- 1Department of Animal Science, College of Agriculture and Natural Resources, University of Tehran, Karaj, Iran
- 2Biomedical Center for Systems Biology Science Munich, Ludwig-Maximilians-University, Munich, Germany
- 3Faculty of Science, Earth Sciences Building, University of British Columbia, Vancouver, BC, Canada
- 4Faculty of Paramedical Sciences, Kurdistan University of Medical Sciences, Kurdistan, Iran
- 5Department of Basic Scientific Sciences, AL-Balqa Applied University, AL-Huson University College, AL-Huson, Jordan
- 6Department of Animal and Poultry Science, College of Aburaihan, University of Tehran, Tehran, Iran
- 7Halal Research Center of IRI, FDA, Tehran, Iran
- 8Department of Food Hygiene and Quality Control, Faculty of Veterinary Medicine, University of Tehran, Tehran, Iran
- 9Department of Agronomy and Plant Breeding, College of Agriculture and Natural Resources, University of Tehran, Karaj, Iran
- 10Department of Production Animal Health, Faculty of Veterinary Medicine, University of Calgary, Calgary, AB, Canada
- 11Regional Department of Bioengineering, Tecnológico de Monterrey, Monterrey, Mexico
Objective: Bovine tuberculosis (bTB) is a chronic respiratory infectious disease of domestic livestock caused by intracellular Mycobacterium bovis infection, which causes ~$3 billion in annual losses to global agriculture. Providing novel tools for bTB managements requires a comprehensive understanding of the molecular regulatory mechanisms underlying the M. bovis infection. Nevertheless, a combination of different bioinformatics and systems biology methods was used in this study in order to clearly understand the molecular regulatory mechanisms of bTB, especially the immunomodulatory mechanisms of M. bovis infection.
Methods: RNA-seq data were retrieved and processed from 78 (39 non-infected control vs. 39 M. bovis-infected samples) bovine alveolar macrophages (bAMs). Next, weighted gene co-expression network analysis (WGCNA) was performed to identify the co-expression modules in non-infected control bAMs as reference set. The WGCNA module preservation approach was then used to identify non-preserved modules between non-infected controls and M. bovis-infected samples (test set). Additionally, functional enrichment analysis was used to investigate the biological behavior of the non-preserved modules and to identify bTB-specific non-preserved modules. Co-expressed hub genes were identified based on module membership (MM) criteria of WGCNA in the non-preserved modules and then integrated with protein–protein interaction (PPI) networks to identify co-expressed hub genes/transcription factors (TFs) with the highest maximal clique centrality (MCC) score (hub-central genes).
Results: As result, WGCNA analysis led to the identification of 21 modules in the non-infected control bAMs (reference set), among which the topological properties of 14 modules were altered in the M. bovis-infected bAMs (test set). Interestingly, 7 of the 14 non-preserved modules were directly related to the molecular mechanisms underlying the host immune response, immunosuppressive mechanisms of M. bovis, and bTB development. Moreover, among the co-expressed hub genes and TFs of the bTB-specific non-preserved modules, 260 genes/TFs had double centrality in both co-expression and PPI networks and played a crucial role in bAMs-M. bovis interactions. Some of these hub-central genes/TFs, including PSMC4, SRC, BCL2L1, VPS11, MDM2, IRF1, CDKN1A, NLRP3, TLR2, MMP9, ZAP70, LCK, TNF, CCL4, MMP1, CTLA4, ITK, IL6, IL1A, IL1B, CCL20, CD3E, NFKB1, EDN1, STAT1, TIMP1, PTGS2, TNFAIP3, BIRC3, MAPK8, VEGFA, VPS18, ICAM1, TBK1, CTSS, IL10, ACAA1, VPS33B, and HIF1A, had potential targets for inducing immunomodulatory mechanisms by M. bovis to evade the host defense response.
Conclusion: The present study provides an in-depth insight into the molecular regulatory mechanisms behind M. bovis infection through biological investigation of the candidate non-preserved modules directly related to bTB development. Furthermore, several hub-central genes/TFs were identified that were significant in determining the fate of M. bovis infection and could be promising targets for developing novel anti-bTB therapies and diagnosis strategies.
Introduction
Bovine tuberculosis (bTB) is domestic livestock’s infectious and chronic respiratory disease, especially in beef and dairy cattle (Brosch et al., 2002; Waters et al., 2012; Hall et al., 2021), which has paramount economic, animal welfare, and public health consequences. Despite implementing management strategies to control and eradicate it, bTB is still a major global health threat to animal populations (Vegh et al., 2015; Lu et al., 2021). Econometric analysis has ranked bTB as the fourth most important cattle disease, causing ~$3 billion in annual losses to global agriculture (Garnier et al., 2003; McLoughlin et al., 2014). bTB is caused by infection with Mycobacterium bovis, a pathogenic intracellular mycobacterial species belonging to Mycobacterium tuberculosis complex (MTBC; Smith et al., 2006; Djelouadji et al., 2011). Previous studies have shown that at the nucleotide level, M. bovis has a genome sequence 99.95% identical to M. tuberculosis, the infectious agent of human tuberculosis (TB; Garnier et al., 2003; Hall et al., 2020), and many features of M. tuberculosis infection in human are also characteristic of M. bovis infection in cattle (Pollock and Neill, 2002; Killick et al., 2014; Waters et al., 2014; Buddle et al., 2016). Therefore, as a zoonotic agent, M. bovis has serious implications for human health (Olea-Popelka et al., 2017; Vayr et al., 2018).
Considering the significant perturbation that occur in the normal functioning of alveolar macrophages in response to M. bovis infection, greater understanding the molecular regulatory mechanisms of interactions between M. bovis and bovine alveolar macrophages (bAMs) as well as identification of transcriptional biomarkers could be a fundamental step in the development of next-generation diagnostics and therapeutic strategies against bTB, thereby providing novel tools for disease management (Walzl et al., 2011; Nalpas et al., 2015).
The main hypothesis is that the reprogramming of bAMs by M. bovis occurs through extensive changes in the expression of the genes of these cells (Nalpas et al., 2015). In a previous study, Magee et al. (2014) used the RT-PCR protocol to investigate changes in gene expression in M. bovis-infected bAMs and reported a significant upregulation of several innate immune genes including TLR2, CCL4, IL1B, IL6, and TNF and a significant downregulation of PIK3IP1 and FOS genes in infected samples (Magee et al., 2014). Moreover, the recent development of genome-scale high-throughput functional genomic technologies, such as gene expression microarrays and RNA sequencing (RNA-seq), which can generate a deep and global gene expression profile (Quesnel-Vallières et al., 2019), have enabled the whole-transcriptome analysis in bovine and human cells (especially macrophages) in response to M. bovis (Magee et al., 2012; Nalpas et al., 2013; Vegh et al., 2015; Shukla et al., 2017; Malone et al., 2018; Wiarda et al., 2020; McLoughlin et al., 2021b; Abdelaal et al., 2022) and M. tuberculosis (Sharma et al., 2017; Papp et al., 2018; Wang Z. et al., 2018) infection. It is worth mentioning that most of these transcriptome studies are based on differential gene expression analysis between different conditions. This method focuses only on the individual effects of genes rather than the effect of clusters of genes (Bakhtiarizadeh et al., 2020). Indeed, genes and their associated proteins interact in complex communication/biological networks (Alm and Arkin, 2003). It is expected that systematic investigation at the networks level can better and more comprehensively explain the etiology of complex diseases and identify new disease genes and drug targets more accurately (Vinayagam et al., 2016; Li M. et al., 2018).
More recently, the integration of experimental-analytical approaches with computational algorithms has been the main perspective of systems biology to understand disease biology or other complex traits (Eissing et al., 2011). Current systems biological approaches apply network theories to multi-omics data that help advance the understanding of disease (Joshi et al., 2021). Networks are computational models that organize complex biological information quantitatively (Barabási et al., 2011). One of the influential network theories to infer system-level gene–disease associations from genome-wide gene expression is the gene co-expression network approach (van Dam et al., 2017), which is based on correlation patterns among the expression of genes (Li J. et al., 2018). Gene co-expression network-based methods have been widely used to process gene expression data obtained from microarray (Wei et al., 2015; Han, 2019; Jaime-Lara et al., 2020) and RNA-seq (Wan et al., 2018; Franco et al., 2020; Kong et al., 2020) techniques in various animal and human diseases. In this regard, a well-known and helpful co-expression network-based method is weighted gene co-expression network analysis (WGCNA; Langfelder and Horvath, 2008). This method is based on expression similarities and considers differences in the response of samples at different time points by measuring the connectivity among the genes based on gene expression correlation patterns across samples and classifying highly correlated genes into specific clusters called modules (Zhang and Horvath, 2005; Langfelder and Horvath, 2008). Furthermore, the WGCNA method can identify intramodular highly connected genes (hub genes) within the modules based on the intramodular gene connectivity, which is centrally located in the module and have the most biological relationship with the relevant trait compared to other genes in that module (Langfelder and Horvath, 2008).
Systemic approaches for disease-based studies are based on the idea that disease-perturbed protein/gene regulatory networks are different from their normal conditions (Hood et al., 2004). In this regard, WGCNA has a specific network approach called module preservation analysis, which is one of the aspects of differential network analysis and is based on changes in network topological features between different conditions (healthy vs. disease; Langfelder et al., 2011). In other words, the network preservation analysis assess whether the topological properties of the modules, such as connectivity patterns and network density in a reference set (normal conditions), are preserved in a test set (disease conditions; Langfelder et al., 2011). Therefore, the presence of the topological changes in some modules (non-preserved modules) between normal and disease conditions indicates a systemic perturbation in the co-expression patterns of that modules by the disease (Hasankhani et al., 2021b). The non-preserved modules have been highlighted as key modules for investigating complex molecular mechanisms in many diseases (Mukund and Subramaniam, 2015; Riquelme Medina and Lubovac-Pilav, 2016; Bakhtiarizadeh et al., 2018; Hasankhani et al., 2021a).
In the present study, the main assumption was that the non-preserved modules could be important candidates for a better understanding of the bTB immunomodulatory mechanisms and may also contain genes that play key roles in the M. bovis pathogenesis. For this purpose, for the first time, we used a combination of RNA-seq data obtained from bAMs with the network preservation method of WGCNA and functional enrichment analysis to identify non-preserved modules biologically related to the molecular mechanisms behind the interactions of alveolar macrophages and M. bovis. Moreover, for deeper exploration, protein–protein interaction (PPI) networks derived from the co-expressed hub genes of candidate non-preserved modules were extracted to identify crucial genes and transcription factors (TFs) that had a double centrality (hub-central genes/TFs) in both co-expression and PPI networks. This study can help us to better understand of the novel molecular regulatory mechanisms underlying bTB and accelerate the discovery of sensitive genes that lead to the immunopathogenesis of M. bovis infection.
Materials and methods
Gene expression datasets
Raw RNA-seq data from alveolar macrophages of unrelated and age-matched Holstein-Friesian male calves from a TB-free herd that were challenged with M. bovis AF2122/97 strain in vitro, were obtained from the Gene Expression Omnibus (GEO) database at the National Center for Biotechnology Information (NCBI) under the accession number of GSE62506. The data included samples from 39 M. bovis-infected and 39 non-infected control of 10 bAMs at 2, 6, and 24 hpi and 9 bAMs at 48 hpi. An Illumina® HiSeq™ 2000 was used for RNA sequencing, and a total of 1.8 billion paired-end (2 × 90 bp) reads were generated from 78 libraries. More information of preparing data can be found in the source paper (Nalpas et al., 2015).
RNA-seq data analysis and preprocessing
To ensure the quality of the RNA-seq data, FastQC1 software (version 0.11.9) was used to quality control of the raw reads. Next, in order to obtain high-quality clean reads, low-quality raw reads/bases (Q < 20) and adapter contamination were trimmed by Trimmomatic software (version 0.39; Bolger et al., 2014) with the following parameters: ILLUMINACLIP:Adapter.fa:2:30:10, SLIDINGWINDOW:6:20, TRAILING:20, and MINLEN:60. FastQC was used again to check the quality of the clean reads and confirm improvements. Next, the paired-end clean reads were aligned to the latest bovine reference genome (ARS-UCD1.2, release-106 from Ensemble database) using Hisat2 software (version 2.2.1; Kim et al., 2019). Finally, ENSEMBL bovine GTF (release 106) and Hisat2 SAM files were used as input for the python script HTSeq-count (version 0.13.5; Anders et al., 2014) to count the uniquely mapped reads to annotated genes using intersection-strict mode. Then, all the count files were merged into a table, and a raw gene expression matrix was created that contained read counts information of all genes for all samples. In the next step, the “voom” function of the limma R package (version 3.46.0) was used for normalization of the raw gene expression matrix to log counts per million (log-CPM; Smyth, 2005; Law et al., 2014). This normalization method estimates the mean–variance relationship of the log-counts. It generates a precision weight for each observation, therefore, works better than the RNA-seq count-based methods, opening access to the RNA-seq gene expression data to computational methods (such as WGCNA) initially developed for microarrays (Law et al., 2014). Additionally, to prevent negative effects of sampling noise and unreal correlations caused by low expressed and low variance genes for the co-expression network construction, genes with expression levels ≥1 CPM in at least five samples and standard deviation >0.25 were selected for further analysis.
Weighted gene co-expression network analysis
Based on the assumption that (1) M. bovis may cause systemic perturbation in the topological structure between non-infected control and M. bovis-infected bAMs, (2) non-preserved modules can help to better understand the molecular mechanisms of bTB, and (3) may contain important genes that lead to the development of diagnostics strategies and therapeutic methods against M. bovis pathogenesis, non-infected control samples (n = 39) were selected as a reference set for WGCNA analysis and module detection. A weighted gene co-expression network was constructed in the non-infected control samples using the WGCNA R package (version 1.70; Langfelder and Horvath, 2008) procedures in the following steps:
1. Considering the sensitivity of WGCNA to outliers, adjacency matrices of samples were constructed using the “adjacency” function of the WGCNA R package and sample network connectivity was standardized according to the distances. Samples with a standardized connectivity score < −2.5 were defined as an outlier and excluded from the downstream analysis. Afterward, the “goodSamplesGenes” function of the WGCNA R package was used to ensure the absence of samples and genes with >50% missing entries and zero variance.
2. To construct a co-expression network with a scale-free topology, β = 13 was calculated using the “pickSoftThreshold” function of the WGCNA R package as an acceptable soft-thresholding power β value.
3. The weighted adjacency matrix at β = 13 was constructed using the bi-weight mid-correlation coefficient, which is much more robust to the outliers than the Pearson correlation (Song et al., 2012), and then transformed to into the topological overlap matrix (TOM).
4. A signed weighted gene co-expression network was constructed using average linkage hierarchical clustering analysis based on the TOM dissimilarity (1-TOM), and modules with different sizes were detected through a dynamic hybrid tree cutting algorithm.
5. Finally, modules with highly similar expression profiles were identified and then merged based on the correlation between the module eigengenes (the first principal component of the gene expression profile for a given module).
All the above steps were performed using automatic, one-step network construction and module detection function “blockwiseModules” of the WGCNA R package with the following major parameters: networkType = “signed,” TOMType = “signed,” corType “bicor,” power = 13, maxBlockSize = 16,000, reassignThreshold = 0, mergeCutHeight = 0.25, and minModuleSize 30.
Module preservation analysis
Network preservation analysis was performed using a permutation test based on 200 random permutations via “modulePreservation” function of the WGCNA R package. This network-based approach examines whether the mean connection strength among all genes in a module (known as network density) and the sum of the connection strengths for a gene with other network genes (known as connectivity) are preserved between non-infected control bAMs (n = 39) as a reference set and M. bovis-infected bAMs (n = 39) as a test set through two composite module preservation statics including Zsummary and medianRank (Langfelder et al., 2011). To get an accurate result of testing the preservation level between the respective conditions, especially when modules are compared with different sizes, the Zsummary statistic, which is highly dependent on the module size and increases with increasing module size, should be combined with the medianRank statistic, which is module-size independent (Langfelder et al., 2011). Overall, higher Zsummary values and lower medianRnak values indicates a high level of preservation between different conditions, so modules with Zsummary > 10 or medianRank <8 are considered highly-preserved (Langfelder et al., 2011). Therefore, in the current study, modules with Zsummary ≤ 10 or medianRank ≥8 were defined as non-preserved between non-infected control and M. bovis-infected samples.
Functional enrichment analysis of the non-preserved modules and TFs prediction
To investigate and interpret the biological behavior of non-preserved modules and to identify bTB-specific non-preserved modules, the co-expressed genes in each non-preserved module were analyzed using the Enrichr2 online tool based on the Gene ontology (GO) terms for biological process and Kyoto Encyclopedia of Genes and Genomes (KEGG) pathway enrichment (Chen et al., 2013). A significant threshold for functional terms was defined as an adjusted p-value < 0.05 (corrected by the Benjamini–Hochberg method). Additionally, a set of bovine TFs extracted from the AnimalTFDB3.03 database was used to identify co-regulated TFs that played a crucial immunoregulatory role in the non-preserved modules (Hu et al., 2018).
Identification of hub-central genes in the bTB-specific non-preserved modules
In the co-expression modules, highly connected intramodular genes, also known as hub genes, which have the highest degree of connection compared to other genes in that module, are expected to play important roles in the complex biological mechanisms of that module (Bi et al., 2015; Das et al., 2017). The central role of intramodular hub genes in candidate modules has led them to be used as potential novel biomarkers to accelerate the development of the next-generation diagnostics and therapeutic strategies against various diseases (Li S. et al., 2017; Wang L.-X. et al., 2018; Miao et al., 2019). In this regard, multiple steps were performed to identify genes with double centrality (hub-central) in the candidate non-preserved modules associated with M. bovis infection.
1. Module memberships (MM) or eigengene-based connectivity kME criterion, which interprets the relationship between modules and genes (Langfelder and Horvath, 2008), was calculated through the correlation between the gene expression profile and the module eigengenes by WGCNA R package. Taken together, co-expressed genes with values of kME ≥ 0.7 were defined as highly connected intramodular hub genes in the non-preserved modules.
2. In order to explore network density and protein interactions, the co-expressed hub genes of the bTB-specific non-preserved modules were subjected to PPI network construction using Search Tool for the Retrieval of Interacting Genes (STRING) database4 with medium stringency options (Szklarczyk et al., 2018).
3. Maximal clique centrality (MCC) is one of the novel local-based topological algorithms for node centrality in a network that has better performance than other topological algorithms for identifying the PPI networks hub genes (Chin et al., 2014) and has been proposed to increase the sensitivity and specificity for discovering featured nodes (Chin et al., 2014; Li and Xu, 2019). For this purpose, co-expressed hub genes-based PPI networks of each candidate non-preserved module were inputted into Cytoscape5 software (version 3.7.1) and interpreted with the cytoHubba plugin (version 0.1; Chin et al., 2014) based on the MCC algorithm to identify co-expressed intramodular hub genes with the highest MCC score (hub-central genes).
4. Next, the top 50 genes in co-expressed hub genes-based PPI networks of candidate non-preserved modules with a size of ≥350 and the top 20 genes in co-expressed hub genes-based PPI networks of candidate non-preserved modules with a size of ≤200 in terms of MCC score were considered as hub-central genes. Besides, co-expressed hub genes-based PPI networks of the bTB-related non-preserved modules were visualized using Cytoscape software (version 3.7.1; Cline et al., 2007).
Validation of RNA-Seq results using quantitative real-time PCR
To validate the reproducibility of RNA-seq data, five DEGs including BCL2L1, MMP1, EDN1, MAPK8, and CTSS were selected for analysis by qRT-PCR. The same RNAs extracted from bovine alveolar at each non-infected control vs. Mycobacterium bovis-infected animals were used for qRT-PCR validation. Quantitative reverse-transcription-PCR was carried out according to the manufacturer specifications for reference to SYBR® Premix Ex TaqTM. SYBR Green PCR cycling was denatured using a program of 95°C for 10 s, 35 cycles of 95°C for 5 s, and 60°C for 40 s, and performed on an ABI 7500 instrument (United States). The specificity of each PCR product was confirmed by melting curve analysis. All qRT-PCR assays were performed in triplicate reactions. The housekeeping genes RPL19 and GAPDH were used as the internal control genes. The expression levels of target mRNAs were obtained based on RNAs extracted from bovine alveolar and were shown to be normalized to GAPDH. Forward and reverse primer sequences and accession numbers of selected genes are given in Supplementary Table S1.
Results
RNA-seq data summary
Overall details of RNA-seq data analysis and weighted gene co-expression network construction steps are schematically presented in Figure 1. Totally 1,769,182,596 RNA-seq raw reads of 39 non-infected control bAMs and 39 M. bovis-infected bAMs (an average of 23 million paired-end reads per sample) were retrieved and processed. After quality control and trimming, a total of 1,751,490,782 high quality clean reads were obtained. On average, 85% of the clean reads were uniquely aligned to the bovine reference genome, and the overall mapping rate was 94%. complete details of RNA-seq data and preprocessing are provided in Supplementary Table S2. In order to minimize the sampling noise, different parameters were applied and a total of 10,563 genes were kept for co-expression network analysis. The final normalized gene expression profile is available in Supplementary Table S3.
