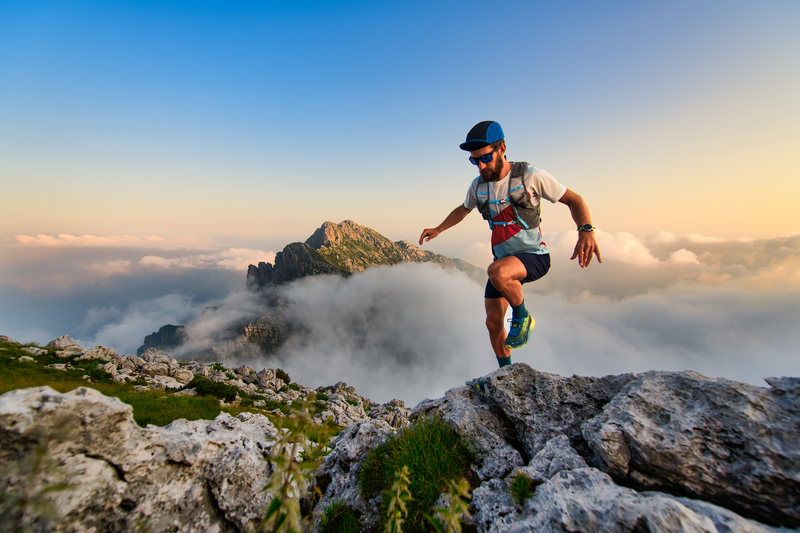
94% of researchers rate our articles as excellent or good
Learn more about the work of our research integrity team to safeguard the quality of each article we publish.
Find out more
ORIGINAL RESEARCH article
Front. Microbiol. , 25 January 2022
Sec. Microbe and Virus Interactions with Plants
Volume 13 - 2022 | https://doi.org/10.3389/fmicb.2022.778927
This article is part of the Research Topic Population and Comparative Genomics of Plant Pathogenic Bacteria View all 16 articles
Dickeya species are causal agents of soft rot diseases in many economically important crops, including soft rot disease of potato (Solanum tuberosum). Using random barcode transposon-site sequencing (RB-TnSeq), we generated genome-wide mutant fitness profiles of Dickeya dadantii 3937, Dickeya dianthicola ME23, and Dickeya dianthicola 67-19 isolates collected after passage through several in vitro and in vivo conditions. Though all three strains are pathogenic on potato, D. dadantii 3937 is a well-characterized model while D. dianthicola strains ME23 and 67-19 are recent isolates. Strain ME23 specifically was identified as a representative strain from a 2014 outbreak on potato. This study generated comparable gene fitness measurements across ecologically relevant conditions for both model and non-model strains. Tubers from the potato cultivars “Atlantic,” “Dark Red Norland,” and “Upstate Abundance” provided highly similar conditions for bacterial growth. Using the homolog detection software PyParanoid, we matched fitness values for orthologous genes in the three bacterial strains. Direct comparison of fitness among the strains highlighted shared and variable traits important for growth. Bacterial growth in minimal medium required many metabolic traits that were also essential for competitive growth in planta, such as amino acid, carbohydrate, and nucleotide biosynthesis. Growth in tubers specifically required the pectin degradation gene kduD. Disruption in three putative DNA-binding proteins had strain-specific effects on competitive fitness in tubers. Though the Soft Rot Pectobacteriaceae can cause disease with little host specificity, it remains to be seen the extent to which strain-level variation impacts virulence.
The Soft Rot Pectobacteriaceae comprise Dickeya and Pectobacterium species that are the causal agents of bacterial soft rot diseases on economically important vegetables and ornamentals (Adeolu et al., 2016; Motyka et al., 2017). These necrotrophic pathogens rely on numerous traits to survive the various stress conditions encountered in the host, including oxidative stress, osmotic stress, iron starvation, and toxic compounds (Jiang et al., 2016; Reverchon et al., 2016). During initial plant colonization, cells encounter a slightly acidic apoplast (pH 5.0–6.5) or tuber (pH 5.5–6.2), and pH levels increase to slightly basic (pH 8) by the late stage of infection (Grignon and Sentenac, 1991; Nachin and Barras, 2000; Kiszonas and Bamberg, 2010). At high cell densities, the production of plant cell wall degrading enzymes is induced, leading to the maceration of plant tissues and the formation of visible symptoms (Reverchon et al., 2016). In response to these changing conditions, cells must respond to environmental cues to adapt global expression of stress response genes and virulence factors (Jiang et al., 2016; Raoul des Essarts et al., 2019).
The taxonomy of the Dickeya genus has undergone substantial revision with the addition of novel species D. solani, D. aquatica, and D. fangzhongdai (Samson et al., 2005; Parkinson et al., 2014; Van Der Wolf et al., 2014; Tian et al., 2016). However, an increase in available whole-genome sequence data has improved species-level identification based on pairwise average nucleotide identity (ANI), in silico DNA-DNA hybridization (isDDH), and core genome multilocus sequence analysis (MLSA) (Zhang et al., 2016). There is little known about host-specific traits, as these species generally have broad host ranges (Van Gijsegem et al., 2021). In addition, there are no known resistance genes for potato soft rot, and it is therefore impossible to predict cultivar resistance without testing (Lyon, 1989; Czajkowski et al., 2011; Chung et al., 2013). Without gene-for-gene resistance, potato cultivar tolerance is reliant on physical barriers and antimicrobial small molecules such as phenolics or the phytoalexin rishitin (Lyon, 1989). An alternative strategy being explored is the use of bacteriophage-based biocontrol for potato plants and tubers, particularly of the highly virulent D. solani (Adriaenssens et al., 2012; Czajkowski et al., 2017). Dickeya virulence factors and transcriptional regulators of virulence genes are generally conserved. Studies in D. solani have suggested a closed pangenome with many conserved virulence factors and transcriptional regulators (Golanowska et al., 2018; Motyka-Pomagruk et al., 2020). However, virulence regulon differences indicate some virulence genes could have differential expression among strains (Golanowska et al., 2018). Pangenomic analysis of D. dianthicola also reflects a closed pangenome, though almost all sequenced strains were originally isolated from potato (Ge et al., 2021c).
Transposon mutagenesis followed by high-throughput sequencing (TnSeq) is a valuable screening tool to identify genes important for growth in a given condition. Gene fitness is functionally defined as the change in relative mutant abundance within a population and is a quantitative measure of growth rate (van Opijnen et al., 2009). TnSeq has been used to identify D. dadantii genes important for growth in chicory (Royet et al., 2019). This work identified several metabolic pathways essential for in planta growth, primarily those involved in biosynthesis of nucleotides, amino acids, and some vitamins (Royet et al., 2019). A modification of TnSeq to add 20-nucleotide DNA “barcodes” to transposon donor plasmids, known as random barcode transposon-site sequencing (RB-TnSeq) enables highly scalable TnSeq assays (Wetmore et al., 2015). This method has been applied to over 44 bacterial strains to date (Price et al., 2018), including plant pathogenic Pseudomonas spp. and Ralstonia spp. (Cole et al., 2017; Helmann et al., 2019; Georgoulis et al., 2021). A motivating factor for this study was to demonstrate the value of RB-TnSeq to characterize necrotrophic plant pathogenic bacteria in planta; using both an established model strain as well as recently isolated and uncharacterized strains (Liu and Deutschbauer, 2018).
