- 1Medical Science Research Institute, School of Medicine, Sungkyunkwan University (SKKU), Suwon, South Korea
- 2Department of Environmental Health Sciences, Graduate School of Public Health, Seoul National University, Seoul, South Korea
- 3Center for Human and Environmental Microbiome, Seoul National University, Seoul, South Korea
- 4Department of Microbiology and Immunology, Rega Institute for Medical Research, Leuven, Belgium
- 5VIB-KU Leuven Center for Microbiology, Leuven, Belgium
- 6Bio-MAX/N-Bio, Seoul National University, Seoul, South Korea
- 7KobioLabs, Seoul, South Korea
- 8Institute of Health and Environment, Seoul National University, Seoul, South Korea
Background and Objective: Cluster-based analysis, or community typing, has been attempted as a method for studying the human microbiome in various body niches with the aim of reducing variations in the bacterial composition and linking the defined communities to host health and disease. In this study, we have presented the bacterial subcommunities in the healthy and the diseased population cohorts and have assessed whether these subcommunities can distinguish different host health conditions.
Methods: We performed community typing analysis on the sputum microbiome dataset obtained from a healthy Korean twin-family cohort (n = 202) and an external chronic obstructive pulmonary disease (COPD) cohort (n = 324) and implemented a networks analysis to investigate the associations of bacterial metacommunities with host health parameters and microbial interactions in disease.
Results: The analysis of the sputum microbiome of a healthy Korean cohort revealed high levels of interindividual variation, which was driven by two dominant bacteria: Neisseria and Prevotella. Community typing of the cohort samples identified three metacommunities, namely, Neisseria 1 (N1), Neisseria 2 (N2), and Prevotella (P), each of which showed different functional potential and links to host traits (e.g., triglyceride levels, waist circumference, and levels of high-sensitivity C-reactive protein). In particular, the Prevotella-dominant metacommunity showed a low-community diversity, which implies an adverse health association. Network analysis of the healthy twin cohort illustrated co-occurrence of Prevotella with pathogenic anaerobic bacteria; this bacterial cluster was negatively associated with high-density lipoproteins but positively correlated with waist circumference, blood pressure, and pack-years. Community typing of the external COPD cohort identified three sub-metacommunities: one exclusively comprising healthy subjects (HSs) and the other two (CS1 and CS2) comprising patients. The two COPD metacommunities, CS1 and CS2, showed different abundances of specific pathogens, such as Serratia and Moraxella, as well as differing functional potential and community diversity. Network analysis of the COPD cohort showed enhanced bacterial coexclusions in the CS metacommunities when compared with HS metacommunity.
Conclusion: Overall, our findings point to a potential association between pulmonary Prevotella and host health and disease, making it possible to implement community typing for the diagnosis of heterogenic respiratory disease.
Introduction
Advances in high-throughput sequencing in the field of human microbiome research have expanded our knowledge of host–microbe interactions and their effects on human health and disease. The respiratory tract, which has one of the largest surface areas in the human body (70 m2) (Huffnagle et al., 2017), provides a distinct ecological habitat for microbes. In particular, the upper respiratory tract (nasal and oral passages), which connects the external environment to the deeper lower airways, undergoes constant interaction with inhaled microbes and resident bacteria. Furthermore, because sputum samples can be collected in a non-invasive way, researchers have shown interest in investigating the microbiome of the upper respiratory tract to assess its value as a biomarker for lung cancer, tuberculosis, asthma, cystic fibrosis, and chronic obstructive pulmonary disease (COPD) (Krishna et al., 2016; Cameron et al., 2017; Leitao Filho et al., 2019; Pang et al., 2019; Quinn et al., 2019). Of the pulmonary diseases, COPD has had the fourth highest global mortality rate since 2015 (Sidhaye et al., 2018). The unique characteristics of this disease stem from its clinical heterogeneity with respect to symptoms, progression, and survival; indeed, there are up to seven different phenotypes of the disease (Han et al., 2010; Mirza and Benzo, 2017). Because of this complexity, medical treatment is still at an early stage, making prevention or rapid diagnosis prior to disease exacerbation the best treatment strategy.
The human microbiome harbors high levels of interindividual variation, which complicates linkage with host features. To separate disease-relevant/-specific signals linked to the microbiome from the background noise caused by interindividual variations, attempts have been made to stratify host populations in the gut, vagina, lung, and saliva microbiomes according to community composition (Arumugam et al., 2011; Ravel et al., 2011; Segal et al., 2013; Xun et al., 2018). In particular, a unique microbial cluster in the gut has biological linkages with intestinal activity, host health, and disease (Vandeputte et al., 2017; Valles-Colomer et al., 2019; Vieira-Silva et al., 2019). Therefore, to find out whether the highly variable sputum microbiota can be grouped and connected to host health status, we first implemented community typing of 202 sputum microbiome sequencing data from the Healthy Twin Study (Sung et al., 2006) and identified three distinct metacommunities. Interestingly, a Prevotella-dominant metacommunity with the lowest community diversity showed potential associations with smoking, hsCRP, triglyceride (TG) levels, and waist circumference. Next, we hypothesized that patients with COPD would harbor multiple metacommunities, in line with the heterogenous disease traits of COPD. The same community typing approach applied to an independent COPD cohort identified two sub-metacommunities showing distinct functional differences. Taken together, this study provides evidence of a potential link between Prevotella and host (pulmonary) health and raise the possibility that community typing can help in distinguishing between heterogeneous host respiratory health disorders, making it useful for prognostic or diagnostic purposes.
Materials and Methods
Study Cohorts
Sputum sequencing data generated from the Healthy Twin Study in Korea were used for this study (European Nucleotide Archive; ERP010734) (Sung et al., 2006; Lim et al., 2016). Subjects were excluded from this study if they had received antibiotic treatment or cold medication within the past 3 months. Also, subjects with symptoms of airway diseases such as asthma or COPD were excluded. The sequencing data were further filtered to ensure that only subjects aged 30–60 years old were included. A gender ratio was balanced using the optmatch R package (Hansen and Klopfer, 2006). Consequently, sequencing data from 202 participants (including 53 monozygotic and 12 dizygotic twin pairs and their family members) and their associated metadata were analyzed. Additional sputum sequencing data from an external COPD cohort (n = 324) (Haldar et al., 2020) were obtained from the Sequence Read Archive [under accession numbers PRJNA491861 (healthy samples, n = 121) and SRP102480 (COPD samples, n = 203)].
16S rRNA Sequence Data Analysis
Raw sequence data were processed further using QIIME (version 1.8.0) (Kuczynski et al., 2011). Operational taxonomic unit (OTU) picking was performed by clustering sequences at 97% similarity. Taxonomic classification of each OTU was performed using the Ribosomal Database Project classifier (Wang et al., 2007) and the Greengenes database (version 13_5) (DeSantis et al., 2006). An OTU count table was then agglomerated at the genus level before downstream analysis. Sequence files from the COPD cohort were analyzed following DADA2 pipelines (1.12.1) using default settings (Callahan et al., 2016). The trim parameters for the healthy samples were as follows: sequence truncate 200 bp for both the forward and reverse sequence reads; trim left 10. Trim parameters for patients with COPD were as follows: sequence truncate 150 bp for forward and 130 bp for reverse sequence reads; trim left 10.