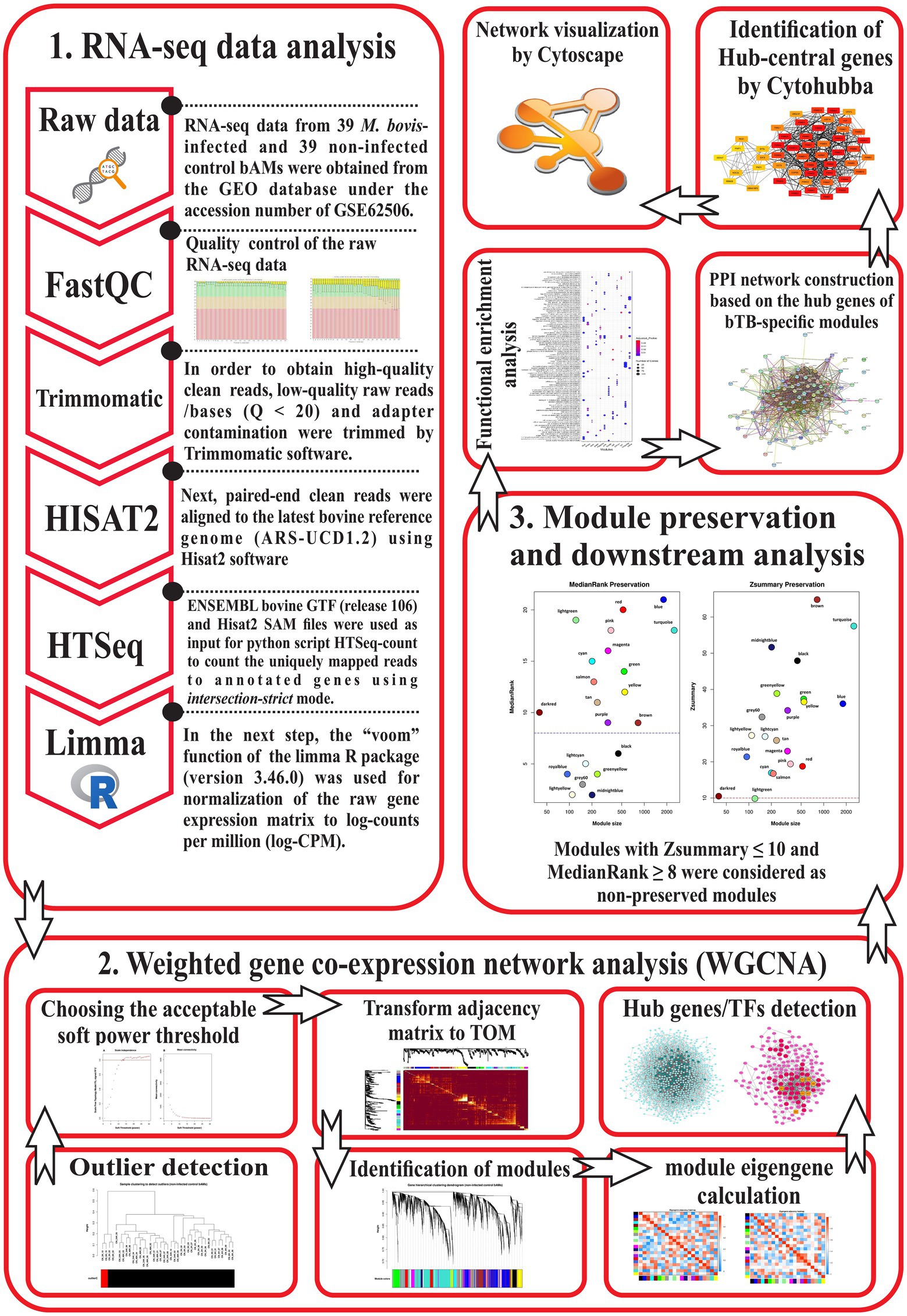
Figure 1. Schematic pipeline of RNA-seq data analysis and weighted gene co-expression network construction steps.
Weighted gene co-expression network construction and module detection
To better understand the molecular mechanisms underlying bTB, the normalized and filtered gene expression matrix obtained from RNA-seq data analysis were combined with WGCNA approaches. Based on the details obtained from the adjacency matrices of samples, two samples, GSM1528042 and GSM1528044, had a standardized connectivity score < −2.5 and were excluded as an outlier (Figure 2; Supplementary Table S4). The weighted gene co-expression network was constructed based on the TOM dissimilarity at β = 13, which represents a scale free topology fitting index (R2) ≥ 0.80 (Figure 3; Supplementary Table S5), and a total of 21 modules (excluding 576 uncorrelated genes in gray module) in different sizes were identified in the non-infected control samples as the reference set through hierarchical clustering analysis and dynamic hybrid tree cutting algorithms, and each module was labeled with a specific color by WGCNA method. The identified co-expression modules as branches of the gene hierarchical clustering dendrogram are shown in Figure 4. The average size of each module was 476 genes and turquoise module with a size of 2,521 genes and darkred module with a size of 40 genes were identified as the largest and smallest module, respectively. Complete information of the identified modules is presented in Supplementary Table S6.
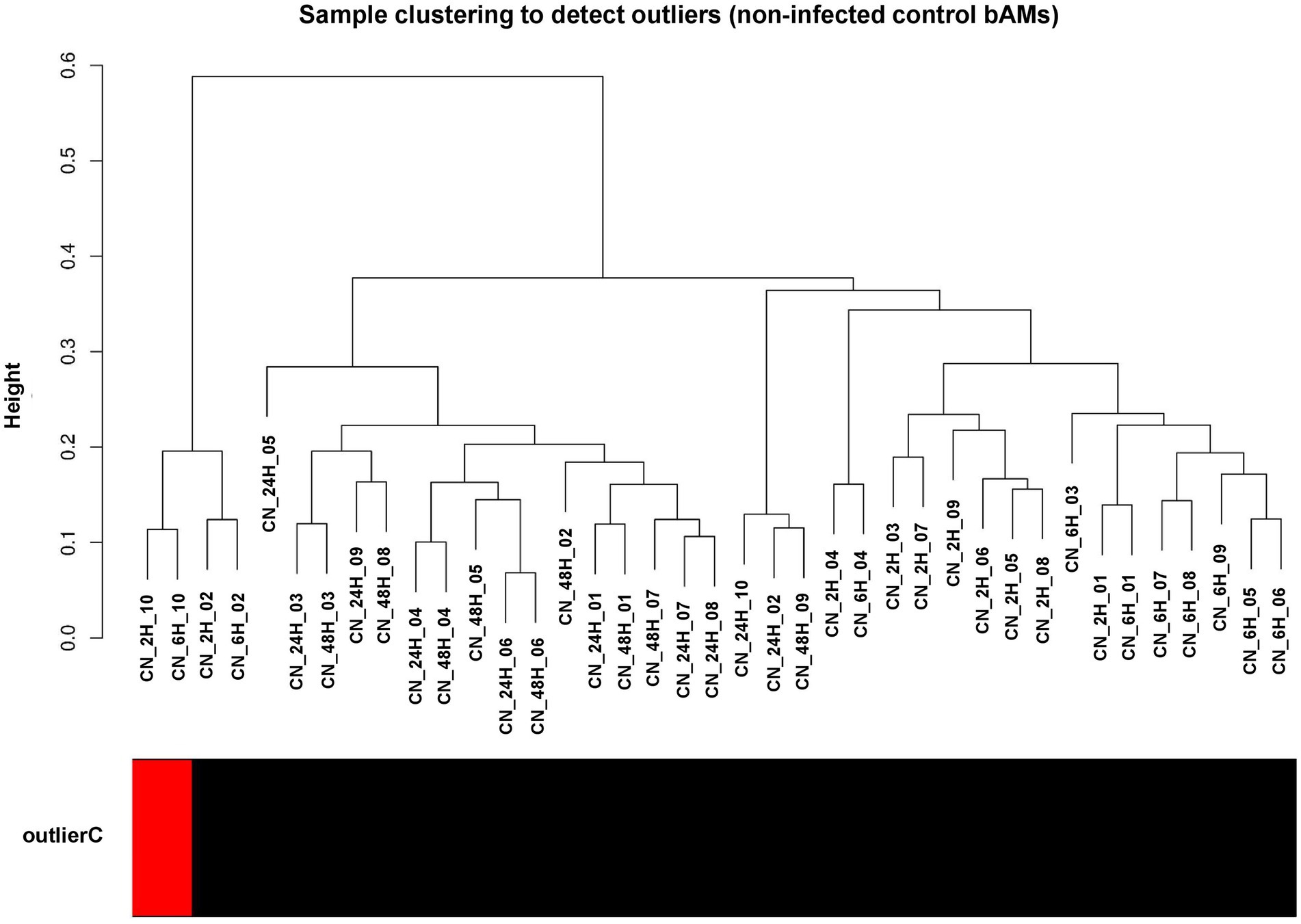
Figure 2. Sample clustering to detect outliers in the non-infected controls bAMs as reference set for module detection. Based on the adjacency matrices of samples, two samples including GSM1528042 and GSM1528044 had a standardized connectivity score < −2.5 and were excluded as outlier.
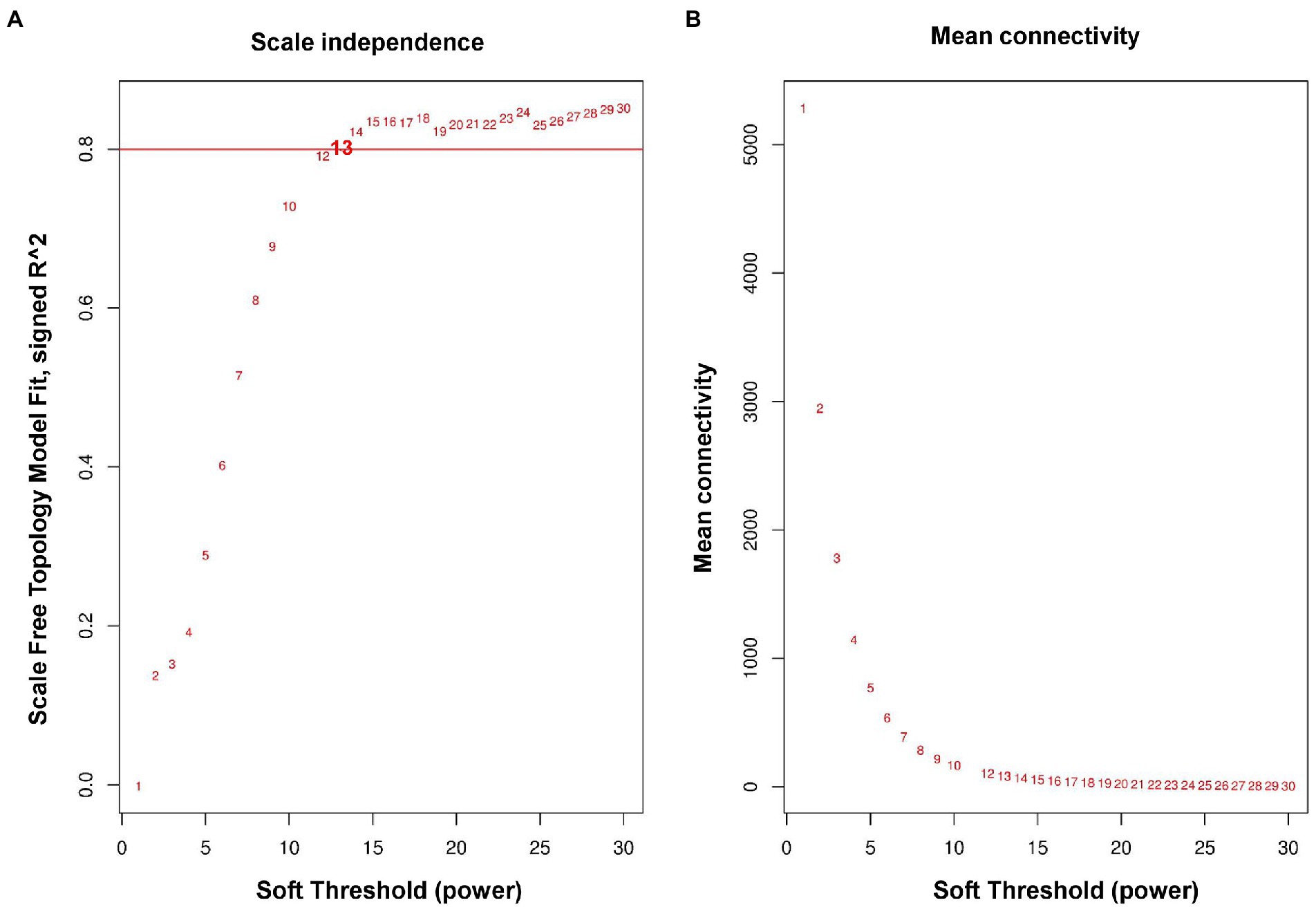
Figure 3. Network topology analysis. (A) Scale-free topology fitting index (R2) (y-axis) and (B) mean connectivity (y-axis) for different soft-threshold powers (β) (x-axis). The weighted gene co-expression network was constructed at β = 13, which represents a scale free topology fitting index (R2) ≥ 0.80.
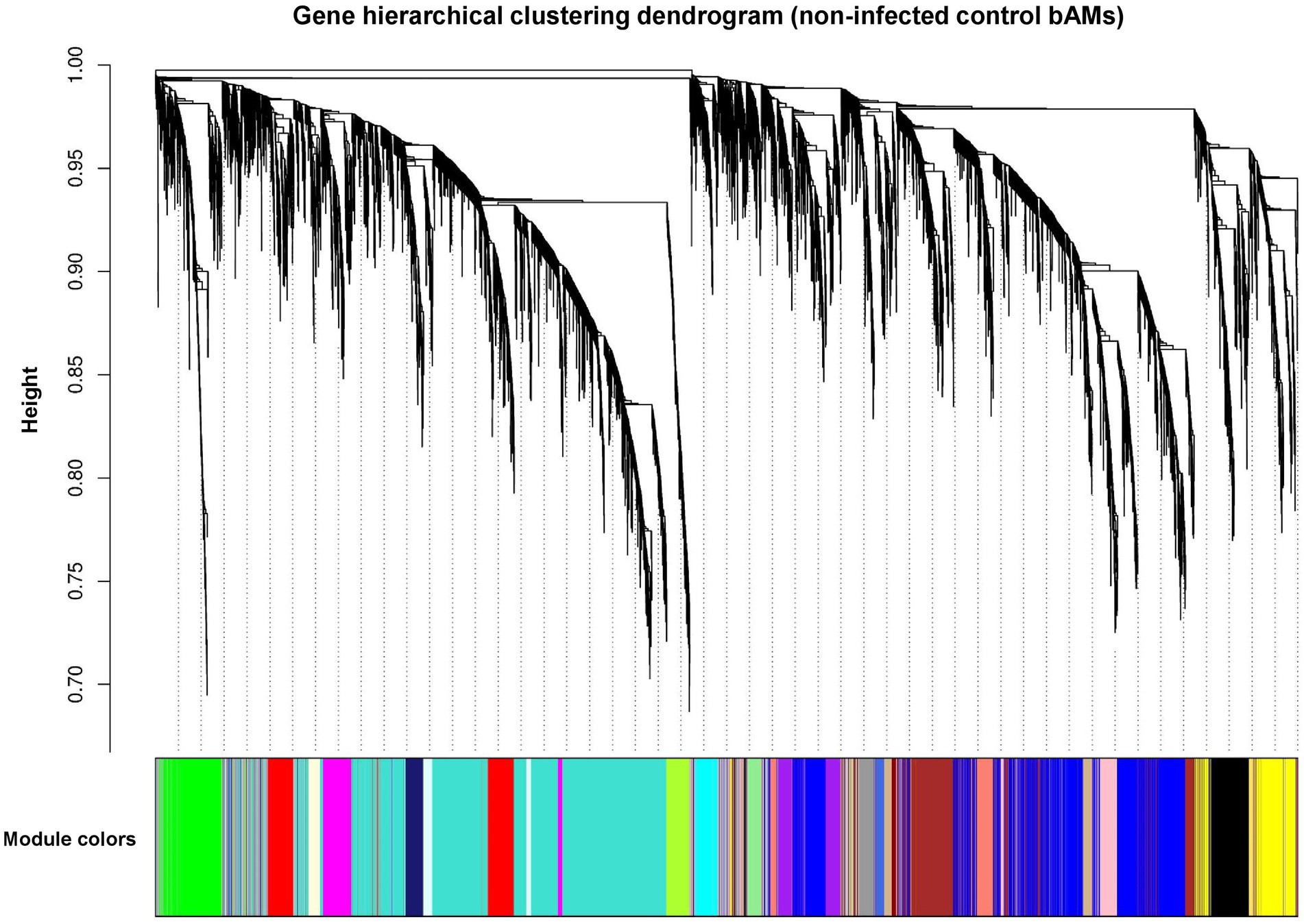
Figure 4. Gene hierarchical clustering dendrogram of detected modules across all samples. A total of 21 modules in different sizes were identified based on the TOM dissimilarity (1-TOM) in the non-infected control bAM samples as reference set through hierarchical clustering analysis and dynamic hybrid tree cutting algorithms. The x-axis represents the genes and the y-axis represents the co-expression distance. The branches indicate the modules, and each module was labeled with a unique color. The gray module including 576 genes indicate uncorrelated genes.
Network preservation analysis
Module preservation analysis was performed to investigate changes in network properties between non-infected control samples (n = 39) as a reference set and M. bovis-infected samples (n = 39) as a test set. The results showed that among 21 modules identified in non-infected control samples, the network density and connectivity patterns of 14 modules were altered in M. bovis-infected samples, making them key candidates for studying the biological mechanisms of bTB disease. Accordingly, the topological structure of 7 modules, including lightyellow, midnightblue, gray60, greenyellow, royalblue, lightcyan, and black, was highly preserved between the respective conditions (Figure 5). Among the highly preserved modules, lightyellow and midnightblue modules had the highest degree of topological preservation between non-infected control and M. bovis-infected samples. On the other hand, in agreement with our primary assumption, 14 modules, including brown, purple, darkred, tan, yellow, salmon, green, cyan, magenta, pink, turquoise, lightgreen, red, and blue were systematically perturbed by M. bovis infection (Figure 5). Moreover, the blue module with a size of 1805 co-expressed genes had the most significant alteration in network characteristics in response to M. bovis infection. Further details of the module preservation analysis are provided in Supplementary Table S7.
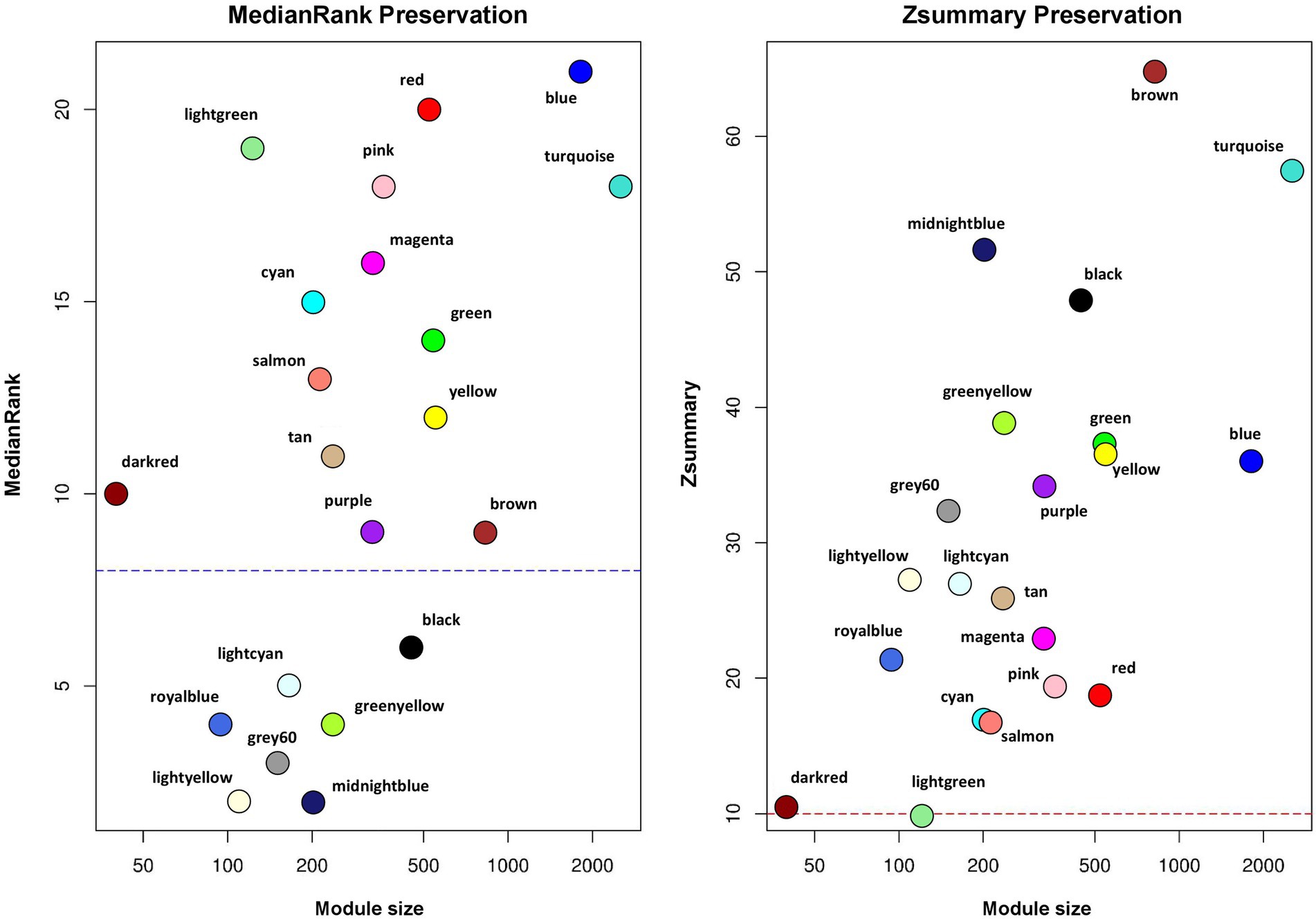
Figure 5. Module preservation analysis. (A) The medianRank preservation statistics. The y-axis represents medianRank values for different modules and the x-axis represents module size. Each point indicates a module labeled by a unique color. The blue dashed line represents the medianRank threshold. (B) The Zsummary preservation statistics. The y-axis represents Zsummary values for different modules and the x-axis represents module size. Each point indicates a module labeled by a unique color. The red dashed line represents the Zsummary threshold. Modules with Zsummary ≤ 10 or medianRank ≥8 were considered as non-preserved between non-infected control and Mycobacterium bovis-infected conditions.