To identify bacterial traits important for growth in potato (Solanum tuberosum) tubers, we examined three strains across two species: D. dadantii 3937 (Dda3937), D. dianthicola ME23 (DdiaME23), and D. dianthicola 67-19 (Ddia6719). While these three strains are all pathogenic on potato, Dda3937 was originally isolated from Saintpaulia ionantha (Lemattre and Narcy, 1972), and Ddia6719 was recently isolated from New Guinea impatiens (Impatiens hawkeri) (Liu et al., 2020a,b). Dda3937 has been a model strain used for molecular studies since its isolation in 1972 (Lemattre and Narcy, 1972), while DdiaME23 was isolated as a representative strain for a 2014 potato disease outbreak (Ma et al., 2019). Pairwise ANI between Dda3937 and DdiaME23 is 92.8% (Chen et al., 2019). Using barcoded transposon insertion mutant libraries in these three strains, we screened for genes that contributed to competitive fitness during growth in vitro and in potato tubers. By leveraging RB-TnSeq in a shared susceptible host for D. dadantii and D. dianthicola, we aimed to identify common and unique virulence factors among representative strains for these two species.
Gene ortholog groups were generated using the PyParanoid analysis pipeline v0.4.1 (Melnyk et al., 2019). Peptide sequences from the following RefSeq genome assemblies were used to construct ortholog groups: GCF_000147055.1 (Dda3937), GCF_003403135.1 (DdiaME23), and GCF_014893095.1 (Ddia6719). From these assemblies, RefSeq gene loci were then matched to their corresponding protein names to allow comparison to the Barcode Sequencing (BarSeq) fitness data. Additionally, Clusters of Orthologous Groups (COG) categories for Dda3937 were downloaded from the IMG database (Chen et al., 2019), GenBank gene names were replaced with their corresponding RefSeq names, and added to this ortholog table, allowing putative COG assignments for orthologous genes in D. dianthicola strains.
Strains used in this study are described in Supplementary Table 1. All bacteria were cultured in Luria-Bertani (LB) medium (10 g tryptone, 5 g yeast extract, and 10 g NaCl per 1 L) (Bertani, 1951) at 28°C, except for pure culture E. coli grown at 37°C. When noted, kanamycin was used at a final concentration of 50 μg/ml. Barcoded transposon libraries were constructed by conjugating the barcoded mariner transposon plasmid pKMW3 from the E. coli WM3064 donor library APA752 (Wetmore et al., 2015) into wild-type Dda3937, DiaME23, and Ddia6719. Recipient Dickeya strains were grown as 3 ml LB liquid cultures overnight and 1.5 ml of each culture was subcultured into 30 ml LB without antibiotics. An entire 1 ml freezer aliquot of the E. coli donor library was thawed and used to inoculate 25 ml LB containing 300 μM diaminopimelic acid (DAP) (Sigma-Aldrich, United States) and kanamycin. All cultures were grown to OD600 of approximately 1.0, washed with 10 mM KPO4, and combined in equal amounts before plating donor-recipient pairs each on 50 LB plates containing 300 μM DAP. Conjugations were incubated at 28°C overnight, and exconjugants were then scraped into 40 ml 10 mM KPO4. This conjugation mixture was then vortexed, spread onto 200 LB kanamycin plates per strain, and incubated at 28°C for 3 days. All colonies were resuspended in 200 ml LB with kanamycin, diluted back to a starting OD600 of 0.2, and grown at 28°C with shaking for 6–8 h, to a concentration of approximately 109 CFU/ml (measured as a 1/10 dilution at OD600 = 0.15–0.3). Glycerol was added to the library to a final concentration of 15%, and 1 ml aliquots were frozen at –80°C.
For DNA library preparation, genomic DNA from each library was purified from an entire 1 ml cell pellet using the Monarch Genomic DNA Purification Kit (New England Biolabs, United States). Samples were eluted in 50 μl nuclease-free water. Purified DNA was quantified on a NanoDrop One (Thermo Fischer Scientific, United States), and 500 ng DNA was used as input for the NEBNext Ultra II FS DNA Library Prep kit (New England Biolabs, United States), following the manufacturer’s instructions with modifications as follows. For enzymatic DNA fragmentation, a 12-min incubation time was used. DNA fragments were size selected using AMPure XP magnetic beads (Beckman Coulter, United States) at the recommended ratios 0.4X and 0.2X. We used a modified version of the protocol described in Wetmore et al. (2015), with a two-step PCR used to enrich for transposon insertion sites, based on (Rubin et al., 2021). A custom splinkerette adapter was ligated to fragmented DNA, prepared by annealing oligos:/5Phos/G*ATCGGAAGAGCACACGTCTGGGTTTTTT TTTTCAAAAAAA*A and G*AGATCGGTCTCGGCATTCCC AGACGTGTGCTCTTCCGATC*T (Rubin et al., 2021). Between rounds of PCR and before submitting for sequencing, DNA was cleaned by binding to AMPure XP magnetic beads, using a bead ratio of 0.9X and eluted in 15 μl 0.1X TE buffer for intermediate steps and 30 μl 0.1X TE for sequencing. Finally, the sequencing library was quantified using a Qubit dsDNA HS assay kit (Thermo Fischer Scientific, United States). DNA libraries were submitted for sequencing at the Biotechnology Resource Center (BRC) Genomics Facility at the Cornell Institute of Biotechnology on an Illumina MiSeq to check library quality, followed by sequencing on a NextSeq 500 (Illumina, Inc., United States). All mapping used single-end sequencing for 150 bp fragments.
Sequence data were analyzed using the scripts from the FEBA package v1.3.1 (Wetmore et al., 2015). MapTnSeq.pl was used to identify the barcode and location in the genome of each read with identifiable transposon sequence from both MiSeq and NextSeq reads, based on the “model_pKMW3.2” sequence. DesignRandomPool.pl was used to assemble the mutant pool using barcodes seen in a single location 10 or more times. All TnSeq mapping and BarSeq fitness calculation code is available at http://bitbucket.org/berkeleylab/feba/ (Wetmore et al., 2015). Mapping scripts were run on a Cornell University BioHPC Cloud 40-core Linux (CentOS 7.6). server with 256 GB RAM.
Using the output from MapTnSeq.pl, gene essentiality predictions were made using https://bitbucket.org/berkeleylab/feba/src/master/bin/Essentiality.pl and the function “Essentials” from https://bitbucket.org/berkeleylab/feba/src/master/lib/comb.R (Wetmore et al., 2015). Using the median insertion density and the median length of genes > 100 bp, this method calculates how short a gene can be and still be unlikely to have no insertions by chance (P < 0.02, Poisson distribution); genes shorter than this threshold are then excluded (Price et al., 2018). For the Dickeya strains examined here, the minimum gene length for a gene to be predicted as essential for growth in LB was 175 bp (Dda3937) or 150 bp (DdiaME23 and Ddia6719). Protein-coding genes are then considered to be essential or nearly essential if there are no fitness values and the normalized central insertion density score and normalized read density score as computed by the FEBA package were < 0.2 (Price et al., 2018).