Functional Potential of the Sputum Microbiota
The functional potential of the sputum microbiota was predicted using Piphillin based on the 16s rRNA sequences (Iwai et al., 2016). Piphillin infers metagenomic functions using OTU counts and their representative sequences; it then matches the sequences to the nearest neighbor in the genome database. Prior to analysis, OTUs observed in less than 50% of samples were excluded. A 95% identity cut-off was used to determine the similarity between 16s rRNAs and the reference genome database. Amplicon sequence variant sequences obtained for the COPD cohort were implemented at a 97% identity cutoff. For functional analysis, the Kyoto Encyclopedia of Genes and Genomes (KEGG) database was used at the pathway level.
Identification of Bacterial Clusters
The weighted correlation network analysis (WGCNA) was performed to determine bacterial interactions with the host (Langfelder and Horvath, 2008). This software implements a signed weighted co-occurrence network in which the negatively connected nodes are considered unconnected. Genera observed in < 50% of samples were excluded to reduce false positives due to low abundance taxa; data were then subjected to Hellinger transformation and were log-scaled. Soft-thresholding power (β = 3), which satisfies the scale-free topology of the network, was determined using the pickSoftThreshold function. A signed adjacency matrix was then converted to a Topological Overlap Matrix and used to define bacterial clusters based on hierarchical clustering (average linkage). Association of the bacterial clusters with clinical variables was determined by correlating the eigenvector of each cluster with host variables.
Network Analysis to Determine Microbe–Microbe Interactions
To investigate microbe–microbe interactions, network analysis was performed using SparCC, with 500 bootstraps used to estimate p values (Friedman and Alm, 2012). A Q-value < 0.002 was considered significant. Taxa comprising < 20% of the population were excluded. Final networks were plotted using Cytoscape (version 3.7.2; perfused force directed layout) (Shannon et al., 2003).
Statistical Analysis
After randomly rarefied to 9,000 reads, microbial diversity was determined using the phyloseq and vegan R packages (Dixon, 2003; McMurdie and Holmes, 2013). The COPD cohort samples were rarefied to 10,000 reads. Community variation was determined by nonmetric non-metric multidimensional scaling and principal coordinates analysis using the metaMDS and envfit functions in the vegan package. Identification of metacommunities was performed by fitting the models of Dirichlet multinomial mixtures to the community structure (Holmes et al., 2012). The number of metacommunities was determined as the number that provides the minimum Laplace approximation to the model evidence. Associations between metacommunities and clinical variables were analyzed using the Wilcoxon rank sum test, the Kruskal–Wallis test followed by the Dunn’s post hoc test (for more than the two groups), or the chi-square test with post hoc tests. A pairwise Adonis test was performed using the pairwiseAdonis package (Martinez Arbizu, 2020). When testing more than two parameters, all the statistical tests were followed by multiple testing correction using the Benjamini–Hochberg method (denoted as a q value). All the analyses were conducted using R (version 3.4.1).
Results
Metacommunity of the Sputum Microbiota in a Healthy Korean Twin-Family Cohort
Despite the high degree of interindividual variation, we found distinct patterns of dominant genera in the sputum microbiota of the healthy Korean twin-family cohort (n = 202; Figure 1A). To analyze whether these patterns allow stratification of the cohort, we clustered the airway microbiota by fitting the taxonomic abundances to a probabilistic model fitting a Dirichlet multinomial mixture, which gives the optimal number of clusters by modeling sparse microbiome data (Supplementary Figure 1A). This process identified three metacommunities, in which the dominant bacteria were Prevotella and Neisseria (Supplementary Figures 1B, C). Because these two genera were the major driving taxa for two of the three clusters (the other cluster was driven by multiple taxa) on an NMDA plot, we defined clusters according to predominance; however, we subclassified the Neisseria-dominant clusters into N1 and N2 based on Neisseria abundance (Figure 1B). Microbial composition at the phylum level revealed more abundant Proteobacteria in the N1 and N2 metacommunities than in the Prevotella-dominant metacommunity, while Bacteroidetes and Firmicutes showed the opposite pattern (Supplementary Figure 1D). Community diversity analysis showed that the Prevotella (P) metacommunity had significantly lower richness and evenness than the other subtypes (the Kruskal–Wallis test, q < 0.1; Figure 1C). Because the metacommunities in the sputum microbiota showed compositional differences, we further analyzed them to evaluate whether they also showed functional differences. Functional potential was inferred based on 16S rRNA sequence data, which showed distinct functional clustering of the P metacommunity (Figure 1D and Supplementary Table 1). More than 35% of the community variation could be explained by the P metacommunity associating with the other N subtypes. However, the N metacommunities together explained only 4% of community variation, suggesting homogeneity Between N metacommunities with regard to their functions.