Functional enrichment analysis of the non-preserved modules
Functional enrichment analysis was performed to investigate biological processes and KEGG pathways to detect the specific molecular mechanisms of the non-preserved modules and the functional differentiation between them. In total, 642 biological processes were significantly enriched in 12 non-preserved modules. No biological processes were significantly enriched in the other two non-preserved modules, the darkred and lightgreen modules. Furthermore, the KEGG pathway enrichment analysis showed that 194 pathways were significantly enriched in 11 non-preserved modules, including blue, brown, green, red, pink, purple, salmon, tan, turquoise, yellow, and magenta. Interestingly, the most non-preserved module between non-infected control and M. bovis-infected bAMs, the blue module, had the highest enrichment rate in the KEGG pathways and biological processes (234 and 50 biological processes and KEGG pathways, respectively). The top 10 significant biological processes and KEGG pathway GO terms of the non-preserved modules are shown in Figures 6, 7, respectively. Based on the interpretation of the functional enrichment results, out of 14 non-preserved modules, 7 non-preserved modules, including blue, brown, green, pink, salmon, tan, and turquoise, were directly related to the host immune response, immunomodulatory mechanisms of M. bovis infection, and bTB development. Some of these critical pathways and processes were included “Apoptosis,” “Ferroptosis,” “regulation of cell cycle phase transition (GO:1901987),” “negative regulation of mitotic cell cycle phase transition (GO:1901991),” “negative regulation of cell cycle G2/M phase transition (GO:1902750),” “positive regulation of Wnt signaling pathway (GO:0030177),” “JAK–STAT signaling pathway,” “PI3K-Akt signaling pathway,” “negative regulation of programmed cell death (GO:0043069),” “negative regulation of apoptotic process (GO:0043066),” “T cell receptor signaling pathway,” “regulation of T cell activation (GO:0050863),” “Th17 cell differentiation,” “Th1 and Th2 cell differentiation,” “Natural killer cell mediated cytotoxicity,” “gamma-delta T cell activation (GO:0046629),” “positive regulation of interferon-gamma production (GO:0032729),” “B cell receptor signaling pathway (GO:0050853),” “Toll-like receptor signaling pathway,” “C-type lectin receptor signaling pathway,” “NOD-like receptor signaling pathway,” “RIG-I-like receptor signaling pathway,” “Cytosolic DNA-sensing pathway,” “IL-17 signaling pathway,” “NF-kappa B signaling pathway,” “MAPK signaling pathway,” “negative regulation of type I interferon production (GO:0032480),” “Necroptosis,” “Fatty acid degradation,” “fatty acid catabolic process (GO:0009062),” “fatty acid beta-oxidation (GO:0006635),” “regulation of lipid metabolic process (GO:0019216),” “fatty acid oxidation (GO:0019395),” “cholesterol metabolic process (GO:0008203),” “secondary alcohol biosynthetic process (GO:1902653),” “fatty acid beta-oxidation using acyl-CoA oxidase (GO:0033540),” “regulation of cholesterol biosynthetic process (GO:0045540),” “cellular amino acid catabolic process (GO:0009063),” “Tryptophan metabolism,” “Valine, leucine and isoleucine degradation,” “Glycine, serine and threonine metabolism,” “Autophagy,” “regulation of macroautophagy (GO:0016241),” and “regulation of autophagy (GO:0010506).” Complete information from the results of the functional enrichment analysis of non-preserved modules is presented in Supplementary Table S8.
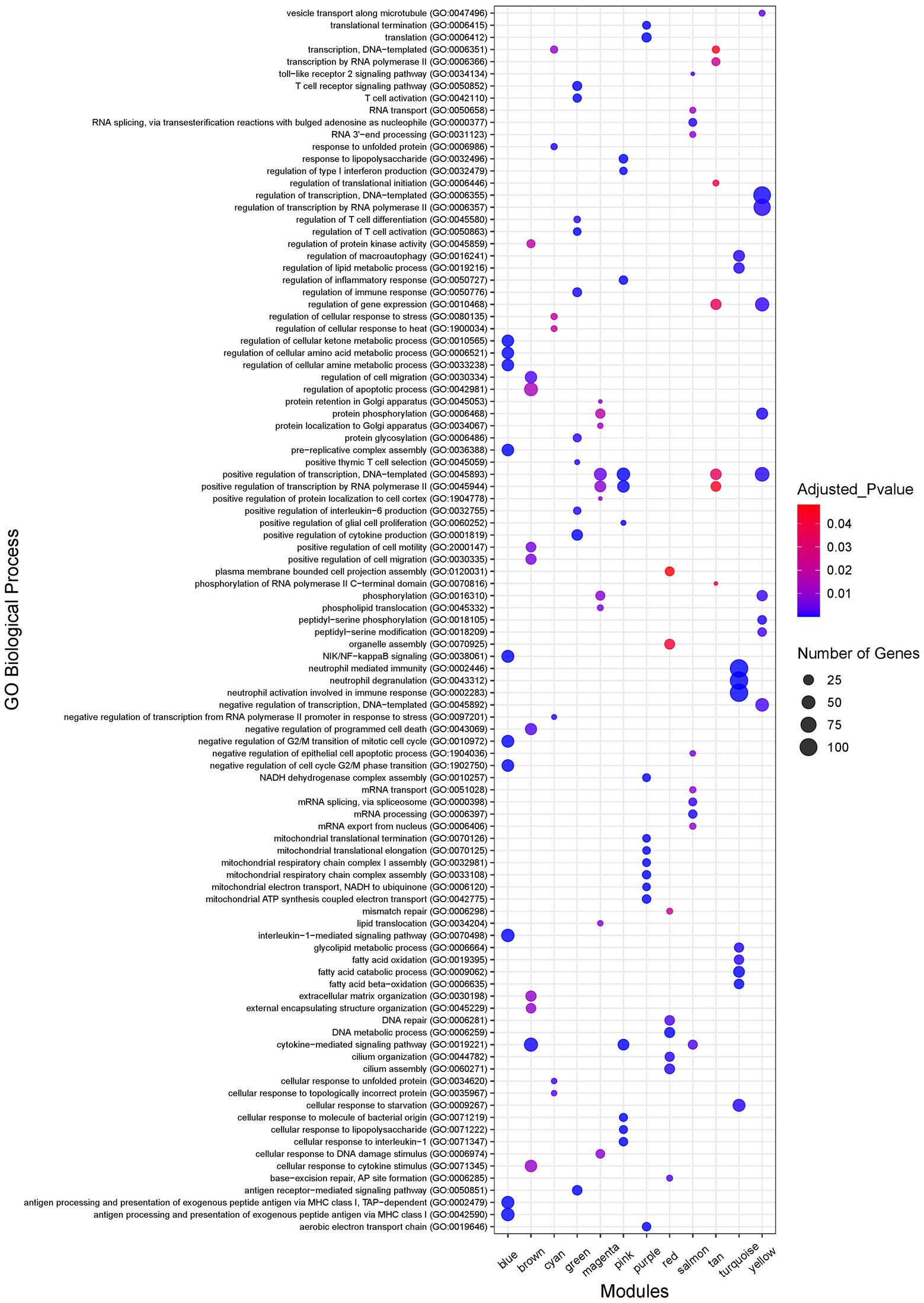
Figure 6. Dot plot of functional enrichment analysis. The top 10 significant biological process GO terms of the non-preserved modules. The y-axis represents significant enriched GO terms and the x-axis represents module name. Color and size of each point represent adjusted p-value and number of genes for each term, respectively.
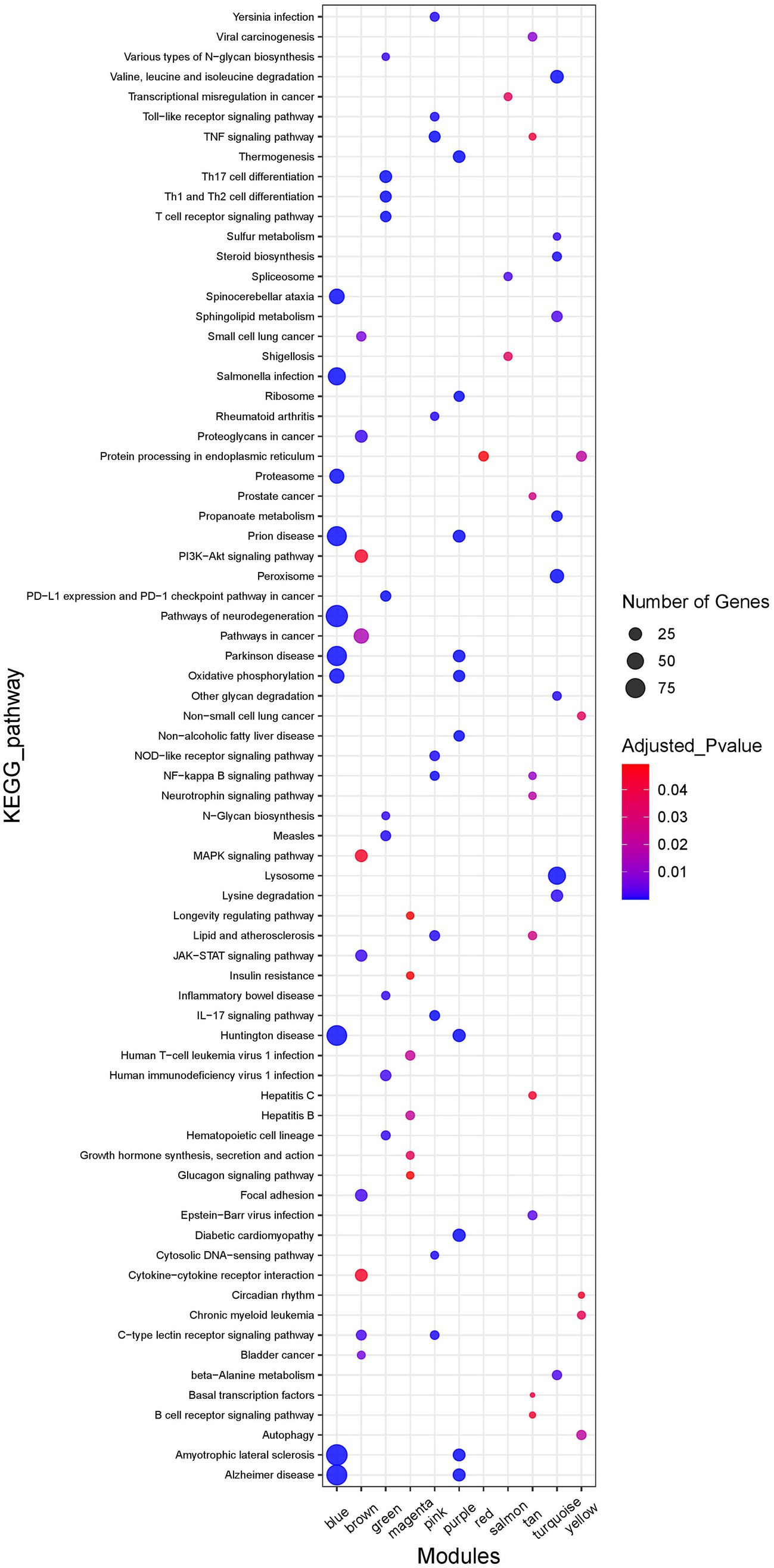
Figure 7. Dot plot of functional enrichment analysis. The top 10 significant KEGG pathway GO terms of the non-preserved modules. The y-axis represents significant enriched GO terms and the x-axis represents module name. Color and size of each point represent adjusted p-value and number of genes for each term, respectively.
Identification of TFs, hub TFs, hub genes, and hub-central genes in the candidate non-preserved modules
In this study, module preservation and functional enrichment analysis identified 7 candidate non-preserved modules, including blue, brown, green, pink, salmon, tan, and turquoise, that were biologically related to bTB pathogenesis. To identify crucial intramodular hub genes that played a central role in the biological function of these modules, the MM criterion was calculated by the WGCNA R package. A total of 3,653 co-expressed hub genes were identified with kME ≥ 0.7 in all non-preserved modules (Supplementary Table S9). Taken together, a total of 725, 382, 170, 222, 140, 134, and 938 highly connected hub genes were screened in the blue, brown, green, pink, salmon, tan, and turquoise candidate non-preserved modules, respectively. Additionally, important TFs that regulated the transcription of co-expressed genes in the non-preserved modules were extracted based on the bovine transcriptional regulatory factors of AnimalTFDB3.0 database, and a total of 491 TFs were identified in all non-preserved modules (Supplementary Table S10). Besides, among the co-expressed hub genes identified in the non-preserved modules, a total of 22, 12, 8, 26, 6, 14, and 29 TFs (hub TFs) were detected in the blue, brown, green, pink, salmon, tan, and turquoise bTB-specific non-preserved modules, respectively (Supplementary Table S11). Intriguingly, the co-expressed intramodular hub genes of the 7 candidate non-preserved modules were densely connected in the PPI networks based on the STRING database information, indicating close biological relationships between proteins encoded by these genes. Eventually, 260 hub-central genes were identified in the bTB-specific non-preserved modules, which have a double centrality in both PPI and co-expression networks and could be key candidates for better understanding the complex etiology of bTB, development of diagnostics and potential therapeutic targets for M. bovis infection (Table 1; Supplementary Table S12). Moreover, the co-expressed hub genes-based PPI networks of the bTB-specific modules are displayed in Figure 8.
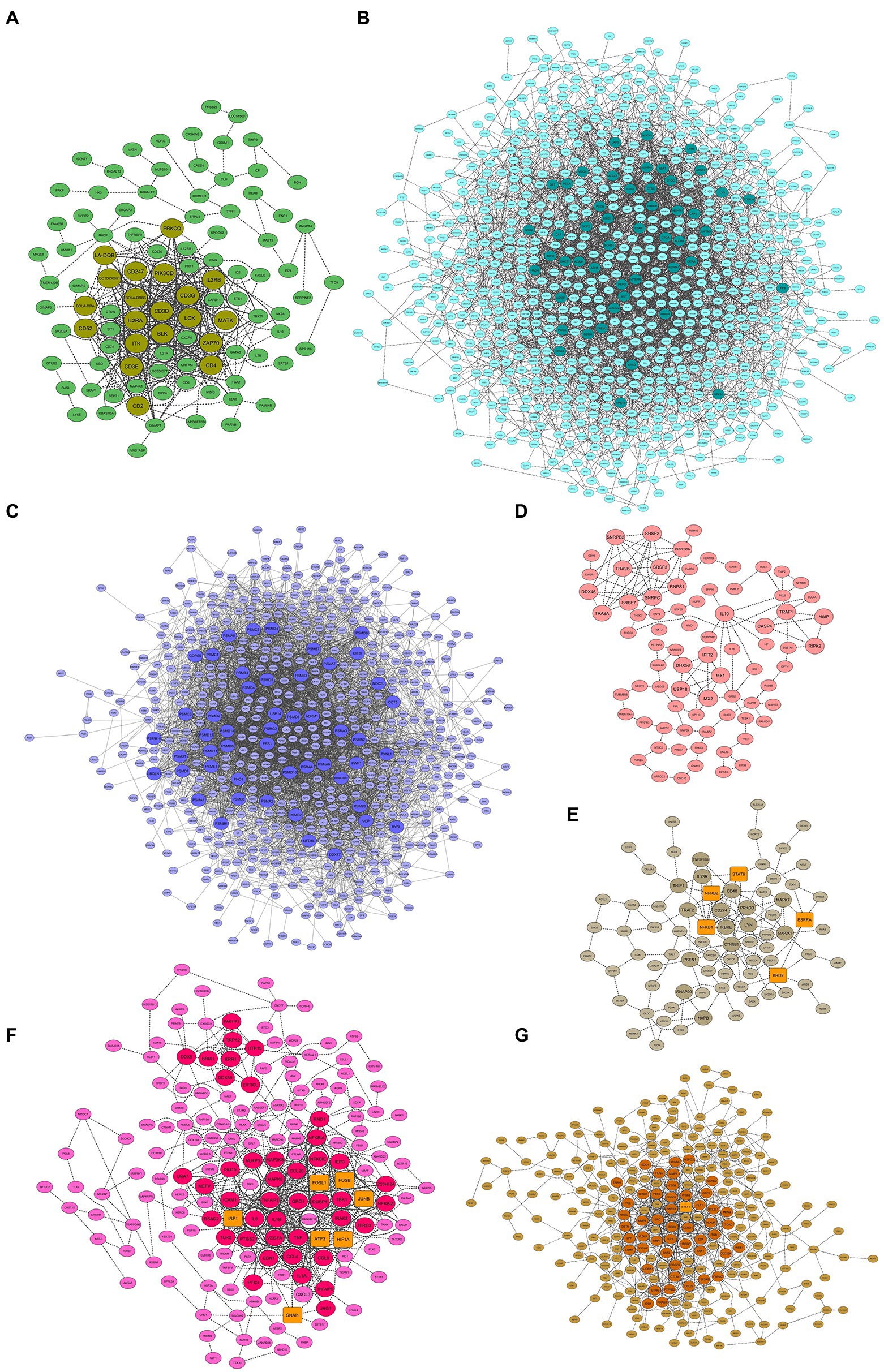
Figure 8. PPI network derived from co-expressed hub genes of the (A) green, (B) turquoise, (C) blue, (D) salmon, (E) tan, (F) pink, and (G) brown modules. Large circles and orange round rectangles represent hub-central genes and TFs, respectively.
Analysis of expression based on qRT-PCR data
To assess the accuracy and the reliability of differential expression genes identified by RNA-seq, five DEGs from non-infected control vs. Mycobacterium bovis-infected samples were selected to perform qRT-PCR tests. The expression results for five genes were assessed using RNA-seq and qRT-PCR and are shown in Figure 9. As can be observed, the expression patterns of five genes showed a general agreement between the two technologies.
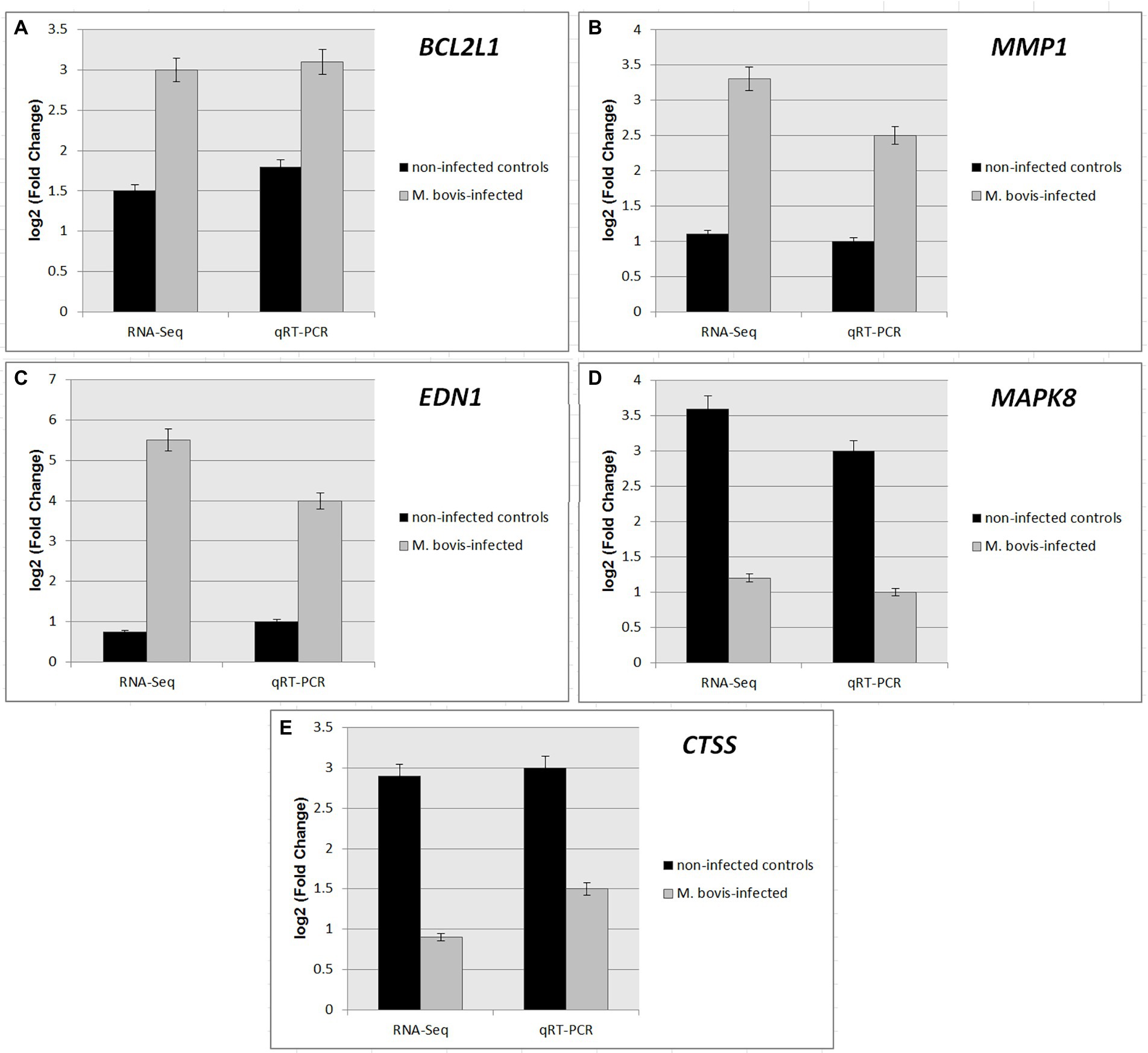
Figure 9. Differentially expressed genes were selected from transcriptome comparison combinations at different non-infected control vs. M. bovis-infected samples. The black-filled columns represent the relative mRNA expression levels obtained by qRT-PCR, which were normalized by GAPDH; (A) BCL2L1 gene expression, (B) MMP1 gene, (C) EDN1 gene expression, (D) MAPK8 gene expression, (E) CTSS gene expression, The gray columns show the log2 (FC) value obtained by RNA-seq.