For a given BarSeq experiment, a single transposon library freezer aliquot was thawed and recovered in 25 ml LB containing kanamycin at 28°C until OD600 = 0.5–0.7, approximately 6–8 h. At this point, two 1 ml cell pellets were frozen as time0 measurements, and the remaining culture was washed in 10 mM KPO4 and used to inoculate experimental samples.
All in vitro cultures were grown in 1 ml volumes in 24-well plates. In each well, 50 μl starter culture at 0.3 OD600 was added to 950 μl medium containing kanamycin. Media tested were LB, Potato Dextrose Broth (PDB)(pH 5) (Sigma-Aldrich, United States), and M9 minimal medium (M9) as described in M9 Minimal Medium (Standard) (2010) but containing 0.4% glycerol instead of 0.4% glucose. Plates were incubated at 28°C with shaking at 200 rpm. After 1 day (LB and PDB) or 2 days (M9), each 1 ml sample was pelleted and frozen prior to genomic DNA extraction.
Prior to inoculation, all tubers were rinsed and then surface sterilized by submerging in 70% ethanol for 10 min, followed by two washes with distilled water. Inoculum was standardized to 109 CFU/ml by measuring a 1/10 culture dilution at OD600 = 0.3 (corresponding to 108 CFU/ml), and 10 μl was inoculated in two replicate stab wounds created by pushing a 200 μl pipet tip roughly 3 mm into each tuber. Six replicate tubers were used for each bacterial strain and potato cultivar. Inoculated tubers were stored in plastic bags at 28°C. Two days after inoculation, ∼2 cm length cores were taken at each site of inoculation using a 1 cm diameter cork borer. Duplicate cores from each tuber were pooled in 8 ml 10 mM KPO4 and shaken at 200 rpm at 28°C for 10 min. For each sample, 2 ml of bacterial suspension were pelleted and frozen prior to DNA extraction.
Genomic DNA was purified from cell pellets using the Monarch Genomic DNA Purification Kit (New England Biolabs, United States). Purified DNA samples were eluted in 30 μl nuclease-free water and quantified on a Nanodrop One (Thermo Fischer Scientific, United States). After gDNA extraction, the 98°C BarSeq PCR as described in Wetmore et al. (2015) was used to specifically amplify the barcode region of each sample. The PCR for each sample was performed in 50 μl total volume: containing 0.5 μl Q5 High-Fidelity DNA polymerase (New England Biolabs, United States), 10 μl 5X Q5 buffer, 10 μl 5X GC enhancer, 1 μl 10 mM dNTPs, 150–200 ng template gDNA, 2.5 μl common reverse primer (BarSeq_P1), and 2.5 μl of forward primer from one of the 96 indexed forward primers (BarSeq_P2_ITXXX), both at 10 μM (Wetmore et al., 2015). Following the BarSeq PCR, 10 μl of each reaction was pooled (46–49 samples per pool), and 200 μl of this DNA pool was subsampled and purified using the DNA Clean and Concentrator Kit (Zymo Research, United States). The final DNA sequencing library was eluted in 30 μl nuclease-free water, quantified on a Nanodrop One, and submitted for sequencing at the BRC Genomics Facility at the Cornell Institute of Biotechnology. Prior to sequencing, the quality of each amplicon pool was assessed using a Bioanalyzer. Each sequencing pool was run on a single NextSeq 500 (Illumina, Inc., United States) lane for 75 bp single-end reads.
Sequencing reads were used to calculate genome-wide gene fitness using the FEBA scripts MultiCodes.pl, combineBarSeq.pl, and BarSeqR.pl (Wetmore et al., 2015). Scripts to calculate gene fitness values were run on a Cornell University BioHPC Cloud 40-core Linux (CentOS 7.6). Server with 256 GB RAM. From the raw fastq reads for each sample, individual barcode sequences were identified and counted using MultiCodes.pl, and these counts were combined across samples for a given transposon library using combineBarSeq.pl. Using BarSeqR.pl, fitness values for each insertion strain were calculated as the log2 ratio of barcode abundance following library growth under a given experimental condition divided by the abundance in the time0 sample. The fitness of each gene is the weighted average of strain fitness values based on the “central” transposon insertions only, i.e., those within the central 10–90% of a gene. Barcode counts were summed between replicate time0 samples. For analysis, genes were required to have at least 30 reads per gene in the time0 sample, and 3 reads per individual strain (Wetmore et al., 2015). Gene fitness values were normalized across the chromosome so that the median gene fitness value was 0. All experiments described here passed previously described quality control metrics (Wetmore et al., 2015; Price et al., 2018).
We focused on genes having fitness values > 1 or < –1 and absolute t-like test statistic > 4. This t score is an estimate of the reliability of the fitness measurement for a gene, and is equal to the fitness value divided by the square root of the maximum variance calculated in two ways (Wetmore et al., 2015). With these cutoffs, we also calculated gene fitness values comparing replicate time0 samples (Price et al., 2018; Liu et al., 2021). Across 6 (each Dda3937 and DdiaME23) and 2 (Ddia6719) replicate time0 samples, 0 gene fitness values had fitness > 1 or < –1 and absolute t > 4. Data were analyzed in R v4.0.3 (R Core Team, 2017) and visualized using the package ggplot2 v3.3.5 (Wickham, 2016). The principal components analysis was performed on the gene fitness matrix for each Dickeya strain using the R function prcomp, which performs centered singular value decomposition.
All raw Illumina reads used for mapping and fitness assays have been deposited in the Sequence Read Archive under BioProject accession number PRJNA692477. Individual sample accession numbers are listed in Supplementary Table 2. Annotated scripts used for computational analysis are available at http://github.com/tylerhelmann/dickeya-barseq-2021. Experimental fitness values are publicly available on the interactive Fitness Browser at http://fit.genomics.lbl.gov.
To enable direct comparison of gene fitness measurements between strains, we constructed a database of homologous gene families using the PyParanoid analysis pipeline (Melnyk et al., 2019). Based on clustering of all predicted protein sequences from Dda3937, DdiaME23, and Ddia6719, 3,821 total homolog groups were identified, representing 88.1% of the total input sequences. Of these, 3,310 groups contained single-copy genes in all three strains. For each group, gene loci, protein identifiers, and gene descriptions are listed in Supplementary Table 3. This table also contains COG assignments matched from the Dda3937 IMG genomic annotation (Chen et al., 2019).
To measure contributions of individual genes to fitness, we constructed barcoded transposon mutant libraries in the Dickeya strains using a barcoded mariner E. coli donor library (Wetmore et al., 2015). These libraries ranged in size from 334,893 to 541,278 mapped genomic insertional strains, with 37–62 median strains per hit gene (Table 1). Of the three strains tested, only one gene in the Ddia6719 genome did not contain any TA dinucleotide sites and was therefore inaccessible to the mariner transposon. Mapped insertions were evenly distributed across the chromosome of each strain (Supplementary Figure 1).