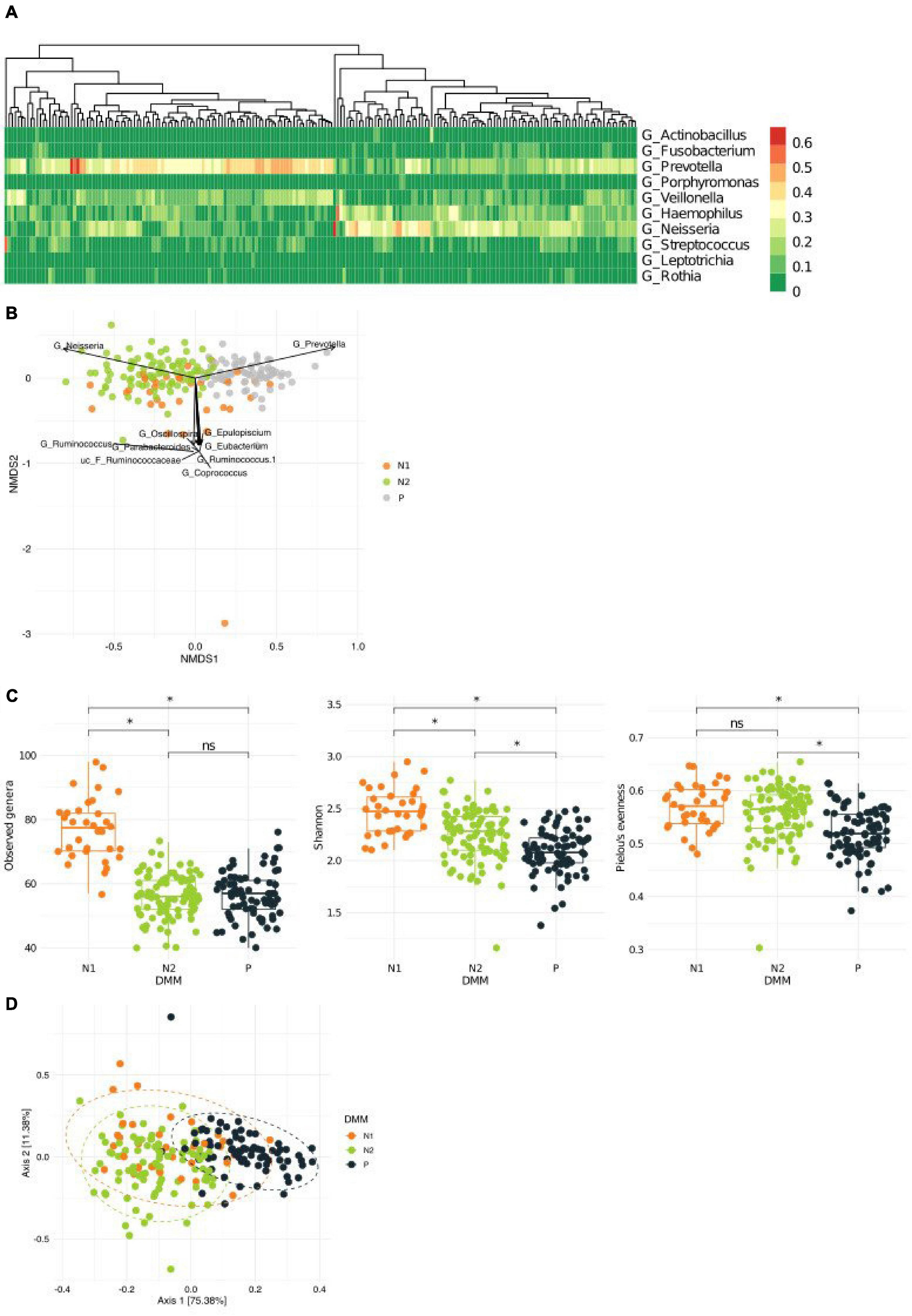
Figure 1. Variations in the microbiota in sputum samples collected from a healthy Korean cohort. (A) Heatmap showing the top 10 most abundant bacteria in the sputum microbiota. (B) A non-metric multidimensional scaling (NMDS) plot in which metacommunities are assigned a color. Arrows indicate the top 10 bacteria contributing to community variation. (C) Metacommunity diversity at the genus level. (D) Different inferred functional characteristics of the sputum microbiome among metacommunities. A principal coordinates analysis (PCoA) plot showing the distribution of functional features of metacommunity. *q < 0.001.
Link Between Metacommunities, Host Health, and Smoking Status
To better understand the link between metacommunities and host health status, we analyzed the association between metabolic parameters and the metacommunities (Table 1). We found that TG levels and waist circumference were significantly different among individuals with different metacommunities: the P metacommunity was associated with significantly greater TG levels and waist circumference than the N2 community (the Kruskal–Wallis test, q < 0.1; Supplementary Figure 2A). Further taxonomic association analyses revealed that, out of the top six most prevalent genera in the metacommunities, Haemophilus and Prevotella showed the most significant associations with clinical characteristics (Supplementary Figure 2B). In addition, we analyzed the association between the host smoking status and different metacommunities. The number of current smokers was not significantly different among metacommunities (chi-squared test, p > 0.05; Supplementary Figure 3A). However, we observed an increasing trend between smoking and the P metacommunity after clustering of the N metacommunities (chi-squared test, p = 0.052; Supplementary Figure 3B). Further clustering of smokers by “ever-smoking experience” led to even more marked distinction between the P metacommunity and the other two (chi-squared test, p = 0.032; Figure 2A and Supplementary Table 2). The significant increase in hsCRP levels in those with the P metacommunity indicated a potential association between this metacommunity and the host inflammatory status (the Kruskal–Wallis test, q < 0.1; Figure 2B).
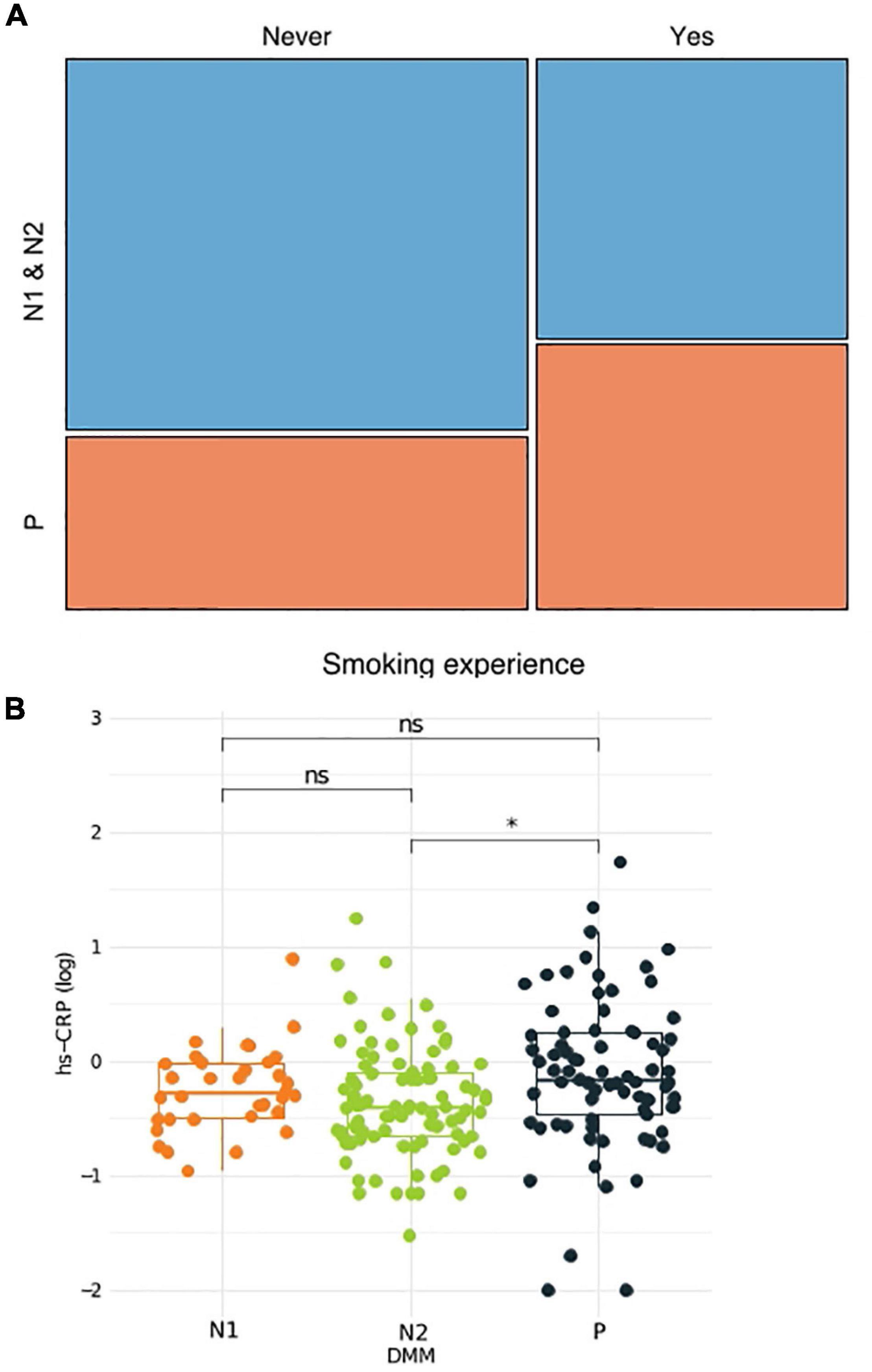
Figure 2. Association between the host health status and metacommunity. (A) Subdivision of a metacommunity and the association between each subcommunity and host smoking experience. (B) A box plot and a whisker plot showing the 25th percentile, the median, the 75th percentile, and minimum and maximum data points. *q < 0.01.