Discussion
bTB is a severe infectious disease caused by infection with M. bovis and inflicts irreparable economic losses on the dairy and beef cattle industry (Middleton et al., 2021). However, an insufficient understanding of the molecular regulatory mechanisms behind bTB has been one of the main reasons for the limitation of various techniques to control or eradication this disorder in recent decades (Schiller et al., 2010; Fang et al., 2020). Combining high-throughput technologies with novel computational systems biology approaches provide new opportunities to better understand the molecular mechanisms underlying various diseases (Sharifi et al., 2019). Therefore, in this study, a combination of RNA-seq data with module preservation analysis (a network-based method of WGCNA) was used to obtain a comprehensive insight into the complex mechanisms involved in the interactions of bovine host and M. bovis infection. Briefly, a signed weighted gene co-expression network was constructed and a total of 21 modules were identified in the non-infected control bAM samples as a reference set for network preservation analysis. Generally, signed networks provide a better understanding of the biological mechanisms behind traits/diseases at the systematic level and differentiate the potential functions of the modules better and more accurately (Mason et al., 2009). In agreement with the main hypothesis of this study, M. bovis infection in bAMs was able to change the network characteristics of 67% of the identified modules (14 out of 21) compared to the non-infected control bAMs. Then, functional enrichment analysis based on the biological processes and KEGG pathways showed that among the 14 non-preserved modules, 7 non-preserved modules, including blue, brown, green, pink, salmon, tan, and turquoise were directly involved in host-pathogen interactions and could be important candidates for studying pathogenic mechanisms of bTB as in previous similar studies, these candidate non-preserved modules were successfully used as key modules to describe the complex etiology of several bovine diseases, such as bovine mastitis (Bakhtiarizadeh et al., 2020), bovine respiratory disease (BRD; Hasankhani et al., 2021b), bovine endometritis (Sheybani et al., 2021), and Johne’s disease (Heidari et al., 2021). It should be noted that loss of connection or alteration of the connectivity patterns and network density in the non-preserved modules can be attributed to the abnormal expression of several genes in M. bovis-infected conditions, which can be key factors in the development of bTB. Therefore, several steps were performed to identify these key dysregulated genes, including identification of intramodular hub genes in the non-preserved modules, integration of co-expressed hub genes with PPI networks, and identification of the hub-central genes in the bTB-specific co-expressed hub genes-based PPI networks through MCC topological algorithm. Noteworthy, in parallel with the current study, the MCC algorithm has been used as an objective criterion for measuring node centrality and identifying important genes/proteins in candidate networks in disease-based system biology studies (Bai et al., 2020; Yang et al., 2020; Ma et al., 2021; Wang Y. et al., 2021). Finally, a total of 260 hub-central genes were found in the 7 bTB-specific non-preserved modules that these genes were hubs in their co-expression networks and also played a central role in the respective co-expressed hub genes-based PPI networks (so-called double centrality) which were as critical targets in related to the promotion of the bTB establishment.
Co-expressed genes in the blue module showed high enrichment in KEGG pathways such as “Apoptosis,” “Ferroptosis,” “Tuberculosis,” and “Proteasome,” as well as biological processes including “regulation of cell cycle phase transition (GO:1901987),” “negative regulation of mitotic cell cycle phase transition (GO:1901991),” “negative regulation of cell cycle G2/M phase transition (GO:1902750),” “positive regulation of Wnt signaling pathway (GO:0030177),” and “Fc-gamma receptor signaling pathway involved in phagocytosis (GO:0038096).” Apoptosis is a programmed cell death that is one of the possible consequences of host-pathogen interaction in mycobacterial infections (Behar et al., 2011; Mohareer et al., 2018). Apoptosis is a potential defense mechanism against intracellular pathogens. There is growing evidence that apoptosis of infected macrophages can limit the proliferation and growth of intracellular mycobacteria and subsequently reduce mycobacterial viability (Allen et al., 2001; Benítez-Guzmán et al., 2018; Abdalla et al., 2020). Several previous studies have shown that M. tuberculosis infection in humans (Keane et al., 1997; PLACIDO et al., 1997) and murine (Rojas et al., 1998) and M. bovis infection in cattle (Gutiérrez-Pabello et al., 2002; Vega-Manriquez et al., 2007) induce apoptosis in macrophages. Additionally, it has been highlighted that the reduction of M. bovis growth in bovine macrophages has a positive and significant correlation with the induction of apoptosis in infected macrophages (Denis et al., 2007). Therefore, it has been suggested that the induction of apoptosis is closely linked to the emergence of macrophage resistance to M. bovis replication (Denis et al., 2005). On the other hand, apoptosis may act as a double-edged sword, so uncontrolled apoptosis of macrophages and T cells during infection may play an important role in the formation of tuberculous lesions (Fayyazi et al., 2000; Cassidy, 2006).
During M. bovis infection, various types of cell death may be induced, among which apoptosis and autophagy restricts bacterial growth and facilitates host defense mechanisms, while ferroptosis and necroptosis are beneficial for pathogen growth and transmission (Chai et al., 2020). Ferroptosis is a new type of iron-dependent programmed necrotic cell death caused by intracellular iron accumulation and lipid peroxidation, leading to oxidative stress and cell death (Chen et al., 2021b). Significantly, infections with MTBC agents such as M. bovis and M. tuberculosis induce the appearance of necrotic lesions (Cassidy, 2006; Harper et al., 2011; Roy et al., 2019). Indeed, it has been hypothesized that ferroptosis plays an essential role in the pathogenesis of MTBC infectious agents through (1) iron accumulation which is an essential component for successful infection of various infectious bacilli causing TB, and (2) induction of necrosis (Meunier and Neyrolles, 2019). Consistent with our results, these findings suggest the importance of ferroptosis during infection with infectious agents of MTBC, which could be a promising target for the control and treatment of M. bovis and M. tuberculosis infections.
Bacterial pathogens use a variety of strategies to manipulate host cell function to their advantage, thereby evading the host’s immune responses and prolonging infection (Nougayrède et al., 2005). One of these immunomodulatory mechanisms for escaping the immune responses is to induce the host cell cycle arrest. In this regard, an in-depth transcriptomic effort showed that M. tuberculosis could arrest the cell cycle of macrophages in mice, potentially modulating the host immune response and enhancing long-term persistence (Cumming et al., 2017). Therefore, treatment strategies based on interference with pathogen-host cell cycle interactions can be effective approaches for chemotherapeutic intervention to prevent long-term infection of intracellular bacilli (Cumming et al., 2017).
The Wnt signaling pathway is an important ancient molecular cascade that plays a key role in many developmental processes and the maintenance of adult tissue homeostasis by interfering with processes such as regulating cell proliferation, migration, preservation of adult stem cells, differentiation, apoptosis, the immune response (Blumenthal et al., 2006; Schaale et al., 2011), and genetic stability (Kahn, 2014; Duan and Bonewald, 2016). Additionally, it has recently been reported that dysregulation in the Wnt signaling are linked to the pathogenesis of lung diseases, especially lung cancer, pulmonary fibrosis, and pulmonary arterial hypertension (Königshoff and Eickelberg, 2010).
Interestingly, several hub-central genes of the blue module, such as PSMB3, PSMA3, PSMA4, PSMB4 (Seto et al., 2020), PSMA5 (Widdison et al., 2011), PSMD6, PSMB6, PSMD8 (Zhao et al., 2022), PSME2 (Maji et al., 2015), PSME1 (Bell et al., 2017), and PSMC4 (Shi et al., 2021) involved in the proteasome pathway, played important roles in the pathogenesis of M. bovis and M. tuberculosis. For instance, the PSMB3, PSMA3, PSMA4, and PSMB4 hub-central genes are associated with mycobacterial granulomatous lesions (Seto et al., 2020). Moreover, integrated bioinformatic research identified the PSMC4 hub-central gene as one of the important biomarkers for tuberculous pleurisy (Shi et al., 2021). Among the other hub-central genes in the blue module, we also identified two genes, including COPS5 (Meenu et al., 2016; Sambarey et al., 2017) and UBQLN1 (Sakowski et al., 2015; Franco et al., 2017), that were associated with the host immune responses, leading to restriction of mycobacterial growth/replication and clearance of intracellular M. bovis, respectively.
Functional enrichment analysis revealed that the brown module was significantly enriched in several immune/pathogenic-related pathways such as “JAK–STAT signaling pathway,” “PI3K-Akt signaling pathway,” “negative regulation of programmed cell death (GO:0043069),” and “negative regulation of apoptotic process (GO:0043066).” The Janus kinase/signal transducers and activators of transcription (JAK–STAT) pathway is one of a handful of pleiotropic cascades that is the major signal transducer for a wide range of cytokines and growth factors (Vainchenker and Constantinescu, 2013). The JAK–STAT signaling pathway begins with the extracellular binding of cytokines as well as IFNs to their respective receptors, which leads to receptor oligomerization and then accelerates JAKs trans-activation. Following the activation of JAKs, the cytoplasmic tails of the receptors are phosphorylated, which puts JAKs and STATs in spatial proximity. Then, JAKs mediate tyrosine-phosphorylation (p-Tyr) of STATs, which results in STAT dimerization, nuclear translocation, DNA binding and, finally, regulation of gene transcription (Villarino et al., 2017; Xin et al., 2020). Pathogenic mycobacteria can interfere with the JAK–STAT signaling pathway and attenuate the cytokine-induce immune response. Previous studies have discovered one of the immunosuppression and survival strategies of pathogenic mycobacteria such as M. bovis (Imai et al., 2003; Fang et al., 2020), M. tuberculosis (Manca et al., 2005), and Mycobacterium avium subsp. paratuberculosis (MAP; Arsenault et al., 2014) in macrophages is the blockade of the JAK–STAT signaling pathway by inducing this pathway inhibitor’s expression.
It is well known that the phosphatidylinositol 3-kinase (PI3K)/protein kinase B (AKT) signaling pathway plays a vital role in cell growth, metabolism, differentiation, apoptosis, and autophagy (Yu and Cui, 2016; Zhang et al., 2017). As discussed in the blue module, apoptosis of macrophages infected with intracellular bacilli such as M. bovis and M. tuberculosis actively destroys infected host cells and their contents, including intracellular mycobacteria, thereby limiting mycobacterial growth and proliferation (Behar et al., 2011; Nalpas et al., 2015). In contrast, research has shown that one of the major mechanisms of escaping the host immune response and increasing mycobacterial survival is macrophage apoptosis subversion by M. bovis and M. tuberculosis (Keane et al., 2000; Behar et al., 2011; Abdalla et al., 2020; Fang et al., 2020). Interestingly, activation of the PI3K-Akt signaling pathway during mycobacterial infection directly modulates the apoptosis of host cells (Gong et al., 2020). Additionally, Hussain et al. (2019a) observed that M. bovis disrupted autophagosome assembly by activating the PI3K-Akt signaling pathway, thereby modulating autophagy and thus preventing intracellular pathogen degradation (Hussain et al., 2019a). Furthermore, it has recently been revealed that infectious agents of MTBC, through some of its proteins, inhibits the production of proinflammatory cytokines and reduces antigen-presenting cell (APC) function in mouse macrophages via the activation of PI3K-Akt signaling pathway (Liu et al., 2016). Nevertheless, these results suggest that the PI3K-Akt signaling pathway plays important roles in the pathogenesis of M. bovis and other MTBC infectious agents and could be considered in future research as a promising target for bTB control. Moreover, in agreement with the biological performance of the brown module, other immune/pathogenic-related processes of the brown module including “Cytokine-cytokine receptor interaction,” “cytokine-mediated signaling pathway (GO:0019221),” and “Focal adhesion” have been observed in similar network-based TB studies (Lin et al., 2019; Li L. et al., 2020; Alam et al., 2022; Liang et al., 2022).
Additionally, in terms of the hub-central genes identified in the brown module, several hub-central genes such as SRC (Chandra et al., 2016), ITGB3 (Chen et al., 2021a), BCL2L1 (Sharma et al., 2016), CDKN1A (Silva et al., 2021), MDM2 (Shariq et al., 2021), and MMP1 (Villarreal-Ramos et al., 2018) have been reported as key factors in the immunomodulatory mechanisms of MTBC agents such as M. bovis. It is well known that phagocytosis is an effective immune response process in killing intracellular mycobacteria, while mycobacteria prevent phagocytosis by host cells to maintain survival within macrophages (Fang et al., 2020). Interestingly, research has reported that M. tuberculosis infection leads to upregulation of the SRC hub-central gene, which directly inhibits phagosome-lysosome fusion and plays an effective role in maintaining mycobacterial survival within macrophages (Lechartier et al., 2014). In this regard, it has been highlighted that inhibition of SRC promotes phagosome acidification and xenophagy flux in macrophages, and SRC inhibitors have a substantial potential for developing anti-TB drugs (Chandra et al., 2016). Moreover, another study showed that, unlike the SRC hub-central gene, upregulation of the ITGB3 hub-central gene could overcome the inhibition of phagosome maturation due to mycobacterial infection, and activation of ITGB3 could facilitate M. tuberculosis clearance in vivo (Chen et al., 2021a). The BCL2L1 hub-central gene is an important anti-apoptotic factor that has shown a significant upregulation in response to M. bovis and M. tuberculosis infections, and it has been suggested that this hub-central gene plays a central role in the pathogenic mechanisms of infectious bacilli by inhibiting apoptosis (Xaus et al., 1999; Nalpas et al., 2015; Silva et al., 2021). In addition to BCL2L1, the CDKN1A hub-central gene encodes the p21 protein, a member of the Cip/Kip family, whose high levels are associated with pulmonary sarcoidosis and, as an inhibitor of apoptosis, facilitate the formation and maturation of TB granulomas (Xaus et al., 1999, 2003; Silva et al., 2021). Furthermore, the M. tuberculosis RipA (a peptidoglycan hydrolase) suppresses the caspase-mediated apoptosis pathway by activation the MDM2 hub-central gene and continues its survival in the infected host (Shariq et al., 2021). Interestingly, the results of previous studies suggest that the MDM2 hub-central gene showed higher levels of expression in response to infection with virulent strains of M. bovis (AF2122/97) than attenuated strains (G18) and with greater inhibition of apoptosis in macrophages infected with AF2122/97 played a crucial role in the development of bTB (Jensen et al., 2018). The MMP1 is another hub-central gene whose gene products are key to collagen degradation and alveolar destruction (Salgame, 2011). Indeed, it has been reported that M. tuberculosis, as well as M. bovis, selectively upregulated MMP1 gene expression, which leads to tissue destruction in TB and immunopathology of the lungs (Elkington et al., 2011; Parasa et al., 2017; Villarreal-Ramos et al., 2018).
Other hub-central gene of the brown module, including CSF1 (Chatterjee et al., 2021), PLAUR (McLoughlin et al., 2021b), ITGB1 (Yang et al., 2017), CCND1 (Koo et al., 2012; Looney et al., 2021), CSF2 (Marsay et al., 2013; Shukla et al., 2017; Abdelaal et al., 2022), CTLA4 (Zhang et al., 2021), FLNA (Xu et al., 2015), CCND2 (Lavalett et al., 2017), WEE1 (Jayaswal et al., 2010), MMP9 (Blanco et al., 2012; McLoughlin et al., 2014), CDC25A (Shapira et al., 2020), CSF2RB (Benmerzoug et al., 2018), TIMP1 (Sun et al., 2020), CD69 (Li et al., 2011; Chen et al., 2020), PTPN22 (Boechat et al., 2013), CD38 (Silveira-Mattos et al., 2019), IL7R (Jenum et al., 2016; Alsulaimany et al., 2022), IL1RN (Alcaraz-López et al., 2020), and IDO1 (Weiner et al., 2012; Gautam et al., 2018) were also involved in host-pathogen interactions as well as suppression of host immune response. For example, the CTLA4 hub-central gene encodes an inhibitor of T cell-mediated response (Schneider et al., 2006), and the upregulation of this gene in response to M. bovis infection may reflect a mechanism of immunomodulation used by M. bovis to subvert a host T-cell response (Killick et al., 2011). The WEE1 hub-central gene plays an important role in combating the progression of infection and intracellular survival of M. tuberculosis. It has been reported that knocking down the WEE1 gene leads to a significant increase in Mycobacterium levels in host macrophages (Jayaswal et al., 2010). It has also been reported that the MMP9 and TIMP1 hub-central genes were highly correlated with TB development (Klepp et al., 2019), and have been suggested as valuable diagnostic biomarkers for TB (Xu et al., 2015; Sun et al., 2020) and bTB (Blanco et al., 2012). Moreover, the IL1RN hub-central gene has been suggested as a promising candidate biomarker for natural resistance to bTB in Holstein-Friesian cattle (Alcaraz-López et al., 2020).
In the brown module, we also identified STAT1 hub-central TF, a pivotal component of the JAK–STAT signaling pathway and a signal transducer and transcription activator that mediates cellular responses to IFNs, cytokines, and growth factors (Hall et al., 2020). Interestingly, M. bovis counteracts the immune response by suppressing STAT1 expression and exacerbates its pathogenesis in the host cells (Chen J. et al., 2021). Studies in patients with active TB have shown that STAT1 activation was impaired in host macrophages (Esquivel-Solís et al., 2009). Additionally, it has been proved that M. tuberculosis EspB protein suppresses IFN-γ-induced autophagy in murine macrophages by inhibiting IFN-γ-activated STAT1 phosphorylation (Huang and Bao, 2016). Most importantly, unphosphorylated STAT1 inhibits apoptosis in M. tuberculosis-infected macrophages (Yao et al., 2017). Surprisingly, STAT1 leads to the expression of inducible nitric oxide (NO) synthase and subsequently releases NO at sufficient concentrations for mycobactericidal. Thus, it can be concluded that M. bovis inhibits the mycobactericidal mechanism of NO by inhibiting STAT1 phosphorylation (Sharma et al., 2007). Therefore, these findings demonstrate the importance of STAT1 hub-central TF in the host immune response during mycobacterial infection, which could be a key target for counteracting M. bovis immunosuppressive strategies and developing a treatment for bTB in the future.
Functional terms such as “T cell receptor signaling pathway,” “regulation of T cell activation (GO:0050863),” “Th17 cell differentiation,” “Th1 and Th2 cell differentiation,” “Natural killer cell mediated cytotoxicity,” “gamma-delta T cell activation (GO:0046629),” “positive regulation of interferon-gamma production (GO:0032729),” and “B cell receptor signaling pathway (GO:0050853)” showed that the green module is closely related to the cell-mediated and humoral immunity. There is considerable evidence from various in vitro and in vivo studies that indicate the central role of T-cell subtypes (γδ, CD4 and CD8 T-cells) in host defense against mycobacterial pathogens, including M. bovis (POLLOCK et al., 1996; Cassidy et al., 2001), as demonstrated in the absence of T-cells, TB susceptibility increases (Mogues et al., 2001; Moguche et al., 2017). Furthermore, progressive impairment of the M. tuberculosis-specific T-cell responses with increasing mycobacterial load and subsequent recovery of responses during the treatment period indicates an inverse relationship between T-cell activation and disease severity of TB (Day et al., 2011). The production of IFN-γ by CD4 T-cells to activate the bactericidal mechanisms of infected macrophages is an essential process for host defense against bTB and TB (Flynn et al., 1993; Vordermeier et al., 2002; Gallegos et al., 2011; Cooper and Torrado, 2012). It has also been reported that γδ T-cells may significantly limit M. bovis infection by producing IFN-γ (Kennedy et al., 2002). On the other hand, it has been observed that cytotoxic T-cells inhibit the growth of intracellular mycobacteria by special lysis of M. bovis-infected macrophages (Skinner et al., 2003). In particular, the design of new vaccines and vaccination strategies based on CD8 T-cell responses has been proposed (Kaufmann et al., 1999). According to previous research, in agreement with the adaptive immune response of the green module, following the initiation of a cell-mediated immune response, the initiation of humoral immunity specially B-cell-dependent signals, such as “B cell receptor signaling pathway” during M. bovis infection, may be involved in the mycobactericidal response in bTB (Pollock et al., 2006; Aranday-Cortes et al., 2012).
Interestingly, most of the hub-central genes in the green module, including CD3E (Mair et al., 2021), ZAP70 (Samten et al., 2009), CD4 (Boggiatto et al., 2021), IL2RA (Lu et al., 2011), CD247, LCK, CD3D, CD3G, PRKCQ (McLoughlin et al., 2021a), and ITK (Huang et al., 2020), were closely related to T-cell activation and the host immune response to infection with MTBC intracellular pathogens. The CD3E hub-central gene is an essential core for T-cell activation (Mair et al., 2021) and plays a crucial role in the immune response against TB (Gebremicael et al., 2019). Based on the results of previous research, an intense decrease in the expression of CD3E in patients with active TB and then an increase in the expression of this gene during the treatment period exhibited that this gene has a negative correlation with the progression of mycobacterial infection (Jenum et al., 2016; Gebremicael et al., 2018). In addition to CD3E, the ZAP70 and LCK hub-central genes are key components of T-cell activation and signaling, and there is growing evidence that intracellular mycobacteria such as M. bovis and M. tuberculosis interfere with the function of host T-cells by downregulating the phosphorylation of these genes (Mahon et al., 2012; Sande et al., 2016). ITK is a tyrosine kinase that regulates T-cells development and function. Indeed, ITK deficiency and alternation in T-cell receptor/ITK signaling impairs early protection against M. tuberculosis in human lungs (Huang et al., 2020). Therefore, enhancing of ITK signaling has been introduced as an alternative strategy to target infection with highly virulent strains of M. tuberculosis (Huang et al., 2020). Remarkably, several hub-central genes of the green module, including ITK, CD2, CD247, ZAP70, CD3D, and CD3E, were identified as potential therapeutic targets for pulmonary TB by a computational drug-ability effort (Alsulaimany et al., 2022).