Based on analysis of the TnSeq mapping data, essential genes were predicted using the FEBA RB-TnSeq analysis pipeline (Wetmore et al., 2015; Price et al., 2018). We identified 374–426 genes per strain that are likely to encode essential or near-essential genes for growth in LB (Supplementary Table 4). Using the ortholog group assignments for these genes, 316 of these predicted essential genes (74–84%) are shared among all three strains (Supplementary Figure 2). Most predicted essential genes are in the functional categories of “Translation, ribosomal structure, and biogenesis,” “Cell wall/membrane/envelope biogenesis,” “Coenzyme transport and metabolism,” “Energy production and conversions,” and “Replication, recombination, and repair” (Supplementary Table 5).
To generate genome-wide gene fitness values for the barcoded transposon libraries, each strain was grown in the rich media LB and Potato Dextrose Broth (PDB) as well as M9 minimal medium supplemented with 0.4% glycerol (Supplementary Figure 3). Strain fitness values were calculated as a log2 ratio of barcode abundance following sample growth with barcode abundance measured in the time0 duplicate samples. Gene fitness is the weighted average of individual strain fitness values (Wetmore et al., 2015). For fitness calculations, insertions in the first and last 10% of coding regions were excluded, with insertions in the remaining 80% of the gene considered “central.” While 91–92% of genes in all strains contained centrally mapped insertions, not all genes were used in fitness calculations due to low read or insertion abundance. We focused our analysis on genes with fitness values > 1 or < –1, and absolute t-score > 4 (Supplementary Table 6). Across all conditions, we calculated fitness values for 3,705 (Dda3937), 3,761 (DdiaME23), and 3,528 (Ddia6719) genes, representing 88, 90, and 86% of the total genes in each strain, respectively.
Principal component analysis showed gene fitness values of the three tuber conditions overlapped (Figure 1), and so these samples were jointly considered as a single “Tuber” condition for some subsequent analyses.
Figure 1. PCA of experimental samples based on fitness values calculated for D. dadantii 3937, D. dianthicola ME23, and D. dianthicola 67–19. Available fitness values for each sample, respectively: N = 3,705; 3,761; 3,528. Superimposed ellipses are based on a multivariate t-distribution.
As the libraries were constructed on LB medium, relatively few mutations deleterious in LB were maintained in the populations (Figure 2). Figure 3 presents these data split by COG category. Limited mutations in genes categorized as “cell wall/membrane/envelope biogenesis” (mdoGH) and “cell cycle control, cell division, chromosome partitioning” (ftsX) were present in the mapped populations but generally detrimental in LB for all three strains (Supplementary Figure 4). Even in LB, some variation was apparent between strains, such as disruptions in the gene encoding the cell division protein ZapB which decreased competitive fitness in Dda3937 but not DdiaME23 or Ddia6719 (Supplementary Figure 4).
Figure 2. Number of unique genes for each condition with fitness values of < –1 or > 1, and absolute t-like test statistic > 4 in at least one replicate sample.
Figure 3. Number of unique genes for each condition with fitness values of < –1 or > 1, and absolute t-like test statistic > 4 in at least one replicate sample. Genes where fit < –1 are show below the line y = 0, while genes where fit > 1 are shown above. COG assignments are based on the D. dadantii 3937 annotation in the IMG database (Chen et al., 2019), and extrapolated to D. dianthicola ME23 and D. dianthicola 67-19 based on PyParanoid-generated ortholog groups.
The rich medium PDB provided a very different gene fitness profile than LB, likely due to nutritional differences and its slightly acidic nature (pH 5). For all three strains, more genes were detrimental (fit > 1) and beneficial (fit < −1) in PDB relative to LB (Figure 2). Genes in diverse metabolic categories contributed to competitive fitness, including “amino acid transport and metabolism,” “carbohydrate transport and metabolism,” “cell wall/membrane/envelope biogenesis,” “coenzyme transport and metabolism,” “inorganic ion transport and metabolism,” “nucleotide transport and metabolism,” “signal transduction mechanisms,” “transcription,” and “translation, ribosomal structure, and biogenesis” (Figure 3). For example, in all three strains oligopeptidase A and the low affinity potassium transporter Kup were specifically important in PDB for growth (Supplementary Figure 5). Disruptions in the two-component system RtsAB were specifically beneficial for Dda3937 in PDB, as were disruptions in the zinc uptake transcriptional repressor Zur (Supplementary Figure 5). Though LB and PDB are both complex rich media, pH and/or specific available nutrients differed enough to clearly separate the gene fitness profiles for Dda3937 and Ddia6719, though not for DdiaME23 (Figure 1).
In the minimal medium M9 containing glycerol as a carbon source, important genes included categories such as “amino acid transport and metabolism,” “carbohydrate transport and metabolism,” “coenzyme transport and metabolism,” and “nucleotide transport and metabolism.” While many amino acids were limiting in both M9 and tuber samples, arginine biosynthetic genes (argCEFGH) were uniquely important in M9, suggesting the presence of available arginine in tubers (Figure 4). Conversely, mutations in many “cell motility” genes had a large positive effect, though this effect was often limited to Dda3937 (Figure 5). This is indicative of the limited energy available in minimal medium, and the high energy cost of motility.
Figure 4. Mutations in arginine biosynthetic genes are detrimental only in M9 minimal medium. Gene fitness values for argininosuccinate synthase ArgG (group 00579), argininosuccinate lyase ArgH (group 00633), acetylornithine deacetylase ArgE (group 00844), N-acetyl-gamma-glutamyl-phosphate reductase ArgC (group 01191), and ornithine carbamoyltransferase ArgF (group 01248).
Figure 5. Mutations in flagellar-associated genes increase competitive fitness of D. dadantii 3937 in M9 minimal medium. Gene fitness values for flagellar biosynthesis protein FlhA (group 00200), flagellar hook-associated protein FlgK (group 00303), flagellar motor protein MotB (group 001162), and RNA polymerase sigma factor FliA (group 02116).
To calculate genome-wide gene fitness values in an ecologically and economically relevant condition, we inoculated the transposon libraries into tubers of three potato cultivars: “Atlantic,” “Dark Red Norland,” and “Upstate Abundance.” As each transposon library contains over 300,000 unique strains, we inoculated approximately 107 cells into each tuber (10 μl of a 109 CFU/ml solution). After 2 days incubation at high humidity, we recovered cells by streaming for barcode sequencing and calculation of gene fitness values. Many genes involved in amino acid biosynthesis that were important for growth in M9 were also important in tubers (leuAC, thrC, serB), highlighting potentially limiting factors for growth during potato soft rot (Figure 6).
Figure 6. Amino acid biosynthetic genes important in M9 minimal medium as well as growth in potato tubers. Gene fitness values for 2-isopropylmalate synthase LeuA (group 00407), 3-isopropylmalate dehydratase large subunit LeuC (group 00578), threonine synthase ThrC (group 00763), and phosphoserine phosphatase SerB (group 01340).