Microbial Clusters in Networks
Microbe–microbe interactions were analyzed to determine which bacterial clusters are correlated with which host traits (Figure 3). Using the WGCNA approach, we identified three bacterial clusters and defined them using different colors (Supplementary Table 3). The green cluster, to which Prevotella belongs, correlated significantly with high-density lipoprotein cholesterol (HDL) levels (negative), waist circumference (positive), systolic blood pressure (positive), diastolic blood pressure (positive), and pack-years (PYs) (positive). The cluster containing Neisseria showed a negative correlation with fasting blood sugar (FBS), TG, waist circumference, body mass index (BMI), and PYs, whereas the blue cluster (represented by TM5) showed an association with HDL only. Bacterial network analysis also identified positive interactions between Prevotella and anaerobic bacteria such as Megasphaera, Atopobium, Oribacterium, Actinomyces, and Veillonea (green cluster).
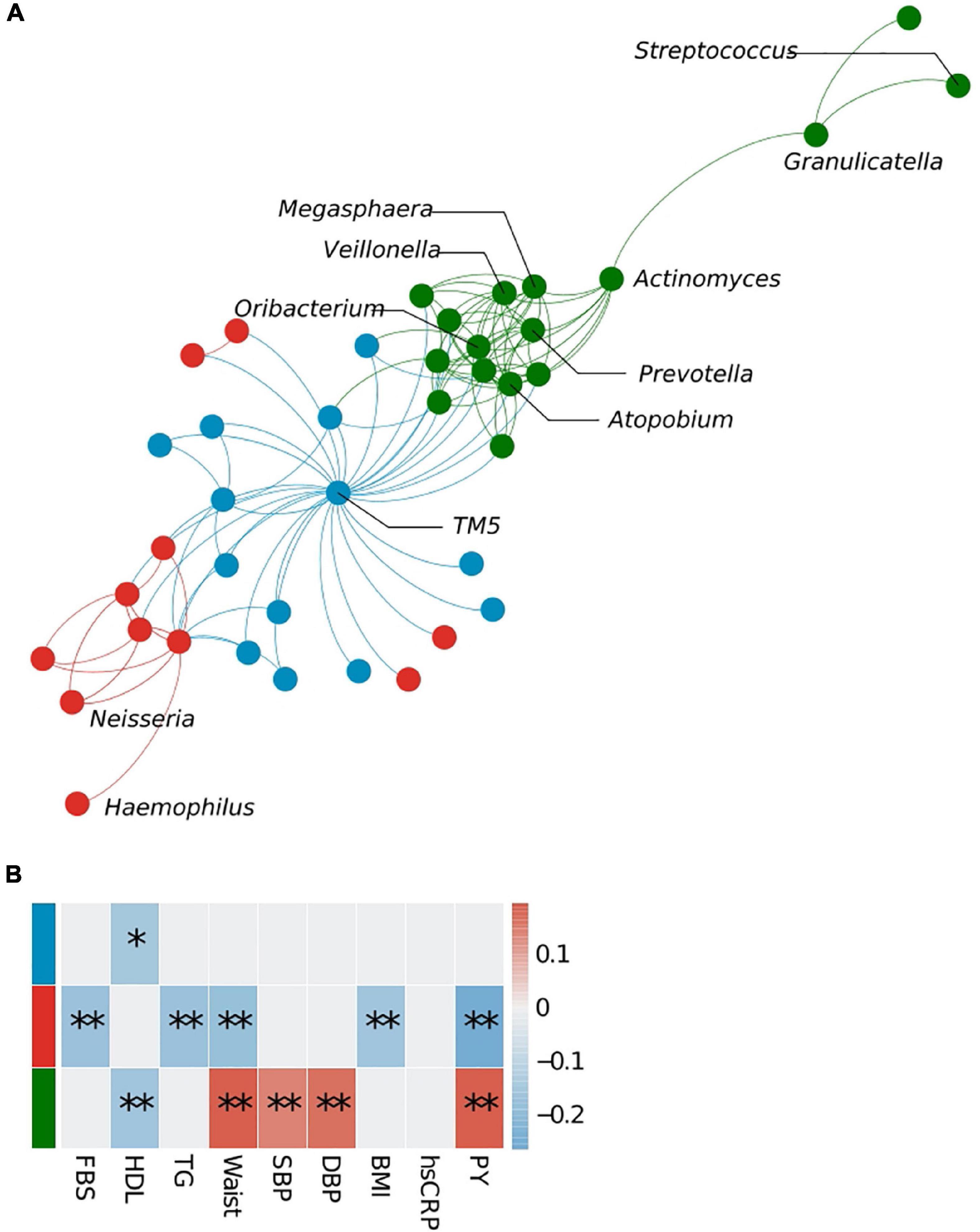
Figure 3. Bacterial cluster analysis in the sputum microbiota. (A) Microbial network at the genus level. Different colors represent different microbial clusters. (B) The Pearson correlation analysis of eigenvectors between each cluster and host clinical variables. Cluster colors (red, blue, and green) correspond with those shown in panels (A,B). FBS, fasting blood sugar; HDL, high-density lipoprotein cholesterol; TG, triglycerides; SBP, systolic blood pressure; DBP, diastolic blood pressure; PY, pack-years. *p < 0.05, **q < 0.1.