The results of the functional enrichment analysis suggested that co-regulated genes of the pink module were highly enriched in the host innate immune response and inflammatory mechanisms such as “Toll-like receptor signaling pathway,” “C-type lectin receptor signaling pathway,” “NOD-like receptor signaling pathway,” “RIG-I-like receptor signaling pathway,” “Cytosolic DNA-sensing pathway,” “IL-17 signaling pathway,” “NF-kappa B signaling pathway,” “MAPK signaling pathway,” “negative regulation of type I interferon production (GO:0032480)” and “Necroptosis.” Pathogen-associated molecular pattern molecules (PAMPs) are essential components derived from microorganisms that are critical to the survival and function of microorganisms (Akira et al., 2006; Tang et al., 2012). Indeed, recognition of mycobacterial PAMPs by PRRs of innate immune cells, such as macrophages, activates a cascade of downstream signaling, which ultimately leads to the activation of the nuclear factor kappa B (NF-κB) and mitogen-activated protein kinase (MAPK) signaling pathways (Trinchieri and Sher, 2007; Gong et al., 2020). Finally, activation of the NF-κB and MAPK downstream signaling pathways leads to the host inflammatory response through the production of proinflammatory cytokines and chemokines such as IL6, IL1B, TNF, IL18, and IL8, which in addition to inducing an innate immune response, regulate subsequent adaptive immune response (Means et al., 2000; Mahla et al., 2013; Thakur et al., 2018). However, various reports suggest that M. tuberculosis and M. bovis modulates proinflammatory cytokine production via the NF-κB and MAPK signaling inhibition in favor of their survival and thus suppresses the innate immune response (Pathak et al., 2007; Wang et al., 2015; Liu et al., 2016; Ha et al., 2020; Lu et al., 2020). On the other hand, activation of NF-κB and MAPK signaling pathways can also play an important role in TB immunopathology (Bai et al., 2013). Moreover, overactivity of the NF-κB and IL-17 signaling pathways in response to mycobacterial infection leads to the induction of pyroptosis which is a highly inflammatory form of lytic programmed cell death, thereby facilitating the spread of mycobacteria to neighboring cells (Beckwith et al., 2020) as well as severe TB sepsis (Li L.-L. et al., 2020). This finding indicates the importance of the inflammatory pathways as key targets for inducing different immunosuppressive strategies by MTBC pathogens.
Overall, PRRs are divided into two main categories: (1) membrane-bound PRRs including Toll-like receptors (TLRs) and C-type lectin receptors (CLRs); and (2) cytoplasmic PRRs including NOD-like receptors (NLRs) and RIG-I-like receptors (RLRs; Killick et al., 2013). Several previous transcriptomic studies have highlighted that M. bovis infection induces the toll-like receptor signaling pathway in bovine macrophages (Lin et al., 2015; Ma et al., 2016; Shukla et al., 2018). Nevertheless, in addition to the important role that TLRs play in initiating an innate immune response and enhancing adaptive immunity, mycobacterial activation of TLR signaling may act as an escape mechanism from host defense (Netea et al., 2004). Therefore, it has been recommended that modulation of TLR signaling could affect ability of invading mycobacteria such as M. bovis to destroy and escape the host response (Krutzik and Modlin, 2004).
Other factors, including NLRs and RLRs, interact with the activation of inflammatory responses and thus help induce an innate immune response (Zitvogel et al., 2012). The NLR family is a group of cytoplasmic receptors involved in inflammation and immunity by interfering with the secretion of several cytokines and playing a considerable role in mycobacterial-host interactions (Pahari et al., 2017). Based on the results of time series studies, activation of the NOD-like receptor signaling pathway in the early stages of infection with virulent strains of M. bovis in bovine macrophages indicates a key role of these pathways to induce a robust macrophage response to infection with mycobacterial pathogens (Jensen et al., 2018). Additionally, several studies have highlighted the central role of NLRs in various aspects of the host immunity, including resistance to M. tuberculosis infection (Divangahi et al., 2008) and restriction of intracellular M. tuberculosis growth by inducing autophagy in infected human alveolar macrophages (Juárez et al., 2012), optimal production of proinflammatory cytokines (Brooks et al., 2011), and enhancing NO production (Landes et al., 2015). RLRs are a group of RNA helicases that play an important role in the detection of viral RNA in the host cytoplasm (Killick et al., 2013). Generally, RLR signaling is involved in the production of type I IFNs and antiviral proteins by activating downstream transcription factors (Loo and Gale, 2011). It has been made clear that the expression of several genes commonly associated with the detection of viral PAMPs, such as viral RNA, is manipulated during the M. bovis challenge in vitro, suggesting that the RIG-I-like receptor signaling pathway may be involved in the mycobacterial infections (Magee et al., 2012). Furthermore, based on the functional biological process term “negative regulation of type I interferon production (GO:0032480)” of the pink module, we hypothesized that M. bovis may modulate the production of type I IFNs. This hypothesis is supported by previous transcriptomic studies that reported that M. bovis and M. tuberculosis actively reduced the production of type I IFNs in bovine, human, and murine macrophages and dendritic cells to increase their survival and immune evasion (Simmons et al., 2010; Nalpas et al., 2015; Banks et al., 2019).
As mentioned, mycobacterial pathogens such as M. bovis and M. tuberculosis kills infected macrophages by inhibiting apoptosis and autophagy and promoting necrosis. However, the induction of necrosis is associated with the formation of granuloma, which is the hallmark of TB infection (Butler et al., 2012). Necroptosis is a prototype of programmed necrosis death, also known as inflammatory programmed cell death, and is considered as the link between cell death and inflammation (Mohareer et al., 2018). In other words, necroptosis exacerbates the host inflammatory response to infection and therefore contributes significantly to tissue damage (Tripathi et al., 2018). Besides, previous reports have shown that intracellular M. tuberculosis induces necroptosis in myeloid lineage cells such as monocytes and macrophages, leading to (1) exacerbation of necrosis and (2) impaired trained immunity, thereby facilitates mycobacterial escape and dissemination (Khan et al., 2020). Interestingly, a recent study suggested that inhibition of necroptosis may improve the health status of TB patients and enhance antibacterial TB chemotherapy (Pajuelo et al., 2020).
Several inflammation-related genes, including TLR2 (Meade et al., 2007), NLRP3 (Malone et al., 2018), CCL4 (Widdison et al., 2008), IL1A, IL1B, TNF (Salgame, 2005), and IL6 (Magee et al., 2012) had double centrality in both co-expression network and co-expressed hub gene-based PPI network of the pink module and played a central role in the interactions between bAMs and M. bovis. One of the essential mechanisms of host defense against intracellular pathogens is innate immunity, which is highly dependent on the behavior of inflammatory molecules. Thus, proinflammatory cytokines such as TNF, IL6, IL1A, and IL1B are core component of the host’s innate immune response against invading M. bovis (Salgame, 2005). Increased expression of TNF, IL6, IL1B, and IL1A hub-central genes in bovine monocyte-derived macrophages (bMDMs) following in vitro stimulation with M. bovis in a 48 h time series indicates their important role in the early-stage of infection (Wang et al., 2011; Magee et al., 2014; Sabio y García et al., 2020). Conversely, suppression TNF and IL6 gene expression to counteract the host immune response is a key feature of late-stage of M. bovis infection (MacHugh et al., 2009). Interestingly, various reports have demonstrated that the use of TNF antagonists and inhibitors increases (1) TB susceptibility, (2) reactivation of M. bovis and M. tuberculosis, (3) and risk of TB mortality in humans and cattle (Ehlers, 2003; Nager et al., 2009; Xie et al., 2014; Arbués et al., 2020). Moreover, blocking or inactivating the TNF hub-central gene leads to M. bovis escaping from the TNF-induced apoptosis of host macrophages (Piercy et al., 2007). These findings indicate a key role of TNF hub-central gene in preventing TB or bTB reactivation and limiting the pathogenic response of the host.
Another study showed that in addition to TNF, polymorphisms of another cytokine genes such as IL1B and IL6 were associated with latent TB infection and pulmonary TB (Wu et al., 2018, 2019). Besides, the use of an additional readout system, such as IL1B, has been suggested to increase the sensitivity of IFN-γ release assay (IGRA) test for the detection of M. bovis infection in cattle (Jones et al., 2010). Additionally, TNF and IL1A hub-central genes have been identified as promising biomarkers for the development of bTB diagnosis strategies (Sánchez-Soto et al., 2017; Palmer et al., 2020). TLR2 hub-central gene is a major component of the TLR family and plays an important role in recognizing mycobacterial PAMPs and activating the innate immune response (Nalpas et al., 2015). TLR2 signaling acts as a potential defense system against M. bovis infection because the host innate immune response to MTBC infectious agents is mainly mediated by TLR2 in macrophages and leads to the activation of macrophages in the early stage of infection (Krutzik and Modlin, 2004). Moreover, activation of TLR2 also induces apoptosis as a direct bactericidal effect in infected macrophages and suppresses the proliferation of intracellular mycobacteria (Gerold et al., 2007). Interestingly, as a survival strategy and subversion mechanism of the host immune response, M. tuberculosis suppresses TLR2 through several of its components, such as LprE lipoprotein and PPE51 protein, and subsequently inhibits TLR2-dependent autophagy and cathelicidin (Padhi et al., 2019; Strong et al., 2022). The use of TLR2 agonists has also been highlighted as an effective tool for optimizing vaccination strategies to protect cows against bTB (Wedlock et al., 2008).
However, in addition to the key role that these inflammation-related hub-central genes play in the host-pathogen interactions, they can act as a double-edged sword and play an effective immunopathological role during M. tuberculosis and M. bovis infection. For instance, overexpression of the IL6 in M. tuberculosis or M. bovis-infected macrophages can inhibit the macrophage response to IFN-γ (Nagabhushanam et al., 2003) and suppress the T-cell response (Magee et al., 2012). Significantly, pathogenic mycobacteria can interfere with host defense mechanisms through TLRs. As mentioned earlier, long-term stimulation of TLR2 in M. tuberculosis-infected macrophages suppresses the IFN-γ response (Gehring et al., 2003) and inhibits antigen presentation in infected macrophages (Noss et al., 2001). NLRP3 inflammasome is an important member of the intracellular NLR family, and previous bioinformatics research has reported a rapid increase in the expression of this gene in response to virulent strains of M. bovis infection in macrophages (Zhou et al., 2016). Indeed, NLRP3 inflammasome activates CASP1, leading to the release of IL1B, which in turn leads to pyroptosis in M. tuberculosis-infected macrophages, resulting in severe ultrastructural disruptions and spread of the pathogen in the host cells (Beckwith et al., 2020; Kanipe and Palmer, 2020). Additionally, NLRP3 activation is directly related to necrotic death triggered by M. tuberculosis (Wong and Jacobs, 2011). CCL4 is a proinflammatory and chemotactic beta chemokine that has been shown to play an important role in the respiratory syncytial virus (RSV), bovine immune deficiency virus, and M. bovis infections (Widdison and Coffey, 2011). Previous reports indicate an increase in the CCL4 expression levels in response to M. bovis (Nalpas et al., 2013), and growing evidence suggests a direct positive correlation between CCL4 plasma levels and bTB-induced lung lesions (Widdison et al., 2009).
Other hub-central genes of the pink module were included CCL8 (Rusk et al., 2017), CCL20 (Malone et al., 2018), CXCL3 (Zhang et al., 2019), DUSP1 (Abo-Kadoum et al., 2021), EDN1 (Lin et al., 2015), ICAM1 (Li P. et al., 2017), IER3 (Widdison et al., 2011), ISG15 (Kimmey et al., 2017), MAP3K8 (Naeem et al., 2021), NFKBIA (Tsai et al., 2009), NFKBIZ (Dong et al., 2022), PTGS2 (Xiong et al., 2018), PTX3 (Wang et al., 2013), RSAD2 (Andreu et al., 2017), TBK1 (Wang J. et al., 2018), MAPK8 (Gautam et al., 2014), BIRC3 (MacHugh et al., 2012), TNFAIP3 (Hall et al., 2020), TNFAIP6 (Lin et al., 2015), and VEDFA (Ndlovu and Marakalala, 2016), and the intracellular pathogen of MTBC such as M. bovis, could induce various strategies to escape the host immune response by activating or suppressing these genes. According to the literature reports on models of M. tuberculosis and M. bovis infection, some of these molecular mechanisms that contribute to the TB pathogenesis include the following:
1. Overexpression of CCL20 in response to M. tuberculosis infection reduces ROS production and subsequently inhibits ROS-dependent apoptosis (Rivero-Lezcano et al., 2010).
2. A 700-fold increase in expression of the EDN1 hub-central gene has been reported in M. bovis-infected cows. The EDN1 gene encodes the ET-1 protein, which leads to increased pulmonary hypertension, delayed T-cell response, and inhibition of the migration of antigen-presenting cells (Lin et al., 2015).
3. Mycobacterium tuberculosis inhibits P53-dependent apoptosis by activating PTGS2 (Dutta et al., 2012).
4. The ICAM1, PTGS2, CCL20, and IL6 hub-central genes showed a close relationship with the development of pulmonary TB and had the potential to use biomarkers for TB (Sun et al., 2020).
5. A recent study using in vitro and ex vivo approaches discovered that miR-199a expression increased significantly in response to M. bovis infection. Subsequently, miR-199a suppresses cellular autophagy, apoptosis and modulates the production of type I IFNs by directly targeting the TBK1 hub-central gene (a major regulator of autophagy), thereby accelerating intracellular growth and survival of M. bovis (Wang J. et al., 2018).
6. Mycobacterium bovis and M. tuberculosis inhibit host cell apoptosis by increasing expression in anti-apoptotic factors such as BIRC3 (Killick et al., 2014) and decreasing expression in pro-apoptotic factors such as MAPK8 (Gautam et al., 2014). Moreover, interventional methods to activate MAPK8 have been proposed as a potential therapeutic strategy to increase apoptosis of infected cells and destruction of intracellular mycobacteria (Alam et al., 2021).
7. Increased expression of VEGFA in patients with active TB leads to the development of TB granuloma associated angiogenesis (Ndlovu and Marakalala, 2016).
8. The TNFAIP3 hub-central gene is a central regulator of immunopathology because it is a key player in the negative feedback regulation of the NF-κB signaling pathway (Vereecke et al., 2009), and increased of TNFAIP3 expression levels in M. bovis-infected animals modulates the host immune response and decreases proinflammatory cytokines (especially TNF) by inhibition NF-κB signaling, thereby leads to progression of M. bovis infection (Kumar et al., 2015).
Several hub-central TFs, including ATF3 (Chen Y. et al., 2021), FOSB (Green et al., 2010), HIF1A (Li F. et al., 2020), and IRF1 (Pathak et al., 2007) were also identified that played a key immunoregulatory role in the biological behavior of the pink module. For example, HIF1A hub-central TF is a master transcriptional regulator and an important factors in regulating gene expression in response to hypoxia (Cimmino et al., 2019). HIF1A TF plays a key role in combating M. bovis infection, as previous studies have shown that interfering HIF1A with siRNA defected the capacity of phagocytosis, ROS generation, and glucose metabolism (Li F. et al., 2020). On the other hand, HIF1A is also effective in host-directed anti-TB immunometabolism processes (Shi et al., 2015). IRF1 hub-central TF is the first member of the interferon-regulatory transcription factor (IRF) family to be initially introduced as an IFN-beta (a type I IFN) transcription activator (Yarilina et al., 2008). In this regard, as mentioned earlier, M. tuberculosis through some of its proteins, inhibits the activation of IFN-associated TFs, such as IRF1, and modulates the production of type I IFNs (Pathak et al., 2007).
In addition to the pink module, we identified several critical functional terms related to inflammation and immune response, such as “NF-kappa B signaling pathway,” “TNF signaling pathway,” and “B cell receptor signaling pathway” in the tan module, as well as terms such as “cytokine-mediated signaling pathway (GO:0019221),” “negative regulation of programmed cell death (GO:0043069),” “toll-like receptor 2 signaling pathway (GO:0034134),” and “negative regulation of epithelial cell apoptotic process (GO:1904036)” in the salmon module. Moreover, several hub-central genes/TFs of the tan module such as CD40 (Khan et al., 2016), CD274 (Petrilli et al., 2020), CTNNB1 (Subuddhi et al., 2020), IKBKE (Killick et al., 2014), IL23R (Jiang et al., 2015), MAPK7 (María Irene et al., 2021), TRAF2 (Killick et al., 2014), NFKB1 (Meade et al., 2007), NFKB2 (Magee et al., 2012), and STAT6 (Cronan et al., 2021), as well as hub-central genes of the salmon module, such as CASP4 (Malone et al., 2018), DHX58 (Nalpas et al., 2015), IL10 (Wang et al., 2011), MX1, MX2, IFIT2 (Yi et al., 2021), RIPK2 (Widdison et al., 2011), TRAF1 (Li H. et al., 2017), and USP18 (Carranza et al., 2020), have been reported to be involved in the host immunity and M. bovis pathogenesis. The NFKB1 hub-central TF is a major mediator of the proinflammatory immune response that stimulates the transcription of proinflammatory cytokines and chemokines and has shown a significant reduction in the response to M. bovis infection (Meade et al., 2007). Interestingly, several previous studies have highlighted that a decrease in NFKB1 expression in response to M. tuberculosis and M. bovis infection is directly related to suppression of the host innate immune signaling as well as prevention of phagosome maturation in the chronic stages of bTB and TB (MacHugh et al., 2009; Alam et al., 2019). Furthermore, IL10 is an anti-inflammatory cytokine that has been upregulated in response to M. bovis infected bovine macrophages (Wang et al., 2011). Indeed, several previous researches suggests that M. bovis, as well as M. tuberculosis induces various immunomodulatory mechanisms, including inhibition of phagosome-lysosome fusion and, thus prevention of phagosome maturation (O'Leary et al., 2011), suppression of the production of IFN-γ, NO, and proinflammatory cytokines such as TNF, IL6 and IL1B (Jensen et al., 2019), in infected macrophages by upregulating IL10 expression levels (Sheridan et al., 2017). Therefore, direct gene repression of IL10 during M. tuberculosis infection has been proposed as a novel solution to improve macrophage bactericidal functions and M. tuberculosis clearance (Chandra et al., 2013).
Significant functional terms such as “Fatty acid degradation,” “fatty acid catabolic process (GO:0009062),” “fatty acid beta-oxidation (GO:0006635),” “regulation of lipid metabolic process (GO:0019216),” “fatty acid oxidation (GO:0019395),” “cholesterol metabolic process (GO:0008203),” “secondary alcohol biosynthetic process (GO:1902653),” “fatty acid beta-oxidation using acyl-CoA oxidase (GO:0033540),” “regulation of cholesterol biosynthetic process (GO:0045540),” “cellular amino acid catabolic process (GO:0009063),” “Tryptophan metabolism,” “Valine, leucine and isoleucine degradation,” and “Glycine, serine and threonine metabolism” in the turquoise module have supported the hypothesis that host metabolic processes are reprogrammed by intracellular mycobacteria such as M. bovis as well as M. tuberculosis (Lee et al., 2013).
During mycobacterial infections, especially the virulent strains of M. tuberculosis and M. bovis, extraction and utilization of host nutrients, especially fatty acids and cholesterol (preferably) for the survival and viability of mycobacteria is essential for all pathogenic activities by these pathogens (Lee et al., 2013). Several studies using M. tuberculosis infection models have been reported that this pathogen has a unique ability to assimilate and utilize host-derived lipids, especially fatty acids and cholesterol, which catabolized as important carbon sources to fuel central metabolic pathways to facilitate the mycobacterial growth and persistence (Cole et al., 1998; Russell et al., 2009; Wilburn et al., 2018). In addition to carbon sources, mycobacterial pathogens can provide the required nitrogen sources through the metabolism of amino acids in the host (Gouzy et al., 2014). For example, host serine (Ser) biosynthesis is one of the most important processes to provide the nitrogen sources needed for M. tuberculosis survival (Borah et al., 2019). Additionally, tryptophan (Trp) metabolism plays a vital role in the growth and activation of MTBC infectious agents (Qualls and Murray, 2016), so there is growing evidence that during M. tuberculosis infection, activated macrophages try to limit growth of intracellular pathogen through Trp starvation. However, in return, M. tuberculosis induces Trp biosynthesis in the host to counteract this auxotroph threat (Wang X. et al., 2021).
Important hub-central genes of the turquoise module were included ACAA1 (Behera et al., 2022), ACAD10 (Nalpas et al., 2015), ACSS2 (Koo et al., 2012), ALDH2 (Park et al., 2014), ALDH9A1 (Aiyaz et al., 2014), C1QA, C1QB, CIQC (Cai et al., 2014), C3AR1 (Zhang et al., 2019), ECHS1 (Bell et al., 2017), EHHADH (Aiyaz et al., 2014), FCGRA1 (Jenum et al., 2016), LAPTM5 (Kang et al., 2011), PCCA, PCCB (Katiyar et al., 2018), PEPD (White et al., 2010), TREM2 (Iizasa et al., 2021), VPS11, VPS18 (Chandra et al., 2015), and VPS33B (Mascarello et al., 2010), which were involved in the host immune response and bAMs-M. bovis interactions. For instance, ACAA1 is one of the core component of fatty acid metabolic process which encodes a hallmark enzyme of fatty acid β-oxidation, and it has been reported that M. tuberculosis increases the rate of fatty acid β-oxidation for its survival by enhancing the expression of this gene (Behera et al., 2022). Besides, ALDH2 has a protective effect on TB by interfering with alcohol metabolism (Park et al., 2014). C1q is a 460 kDa protein consisting of 18 polypeptide chains (6A, 6B, and 6C) whose main function is to initiate complement activation. It has been observed that high levels of C1q subtype proteins such as C1QA, C1QB, and C1QC are strongly associated with active TB and disease severity (Lubbers et al., 2018). In addition, C1QC has been introduced as a potential biomarker for active TB diagnosis (Cai et al., 2014). Moreover, the PEPD hub-central gene is essential in facilitating mycobacterial adaptation (White et al., 2010) and is directly associated with cavity formation in patients with pulmonary TB (Wang et al., 2014). VPS33B is a subset of the class C vacuolar protein sorting complex (Vps-C) that acts as the core of membrane fusion and protein sorting (HOPS) and regulates membrane trafficking throughout the endocytic pathway (Wong et al., 2013). Intriguingly, M. tuberculosis protein tyrosine phosphatase A (PtpA) dephosphorylates and inactivates VPS33B, thereby shutting down the membrane fusion machinery in the host macrophages (Wong et al., 2011). As a result, inactivation of VPS33B directly blocks phagosome-lysosome fusion and prevents phagosome acidification (Bach et al., 2008; Chen, 2015).