The pectin degradation protein 2-dehydro-3-deoxy-D-gluconate 5-dehydrogenase KduD was specifically important for growth in tubers (Figure 7). Interestingly, we identified several putative DNA-binding or helix-turn-helix transcriptional regulators where mutant strains had strain-specific increased fitness in tubers (Figure 7). Insertions in the Ddia6719 helix-turn-helix transcriptional regulator HGI48_RS01985 increased fitness in tubers, while insertions in the paralog HGI48_RS02000 had no effect on fitness. There is no ortholog for this gene in Dda3937, and the ortholog in DdiaME23 had no disruption phenotype in any condition tested (Figure 7).
Figure 7. In tubers, pectin degradation contributes to growth, while the role of putative DNA-binding proteins varies among strains. Pectin degradation by 2-dehydro-3-deoxy-D-gluconate 5-dehydrogenase KduD (group 01938) is specifically important for growth in tubers. Gene fitness values shown for three putative DNA binding proteins: ortholog groups 02970, 03140, and 03332. No ortholog was detected in D. dadantii 3937 for group 03332 (helix-turn-helix transcriptional regulators). In D. dianthicola 67-19, there are two genes in group 03332 (HGI48_RS01985, triangles; HGI48_RS02000, circles). All other orthogroup members are single-copy in each strain.
The soft rot infection process is complex, requiring tolerance of stress conditions and coordination of disease-stage specific virulence traits (Jiang et al., 2016; Reverchon et al., 2016). Despite taxonomic and diagnostic improvements within the soft rot Pectobacteriaceae, our molecular understanding of these pathogens remains highly reliant on the model strain D. dadantii (previously Erwinia chrysanthemi) 3937 (Toth et al., 2011; Van Gijsegem et al., 2021). However, D. dianthicola strains have been among those more recently responsible for outbreaks in the United States and globally (Toth et al., 2011; van der Wolf et al., 2021). It is therefore important to consider common and variable virulence traits of Dickeya species; to better understand the infection processes and associated risk factors.
In this study, we focused specifically on bacterial colonization of potato tubers, an ecologically important infection model. Dickeya species share many common virulence strategies, including the production of plant cell wall degrading enzymes such as pectate lyases (Reverchon et al., 2016). However, virulence traits can vary even among closely related strains. For example, Ge et al. (2021b,c) reported the D. dianthicola strains isolated in the United States comprise multiple genetic groups, with differing type IV and type VI secretion system genes. These differences, determined by pan-genomic analysis, suggest driving forces for population level changes in outbreaks which can be explored by molecular characterization (Ge et al., 2021c). By constructing barcoded transposon insertion libraries in D. dadantii and D. dianthicola, and testing these mutant populations in parallel conditions, we were able to directly compare gene contributions to fitness among these related strains. The scalability of RB-TnSeq, paired with ortholog prediction or gene co-fitness measurements, has proven to be a useful method to compare ortholog presence and function (Price et al., 2018). By measuring genome-wide gene contributions to growth, we identified a comprehensive list of genes in three Dickeya strains that contribute to fitness in three in vitro conditions and tubers. This approach could be applied to any (genetically tractable) soft rot causing strains, particularly building upon pan-genomic analysis to identify key strains of interest.
In the strains tested here, many core metabolic processes were highly important in both minimal medium and tuber samples, such as biosynthesis of many amino acids. Notably, potato tubers contain higher arginine concentrations than other essential amino acids (Bártová et al., 2015), correlating with the dispensability of the arginine biosynthetic genes (argCEFGH) in tubers (Figure 4). Interestingly, though an Erwinia amylovora argD mutant is auxotrophic and non-pathogenic in apple (Ramos et al., 2014), argD is present in two apparently redundant copies in Dda3937 (DDA3937_RS19450 and DDA3937_RS03635), and mutants in these genes had no phenotype in the conditions tested. Previous studies have shown the important of chemotaxis and motility for early stage virulence (Jahn et al., 2008), but these traits were dispensable for growth in tubers with the inoculation and sampling methods used here. We used potato dextrose broth as an in vitro condition to mimic the nutritional composition of potato tubers more closely. This medium was slightly acidic, reflecting conditions encountered in the tuber or apoplast (Grignon and Sentenac, 1991; Kiszonas and Bamberg, 2010). Of the PDB-specific fitness factors, the low affinity potassium transporter Kup was likely required because of its role in ion transport particularly at low pH (Trchounian and Kobayashi, 1999).
Bacterial growth on potato tubers requires plant-specific virulence traits. Interestingly, the gene fitness profiles were indistinguishable among the potato cultivars tested. It is possible that tubers of alternative cultivars might sufficiently differ in their chemical composition to alter the metabolic genes needed for colonization. It would be useful to see if these results are consistent across additional plant tissues, such as the stem. Our data generally support in planta findings from other groups, from the importance of core metabolic capabilities during tuber colonization to the production of the pectin degradation protein KduD (Condemine and Robert-Baudouy, 1991; Royet et al., 2019; Czajkowski et al., 2020). Transposon mutagenesis of P. carotovorum followed by screening for altered soft rot symptoms in Chinese cabbage identified homologous genes involved in nutrient utilization, production of plant cell wall degrading enzymes, motility, biofilm formation, and toxin susceptibility (Lee et al., 2013). In chicory, Dda3937 required core metabolic and nucleic acid biosynthetic genes, flagellar motility, and iron uptake genes for full virulence (Royet et al., 2019). As in Royet et al. (2019) study, type III secretion system genes were dispensable for virulence when inoculated at high concentrations here. Type III effectors may promote initial multiplication within the host, but their role in Dickeya necrotrophic growth is secondary to the production of plant cell wall degrading enzymes (Yang et al., 2010). Though pectate lyases are collectively important for virulence (Condemine and Robert-Baudouy, 1991; Nachin and Barras, 2000), their redundancy makes mutagenesis-based study difficult (Schoedel and Collmer, 1986; Lojkowska et al., 1995). In all three Dickeya strains tested here, each of six pectate lyase genes were individually dispensable, supporting functional redundancy and/or complementation in trans (Supplementary Figure 6).
While D. dadantii and D. dianthicola can cause soft rot on potato tubers, variation in some key genes under these conditions suggests species- and strain-level differences in virulence strategies and stress responses. General strategies for environmental growth and host colonization are consistent, such as general metabolic capabilities and stress tolerance. However, gene fitness data suggest variation in gene regulation, such as the helix-turn-helix and other putative DNA-binding proteins. Large positive fitness values in Dda3937 flagellar mutants in vitro suggest that in liquid minimal medium flagella are costly. Since the same phenotype is not observed for DdiaME23 or Ddia6719, this might imply flagella are controlled differently in liquid media in these species. Further characterization of the regulation of these traits is needed.
The scalability of RB-TnSeq, paired with ortholog identification, has proven to be a useful method to directly compare gene fitness between related strains. Dickeya species generally have common virulence strategies, primarily the production of plant cell wall degrading enzymes such as pectate lyases (Reverchon et al., 2016). However, genomic and transcriptomic variation at the strain and species level highlights distinctive virulence traits (Raoul des Essarts et al., 2019). This supports the intriguing possibility that while enzymatic virulence traits are shared across pathogens, there exists strain-specific virulence regulation. This idea has been proposed, but not directly tested, in D. solani based on predicted binding sites for transcriptional regulators (Golanowska et al., 2018). In the case of our study, while Dda3937 is pathogenic on potato, it was originally isolated from Saintpaulia ionantha (Lemattre and Narcy, 1972), suggesting potato infection is simply opportunistic. Ddia6719 was originally isolated from New Guinea impatiens (Impatiens hawkeri) (Liu et al., 2020a), but observed symptoms in tubers were similar to those caused by DdiaME23.