Metacommunities Within the Sputum Microbiota of an External Chronic Obstructive Pulmonary Disease Cohort
To determine whether community typing is capable of distinguishing different COPD subtypes, we examined an external COPD cohort dataset (n = 324; healthy controls, n = 121; patients with COPD, n = 203) (Haldar et al., 2020). Again, we identified three main communities in this independent cohort; however, whereas all the healthy subjects (HSs) were grouped into one metacommunity (HS), and patients with COPD were subdivided into two (CS1 and CS2) (Figure 4A; Supplementary Figure 4A). The HS metacommunity was strongly driven by Streptococcus, as reported previously (Supplementary Figure 4B) (Haldar et al., 2020). CS1 and CS2 shared common driving bacteria: Haemophilus, Veillonella, Streptococcus, and Prevotella (Supplementary Table 4); however, CS1 was more strongly driven by Serratia, while CS2 was more strongly driven by Moraxella. Community diversity analysis showed that the richness of the CS1 metacommunity was significantly higher than that of the HS metacommunity, whereas the CS2 metacommunity showed significantly lower richness and evenness than the other two (the Kruskal–Wallis test, q < 0.1; Figure 4B). An analysis of the functional potential also revealed distinct functional clusters between the two COPD metacommunities (Pairwise Adonis test, q < 0.05; Figure 4C and Supplementary Table 5). Network analysis, performed to examine microbial interactions between metacommunities (Supplementary Figure 4D; Supplementary Tables 6–8), revealed that the number of interactions was higher in the COPD metacommunities (CS1 n = 1,107 and CS2 n = 1,188) than in the HS community (n = 269). Interestingly, the number of negative interactions also increased: 37.17, 49.86, and 52.19% in the HS, CS1, and CS2 metacommunities, respectively. Moreover, the Prevotella cluster (e.g., Actinomyces, Atopobium, Veillonea, and Megasphaera) identified in the Korean twin-family cohort showed changes in their interactions with COPD-related bacteria (e.g., Haemophilus, Serratia, and Moraxella) (Figure 4D). For example, in the CS1 metacommunity, the cluster maintained its positive correlation with the COPD taxa. However, in the CS2 metacommunity, Prevotella, Megaspaera, and Atopobium developed a negative correlation with Serratia and Moraxella. Veillonella maintained its positive correlation with Moraxella in both metacommunities. These differences in microbial interactions throughout COPD metacommunities point to the disease’s complexity, which may necessitate alternative therapeutic approaches depending on the subtype.
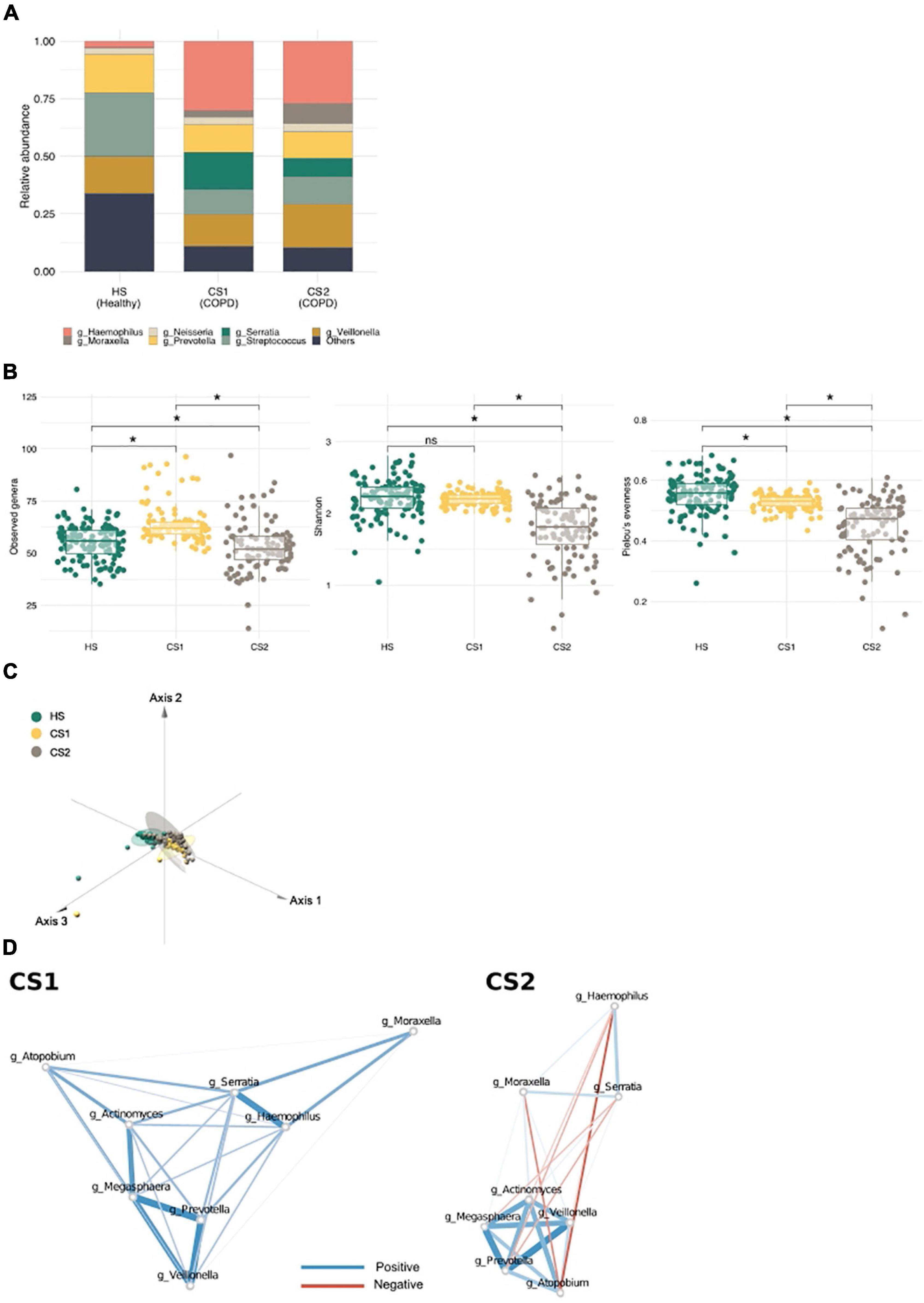
Figure 4. Metacommunities in the chronic obstructive pulmonary disease (COPD) cohort (Haldar et al.). (A) A stack bar graph showing the most abundant genera in each metacommunity. (B) Metacommunity diversity at the genus level. *q < 0.1. (C) A principal coordinate analysis (PCoA) plot showing the distribution of functional features between metacommunities. (D) The interaction of inflammatory bacteria with COPD-related taxa in CS metacommunities. Red and blue edges indicate negative and positive correlations, respectively. Edge width reflects the strength of the correlation.