We also identified autophagy related pathways such as “Autophagy,” “regulation of macroautophagy (GO:0016241),” and “regulation of autophagy (GO:0010506)” in the turquoise module. During the autophagy, cytoplasmic packages, including damaged organelles, misfolded proteins, and intracellular pathogens, are enclosed in a double-membrane vesicle called autophagosome and after fusion with a lysosome (autophagosome maturation), an autolysosome is formed which decomposes its contents (Hasankhani et al., 2021a). Numerous studies have reported that autophagy is a direct mechanism for killing intracellular M. tuberculosis and M. bovis, and protecting the host against TB (Ní Cheallaigh et al., 2011; Castillo et al., 2012; Songane et al., 2012; Hussain et al., 2019b). Conversely, as discussed, intracellular tubercle bacilli escape autophagy using specific immunosuppressive strategies. In this regard, we identified several hub-central genes, including CTSS, VPS11, VPS18, and VPS33B in the turquoise module, that were potential targets for M. bovis to modulate host autophagy. The CTSS hub-central gene encodes the proteolytic enzyme cathepsin S, which acts primarily on lysosomes (González-Ruiz et al., 2019). Surprisingly, research has shown that pathogens such as M. tuberculosis and M. bovis prevent lysosome-autophagosome fusion (autophagosome maturation; Pawar et al., 2016) and lysosome-phagosome fusion (phagosome maturation; Pires et al., 2017) by suppressing CTSS gene expression, and prevent autophagy and phagocytosis, respectively. In addition to VPS33B, VPS11 and VPS18 are key mediators for autophagosome-lysosome fusion, and their dephosphorylation during M. tuberculosis infection prevents autophagosome maturation (Rohde et al., 2007; Chandra et al., 2015). Therefore, developing anti-TB therapies based on autophagy targeting can be a key strategy for controlling the intracellular growth and proliferation of pathogenic mycobacteria (Paik et al., 2019).
In conclusion, in the current study, we use a systems biology approach for a deep investigation of the interactions of bAMs and M. bovis in order to better understand the molecular regulatory mechanisms underlying bTB and to identify novel insights into immunomodulatory mechanisms inducted by intracellular M. bovis for maintaining mycobacterial survival and replication. Combining RNA-seq data and WGCNA module preservation analysis with functional enrichment analysis resulted in the identification of 7 bTB-specific modules in reference samples whose (1) topological properties, such as connectivity patterns and network density, were altered under M. bovis-infected conditions, and (2) they were directly biologically related to the bAMs-M. bovis interactions such as host immune response, M. bovis immune subversion mechanisms, and bTB development. Moreover, the integration of co-expression gene networks based on hub genes of the bTB-specific modules with PPI networks led to the identification of 260 genes that had double centrality in their respective networks (co-expression modules and downstream co-expressed hub genes-based PPI networks). Additionally, our results provided evidence that these hub-central genes played a key role in the fate of M. bovis infection and maybe act as the core of several immunosuppressive mechanisms of the M. bovis, such as prevention of macrophage phagosome-lysosome fusion, induction of necrosis, inhibition of apoptosis and autophagy, suppression of antigen presentation, modulation of type I IFNs, modulation of IFN-γ production and signaling, modulation of macrophage signaling mechanisms, manipulation of host macrophage metabolism, recruitment of cell surface receptors on the host macrophage, cytosolic escape from the phagosome, and inhibition of ROS production, to escape the host immune response. Notwithstanding this, further research is needed to deep explore the key role of hub-central genes reported in this study to develop novel and more effective therapeutic and diagnostic approaches to control or eradication bTB.
Data availability statement
The original contributions presented in the study are included in the article/Supplementary material, further inquiries can be directed to the corresponding authors.
Author contributions
AH and AB conceived the ideas. AH, FS and AB designed the study. AH, AB, HA, and NS analyzed the data. AH, AB, and FS administrated the project. AH, SM, ShM and AB interpreted the data. HA, AH, SaM, ShM, FS and AB validated the data. AH, FS and AB wrote the main manuscript. FR, GJ, and HK helped in writing the manuscript. ShM, HB, MD, AH, and AB reviewed and edited the manuscript. All authors read and approved the final version of manuscript.
Acknowledgments
The authors thank all the teams for their comprehensive cooperation in implementation of this research and who provided technical assistance in the laboratory during this study. Furthermore, all dear participants who cooperated in this study are appreciated. We also thank the reviewers whose critical comments helped in improving the manuscript.
Conflict of interest
The authors declare that the research was conducted in the absence of any commercial or financial relationships that could be construed as a potential conflict of interest.
Publisher’s note
All claims expressed in this article are solely those of the authors and do not necessarily represent those of their affiliated organizations, or those of the publisher, the editors and the reviewers. Any product that may be evaluated in this article, or claim that may be made by its manufacturer, is not guaranteed or endorsed by the publisher.
Supplementary material
The Supplementary material for this article can be found online at: https://www.frontiersin.org/articles/10.3389/fmicb.2022.1041314/full#supplementary-material
Abbreviations
bAMs, Bovine alveolar macrophages; bTB, Bovine tuberculosis; CPM, Count per million; DEGs, Differentially expressed genes; GEO, Gene expression omnibus; GO, Gene ontology; hpi, Hours post infection; IFN, Interferon; KEGG, Kyoto encyclopedia of genes and genomes; MCC, Maximal clique centrality; MM, Module memberships; MTBC, Mycobacterium tuberculosis complex; NCBI, National center for biotechnology information; NO, Nitric oxide; PAMPs, Pathogen associated molecular patterns; PPI, Protein–protein interaction; PRRs, Pattern recognition receptors; RNA-seq, RNA sequencing; ROS, Reactive oxygen species; STRING, Search tool for the retrieval of interacting genes; TB, Tuberculosis; TFs, Transcription factors; TOM, Topological overlap matrix; WGCNA, Weighted gene co-expression network analysis.
Footnotes
1. ^https://www.bioinformatics.babraham.ac.uk/projects/fastqc/
2. ^https://maayanlab.cloud/Enrichr/
References
Abdalla, A. E., Ejaz, H., Mahjoob, M. O., Alameen, A. A. M., Abosalif, K. O. A., Elamir, M. Y. M., et al. (2020). Intelligent mechanisms of macrophage apoptosis subversion by mycobacterium. Pathogens 9:218. doi: 10.3390/pathogens9030218
Abdelaal, H. F. M., Thacker, T. C., Wadie, B., Palmer, M. V., Talaat, A. M., and Ehrt, S. (2022). Transcriptional profiling of early and late phases of bovine tuberculosis. Infect. Immun. 90:e0031321. doi: 10.1128/iai.00313-21
Abo-Kadoum, M. A., Assad, M., Ali, M. K., Uae, M., Nzaou, S. A. E., Gong, Z., et al. (2021). Mycobacterium tuberculosis PE17 (Rv1646) promotes host cell apoptosis via host chromatin remodeling mediated by reduced H3K9me3 occupancy. Microb. Pathog. 159:105147. doi: 10.1016/j.micpath.2021.105147
Aiyaz, M., Bipin, C., Pantulwar, V., Mugasimangalam, R., Shanley, C. A., Ordway, D. J., et al. (2014). Whole genome response in Guinea pigs infected with the high virulence strain Mycobacterium tuberculosis TT372. Tuberculosis 94, 606–615. doi: 10.1016/j.tube.2014.10.001
Akira, S., Uematsu, S., and Takeuchi, O. (2006). Pathogen recognition and innate immunity. Cells 124, 783–801. doi: 10.1016/j.cell.2006.02.015
Alam, A., Abubaker Bagabir, H., Sultan, A., Siddiqui, M. F., Imam, N., Alkhanani, M. F., et al. (2022). An integrative network approach to identify common genes for the therapeutics in tuberculosis and its overlapping non-communicable diseases. Front. Pharmacol. 12:770762. doi: 10.3389/fphar.2021.770762
Alam, A., Imam, N., Ahmed, M. M., Tazyeen, S., Tamkeen, N., Farooqui, A., et al. (2019). Identification and classification of differentially expressed genes and network meta-analysis reveals potential molecular signatures associated with tuberculosis. Front. Genet. 10:932. doi: 10.3389/fgene.2019.00932
Alam, A., Imam, N., Siddiqui, M. F., Ali, M. K., Ahmed, M. M., and Ishrat, R. (2021). Human gene expression profiling identifies key therapeutic targets in tuberculosis infection: A systematic network meta-analysis. Infect. Genet. Evol. 87:104649. doi: 10.1016/j.meegid.2020.104649
Alcaraz-López, O. A., Villarreal-Morales, Y., Rangel-Escareño, C., and Gutiérrez-Pabello, J. A. (2020). Assessment of candidate biomarkers to detect resistance to Mycobacterium bovis in Holstein-Friesian cattle. Res. Vet. Sci. 132, 416–425. doi: 10.1016/j.rvsc.2020.07.016
Allen, S., Sotos, J., Sylte, M. J., and Czuprynski, C. J. (2001). Use of hoechst 33342 staining to detect apoptotic changes in bovine mononuclear phagocytes infected with Mycobacterium avium subsp. paratuberculosis. Clin. Diagn. Lab. Immunol. 8, 460–464. doi: 10.1128/CDLI.8.2.460-464.2001
Alm, E., and Arkin, A. P. (2003). Biological networks. Curr. Opin. Struct. Biol. 13, 193–202. doi: 10.1016/S0959-440X(03)00031-9
Alsulaimany, F. A., Zabermawi, N. M. O., Almukadi, H., Parambath, S. V., Shetty, P. J., Vaidyanathan, V., et al. (2022). Transcriptome-based molecular networks uncovered interplay between druggable genes of CD8+ T cells and changes in immune cell landscape in patients with pulmonary tuberculosis. Front. Med. 8:812857. doi: 10.3389/fmed.2021.812857
Anders, S., Pyl, P. T., and Huber, W. (2014). HTSeq—a python framework to work with high-throughput sequencing data. Bioinformatics 31, 166–169. doi: 10.1093/bioinformatics/btu638
Andreu, N., Phelan, J., de Sessions, P. F., Cliff, J. M., Clark, T. G., and Hibberd, M. L. (2017). Primary macrophages and J774 cells respond differently to infection with Mycobacterium tuberculosis. Sci. Rep. 7:42225. doi: 10.1038/srep42225
Aranday-Cortes, E., Hogarth, P. J., Kaveh, D. A., Whelan, A. O., Villarreal-Ramos, B., Lalvani, A., et al. (2012). Transcriptional profiling of disease-induced host responses in bovine tuberculosis and the identification of potential diagnostic biomarkers. PLoS One 7:e30626. doi: 10.1371/journal.pone.0030626
Arbués, A., Brees, D., Chibout, S.-D., Fox, T., Kammüller, M., and Portevin, D. (2020). TNF-α antagonists differentially induce TGF-β1-dependent resuscitation of dormant-like Mycobacterium tuberculosis. PLoS Pathog. 16:e1008312. doi: 10.1371/journal.ppat.1008312
Arsenault, R. J., Maattanen, P., Daigle, J., Potter, A., Griebel, P., and Napper, S. (2014). From mouth to macrophage: mechanisms of innate immune subversion by Mycobacterium avium subsp. paratuberculosis. Vet. Res. 45:54. doi: 10.1186/1297-9716-45-54
Bach, H., Papavinasasundaram, K. G., Wong, D., Hmama, Z., and Av-Gay, Y. (2008). Mycobacterium tuberculosis virulence is mediated by PtpA dephosphorylation of human vacuolar protein sorting 33B. Cell Host Microbe 3, 316–322. doi: 10.1016/j.chom.2008.03.008
Bai, X., Feldman, N. E., Chmura, K., Ovrutsky, A. R., Su, W.-L., Griffin, L., et al. (2013). Inhibition of nuclear factor-kappa B activation decreases survival of Mycobacterium tuberculosis in human macrophages. PLoS One 8:e61925. doi: 10.1371/journal.pone.0061925
Bai, Q., Liu, H., Guo, H., Lin, H., Song, X., Jin, Y., et al. (2020). Identification of hub genes associated with development and microenvironment of hepatocellular carcinoma by weighted gene co-expression network analysis and differential gene expression analysis. Front. Genet. 11:615308. doi: 10.3389/fgene.2020.615308
Bakhtiarizadeh, M. R., Hosseinpour, B., Shahhoseini, M., Korte, A., and Gifani, P. (2018). Weighted gene co-expression network analysis of endometriosis and identification of functional modules associated with its main hallmarks. Front. Genet. 9:453. doi: 10.3389/fgene.2018.00453
Bakhtiarizadeh, M. R., Mirzaei, S., Norouzi, M., Sheybani, N., and Vafaei Sadi, M. S. (2020). Identification of gene modules and hub genes involved in mastitis development using a systems biology approach. Front. Genet. 11:722. doi: 10.3389/fgene.2020.00722
Banks, D. A., Ahlbrand, S. E., Hughitt, V. K., Shah, S., Mayer-Barber, K. D., Vogel, S. N., et al. (2019). Mycobacterium tuberculosis inhibits autocrine type I IFN signaling to increase intracellular survival. J. Immunol. 202, 2348–2359. doi: 10.4049/jimmunol.1801303
Barabási, A.-L., Gulbahce, N., and Loscalzo, J. (2011). Network medicine: a network-based approach to human disease. Nat. Rev. Genet. 12, 56–68. doi: 10.1038/nrg2918
Beckwith, K. S., Beckwith, M. S., Ullmann, S., Sætra, R. S., Kim, H., Marstad, A., et al. (2020). Plasma membrane damage causes NLRP3 activation and pyroptosis during Mycobacterium tuberculosis infection. Nat. Commun. 11:2270. doi: 10.1038/s41467-020-16143-6
Behar, S. M., Martin, C. J., Booty, M. G., Nishimura, T., Zhao, X., Gan, H. X., et al. (2011). Apoptosis is an innate defense function of macrophages against Mycobacterium tuberculosis. Mucosal Immunol. 4, 279–287. doi: 10.1038/mi.2011.3
Behera, A., Jain, P., Ganguli, G., Biswas, M., Padhi, A., Pattanaik, K. P., et al. (2022). Mycobacterium tuberculosis acetyltransferase suppresses oxidative stress by inducing peroxisome formation in macrophages. Int. J. Mol. Sci. 23:2584. doi: 10.3390/ijms23052584
Bell, L., Peyper, J. M., Garnett, S., Tadokera, R., Wilkinson, R., Meintjes, G., et al. (2017). TB-IRIS: proteomic analysis of in vitro PBMC responses to Mycobacterium tuberculosis and response modulation by dexamethasone. Exp. Mol. Pathol. 102, 237–246. doi: 10.1016/j.yexmp.2017.02.008
Benítez-Guzmán, A., Arriaga-Pizano, L., Morán, J., and Gutiérrez-Pabello, J. A. (2018). Endonuclease G takes part in AIF-mediated caspase-independent apoptosis in Mycobacterium bovis-infected bovine macrophages. Vet. Res. 49:69. doi: 10.1186/s13567-018-0567-1
Benmerzoug, S., Marinho, F. V., Rose, S., Mackowiak, C., Gosset, D., Sedda, D., et al. (2018). GM-CSF targeted immunomodulation affects host response to M. tuberculosis infection. Sci. Rep. 8:8652. doi: 10.1038/s41598-018-26984-3
Bi, D., Ning, H., Liu, S., Que, X., and Ding, K. (2015). Gene expression patterns combined with network analysis identify hub genes associated with bladder cancer. Comput. Biol. Chem. 56, 71–83. doi: 10.1016/j.compbiolchem.2015.04.001
Blanco, F. C., Soria, M., Bianco, M. V., and Bigi, F. (2012). Transcriptional response of peripheral blood mononuclear cells from cattle infected with Mycobacterium bovis. PLoS One 7:e41066. doi: 10.1371/journal.pone.0041066
Blumenthal, A., Ehlers, S., Lauber, J. R., Buer, J., Lange, C., Goldmann, T., et al. (2006). The wingless homolog WNT5A and its receptor Frizzled-5 regulate inflammatory responses of human mononuclear cells induced by microbial stimulation. Blood 108, 965–973. doi: 10.1182/blood-2005-12-5046
Boechat, A. L., Ogusku, M. M., Sadahiro, A., and dos Santos, M. C. (2013). Association between the PTPN22 1858C/T gene polymorphism and tuberculosis resistance. Infect. Genet. Evol. 16, 310–313. doi: 10.1016/j.meegid.2013.02.019
Boggiatto, P. M., Kanipe, C. R., and Palmer, M. V. (2021). Enhanced detection of Mycobacterium bovis-specific T cells in experimentally-infected cattle. Front. Vet. Sci. 8:676710. doi: 10.3389/fvets.2021.676710
Bolger, A. M., Lohse, M., and Usadel, B. (2014). Trimmomatic: a flexible trimmer for Illumina sequence data. Bioinformatics 30, 2114–2120. doi: 10.1093/bioinformatics/btu170
Borah, K., Beyß, M., Theorell, A., Wu, H., Basu, P., Mendum, T. A., et al. (2019). Intracellular Mycobacterium tuberculosis exploits multiple host nitrogen sources during growth in human macrophages. Cell Rep. 29, 3580–3591.e4. doi: 10.1016/j.celrep.2019.11.037
Brooks, M. N., Rajaram, M. V. S., Azad, A. K., Amer, A. O., Valdivia-Arenas, M. A., Park, J.-H., et al. (2011). NOD2 controls the nature of the inflammatory response and subsequent fate of Mycobacterium tuberculosis and M. bovis BCG in human macrophages. Cell. Microbiol. 13, 402–418. doi: 10.1111/j.1462-5822.2010.01544.x
Brosch, R., Gordon, S. V., Marmiesse, M., Brodin, P., Buchrieser, C., Eiglmeier, K., et al. (2002). A new evolutionary scenario for the Mycobacterium tuberculosis complex. Proc. Natl. Acad. Sci. 99, 3684–3689. doi: 10.1073/pnas.052548299
Buddle, B. M., Vordermeier, H. M., Hewinson, R. G. Jr., W, R., McShane, H., Mizrahi, V., et al. (2016). Experimental infection models of tuberculosis in domestic livestock. Microbiol. Spectrum 4:4.4.66. doi: 10.1128/microbiolspec.TBTB2-0017-2016
Butler, R. E., Brodin, P., Jang, J., Jang, M.-S., Robertson, B. D., Gicquel, B., et al. (2012). The balance of apoptotic and necrotic cell death in Mycobacterium tuberculosis infected macrophages is not dependent on bacterial virulence. PLoS One 7:e47573. doi: 10.1371/journal.pone.0047573
Cai, Y., Yang, Q., Tang, Y., Zhang, M., Liu, H., Zhang, G., et al. (2014). Increased complement C1q level marks active disease in human tuberculosis. PLoS One 9:e92340. doi: 10.1371/journal.pone.0092340
Carranza, C., Pedraza-Sanchez, S., de Oyarzabal-Mendez, E., and Torres, M. (2020). Diagnosis for latent tuberculosis infection: new alternatives. Front. Immunol. 11:2006. doi: 10.3389/fimmu.2020.02006
Cassidy, J. P. (2006). The pathogenesis and pathology of bovine tuberculosis with insights from studies of tuberculosis in humans and laboratory animal models. Vet. Microbiol. 112, 151–161. doi: 10.1016/j.vetmic.2005.11.031
Cassidy, J. P., Bryson, D. G., Gutiérrez Cancela, M. M., Forster, F., Pollock, J. M., and Neill, S. D. (2001). Lymphocyte subtypes in experimentally induced early-stage bovine tuberculous lesions. J. Comp. Pathol. 124, 46–51. doi: 10.1053/jcpa.2000.0427
Castillo, E. F., Dekonenko, A., Arko-Mensah, J., Mandell, M. A., Dupont, N., Jiang, S., et al. (2012). Autophagy protects against active tuberculosis by suppressing bacterial burden and inflammation. Proc. Natl. Acad. Sci. 109, E3168–E3176. doi: 10.1073/pnas.1210500109