This study focused on isolated strain growth, to generate a comprehensive dataset of likely essential genes and soft rot virulence traits in D. dadantii and D. dianthicola. Testing other Dickeya species, and closely related pathogens such as Pectobacterium spp., will more broadly expand our understanding of soft rot pathogens. With interest in using biocontrol strains or applying antimicrobial small molecules directly for control of soft rot (Czajkowski et al., 2011), it would be useful to screen pathogenic strains for potential resistance mechanisms. Similarly, in the field, soft rot symptoms can be the result of complex community interactions, with Dickeya and Pectobacterium co-infections frequently observed (Ge et al., 2021a). This presence of additional community members might change the composition of genes required for full competitive fitness. Understanding bacterial virulence strategies will aid in breeding efforts, as well as identify potential bacterial traits that could enable overcoming host tolerance or exacerbating disease at all stages of production. Similarly, the ability to rapidly predict and identify specific virulence traits in novel isolates will be key to addressing emerging outbreak pathogens.
All raw Illumina reads used for mapping and fitness assays have been deposited in the Sequence Read Archive under BioProject accession number PRJNA692477. Individual sample accession numbers are listed in Supplementary Table 2. Annotated scripts used for computational analysis are available at github.com/tylerhelmann/dickeya-barseq-2021. Experimental fitness values are publicly available at fit.genomics.lbl.gov.
TH, MF, and PS contributed to conception and designed of the study. TH performed the experiments and wrote the first draft of the manuscript. TH and PS performed data analysis. MF and PS supervised the project. All authors contributed to data interpretation, contributed to manuscript revision, read, and approved the submitted version.
This project was funded by the USDA-ARS project no. 8062-2100-042.
The authors declare that the research was conducted in the absence of any commercial or financial relationships that could be construed as a potential conflict of interest.
All claims expressed in this article are solely those of the authors and do not necessarily represent those of their affiliated organizations, or those of the publisher, the editors and the reviewers. Any product that may be evaluated in this article, or claim that may be made by its manufacturer, is not guaranteed or endorsed by the publisher.
We would like to thank Mary Ann Karp for technical assistance; Walter De Jong and the Cornell Potato Breeding Program for providing potato tubers; Adam Deutschbauer for providing the donor E. coli library APA752 containing the barcoded mariner vector; and Morgan Price for assisting with the FEBA pipeline and data upload to the Fitness Browser. Sequencing was performed by the Biotechnology Resource Center (BRC) Genomics Facility at the Cornell Institute of Biotechnology. Mention of trade names or commercial products in this publication is solely for the purpose of providing specific information and does not imply recommendation or endorsement by the United States Department of Agriculture. USDA is an equal opportunity provider and employer.
The Supplementary Material for this article can be found online at: https://www.frontiersin.org/articles/10.3389/fmicb.2022.778927/full#supplementary-material
Adeolu, M., Alnajar, S., Naushad, S., and Gupta, R. S. (2016). Genome-based phylogeny and taxonomy of the ‘Enterobacteriales’: proposal for Enterobacterales ord. nov. divided into the families Enterobacteriaceae, Erwiniaceae fam. nov., Pectobacteriaceae fam. nov. Int. J. Syst. Evol. Microbiol. 66, 5575–5599. doi: 10.1099/ijsem.0.001485
Adriaenssens, E. M., van Vaerenbergh, J., Vandenheuvel, D., Dunon, V., Ceyssens, P. J., de Proft, M., et al. (2012). T4-related bacteriophage LIMEstone isolates for the control of soft rot on potato caused by “Dickeya solani.”. PLoS One 7:e33227. doi: 10.1371/journal.pone.0033227
Bártová, V., Bárta, J., Brabcová, A., Zdráhal, Z., and Horáčková, V. (2015). Amino acid composition and nutritional value of four cultivated South American potato species. J. Food Compos. Anal. 40, 78–85. doi: 10.1016/j.jfca.2014.12.006
Bertani, G. (1951). Studies on lysogenesis. I. The mode of phage liberation by lysogenic Escherichia coli. J. Bacteriol. 62, 293–300. doi: 10.1128/JB.62.3.293-300.1951
Chen, I. M. A., Chu, K., Palaniappan, K., Pillay, M., Ratner, A., Huang, J., et al. (2019). IMG/M v.5.0: an integrated data management and comparative analysis system for microbial genomes and microbiomes. Nucleic Acids Res. 47, D666–D677. doi: 10.1093/nar/gky901
Chung, Y. S., Goeser, N. J., Cai, X., and Jansky, S. (2013). The effect of long term storage on bacterial soft rot resistance in potato. Am. J. Potato Res. 90, 351–356. doi: 10.1007/s12230-013-9311-6
Cole, B. J., Feltcher, M. E., Waters, R. J., Wetmore, K. M., Mucyn, T. S., Ryan, E. M., et al. (2017). Genome-wide identification of bacterial plant colonization genes. PLoS Biol. 15:e2002860. doi: 10.1371/journal.pbio.2002860
Condemine, G., and Robert-Baudouy, J. (1991). Analysis of an Erwinia chrysanthemi gene cluster involved in pectin degradation. Mol. Microbiol. 5, 2191–2202. doi: 10.1111/j.1365-2958.1991.tb02149.x
Czajkowski, R., Fikowicz-Krosko, J., Maciag, T., Rabalski, L., Czaplewska, P., Jafra, S., et al. (2020). Genome-wide identification of Dickeya solani transcriptional units up-regulated in response to plant tissues from a crop-host Solanum tuberosum and a weed-host Solanum dulcamara. Front. Plant Sci. 11:580330. doi: 10.3389/fpls.2020.580330
Czajkowski, R., Pérombelon, M. C. M., Van Veen, J. A., and Van der Wolf, J. M. (2011). Control of blackleg and tuber soft rot of potato caused by Pectobacterium and Dickeya species: a review. Plant Pathol. 60, 999–1013. doi: 10.1111/j.1365-3059.2011.02470.x
Czajkowski, R., Smolarska, A., and Ozymko, Z. (2017). The viability of lytic bacteriophage ΦD5 in potato-associated environments and its effect on Dickeya solani in potato (Solanum tuberosum L.) plants. PLoS One 12:e183200. doi: 10.1371/journal.pone.0183200