Discussion
In this study, we used a community typing approach and identified three distinct metacommunities in the sputum microbiota of a healthy Korean twin-family cohort. These metacommunities were strongly driven by two dominant bacterial genera, Prevotella and Neisseria, and illustrated different ecological characteristics, as demonstrated by the community diversity. The N1 metacommunity consistently showed the greatest richness and evenness, whereas the P metacommunity occupied the other end of the spectrum. This lower community diversity was associated with host metabolic parameters similar to those observed in the gut microbiome in which reduced diversity indicates metabolic disorders. A previous study suggests that lower diversity in the sputum microbiome is associated with more severe bronchiectasis and defective lung function, as determined by FEV1 values (Dicker et al., 2021). Lower sputum bacterial diversity and evenness are also associated with an elevated level of proinflammatory cytokines (Durack et al., 2020). A link between reduced microbial diversity and impaired health could be induced by medications, host immune responses, or dominance by the pathogenic taxa (Faner et al., 2017). Therefore, the findings of reduced bacterial diversity in samples from subjects without any pulmonary disease suggest a microbial imbalance and potential associated immune changes.
Bacterial network analysis indicated potential adverse impacts driven by Prevotella and neighboring bacteria on the host health. Megasphaera (an anaerobe), Atopobium (a facultative anaerobe), Oribacterium (an anaerobe), Actinomyce (a facultative anaerobe), and Veillonella (an anaerobe), all of which were directly and strongly connected to Prevotella, are associated with smoking, lung cancer, and COPD (Morris et al., 2013; Lee et al., 2016; Wu et al., 2017). Given that the healthy lung provides an aerobic environment for the bacterial community (Huffnagle et al., 2017), anaerobic metabolism of bacteria coexisting with Prevotella supports their potential pathogenic effects in the lung. Moreover, the bacterial cluster that includes Prevotella showed more significant correlations with various host health parameters (i.e., HDL cholesterol level, waist circumference, blood pressure, and PY) than the P metacommunity. Therefore, we speculate that the negative associations of the P metacommunity with host physiology could be strongly influenced by specific bacterial interactions, with Prevotella as the keystone bacteria. Of note, we cannot exclude the possibility that such characteristics of the P metacommunity identified in this study may be induced by smoking, which has known adverse health effects.
We found it intriguing that respiratory Prevotella interacts with potential inflammatory bacteria, suggesting the potential to trigger local and systemic inflammatory conditions in a healthy population. A previous report suggests that Prevotella is associated with diseases at mucosal sites (i.e., periodontitis, complications related to rheumatoid arthritis, bacterial vaginosis, and metabolic disorders (Larsen, 2017). However, other studies show that Prevotella in the lung is protective because of its limited inflammatory capacity and its ability to increase pulmonary tolerance to respiratory pathogens (Bassis et al., 2015; Dickson et al., 2015). We found that all three metacommunities (i.e., N1, N2, and P) harbor Prevotella as one of the top driving bacteria, but with different abundance (about 20% abundance in N1 and N2 and 40% in P). Prevotella is a distinctive bacterium that lacks consensus regarding its role in inflammatory disease (Larsen, 2017). Given that our findings are based on a healthy population cohort, further studies are warranted to examine the association between host health dynamics and Prevotella abundance in the long term [including characterization at lower taxonomic levels (i.e., species or strain levels)].
Application of community typing to sputum samples collected from an external COPD cohort (Haldar et al., 2020) clearly distinguished HSs from patients; it also identified two sub-metacommunities within patients. Strong separation of the healthy population from diseased subjects confirms complete microbial dysbiosis in patients. Furthermore, the COPD sub-metacommunities themselves illustrated changes in the community diversity, functional potential, and pathogen (i.e., Serratia and Moraxella) abundance. Accordingly, we expected to find different degrees of disease severity between the two COPD metacommunities and assumed that the CS2 metacommunity would include patients with more severe COPD given its reduced microbial diversity and increased abundance of Moraxella. A previous study reported that Moraxella catarrhalis secretes an endonuclease distinct from that of Serratia, and Moraxella endonuclease was found to have enhanced cell–cell interactions and biofilm formation, which can aggravate disease conditions (Tan et al., 2019). Another previous study reported that patients with COPD with mild symptoms harbored only Serratia, not Moraxella (Kostadinova et al., 2015; Wang et al., 2016). Moreover, different numbers of coexclusions observed during network analysis of the two COPD metacommunities implies the competition triggered by environmental changes, such as disease severity. We observed that the microbial interactions, especially with the COPD pathogens, changed according to the COPD metacommunity. A recent study that identified subgroups of neutrophilic patients with COPD (i.e., neutrophilic balanced and neutrophilic Haemophilus subgroups) based on their sputum microbiome supports the possibility of distinguishing COPD severity using the microbiome data (Wang et al., 2021). The authors observed that the neutrophilic balanced subgroup had increased abundances of Prevotella and Veillonella, while these bacteria were lower in exacerbation subjects. Given that the microbial dynamics at deeper taxonomic levels change according to COPD severity (Wang et al., 2020), further studies should determine the taxa driving microbial interactions at the species or strain levels.
In conclusion, we showed that the cluster-based analysis (such as community typing) of the sputum microbiome can unmask key bacteria associated with host clinical phenotypes more effectively than the abundance-based approach; moreover, this approach shows a potential diagnostic and therapeutic application of the sputum microbiome to a heterogenic disease, such as COPD. COPD is a largely heterogeneous disease that shows a variable prognosis and response to drugs. Thus, microbial community typing of patients with COPD can help to identify those who share a similar microbial background and provide relevant targets to prevent disease exacerbation. Further studies are warranted to examine the long-term dynamics of Prevotella in host health conditions and the deeper links between distinct metacommunities and COPD phenotypes.
Data Availability Statement
The datasets presented in this study can be found in online repositories. The names of the repository/repositories and accession number(s) can be found below: https://www.ebi.ac.uk/ena, ERP010734.
Author Contributions
JS and YC analyzed the data. JS, JR, GK, and HY conceived the study objectives and study design. JS and HY prepared the draft manuscript. All authors contributed to the article and approved the submitted version.
Funding
This study was supported by the National Research Foundation of Korea (NRF) (Grant Nos. NRF-2021R1C1C2004318 and NRF-2015M3C9A4053391) and the K-BIO KIURI Center program (Project Number 2020M3H1A1073304).
Conflict of Interest
GK is a founder of KoBioLabs Inc., a company that aims to characterize the role of host–microbiome interactions in chronic diseases.
The remaining authors declare that the research was conducted in the absence of any commercial or financial relationships that could be construed as a potential conflict of interest.
Publisher’s Note
All claims expressed in this article are solely those of the authors and do not necessarily represent those of their affiliated organizations, or those of the publisher, the editors and the reviewers. Any product that may be evaluated in this article, or claim that may be made by its manufacturer, is not guaranteed or endorsed by the publisher.
Supplementary Material
The Supplementary Material for this article can be found online at: https://www.frontiersin.org/articles/10.3389/fmicb.2022.719541/full#supplementary-material
References
Arumugam, M., Raes, J., Pelletier, E., Le Paslier, D., Yamada, T., Mende, D. R., et al. (2011). Enterotypes of the human gut microbiome. Nature 473, 174–180.
