, Y., et al. (2017). Gut microbiome and serum metabolome alterations in obesity and after weight-loss intervention. Nat. Med. 23, 859–868. doi: 10.1038/nm.4358
Lu, W., Luo, M., Fang, X., Zhang, R., Li, S., Tang, M., et al. (2021). Discovery of metabolic biomarkers for gestational diabetes mellitus in a Chinese population. Nutr. Metab. 18:79. doi: 10.1186/s12986-021-00606-8
Masulli, M., Vitacolonna, E., Fraticelli, F., Della Pepa, G., Mannucci, E., and Monami, M. (2020). Effects of probiotic supplementation during pregnancy on metabolic outcomes: A systematic review and meta-analysis of randomized controlled trials. Diabetes Res. Clin. Pract. 162:108111. doi: 10.1016/j.diabres.2020.108111
Olson, C. A., Vuong, H. E., Yano, J. M., Liang, Q. Y., Nusbaum, D. J., and Hsiao, E. Y. (2018). The Gut Microbiota Mediates the Anti-Seizure Effects of the Ketogenic Diet. Cell 173, 1728–1741.e13. doi: 10.1016/j.cell.2018.04.027
Ovesen, P. G., Fuglsang, J., Andersen, M. B., Wolff, C., Petersen, O. B., and David, et al. (2018). Temporal Trends in Gestational Diabetes Prevalence, Treatment, and Outcomes at Aarhus University Hospital, Skejby, between 2004 and 2016. J. Diabetes Res. 2018:5937059. doi: 10.1155/2018/5937059
Pan, Y., Zheng, Q., Jiang, X., Chen, X., Zhang, X., and Wu, J. (2021). Probiotic Supplements Improve Blood Glucose and Insulin Resistance/Sensitivity among Healthy and GDM Pregnant Women: A Systematic Review and Meta-Analysis of Randomized Controlled Trials. Evid. Based Complement. Alternat. Med. 2021:9830200. doi: 10.1155/2021/9830200
Park, S. S., Lee, Y. J., Kang, H., Yang, G., Hong, E. J., Lim, J. Y., et al. (2019). Lactobacillus amylovorus KU4 ameliorates diet-induced obesity in mice by promoting adipose browning through PPARγ signaling. Sci. Rep. 9:20152. doi: 10.1038/s41598-019-56817-w
Peng, T. R., Wu, T. W., and Chao, Y. C. (2018). Effect of Probiotics on the Glucose Levels of Pregnant Women: A Meta-Analysis of Randomized Controlled Trials. Medicina 54:77. doi: 10.3390/medicina54050077
Ponnusamy, S., Diabetes in Pregnancy Working Group, Maternal Medicine Clinical Study Group, and Royal College of Obstetricians and Gynaecologists (2020). Gestational diabetes: opportunities for improving maternal and child health. Lancet Diabetes Endocrinol. 8, 793–800. doi: 10.1016/s2213-8587(20)30161-3
Qin, J., Li, Y., Cai, Z., Li, S., Zhu, J., Zhang, F., et al. (2012). A metagenome-wide association study of gut microbiota in type 2 diabetes. Nature 490, 55–60. doi: 10.1038/nature11450
Rasmussen, L., Poulsen, C. W., Kampmann, U., Smedegaard, S. B., Ovesen, P. G., and Fuglsang, J. (2020). Diet and Healthy Lifestyle in the Management of Gestational Diabetes Mellitus. Nutrients 12:3050. doi: 10.3390/nu12103050
Veeraswamy, S., Vijayam, B., Gupta, V. K., and Kapur, A. (2012). Gestational diabetes: the public health relevance and approach. Diabetes Res. Clin. Pract. 97, 350–358. doi: 10.1016/j.diabres.2012.04.024
Vítek, L., and Haluzik, M. (2016). The role of bile acids in metabolic regulation. J. Endocrinol. 228, R85–R96. doi: 10.1530/JOE-15-0469
Wang, X., Liu, H., Li, Y., Huang, S., Zhang, L., Cao, C., et al. (2020). Altered gut bacterial and metabolic signatures and their interaction in gestational diabetes mellitus. Gut. Microbes. 12, 1–13. doi: 10.1080/19490976.2020.1840765
Yan, F., Li, N., Shi, J., Li, H., Yue, Y., Jiao, W., et al. (2019). Lactobacillus acidophilus alleviates type 2 diabetes by regulating hepatic glucose, lipid metabolism and gut microbiota in mice. Food Funct. 10, 5804–5815. doi: 10.1039/c9fo01062a
Yao, K., Zeng, L., He, Q., Wang, W., Lei, J., and Zou, X. (2017). Effect of Probiotics on Glucose and Lipid Metabolism in Type 2 Diabetes Mellitus: A Meta-Analysis of 12 Randomized Controlled Trials. Med. Sci. Monit. 23, 3044–3053. doi: 10.12659/msm.902600
Zhang, H., Dellsperger, K. C., and Zhang, C. (2012). The link between metabolic abnormalities and endothelial dysfunction in type 2 diabetes: an update. Basic Res. Cardiol. 107:237. doi: 10.1007/s00395-011-0237-1
Zhang, J., Ma, S., Wu, S., Guo, C., Long, S., and Tan, H. (2019). Effects of Probiotic Supplement in Pregnant Women with Gestational Diabetes Mellitus: A Systematic Review and Meta-Analysis of Randomized Controlled Trials. J. Diabetes Res. 2019:5364730. doi: 10.1155/2019/5364730
Zhao, C., Ge, J., Li, X., Jiao, R., Li, Y., Quan, H., et al. (2020). Integrated metabolome analysis reveals novel connections between maternal fecal metabolome and the neonatal blood metabolome in women with gestational diabetes mellitus. Sci. Rep. 10:3660. doi: 10.1038/s41598-020-60540-2
Keywords: gut metabolites, gestational diabetes mellitus rats, probiotic supplements, glucose and insulin resistance, host metabolism
Citation: Zheng Q-X, Wang H-W, Jiang X-M, Ge L, Lai Y-T, Jiang X-Y, Huang P-P, Chen F and Chen X-Q (2022) Changes in the Gut Metabolic Profile of Gestational Diabetes Mellitus Rats Following Probiotic Supplementation. Front. Microbiol. 13:779314. doi: 10.3389/fmicb.2022.779314
Received: 18 September 2021; Accepted: 11 February 2022;
Published: 08 April 2022.
Edited by:
M. Pilar Francino, Fundación para el Fomento de la Investigación Sanitaria y Biomédica de la Comunitat Valenciana (FISABIO), SpainReviewed by:
Ravinder K. Nagpal, Florida State University, United StatesZhentian Lei, University of Missouri, United States
Mengxia Wang, Sun Yat-sen University, China
Copyright © 2022 Zheng, Wang, Jiang, Ge, Lai, Jiang, Huang, Chen and Chen. This is an open-access article distributed under the terms of the Creative Commons Attribution License (CC BY). The use, distribution or reproduction in other forums is permitted, provided the original author(s) and the copyright owner(s) are credited and that the original publication in this journal is cited, in accordance with accepted academic practice. No use, distribution or reproduction is permitted which does not comply with these terms.
*Correspondence: Xiu-Min Jiang, jzc0427@163.com
†These authors have contributed equally to this work and share first authorship