Chai, Q., Wang, L., Liu, C. H., and Ge, B. (2020). New insights into the evasion of host innate immunity by Mycobacterium tuberculosis. Cell. Mol. Immunol. 17, 901–913. doi: 10.1038/s41423-020-0502-z
Chandra, V., Bhagyaraj, E., Nanduri, R., Ahuja, N., and Gupta, P. (2015). NR1D1 ameliorates Mycobacterium tuberculosis clearance through regulation of autophagy. Autophagy 11, 1987–1997. doi: 10.1080/15548627.2015.1091140
Chandra, V., Mahajan, S., Saini, A., Dkhar, H. K., Nanduri, R., Raj, E. B., et al. (2013). Human IL10 gene repression by rev-erbα ameliorates Mycobacterium tuberculosis clearance. J. Biol. Chem. 288, 10692–10702. doi: 10.1074/jbc.M113.455915
Chandra, P., Rajmani, R. S., Verma, G., Bhavesh, N. S., Kumar, D., and Stallings, C. L. (2016). Targeting drug-sensitive and -resistant strains of Mycobacterium tuberculosis by inhibition of SRC family kinases lowers disease burden and pathology. mSphere 1, e00043–e00015. doi: 10.1128/mSphere.00043-15
Chatterjee, S., Yabaji, S. M., Rukhlenko, O. S., Bhattacharya, B., Waligurski, E., Vallavoju, N., et al. (2021). Channeling macrophage polarization by rocaglates increases macrophage resistance to Mycobacterium tuberculosis. iScience 24:102845. doi: 10.1016/j.isci.2021.102845
Chen, Z. (2015). Mycobacterium tuberculosis favors its survival by utilizing host ubiquitin to impair innate immunity. Natl. Sci. Rev. 2, 260–261. doi: 10.1093/nsr/nwv034
Chen, X., Cao, X., Lei, Y., Reheman, A., Zhou, W., Yang, B., et al. (2021a). Distinct persistence fate of Mycobacterium tuberculosis in various types of cells. mSystems 6, e00783–e00721. doi: 10.1128/mSystems.00783-21
Chen, X., Kang, R., Kroemer, G., and Tang, D. (2021b). Ferroptosis in infection, inflammation, and immunity. J. Exp. Med. 218:e20210518. doi: 10.1084/jem.20210518
Chen, J., Liu, C., Liang, T., Xu, G., Zhang, Z., Lu, Z., et al. (2021). Comprehensive analyses of potential key genes in active tuberculosis: A systematic review. Medicine 100:e26582. doi: 10.1097/md.0000000000026582
Chen, E. Y., Tan, C. M., Kou, Y., Duan, Q., Wang, Z., Meirelles, G. V., et al. (2013). Enrichr: interactive and collaborative HTML5 gene list enrichment analysis tool. BMC Bioinf. 14:128. doi: 10.1186/1471-2105-14-128
Chen, Z.-Y., Wang, L., Gu, L., Qu, R., Lowrie, D. B., Hu, Z., et al. (2020). Decreased expression of CD69 on T cells in tuberculosis infection resisters. Front. Microbiol. 11:1901. doi: 10.3389/fmicb.2020.01901
Chen, Y., Wang, Q., Lin, S., Lai, J., Lin, J., Ao, W., et al. (2021). Meta-analysis of peripheral blood transcriptome datasets reveals a biomarker panel for tuberculosis in patients infected with HIV. Front. Cell. Infect. Microbiol. 11:585919. doi: 10.3389/fcimb.2021.585919
Chin, C.-H., Chen, S.-H., Wu, H.-H., Ho, C.-W., Ko, M.-T., and Lin, C.-Y. (2014). cytoHubba: identifying hub objects and sub-networks from complex interactome. BMC Syst. Biol. 8:S11. doi: 10.1186/1752-0509-8-S4-S11
Cimmino, F., Avitabile, M., Lasorsa, V. A., Montella, A., Pezone, L., Cantalupo, S., et al. (2019). HIF-1 transcription activity: HIF1A driven response in normoxia and in hypoxia. BMC Med. Genet. 20:37. doi: 10.1186/s12881-019-0767-1
Cline, M. S., Smoot, M., Cerami, E., Kuchinsky, A., Landys, N., Workman, C., et al. (2007). Integration of biological networks and gene expression data using Cytoscape. Nat. Protoc. 2, 2366–2382. doi: 10.1038/nprot.2007.324
Cole, S. T., Brosch, R., Parkhill, J., Garnier, T., Churcher, C., Harris, D., et al. (1998). Deciphering the biology of Mycobacterium tuberculosis from the complete genome sequence. Nature 393, 537–544. doi: 10.1038/31159
Cooper, A. M., and Torrado, E. (2012). Protection versus pathology in tuberculosis: recent insights. Curr. Opin. Immunol. 24, 431–437. doi: 10.1016/j.coi.2012.04.008
Cronan, M. R., Hughes, E. J., Brewer, W. J., Viswanathan, G., Hunt, E. G., Singh, B., et al. (2021). A non-canonical type 2 immune response coordinates tuberculous granuloma formation and epithelialization. Cells 184, 1757–1774.e14. doi: 10.1016/j.cell.2021.02.046
Cumming, B. M., Rahman, M. A., Lamprecht, D. A., Rohde, K. H., Saini, V., Adamson, J. H., et al. (2017). Mycobacterium tuberculosis arrests host cycle at the G1/S transition to establish long term infection. PLoS Pathog. 13:e1006389. doi: 10.1371/journal.ppat.1006389
Das, S., Meher, P. K., Rai, A., Bhar, L. M., and Mandal, B. N. (2017). Statistical approaches for gene selection, hub gene identification and module interaction in gene co-expression network analysis: an application to aluminum stress in soybean (Glycine max L.). PLoS One 12:e0169605. doi: 10.1371/journal.pone.0169605
Day, C. L., Abrahams, D. A., Lerumo, L., Janse van Rensburg, E., Stone, L., O’rie, T., et al. (2011). Functional capacity of Mycobacterium tuberculosis-specific T cell responses in humans is associated with mycobacterial load. J. Immunol. 187, 2222–2232. doi: 10.4049/jimmunol.1101122
Denis, M., Keen, D. L., Parlane, N. A., Storset, A. K., and Buddle, B. M. (2007). Bovine natural killer cells restrict the replication of Mycobacterium bovis in bovine macrophages and enhance IL-12 release by infected macrophages. Tuberculosis 87, 53–62. doi: 10.1016/j.tube.2006.03.005
Denis, M., Wedlock, D. N., and Buddle, B. M. (2005). IFN-γ enhances bovine macrophage responsiveness to Mycobacterium bovis: impact on bacterial replication, cytokine release and macrophage apoptosis. Immunol. Cell Biol. 83, 643–650. doi: 10.1111/j.1440-1711.2005.01386.x
Divangahi, M., Mostowy, S., Coulombe, F., Kozak, R., Guillot, L., Veyrier, F., et al. (2008). NOD2-deficient mice have impaired resistance to Mycobacterium tuberculosis infection through defective innate and adaptive immunity. J. Immunol. 181, 7157–7165. doi: 10.4049/jimmunol.181.10.7157
Djelouadji, Z., Raoult, D., and Drancourt, M. (2011). Palaeogenomics of Mycobacterium tuberculosis: epidemic bursts with a degrading genome. Lancet Infect. Dis. 11, 641–650. doi: 10.1016/S1473-3099(11)70093-7
Dong, W., Wang, G., Feng, J., Li, P., Wang, R., Lu, H., et al. (2022). MiR-25 blunts autophagy and promotes the survival of Mycobacterium tuberculosis by regulating NPC1. iScience 25:104279. doi: 10.1016/j.isci.2022.104279
Duan, P., and Bonewald, L. F. (2016). The role of the wnt/β-catenin signaling pathway in formation and maintenance of bone and teeth. Int. J. Biochem. Cell Biol. 77, 23–29. doi: 10.1016/j.biocel.2016.05.015
Dutta, N. K., Mehra, S., Martinez, A. N., Alvarez, X., Renner, N. A., Morici, L. A., et al. (2012). The stress-response factor SigH modulates the interaction between Mycobacterium tuberculosis and host phagocytes. PLoS One 7:e28958. doi: 10.1371/journal.pone.0028958
Ehlers, S. (2003). Role of tumour necrosis factor (TNF) in host defence against tuberculosis: implications for immunotherapies targeting TNF. Ann. Rheum. Dis. 62:37ii. doi: 10.1136/ard.62.suppl_2.ii37
Eissing, T., Kuepfer, L., Becker, C., Block, M., Coboeken, K., Gaub, T., et al. (2011). A computational systems biology software platform for multiscale modeling and simulation: integrating whole-body physiology, disease biology, and molecular reaction networks. Front. Physiol. 2:4. doi: 10.3389/fphys.2011.00004
Elkington, P., Shiomi, T., Breen, R., Nuttall, R. K., Ugarte-Gil, C. A., Walker, N. F., et al. (2011). MMP-1 drives immunopathology in human tuberculosis and transgenic mice. J. Clin. Invest. 121, 1827–1833. doi: 10.1172/JCI45666
Esquivel-Solís, H., Quiñones-Falconi, F., Zarain-Herzberg, A., Amieva-Fernández, R. I., and López-Vidal, Y. (2009). Impaired activation of Stat1 and c-Jun as a possible defect in macrophages of patients with active tuberculosis. Clin. Exp. Immunol. 158, 45–54. doi: 10.1111/j.1365-2249.2009.03985.x
Fang, L., Lin, W., Jia, H., Gao, X., Sui, X., Guo, X., et al. (2020). Potential diagnostic value of the peripheral blood mononuclear cell transcriptome from cattle with bovine tuberculosis. Front. Vet. Sci. 7:295. doi: 10.3389/fvets.2020.00295
Fayyazi, A., Eichmeyer, B., Soruri, A., Schweyer, S., Herms, J., Schwarz, P., et al. (2000). Apoptosis of macrophages and T cells in tuberculosis associated caseous necrosis. J. Pathol. 191, 417–425. doi: 10.1002/1096-9896(2000)9999:9999<::AID-PATH664>3.0.CO;2-R
Flynn, J. L., Chan, J., Triebold, K. J., Dalton, D. K., Stewart, T. A., and Bloom, B. R. (1993). An essential role for interferon gamma in resistance to Mycobacterium tuberculosis infection. J. Exp. Med. 178, 2249–2254. doi: 10.1084/jem.178.6.2249
Franco, L. H., Nair, V. R., Scharn, C. R., Xavier, R. J., Torrealba, J. R., Shiloh, M. U., et al. (2017). The ubiquitin ligase smurf1 functions in selective autophagy of Mycobacterium tuberculosis and anti-tuberculous host defense. Cell Host Microbe 21, 59–72. doi: 10.1016/j.chom.2016.11.002
Franco, E. F., Rana, P., Queiroz Cavalcante, A. L., da Silva, A. L., Gomide A, C. P., Carneiro Folador, A. R., et al. (2020). Co-expression networks for causal gene identification based on RNA-seq data of Corynebacterium pseudotuberculosis. Genes 11:794. doi: 10.3390/genes11070794
Gallegos, A. M., van Heijst, J. W. J., Samstein, M., Su, X., Pamer, E. G., and Glickman, M. S. (2011). A gamma interferon independent mechanism of CD4 T cell mediated control of M. tuberculosis infection in vivo. PLoS Pathog. 7:e1002052. doi: 10.1371/journal.ppat.1002052
Garnier, T., Eiglmeier, K., Camus, J.-C., Medina, N., Mansoor, H., Pryor, M., et al. (2003). The complete genome sequence of Mycobacterium bovis. Proc. Natl. Acad. Sci. 100, 7877–7882. doi: 10.1073/pnas.1130426100
Gautam, U. S., Foreman, T. W., Bucsan, A. N., Veatch, A. V., Alvarez, X., Adekambi, T., et al. (2018). In vivo inhibition of tryptophan catabolism reorganizes the tuberculoma and augments immune-mediated control of Mycobacterium tuberculosis. Proc. Natl. Acad. Sci. 115, E62–E71. doi: 10.1073/pnas.1711373114
Gautam, U. S., Mehra, S., Ahsan, M. H., Alvarez, X., Niu, T., and Kaushal, D. (2014). Role of TNF in the altered interaction of dormant Mycobacterium tuberculosis with host macrophages. PLoS One 9:e95220. doi: 10.1371/journal.pone.0095220
Gebremicael, G., Kassa, D., Alemayehu, Y., Gebreegziaxier, A., Kassahun, Y., van Baarle, D., et al. (2019). Gene expression profiles classifying clinical stages of tuberculosis and monitoring treatment responses in Ethiopian HIV-negative and HIV-positive cohorts. PLoS One 14:e0226137. doi: 10.1371/journal.pone.0226137
Gebremicael, G., Kassa, D., Quinten, E., Alemayehu, Y., Gebreegziaxier, A., Belay, Y., et al. (2018). Host gene expression kinetics during treatment of tuberculosis in HIV-coinfected individuals is independent of highly active antiretroviral therapy. J. Infect. Dis. 218, 1833–1846. doi: 10.1093/infdis/jiy404
Gehring, A. J., Rojas, R. E., Canaday, D. H., Lakey, D. L., Harding, C. V., and Boom, W. H. (2003). The Mycobacterium tuberculosis 19-kilodalton lipoprotein inhibits gamma interferon-regulated HLA-DR and FcγR1 on human macrophages through toll-like receptor 2. Infect. Immun. 71, 4487–4497. doi: 10.1128/IAI.71.8.4487-4497.2003
Gerold, G., Zychlinsky, A., and de Diego, J. L. (2007). What is the role of toll-like receptors in bacterial infections? Semin. Immunol. 19, 41–47. doi: 10.1016/j.smim.2006.12.003
Gong, W.-P., Liang, Y., Ling, Y.-B., Zhang, J.-X., Yang, Y.-R., Wang, L., et al. (2020). Effects of mycobacterium vaccae vaccine in a mouse model of tuberculosis: protective action and differentially expressed genes. Mil. Med. Res. 7:25. doi: 10.1186/s40779-020-00258-4
González-Ruiz, S., Strillacci, M. G., Durán-Aguilar, M., Cantó-Alarcón, G. J., Herrera-Rodríguez, S. E., Bagnato, A., et al. (2019). Genome-wide association study in mexican Holstein cattle reveals novel quantitative trait loci regions and confirms mapped loci for resistance to bovine tuberculosis. Animals 9:636. doi: 10.3390/ani9090636
Gouzy, A., Poquet, Y., and Neyrolles, O. (2014). Amino acid capture and utilization within the Mycobacterium tuberculosis phagosome. Future Microbiol. 9, 631–637. doi: 10.2217/fmb.14.28
Green, J. A., Elkington, P. T., Pennington, C. J., Roncaroli, F., Dholakia, S., Moores, R. C., et al. (2010). Mycobacterium tuberculosis upregulates microglial matrix metalloproteinase-1 and -3 expression and secretion via NF-κB– and activator protein-1–dependent monocyte networks. J. Immunol. 184, 6492–6503. doi: 10.4049/jimmunol.0903811
Gutiérrez-Pabello, J. A., McMurray, D. N., and Adams, L. G. (2002). Upregulation of thymosin β-10 by Mycobacterium bovis infection of bovine macrophages is associated with apoptosis. Infect. Immun. 70, 2121–2127. doi: 10.1128/IAI.70.4.2121-2127.2002
Ha, S.-H., Choi, H., Park, J.-Y., Abekura, F., Lee, Y.-C., Kim, J.-R., et al. (2020). Mycobacterium tuberculosis–secreted protein, ESAT-6, inhibits lipopolysaccharide-induced MMP-9 expression and inflammation through NF-κB and MAPK signaling in raw 264.7 macrophage cells. Inflammation 43, 54–65. doi: 10.1007/s10753-019-01087-x
Hall, T. J., Mullen, M. P., McHugo, G. P., Killick, K. E., Ring, S. C., Berry, D. P., et al. (2021). Integrative genomics of the mammalian alveolar macrophage response to intracellular mycobacteria. BMC Genomics 22:343. doi: 10.1186/s12864-021-07643-w
Hall, T. J., Vernimmen, D., Browne, J. A., Mullen, M. P., Gordon, S. V., MacHugh, D. E., et al. (2020). Alveolar macrophage chromatin is modified to orchestrate host response to Mycobacterium bovis infection. Front. Genet. 10:1386. doi: 10.3389/fgene.2019.01386
Han, H. (2019). Identification of several key genes by microarray data analysis of bovine mammary gland epithelial cells challenged with Escherichia coli and Staphylococcus aureus. Gene 683, 123–132. doi: 10.1016/j.gene.2018.10.004
Harper, J., Skerry, C., Davis, S. L., Tasneen, R., Weir, M., Kramnik, I., et al. (2011). Mouse model of necrotic tuberculosis granulomas develops hypoxic lesions. J. Infect. Dis. 205, 595–602. doi: 10.1093/infdis/jir786
Hasankhani, A., Bahrami, A., Sheybani, N., Aria, B., Hemati, B., Fatehi, F., et al. (2021a). Differential co-expression network analysis reveals key hub-high traffic genes as potential therapeutic targets for COVID-19 pandemic. Front. Immunol. 12:789317. doi: 10.3389/fimmu.2021.789317
Hasankhani, A., Bahrami, A., Sheybani, N., Fatehi, F., Abadeh, R., Ghaem Maghami Farahani, H., et al. (2021b). Integrated network analysis to identify key modules and potential hub genes involved in bovine respiratory disease: A systems biology approach. Front. Genet. 12:753839. doi: 10.3389/fgene.2021.753839
Heidari, M., Pakdel, A., Bakhtiarizadeh, M. R., and Dehghanian, F. (2021). Integrated analysis of lncRNAs, mRNAs, and TFs to identify regulatory networks underlying MAP infection in cattle. Front. Genet. 12:668448. doi: 10.3389/fgene.2021.668448
Hood, L., Heath, J. R., Phelps, M. E., and Lin, B. (2004). Systems biology and new technologies enable predictive and preventative medicine. Science 306, 640–643. doi: 10.1126/science.1104635
Hu, H., Miao, Y.-R., Jia, L.-H., Yu, Q.-Y., Zhang, Q., and Guo, A.-Y. (2018). AnimalTFDB 3.0: a comprehensive resource for annotation and prediction of animal transcription factors. Nucleic Acids Res. 47, D33–D38. doi: 10.1093/nar/gky822
Huang, D., and Bao, L. (2016). Mycobacterium tuberculosis EspB protein suppresses interferon-γ-induced autophagy in murine macrophages. J. Microbiol. Immunol. Infect. 49, 859–865. doi: 10.1016/j.jmii.2014.11.008
Huang, L., Ye, K., McGee, M. C., Nidetz, N. F., Elmore, J. P., Limper, C. B., et al. (2020). Interleukin-2-inducible T-cell kinase deficiency impairs early pulmonary protection against Mycobacterium tuberculosis infection. Front. Immunol. 10:3103. doi: 10.3389/fimmu.2019.03103
Hussain, T., Zhao, D., Shah, S. Z. A., Sabir, N., Wang, J., Liao, Y., et al. (2019a). Nilotinib: A tyrosine kinase inhibitor mediates resistance to intracellular mycobacterium via regulating autophagy. Cells 8:506. doi: 10.3390/cells8050506
Hussain, T., Zhao, D., Shah, S. Z. A., Sabir, N., Wang, J., Liao, Y., et al. (2019b). PP2Ac modulates AMPK-mediated induction of autophagy in Mycobacterium bovis-infected macrophages. Int. J. Mol. Sci. 20:6030. doi: 10.3390/ijms20236030
Iizasa, E. I., Chuma, Y., Uematsu, T., Kubota, M., Kawaguchi, H., Umemura, M., et al. (2021). TREM2 is a receptor for non-glycosylated mycolic acids of mycobacteria that limits anti-mycobacterial macrophage activation. Nat. Commun. 12:2299. doi: 10.1038/s41467-021-22620-3
Imai, K., Kurita-Ochiai, T., and Ochiai, K. (2003). Mycobacterium bovis bacillus Calmette-Gueérin infection promotes SOCS induction and inhibits IFN-γ-stimulated JAK/STAT signaling in J774 macrophages. FEMS Immunol. Med. Microbiol. 39, 173–180. doi: 10.1016/s0928-8244(03)00231-1
Jaime-Lara, R. B., Roy, A., Wang, Y., Stanfill, A., Cashion, A. K., and Joseph, P. V. (2020). Gene co-expression networks are associated with obesity-related traits in kidney transplant recipients. BMC Med. Genet. 13:37. doi: 10.1186/s12920-020-0702-5
Jayaswal, S., Kamal, M. A., Dua, R., Gupta, S., Majumdar, T., Das, G., et al. (2010). Identification of host-dependent survival factors for intracellular Mycobacterium tuberculosis through an siRNA screen. PLoS Pathog. 6:e1000839. doi: 10.1371/journal.ppat.1000839
Jensen, K., Gallagher, I. J., Johnston, N., Welsh, M., Skuce, R., Williams, J. L., et al. (2018). Variation in the early host-pathogen interaction of bovine macrophages with divergent Mycobacterium bovis strains in the United Kingdom. Infect. Immun. 86, e00385–e00317. doi: 10.1128/IAI.00385-17
Jensen, K., Stevens, J. M., and Glass, E. J. (2019). Interleukin 10 knock-down in bovine monocyte-derived macrophages has distinct effects during infection with two divergent strains of Mycobacterium bovis. PLoS One 14:e0222437. doi: 10.1371/journal.pone.0222437
Jenum, S., Bakken, R., Dhanasekaran, S., Mukherjee, A., Lodha, R., Singh, S., et al. (2016). BLR1 and FCGR1A transcripts in peripheral blood associate with the extent of intrathoracic tuberculosis in children and predict treatment outcome. Sci. Rep. 6:38841. doi: 10.1038/srep38841
Jiang, D., Wubuli, A., Hu, X., Ikramullah, S., Maimaiti, A., Zhang, W., et al. (2015). The variations of IL-23R are associated with susceptibility and severe clinical forms of pulmonary tuberculosis in Chinese Uygurs. BMC Infect. Dis. 15:550. doi: 10.1186/s12879-015-1284-2