Bassis, C. M., Erb-Downward, J. R., Dickson, R. P., Freeman, C. M., Schmidt, T. M., Young, V. B., et al. (2015). Analysis of the upper respiratory tract microbiotas as the source of the lung and gastric microbiotas in healthy individuals. mBio 6:e00037. doi: 10.1128/mBio.00037-15
Callahan, B. J., Mcmurdie, P. J., Rosen, M. J., Han, A. W., Johnson, A. J., and Holmes, S. P. (2016). DADA2: high-resolution sample inference from Illumina amplicon data. Nat. Meth. 13, 581–583. doi: 10.1038/nmeth.3869
Cameron, S. J. S., Lewis, K. E., Huws, S. A., Hegarty, M. J., Lewis, P. D., Pachebat, J. A., et al. (2017). A pilot study using metagenomic sequencing of the sputum microbiome suggests potential bacterial biomarkers for lung cancer. PLoS One 12:e0177062. doi: 10.1371/journal.pone.0177062
DeSantis, T. Z., Hugenholtz, P., Larsen, N., Rojas, M., Brodie, E. L., Keller, K., et al. (2006). Greengenes, a chimera-checked 16S rRNA gene database and workbench compatible with ARB. Appl. Environ. Microbiol. 72, 5069–5072. doi: 10.1128/AEM.03006-05
Dicker, A. J., Lonergan, M., Keir, H. R., Smith, A. H., Pollock, J., Finch, S., et al. (2021). The sputum microbiome and clinical outcomes in patients with bronchiectasis: a prospective observational study. Lancet Respir. Med. 9, 885–896. doi: 10.1016/S2213-2600(20)30557-9
Dickson, R. P., Erb-Downward, J. R., Freeman, C. M., Mccloskey, L., Beck, J. M., Huffnagle, G. B., et al. (2015). Spatial Variation in the Healthy Human Lung Microbiome and the Adapted Island Model of Lung Biogeography. Ann. Am. Thorac. Soc. 12, 821–830. doi: 10.1513/AnnalsATS.201501-029OC
Dixon, P. (2003). VEGAN, a package of R functions for community ecology. J. Veg. Sci. 14, 927–930. doi: 10.1111/j.1654-1103.2003.tb02228.x
Durack, J., Christian, L. S., Nariya, S., Gonzalez, J., Bhakta, N. R., Ansel, K. M., et al. (2020). Distinct associations of sputum and oral microbiota with atopic, immunologic, and clinical features in mild asthma. J. Allergy Clin. Immunol. 146, 1016–1026. doi: 10.1016/j.jaci.2020.03.028
Faner, R., Sibila, O., Agustí, A., Bernasconi, E., Chalmers, J. D., Huffnagle, G. B., et al. (2017). The microbiome in respiratory medicine: current challenges and future perspectives. Eur. Respir. J. 49:1602086. doi: 10.1183/13993003.02086-2016
Friedman, J., and Alm, E. J. (2012). Inferring correlation networks from genomic survey data. PLoS Comput. Biol. 8:e1002687. doi: 10.1371/journal.pcbi.1002687
Haldar, K., George, L., Wang, Z., Mistry, V., Ramsheh, M. Y., Free, R. C., et al. (2020). The sputum microbiome is distinct between COPD and health, independent of smoking history. Respir. Res. 21:183. doi: 10.1186/s12931-020-01448-3
Han, M. K., Agusti, A., Calverley, P. M., Celli, B. R., Criner, G., Curtis, J. L., et al. (2010). Chronic Obstructive Pulmonary Disease Phenotypes. Am. J. Respir. Crit. Care Med. 182, 598–604.
Hansen, B. B., and Klopfer, S. O. (2006). Optimal Full Matching and Related Designs via Network Flows. J. Comput. Graph. Stat. 15, 609–627. doi: 10.1198/106186006x137047
Holmes, I., Harris, K., and Quince, C. (2012). Dirichlet multinomial mixtures: generative models for microbial metagenomics. PLoS One 7:e30126. doi: 10.1371/journal.pone.0030126
Huffnagle, G. B., Dickson, R. P., and Lukacs, N. W. (2017). The respiratory tract microbiome and lung inflammation: a two-way street. Mucosal Immunol. 10, 299–306. doi: 10.1038/mi.2016.108
Iwai, S., Weinmaier, T., Schmidt, B. L., Albertson, D. G., Poloso, N. J., Dabbagh, K., et al. (2016). Piphillin: improved Prediction of Metagenomic Content by Direct Inference from Human Microbiomes. PLoS One 11:e0166104. doi: 10.1371/journal.pone.0166104
Kostadinova, V., Radkov, Y., Stoeva, T., Miteva, D., and Dyakova, A. (2015). Sputum microbiology in patients with stabile COPD. Eur. Respir. J. 46:A2532.
Krishna, P., Jain, A., and Bisen, P. S. (2016). Microbiome diversity in the sputum of patients with pulmonary tuberculosis. Eur. J. Clin. Microbiol. Infect. Dis. 35, 1205–1210. doi: 10.1007/s10096-016-2654-4
Kuczynski, J., Stombaugh, J., Walters, W. A., González, A., Caporaso, J. G., and Knight, R. (2011). Using QIIME to analyze 16S rRNA gene sequences from microbial communities. Curr. Protoc. Bioinform. Chapter 10:Unit10.17.
Langfelder, P., and Horvath, S. (2008). WGCNA: an R package for weighted correlation network analysis. BMC Bioinform. 9:559. doi: 10.1186/1471-2105-9-559
Larsen, J. M. (2017). The immune response to Prevotella bacteria in chronic inflammatory disease. Immunology 151, 363–374. doi: 10.1111/imm.12760
Lee, S. H., Sung, J. Y., Yong, D., Chun, J., Kim, S. Y., Song, J. H., et al. (2016). Characterization of microbiome in bronchoalveolar lavage fluid of patients with lung cancer comparing with benign mass like lesions. Lung Cancer 102, 89–95. doi: 10.1016/j.lungcan.2016.10.016
Leitao Filho, F. S., Alotaibi, N. M., Ngan, D., Tam, S., Yang, J., Hollander, Z., et al. (2019). Sputum Microbiome Is Associated with 1-Year Mortality after Chronic Obstructive Pulmonary Disease Hospitalizations. Am. J. Respir. Crit. Care Med. 199, 1205–1213. doi: 10.1164/rccm.201806-1135OC
Lim, M. Y., Yoon, H. S., Rho, M., Sung, J., Song, Y. M., Lee, K., et al. (2016). Analysis of the association between host genetics, smoking, and sputum microbiota in healthy humans. Sci. Rep. 6:23745. doi: 10.1038/srep23745
Martinez Arbizu, P. (2020). pairwiseAdonis: Pairwise Multilevel Comparison using Adonis. R Package Version 0.4. Available online at: https://github.com/pmartinezarbizu/pairwiseAdonis
McMurdie, P. J., and Holmes, S. (2013). phyloseq: an R package for reproducible interactive analysis and graphics of microbiome census data. PLoS One 8:e61217. doi: 10.1371/journal.pone.0061217
Mirza, S., and Benzo, R. (2017). Chronic Obstructive Pulmonary Disease Phenotypes: implications for Care. Mayo Clin. Proc. 92, 1104–1112. doi: 10.1016/j.mayocp.2017.03.020
Morris, A., Beck, J. M., Schloss, P. D., Campbell, T. B., Crothers, K., Curtis, J. L., et al. (2013). Comparison of the respiratory microbiome in healthy nonsmokers and smokers. Am. J. Respir. Crit. Care Med. 187, 1067–1075. doi: 10.1164/rccm.201210-1913OC
Pang, Z., Wang, G., Gibson, P., Guan, X., Zhang, W., Zheng, R., et al. (2019). Airway Microbiome in Different Inflammatory Phenotypes of Asthma: a Cross-Sectional Study in Northeast China. Int. J. Med. Sci. 16, 477–485. doi: 10.7150/ijms.29433
Quinn, R. A., Adem, S., Mills, R. H., Comstock, W., Deright Goldasich, L., Humphrey, G., et al. (2019). Neutrophilic proteolysis in the cystic fibrosis lung correlates with a pathogenic microbiome. Microbiome 7:23. doi: 10.1186/s40168-019-0636-3
Ravel, J., Gajer, P., Abdo, Z., Schneider, G. M., Koenig, S. S. K., Mcculle, S. L., et al. (2011). Vaginal microbiome of reproductive-age women. Proc. Natl. Acad. Sci. U.S.A. 108, 4680–4687.