Ge, T., Jiang, H., Tan, E. H., Johnson, S. B., Larkin, R., Charkowski, A. O., et al. (2021c). Pangenomic analysis of Dickeya dianthicola strains related to the outbreak of blackleg and soft rot of potato in USA. Plant Dis. [Online ahead of print] DIS03210587RE. doi: 10.1094/PDIS-03-21-0587-RE
Ge, T., Jiang, H., Johnson, S. B., Larkin, R. P., Charkowski, A. O., Secor, G., et al. (2021b). Genotyping Dickeya dianthicola causing potato blackleg and soft rot outbreak associated with inoculum geography in the United States. Plant Dis. 105, 1976–1983. doi: 10.1094/PDIS-10-20-2138-RE
Ge, T., Ekbataniamiri, F., Johnson, S. B., Larkin, R. P., and Hao, J. (2021a). Interaction between Dickeya dianthicola and Pectobacterium parmentieri in potato infection under field conditions. Microorganisms 9, 1–10. doi: 10.3390/microorganisms9020316
Georgoulis, S., Shalvarjian, K. E., Helmann, T. C., Hamilton, C. D., Carlson, H. K., Deutschbauer, A. M., et al. (2021). Genome-wide identification of tomato xylem sap fitness factors for three plant-pathogenic Ralstonia species. mSystems 6, e1229–e1221. doi: 10.1101/2020.08.31.276741
Golanowska, M., Potrykus, M., Motyka-Pomagruk, A., Kabza, M., Bacci, G., Galardini, M., et al. (2018). Comparison of highly and weakly virulent Dickeya solani strains, with a view on the pangenome and panregulon of this species. Front. Microbiol. 9:1940. doi: 10.3389/fmicb.2018.01940
Grignon, C., and Sentenac, H. (1991). pH and ionic conditions in the apoplast. Annu. Rev. Plant Physiol. Plant Mol. Biol. 42, 103–128. doi: 10.1146/annurev.pp.42.060191.000535
Helmann, T. C., Deutschbauer, A. M., and Lindow, S. E. (2019). Genome-wide identification of Pseudomonas syringae genes required for fitness during colonization of the leaf surface and apoplast. Proc. Natl. Acad. Sci. U.S.A. 116, 18900–18910. doi: 10.1073/pnas.1908858116
Jahn, C. E., Willis, D. K., and Charkowski, A. O. (2008). The flagellar sigma factor FliA is required for Dickeya dadantii virulence. Mol. Plant-Microbe Interact. 21, 1431–1442. doi: 10.1094/MPMI-21-11-1431
Jiang, X., Zghidi-Abouzid, O., Oger-Desfeux, C., Hommais, F., Greliche, N., Muskhelishvili, G., et al. (2016). Global transcriptional response of Dickeya dadantii to environmental stimuli relevant to the plant infection. Environ. Microbiol. 18, 3651–3672. doi: 10.1111/1462-2920.13267
Kiszonas, A. M., and Bamberg, J. B. (2010). Survey of tuber pH variation in potato (Solanum) species. Am. J. Potato Res. 87, 167–176. doi: 10.1007/s12230-009-9120-0
Lee, D. H., Lim, J. A., Lee, J., Roh, E., Jung, K., Choi, M., et al. (2013). Characterization of genes required for the pathogenicity of Pectobacterium carotovorum subsp. carotovorum Pcc21 in Chinese cabbage. Microbiology (United Kingdom) 159, 1487–1496. doi: 10.1099/mic.0.067280-0
Lemattre, M., and Narcy, J. P. (1972). Une affection bacterienne nouvelle du Saintpaulia due a Erwinia chrysanthemi. C. R. Acad. Sci 58, 227–231.
Liu, H., and Deutschbauer, A. M. (2018). Rapidly moving new bacteria to model-organism status. Curr. Opin. Biotechnol. 51, 116–122. doi: 10.1016/j.copbio.2017.12.006
Liu, H., Shiver, A. L., Price, M. N., Carlson, H. K., Trotter, V. V., Chen, Y., et al. (2021). Functional genetics of human gut commensal Bacteroides thetaiotaomicron reveals metabolic requirements for growth across environments. Cell Rep. 34:108789. doi: 10.1016/j.celrep.2021.108789
Liu, Y., Helmann, T., Stodghill, P., and Filiatrault, M. (2020a). Complete genome sequence resource for the necrotrophic plant-pathogenic bacterium Dickeya dianthicola 67-19 isolated from New Guinea Impatiens. Plant Dis. 105, 1174–1176. doi: 10.1094/PDIS-09-20-1968-A
Liu, Y., Vasiu, S., Daughtrey, M. L., and Filiatrault, M. (2020b). First Report of Dickeya dianthicola causing blackleg on New Guinea Impatiens (Impatiens hawkeri) in New York State, USA. Plant Dis. [Online ahead of print] doi: 10.1094/pdis-09-20-2020-pdn
Lojkowska, E., Masclaux, C., Boccara, M., Robert-Baudouy, J., and Hugouvieux-Cotte-Pattat, N. (1995). Characterization of the pelL gene encoding a novel pectate lyase of Erwinia chrysanthemi 3937. Mol. Microbiol. 16, 1183–1195.
Lyon, G. D. (1989). The biochemical basis of resistance of potatoes to soft rot Erwinia spp.—a review. Plant Pathol. 38, 313–339. doi: 10.1111/j.1365-3059.1989.tb02152.x
M9 Minimal Medium (Standard) (2010). M9 minimal medium (standard). Cold Spring Harb. Protoc. 2010:db.rec12295. doi: 10.1101/pdb.rec12295
Ma, X., Perna, N. T., Glasner, J. D., Hao, J., Johnson, S., Nasaruddin, A. S., et al. (2019). Complete genome sequence of Dickeya dianthicola ME23, a pathogen causing blackleg and soft rot diseases of potato. Microbiol. Resour. Announc. 8, 14–15. doi: 10.1128/mra.01526-18
Melnyk, R. A., Hossain, S. S., and Haney, C. H. (2019). Convergent gain and loss of genomic islands drives lifestyle changes in plant-associated Pseudomonas. ISME J. 13, 1575–1588. doi: 10.1101/345488
Motyka, A., Zoledowska, S., Sledz, W., and Lojkowska, E. (2017). Molecular methods as tools to control plant diseases caused by Dickeya and Pectobacterium spp: a minireview. N. Biotechnol. 39, 181–189. doi: 10.1016/j.nbt.2017.08.010
Motyka-Pomagruk, A., Zoledowska, S., Misztak, A. E., Sledz, W., Mengoni, A., and Lojkowska, E. (2020). Comparative genomics and pangenome-oriented studies reveal high homogeneity of the agronomically relevant enterobacterial plant pathogen Dickeya solani. BMC Genomics 21:449. doi: 10.1186/s12864-020-06863-w
Nachin, L., and Barras, F. (2000). External pH: an environmental signal that helps to rationalize pel gene duplication in Erwinia chrysanthemi. Mol. Plant-Microbe Interact. 13, 882–886. doi: 10.1094/MPMI.2000.13.8.882
Parkinson, N., DeVos, P., Pirhonen, M., and Elphinstone, J. (2014). Dickeya aquatica sp. nov., isolated from waterways. Int. J. Syst. Evol. Microbiol. 64, 2264–2266. doi: 10.1099/ijs.0.058693-0
Price, M. N., Wetmore, K. M., Waters, R. J., Callaghan, M., Ray, J., Liu, H., et al. (2018). Mutant phenotypes for thousands of bacterial genes of unknown function. Nature 557, 503–509. doi: 10.1038/s41586-018-0124-0
R Core Team (2017). R: A Language and Environment for Statistical Computing. Vienna: R Foundation for Statistical Computing.