Segal, L. N., Alekseyenko, A. V., Clemente, J. C., Kulkarni, R., Wu, B., Chen, H., et al. (2013). Enrichment of lung microbiome with supraglottic taxa is associated with increased pulmonary inflammation. Microbiome 1:19. doi: 10.1186/2049-2618-1-19
Shannon, P., Markiel, A., Ozier, O., Baliga, N. S., Wang, J. T., Ramage, D., et al. (2003). Cytoscape: a software environment for integrated models of biomolecular interaction networks. Genom. Res. 13, 2498–2504. doi: 10.1101/gr.1239303
Sidhaye, V. K., Nishida, K., and Martinez, F. J. (2018). Precision medicine in COPD: where are we and where do we need to go? Eur. Respir. Rev. 27:180022. doi: 10.1183/16000617.0022-2018
Sung, J., Cho, S. I., Lee, K., Ha, M., Choi, E. Y., Choi, J. S., et al. (2006). Healthy Twin: a twin-family study of Korea–protocols and current status. Twin Res. Hum. Genet. 9, 844–848. doi: 10.1375/183242706779462822
Tan, A., Li, W. S., Verderosa, A. D., Blakeway, L. V. T. D. M., Totsika, M., and Seib, K. L. (2019). Moraxella catarrhalis NucM is an entry nuclease involved in extracellular DNA and RNA degradation, cell competence and biofilm scaffolding. Sci. Rep. 9:2579. doi: 10.1038/s41598-019-39374-0
Valles-Colomer, M., Falony, G., Darzi, Y., Tigchelaar, E. F., Wang, J., Tito, R. Y., et al. (2019). The neuroactive potential of the human gut microbiota in quality of life and depression. Nat. Microbiol. 4, 623–632. doi: 10.1038/s41564-018-0337-x
Vandeputte, D., Kathagen, G., D’hoe, K., Vieira-Silva, S., Valles-Colomer, M., Sabino, J., et al. (2017). Quantitative microbiome profiling links gut community variation to microbial load. Nature 551:507. doi: 10.1038/nature24460
Vieira-Silva, S., Sabino, J., Valles-Colomer, M., Falony, G., Kathagen, G., Caenepeel, C., et al. (2019). Quantitative microbiome profiling disentangles inflammation- and bile duct obstruction-associated microbiota alterations across PSC/IBD diagnoses. Nat. Microbiol. 4, 1826–1831. doi: 10.1038/s41564-019-0483-9
Wang, Q., Garrity, G. M., Tiedje, J. M., and Cole, J. R. (2007). Naive Bayesian classifier for rapid assignment of rRNA sequences into the new bacterial taxonomy. Appl. Environ. Microbiol. 73, 5261–5267. doi: 10.1128/AEM.00062-07
Wang, Z., Bafadhel, M., Haldar, K., Spivak, A., Mayhew, D., Miller, B. E., et al. (2016). Lung microbiome dynamics in COPD exacerbations. Eur. Respir. J. 47, 1082–1092. doi: 10.1183/13993003.01406-2015
Wang, Z., Liu, H., Wang, F., Yang, Y., Wang, X., Chen, B., et al. (2020). A Refined View of Airway Microbiome in Chronic Obstructive Pulmonary Disease at Species and Strain-Levels. Front. Microbiol. 11:1758. doi: 10.3389/fmicb.2020.01758
Wang, Z., Locantore, N., Haldar, K., Ramsheh, M. Y., Beech, A. S., Ma, W., et al. (2021). Inflammatory Endotype–associated Airway Microbiome in Chronic Obstructive Pulmonary Disease Clinical Stability and Exacerbations: a Multicohort Longitudinal Analysis. Am. J. Respir. Crit. Care Med. 203, 1488–1502. doi: 10.1164/rccm.202009-3448OC
Wu, X., Chen, J., Xu, M., Zhu, D., Wang, X., Chen, Y., et al. (2017). 16S rDNA analysis of periodontal plaque in chronic obstructive pulmonary disease and periodontitis patients. J. Oral Microbiol. 9:1324725. doi: 10.1080/20002297.2017.1324725
Keywords: sputum microbiome, community typing, Prevotella, metacommunity, inflammation, network, COPD metacommunity in sputum microbiome
Citation: Si J, Choi Y, Raes J, Ko G and You HJ (2022) Sputum Bacterial Metacommunities in Distinguishing Heterogeneity in Respiratory Health and Disease. Front. Microbiol. 13:719541. doi: 10.3389/fmicb.2022.719541
Received: 02 June 2021; Accepted: 02 March 2022;
Published: 31 March 2022.
Edited by:
Tian Zhang, Wuhan University of Technology, ChinaReviewed by:
Sunil D. Saroj, Symbiosis International University, IndiaHai-Yue Liu, First Affiliated Hospital of Xiamen University, China
Jin Su, Southern Medical University, China
Copyright © 2022 Si, Choi, Raes, Ko and You. This is an open-access article distributed under the terms of the Creative Commons Attribution License (CC BY). The use, distribution or reproduction in other forums is permitted, provided the original author(s) and the copyright owner(s) are credited and that the original publication in this journal is cited, in accordance with accepted academic practice. No use, distribution or reproduction is permitted which does not comply with these terms.
*Correspondence: Gwangpyo Ko, Z2tvQHNudS5hYy5rcg==; Hyun Ju You, ZGhsb3ZlcjFAc251LmFjLmty