Ramos, L. S., Lehman, B. L., Peter, K. A., and McNellis, T. W. (2014). Mutation of the Erwinia amylovora argD gene causes arginine auxotrophy, nonpathogenicity in apples, and reduced virulence in pears. Appl. Environ. Microbiol. 80, 6739–6749. doi: 10.1128/AEM.02404-14
Raoul des Essarts, Y., Pédron, J., Blin, P., Van Dijk, E., Faure, D., and Van Gijsegem, F. (2019). Common and distinctive adaptive traits expressed in Dickeya dianthicola and Dickeya solani pathogens when exploiting potato plant host. Environ. Microbiol. 21, 1004–1018. doi: 10.1111/1462-2920.14519
Reverchon, S., Muskhelisvili, G., and Nasser, W. (2016). Virulence program of a bacterial plant pathogen: the Dickeya model. Progr. Mol. Biol. Transl. Sci. 142, 51–92. doi: 10.1016/bs.pmbts.2016.05.005
Royet, K., Parisot, N., Rodrigue, A., Gueguen, E., and Condemine, G. (2019). Identification by Tn-seq of Dickeya dadantii genes required for survival in chicory plants. Mol. Plant Pathol. 20, 287–306. doi: 10.1111/mpp.12754
Rubin, B. E., Diamond, S., Cress, B. F., Crits-Christoph, A., Lou, Y. C., Borges, A. L., et al. (2021). Species- and site-specific genome editing in complex bacterial communities. Nat. Microbiol. 7, 34–47. doi: 10.1038/s41564-021-01014-7
Samson, R., Legendre, J. B., Christen, R., Fischer-Le Saux, M., Achouak, W., and Gardan, L. (2005). Transfer of Pectobacterium chrysanthemi (Burkholder et al. 1953) Brenner et al. 1973 and Brenneria paradisiaca to the genus Dickeya gen. nov. as Dickeya chrysanthemi comb. nov. and Dickeya paradisiaca comb. nov. Int. J. Syst. Evol. Microbiol. 55, 1415–1427. doi: 10.1099/ijs.0.02791-0
Schoedel, C., and Collmer, A. (1986). Evidence of homology between the pectate lyase-encoding pelB and pelC genes in Erwinia chrysanthemi. J. Bacteriol. 167, 117–123. doi: 10.1128/jb.167.1.117-123.1986
Tian, Y., Zhao, Y., Yuan, X., Yi, J., Fan, J., Xu, Z., et al. (2016). Dickeya fangzhongdai sp. nov., a plant-pathogenic bacterium isolated from pear trees (Pyrus pyrifolia). Int. J. Syst. Evol. Microbiol. 66, 2831–2835. doi: 10.1099/ijsem.0.001060
Toth, I. K., van der Wolf, J. M., Saddler, G., Lojkowska, E., Hélias, V., Pirhonen, M., et al. (2011). Dickeya species: an emerging problem for potato production in Europe. Plant Pathol. 60, 385–399. doi: 10.1111/j.1365-3059.2011.02427.x
Trchounian, A., and Kobayashi, H. (1999). Kup is the major K+ uptake system in Escherichia coli upon hyper-osmotic stress at a low pH. FEBS Lett. 447, 144–148. doi: 10.1016/S0014-5793(99)00288-4
van der Wolf, J. M., Acuña, I., De Boer, S. H., Brurberg, M. B., Cahill, G., Charkowski, A. O., et al. (2021). “Diseases caused by Pectobacterium and Dickeya species around the world,” in Plant Diseases Caused by Dickeya and Pectobacterium Species, eds F. Van Gijsegem, J. M. van der Wolf, and I. K. Toth (Cham: Springer International Publishing), 215–261.
Van Der Wolf, J. M., Nijhuis, E. H., Kowalewska, M. J., Saddler, G. S., Parkinson, N., Elphinstone, J. G., et al. (2014). Dickeya solani sp. nov., a pectinolytic plant-pathogenic bacterium isolated from potato (Solanum tuberosum). Int. J. Syst. Evol. Microbiol. 64, 768–774. doi: 10.1099/ijs.0.052944-0
Van Gijsegem, F., Toth, I. K., and van der Wolf, J. M. (2021). “Outlook-Challenges and perspectives for management of diseases caused by Pectobacterium and Dickeya species,” in Plant Diseases Caused by Dickeya and Pectobacterium Species, eds F. Van Gijsegem, J. M. van der Wolf, and I. K. Toth (Cham: Springer International Publishing), 283–289.
van Opijnen, T., Bodi, K. L., and Camilli, A. (2009). Tn-seq: high-throughput parallel sequencing for fitness and genetic interaction studies in microorganisms. Nat. Methods 6, 767–772. doi: 10.1038/nmeth.1377
Wetmore, K. M., Price, M. N., Waters, R. J., Lamson, J. S., He, J., Hoover, C. A., et al. (2015). Rapid quantification of mutant fitness in diverse bacteria by sequencing randomly bar-coded transposons. MBio 6, 1–15. doi: 10.1128/mBio.00306-15
Yang, S., Peng, Q., Zhang, Q., Zou, L., Li, Y., Robert, C., et al. (2010). Genome-wide identification of HrpL-regulated genes in the necrotrophic phytopathogen Dickeya dadantii 3937. PLoS One 5:e13472. doi: 10.1371/journal.pone.0013472
Keywords: potato, soft rot, RB-TnSeq, TnSeq, Dickeya dadantii, Dickeya dianthicola
Citation: Helmann TC, Filiatrault MJ and Stodghill PV (2022) Genome-Wide Identification of Genes Important for Growth of Dickeya dadantii and Dickeya dianthicola in Potato (Solanum tuberosum) Tubers. Front. Microbiol. 13:778927. doi: 10.3389/fmicb.2022.778927
Received: 17 September 2021; Accepted: 10 January 2022;
Published: 25 January 2022.
Edited by:
Jeffrey Jones, University of Florida, United StatesReviewed by:
Robert Czajkowski, University of Gdańsk, PolandCopyright © 2022 Helmann, Filiatrault and Stodghill. This is an open-access article distributed under the terms of the Creative Commons Attribution License (CC BY). The use, distribution or reproduction in other forums is permitted, provided the original author(s) and the copyright owner(s) are credited and that the original publication in this journal is cited, in accordance with accepted academic practice. No use, distribution or reproduction is permitted which does not comply with these terms.
*Correspondence: Paul V. Stodghill, cGF1bC5zdG9kZ2hpbGxAdXNkYS5nb3Y=
†ORCID: Tyler C. Helmann, orcid.org/0000-0002-8431-6461; Melanie J. Filiatrault, orcid.org/0000-0001-7704-9097; Paul V. Stodghill, orcid.org/0000-0003-3875-8450
Disclaimer: All claims expressed in this article are solely those of the authors and do not necessarily represent those of their affiliated organizations, or those of the publisher, the editors and the reviewers. Any product that may be evaluated in this article or claim that may be made by its manufacturer is not guaranteed or endorsed by the publisher.
Research integrity at Frontiers
Learn more about the work of our research integrity team to safeguard the quality of each article we publish.