Jones, G. J., Pirson, C., Hewinson, R. G., and Vordermeier, H. M. (2010). Simultaneous measurement of antigen-stimulated interleukin-1β and gamma interferon production enhances test sensitivity for the detection of Mycobacterium bovis infection in cattle. Clin. Vaccine Immunol. 17, 1946–1951. doi: 10.1128/CVI.00377-10
Joshi, A., Rienks, M., Theofilatos, K., and Mayr, M. (2021). Systems biology in cardiovascular disease: a multiomics approach. Nat. Rev. Cardiol. 18, 313–330. doi: 10.1038/s41569-020-00477-1
Juárez, E., Carranza, C., Hernández-Sánchez, F., León-Contreras, J. C., Hernández-Pando, R., Escobedo, D., et al. (2012). NOD2 enhances the innate response of alveolar macrophages to Mycobacterium tuberculosis in humans. Eur. J. Immunol. 42, 880–889. doi: 10.1002/eji.201142105
Kahn, M. (2014). Can we safely target the WNT pathway? Nat. Rev. Drug Discov. 13, 513–532. doi: 10.1038/nrd4233
Kang, D. D., Lin, Y., Moreno, J.-R., Randall, T. D., and Khader, S. A. (2011). Profiling early lung immune responses in the mouse model of tuberculosis. PLoS One 6:e16161. doi: 10.1371/journal.pone.0016161
Kanipe, C., and Palmer, M. V. (2020). Mycobacterium bovis and you: A comprehensive look at the bacteria, its similarities to Mycobacterium tuberculosis, and its relationship with human disease. Tuberculosis 125:102006. doi: 10.1016/j.tube.2020.102006
Katiyar, A., Singh, H., and Azad, K. K. (2018). Identification of missing carbon fixation enzymes as potential drug targets in Mycobacterium tuberculosis. J. Integr. Bioinf. 15:20170041. doi: 10.1515/jib-2017-0041
Kaufmann, S. H. E., Liébana, E., Girvin, R. M., Welsh, M., Neill, S. D., and Pollock, J. M. (1999). Generation of CD8+ T-cell responses to Mycobacterium bovis and mycobacterial antigen in experimental bovine tuberculosis. Infect. Immun. 67, 1034–1044. doi: 10.1128/IAI.67.3.1034-1044.1999
Keane, J., Balcewicz-Sablinska, M. K., Remold, H. G., Chupp, G. L., Meek, B. B., Fenton, M. J., et al. (1997). Infection by Mycobacterium tuberculosis promotes human alveolar macrophage apoptosis. Infect. Immun. 65, 298–304. doi: 10.1128/iai.65.1.298-304.1997
Keane, J., Remold, H. G., and Kornfeld, H. (2000). Virulent Mycobacterium tuberculosis strains evade apoptosis of infected alveolar macrophages. J. Immunol. 164, 2016–2020. doi: 10.4049/jimmunol.164.4.2016
Kennedy, H. E., Welsh, M. D., Bryson, D. G., Cassidy, J. P., Forster, F. I., Howard, C. J., et al. (2002). Modulation of immune responses to Mycobacterium bovis in cattle depleted of WC1+ γδ T cells. Infect. Immun. 70, 1488–1500. doi: 10.1128/IAI.70.3.1488-1500.2002
Khan, N., Downey, J., Sanz, J., Kaufmann, E., Blankenhaus, B., Pacis, A., et al. (2020). M. Tuberculosis reprograms hematopoietic stem cells to limit myelopoiesis and impair trained immunity. Cells 183, 752–770.e22. doi: 10.1016/j.cell.2020.09.062
Khan, N., Pahari, S., Vidyarthi, A., Aqdas, M., and Agrewala, J. N. (2016). Stimulation through CD40 and TLR-4 is an effective host directed therapy against Mycobacterium tuberculosis. Front. Immunol. 7:386. doi: 10.3389/fimmu.2016.00386
Killick, K. E., Browne, J. A., Park, S. D. E., Magee, D. A., Martin, I., Meade, K. G., et al. (2011). Genome-wide transcriptional profiling of peripheral blood leukocytes from cattle infected with Mycobacterium bovis reveals suppression of host immune genes. BMC Genomics 12:611. doi: 10.1186/1471-2164-12-611
Killick, K. E., Magee, D. A., Park, S. D. E., Taraktsoglou, M., Browne, J. A., Conlon, K. M., et al. (2014). Key hub and bottleneck genes differentiate the macrophage response to virulent and attenuated Mycobacterium bovis. Front. Immunol. 5:422. doi: 10.3389/fimmu.2014.00422
Killick, K. E., Ní Cheallaigh, C., O'Farrelly, C., Hokamp, K., MacHugh, D. E., and Harris, J. (2013). Receptor-mediated recognition of mycobacterial pathogens. Cell. Microbiol. 15, 1484–1495. doi: 10.1111/cmi.12161
Kim, D., Paggi, J. M., Park, C., Bennett, C., and Salzberg, S. L. (2019). Graph-based genome alignment and genotyping with HISAT2 and HISAT-genotype. Nat. Biotechnol. 37, 907–915. doi: 10.1038/s41587-019-0201-4
Kimmey, J. M., Campbell, J. A., Weiss, L. A., Monte, K. J., Lenschow, D. J., and Stallings, C. L. (2017). The impact of ISGylation during Mycobacterium tuberculosis infection in mice. Microbes Infect. 19, 249–258. doi: 10.1016/j.micinf.2016.12.006
Klepp, L. I., Eirin, M. E., Garbaccio, S., Soria, M., Bigi, F., and Blanco, F. C. (2019). Identification of bovine tuberculosis biomarkers to detect tuberculin skin test and IFNγ release assay false negative cattle. Res. Vet. Sci. 122, 7–14. doi: 10.1016/j.rvsc.2018.10.016
Kong, Y., Feng, Z.-C., Zhang, Y.-L., Liu, X.-F., Ma, Y., Zhao, Z.-M., et al. (2020). Identification of immune-related genes contributing to the development of glioblastoma using weighted gene co-expression network analysis. Front. Immunol. 11:1281. doi: 10.3389/fimmu.2020.01281
Königshoff, M., and Eickelberg, O. (2010). WNT signaling in lung disease. Am. J. Respir. Cell Mol. Biol. 42, 21–31. doi: 10.1165/rcmb.2008-0485TR
Koo, M.-S., Subbian, S., and Kaplan, G. (2012). Strain specific transcriptional response in Mycobacterium tuberculosis infected macrophages. Cell Commun. Signaling 10:2. doi: 10.1186/1478-811X-10-2
Krutzik, S. R., and Modlin, R. L. (2004). The role of toll-like receptors in combating mycobacteria. Semin. Immunol. 16, 35–41. doi: 10.1016/j.smim.2003.10.005
Kumar, M., Sahu, S. K., Kumar, R., Subuddhi, A., Maji, R. K., Jana, K., et al. (2015). MicroRNA let-7 modulates the immune response to Mycobacterium tuberculosis infection via control of A20, an inhibitor of the NF-κB pathway. Cell Host Microbe 17, 345–356. doi: 10.1016/j.chom.2015.01.007
Landes, M. B., Rajaram, M. V. S., Nguyen, H., and Schlesinger, L. S. (2015). Role for NOD2 in Mycobacterium tuberculosis-induced iNOS expression and NO production in human macrophages. J. Leukoc. Biol. 97, 1111–1119. doi: 10.1189/jlb.3A1114-557R
Langfelder, P., and Horvath, S. (2008). WGCNA: an R package for weighted correlation network analysis. BMC Bioinf. 9:559. doi: 10.1186/1471-2105-9-559
Langfelder, P., Luo, R., Oldham, M. C., and Horvath, S. (2011). Is my network module preserved and reproducible? PLoS Comput. Biol. 7:e1001057. doi: 10.1371/journal.pcbi.1001057
Lavalett, L., Rodriguez, H., Ortega, H., Sadee, W., Schlesinger, L. S., and Barrera, L. F. (2017). Alveolar macrophages from tuberculosis patients display an altered inflammatory gene expression profile. Tuberculosis 107, 156–167. doi: 10.1016/j.tube.2017.08.012
Law, C. W., Chen, Y., Shi, W., and Smyth, G. K. (2014). Voom: precision weights unlock linear model analysis tools for RNA-seq read counts. Genome Biol. 15:R29. doi: 10.1186/gb-2014-15-2-r29
Lechartier, B., Rybniker, J., Zumla, A., and Cole, S. T. (2014). Tuberculosis drug discovery in the post-post-genomic era. EMBO Mol. Med. 6, 158–168. doi: 10.1002/emmm.201201772
Lee, W., VanderVen, B. C., Fahey, R. J., and Russell, D. G. (2013). Intracellular Mycobacterium tuberculosis exploits host-derived fatty acids to limit metabolic stress. J. Biol. Chem. 288, 6788–6800. doi: 10.1074/jbc.M112.445056
Li, L.-L., Dai, B., Sun, Y.-H., and Zhang, T.-T. (2020). The activation of IL-17 signaling pathway promotes pyroptosis in pneumonia-induced sepsis. Ann. Transl. Med. 8:674. doi: 10.21037/atm-19-1739
Li, M., Gao, H., Wang, J., and Wu, F.-X. (2018). Control principles for complex biological networks. Brief. Bioinform. 20, 2253–2266. doi: 10.1093/bib/bby088
Li, S., Liu, X., Liu, T., Meng, X., Yin, X., Fang, C., et al. (2017). Identification of biomarkers correlated with the TNM staging and overall survival of patients with bladder cancer. Front. Physiol. 8:947. doi: 10.3389/fphys.2017.00947
Li, F., Luo, J., Xu, H., Wang, Y., Jiang, W., Chang, K., et al. (2020). Early secreted antigenic target 6-kDa from Mycobacterium tuberculosis enhanced the protective innate immunity of macrophages partially via HIF1α. Biochem. Biophys. Res. Commun. 522, 26–32. doi: 10.1016/j.bbrc.2019.11.045
Li, L., Lv, J., He, Y., and Wang, Z. (2020). Gene network in pulmonary tuberculosis based on bioinformatic analysis. BMC Infect. Dis. 20:612. doi: 10.1186/s12879-020-05335-6
Li, L., Qiao, D., Fu, X., Lao, S., Zhang, X., and Wu, C. (2011). Identification of M.tuberculosis-specific Th1 cells expressing CD69 generated in vivo in pleural fluid cells from patients with tuberculous pleurisy. PLoS One 6:e23700. doi: 10.1371/journal.pone.0023700
Li, P., Wang, R., Dong, W., Hu, L., Zong, B., Zhang, Y., et al. (2017). Comparative proteomics analysis of human macrophages infected with virulent Mycobacterium bovis. Front. Cell. Infect. Microbiol. 7:65. doi: 10.3389/fcimb.2017.00065
Li, H., Wei, S., Fang, Y., Li, M., Li, X., Li, Z., et al. (2017). Quantitative proteomic analysis of host responses triggered by Mycobacterium tuberculosis infection in human macrophage cells. Acta Biochim. Biophys. Sin. 49, 835–844. doi: 10.1093/abbs/gmx080
Li, C., and Xu, J. (2019). Feature selection with the fisher score followed by the maximal clique centrality algorithm can accurately identify the hub genes of hepatocellular carcinoma. Sci. Rep. 9:17283. doi: 10.1038/s41598-019-53471-0
Li, J., Zhou, D., Qiu, W., Shi, Y., Yang, J.-J., Chen, S., et al. (2018). Application of weighted gene co-expression network analysis for data from paired design. Sci. Rep. 8:622. doi: 10.1038/s41598-017-18705-z
Liang, T., Chen, J., Xu, G., Zhang, Z., Xue, J., Zeng, H., et al. (2022). Ferroptosis-related gene SOCS1, a marker for tuberculosis diagnosis and treatment, involves in macrophage polarization and facilitates bone destruction in tuberculosis. Tuberculosis 132:102140. doi: 10.1016/j.tube.2021.102140
Lin, Y., Zhang, Y., Yu, H., Tian, R., Wang, G., and Li, F. (2019). Identification of unique key genes and miRNAs in latent tuberculosis infection by network analysis. Mol. Immunol. 112, 103–114. doi: 10.1016/j.molimm.2019.04.032
Lin, J., Zhao, D., Wang, J., Wang, Y., Li, H., Yin, X., et al. (2015). Transcriptome changes upon in vitro challenge with Mycobacterium bovis in monocyte-derived macrophages from bovine tuberculosis-infected and healthy cows. Vet. Immunol. Immunopathol. 163, 146–156. doi: 10.1016/j.vetimm.2014.12.001
Liu, Y., Li, J.-Y., Chen, S.-T., Huang, H.-R., and Cai, H. (2016). The rLrp of Mycobacterium tuberculosis inhibits proinflammatory cytokine production and downregulates APC function in mouse macrophages via a TLR2-mediated PI3K/Akt pathway activation-dependent mechanism. Cell. Mol. Immunol. 13, 729–745. doi: 10.1038/cmi.2015.58
Loo, Y.-M., and Gale, M. Jr. (2011). Immune signaling by RIG-I-like receptors. Immunity 34, 680–692. doi: 10.1016/j.immuni.2011.05.003
Looney, M., Lorenc, R., Halushka, M. K., and Karakousis, P. C. (2021). Key macrophage responses to infection with Mycobacterium tuberculosis are co-regulated by microRNAs and DNA methylation. Front. Immunol. 12:685237. doi: 10.3389/fimmu.2021.685237
Lu, L., Wei, R., Bhakta, S., Waddell, S. J., and Boix, E. (2021). Weighted gene co-expression network analysis identifies key modules and hub genes associated with mycobacterial infection of human macrophages. Antibiotics 10:97. doi: 10.3390/antibiotics10020097
Lu, C., Wu, J., Wang, H., Wang, S., Diao, N., Wang, F., et al. (2011). Novel biomarkers distinguishing active tuberculosis from latent infection identified by gene expression profile of peripheral blood mononuclear cells. PLoS One 6:e24290. doi: 10.1371/journal.pone.0024290
Lu, Q., Zhang, W., Fang, J., Zheng, J., Dong, C., and Xiong, S. (2020). Mycobacterium tuberculosis Rv1096, facilitates mycobacterial survival by modulating the NF-κB/MAPK pathway as peptidoglycan N-deacetylase. Mol. Immunol. 127, 47–55. doi: 10.1016/j.molimm.2020.08.005
Lubbers, R., Sutherland, J. S., Goletti, D., de Paus, R. A., van Moorsel, C. H. M., Veltkamp, M., et al. (2018). Complement component C1q as serum biomarker to detect active tuberculosis. Front. Immunol. 9:2427. doi: 10.3389/fimmu.2018.02427
Ma, J., Gui, H., Tang, Y., Ding, Y., Qian, G., Yang, M., et al. (2021). In silico identification of 10 hub genes and an miRNA–mRNA regulatory network in acute Kawasaki disease. Front. Genet. 12:585058. doi: 10.3389/fgene.2021.585058
Ma, Y., Han, F., Liang, J., Yang, J., Shi, J., Xue, J., et al. (2016). A species-specific activation of toll-like receptor signaling in bovine and sheep bronchial epithelial cells triggered by mycobacterial infections. Mol. Immunol. 71, 23–33. doi: 10.1016/j.molimm.2016.01.004
MacHugh, D. E., Gormley, E., Park, S. D. E., Browne, J. A., Taraktsoglou, M., O’Farrelly, C., et al. (2009). Gene expression profiling of the host response to Mycobacterium bovis infection in cattle. Transbound. Emerg. Dis. 56, 204–214. doi: 10.1111/j.1865-1682.2009.01082.x
MacHugh, D. E., Taraktsoglou, M., Killick, K. E., Nalpas, N. C., Browne, J. A., De Park, S., et al. (2012). Pan-genomic analysis of bovine monocyte-derived macrophage gene expression in response to in vitro infection with Mycobacterium avium subspecies paratuberculosis. Vet. Res. 43:25. doi: 10.1186/1297-9716-43-25
Magee, D. A., Conlon, K. M., Nalpas, N. C., Browne, J. A., Pirson, C., Healy, C., et al. (2014). Innate cytokine profiling of bovine alveolar macrophages reveals commonalities and divergence in the response to Mycobacterium bovis and Mycobacterium tuberculosis infection. Tuberculosis 94, 441–450. doi: 10.1016/j.tube.2014.04.004
Magee, D. A., Taraktsoglou, M., Killick, K. E., Nalpas, N. C., Browne, J. A., Park, S. D. E., et al. (2012). Global gene expression and systems biology analysis of bovine monocyte-derived macrophages in response to in vitro challenge with Mycobacterium bovis. PLoS One 7:e32034. doi: 10.1371/journal.pone.0032034
Mahla, R., Reddy, C., Prasad, D., and Kumar, H. (2013). Sweeten PAMPs: role of sugar complexed PAMPs in innate immunity and vaccine biology. Front. Immunol. 4:248. doi: 10.3389/fimmu.2013.00248
Mahon, R. N., Sande, O. J., Rojas, R. E., Levine, A. D., Harding, C. V., and Henry Boom, W. (2012). Mycobacterium tuberculosis ManLAM inhibits T-cell-receptor signaling by interference with ZAP-70, Lck and LAT phosphorylation. Cell. Immunol. 275, 98–105. doi: 10.1016/j.cellimm.2012.02.009
Mair, I., McNeilly, T. N., Corripio-Miyar, Y., Forman, R., and Else, K. J. (2021). Embracing nature’s complexity: Immunoparasitology in the wild. Semin. Immunol. 53:101525. doi: 10.1016/j.smim.2021.101525
Maji, A., Misra, R., Kumar Mondal, A., Kumar, D., Bajaj, D., Singhal, A., et al. (2015). Expression profiling of lymph nodes in tuberculosis patients reveal inflammatory milieu at site of infection. Sci. Rep. 5:15214. doi: 10.1038/srep15214
Malone, K. M., Rue-Albrecht, K., Magee, D. A., Conlon, K., Schubert, O. T., Nalpas, N. C., et al. (2018). Comparative 'omics analyses differentiate Mycobacterium tuberculosis and Mycobacterium bovis and reveal distinct macrophage responses to infection with the human and bovine tubercle bacilli. Microbial. Genomics 4:e000163. doi: 10.1099/mgen.0.000163
Manca, C., Tsenova, L., Freeman, S., Barczak, A. K., Tovey, M., Murray, P. J., et al. (2005). Hypervirulent M. tuberculosis W/Beijing strains upregulate type I IFNs and increase expression of negative regulators of the Jak-Stat pathway. J. Interf. Cytokine Res. 25, 694–701. doi: 10.1089/jir.2005.25.694
María Irene, C.-C., Juan Germán, R.-C., Gamaliel, L.-L., Dulce Adriana, M.-E., Estela Isabel, B., Brenda Nohemí, M. C., et al. (2021). Profiling the immune response to Mycobacterium tuberculosis Beijing family infection: a perspective from the transcriptome. Virulence 12, 1689–1704. doi: 10.1080/21505594.2021.1936432
Marsay, L., Matsumiya, M., Tanner, R., Poyntz, H., Griffiths, K. L., Stylianou, E., et al. (2013). Mycobacterial growth inhibition in murine splenocytes as a surrogate for protection against Mycobacterium tuberculosis (M. tb). Tuberculosis 93, 551–557. doi: 10.1016/j.tube.2013.04.007
Mascarello, A., Chiaradia, L. D., Vernal, J., Villarino, A., Guido, R. V. C., Perizzolo, P., et al. (2010). Inhibition of Mycobacterium tuberculosis tyrosine phosphatase PtpA by synthetic chalcones: kinetics, molecular modeling, toxicity and effect on growth. Bioorg. Med. Chem. 18, 3783–3789. doi: 10.1016/j.bmc.2010.04.051
Mason, M. J., Fan, G., Plath, K., Zhou, Q., and Horvath, S. (2009). Signed weighted gene co-expression network analysis of transcriptional regulation in murine embryonic stem cells. BMC Genomics 10:327. doi: 10.1186/1471-2164-10-327
McLoughlin, K. E., Correia, C. N., Browne, J. A., Magee, D. A., Nalpas, N. C., Rue-Albrecht, K., et al. (2021a). RNA-seq transcriptome analysis of peripheral blood from cattle infected with Mycobacterium bovis across an experimental time ccourse. Front. Vet. Sci. 8:662002. doi: 10.3389/fvets.2021.662002
McLoughlin, K. E., Correia, C. N., Browne, J. A., Magee, D. A., Nalpas, N. C., Rue-Albrecht, K., et al. (2021b). RNA-seq transcriptome analysis of peripheral blood from cattle infected with Mycobacterium bovis across an experimental time course. Front. Vet. Sci. 8:662002. doi: 10.3389/fvets.2021.662002
McLoughlin, K. E., Nalpas, N. C., Rue-Albrecht, K., Browne, J. A., Magee, D. A., Killick, K. E., et al. (2014). RNA-seq transcriptional profiling of peripheral blood leukocytes from cattle infected with Mycobacterium bovis. Front. Immunol. 5:396. doi: 10.3389/fimmu.2014.00396
Meade, K. G., Gormley, E., Doyle, M. B., Fitzsimons, T., O'Farrelly, C., Costello, E., et al. (2007). Innate gene repression associated with Mycobacterium bovis infection in cattle: toward a gene signature of disease. BMC Genomics 8:400. doi: 10.1186/1471-2164-8-400
Means, T. K., Golenbock, D. T., and Fenton, M. J. (2000). The biology of toll-like receptors. Cytokine Growth Factor Rev. 11, 219–232. doi: 10.1016/S1359-6101(00)00006-X
Meenu, S., Thiagarajan, S., Ramalingam, S., Michael, A., and Ramalingam, S. (2016). Modulation of host ubiquitin system genes in human endometrial cell line infected with Mycobacterium tuberculosis. Med. Microbiol. Immunol. 205, 163–171. doi: 10.1007/s00430-015-0432-z
Meunier, E., and Neyrolles, O. (2019). Die another way: Ferroptosis drives tuberculosis pathology. J. Exp. Med. 216, 471–473. doi: 10.1084/jem.20190038
Miao, L., Yin, R.-X., Zhang, Q.-H., Liao, P.-J., Wang, Y., Nie, R.-J., et al. (2019). A novel circRNA-miRNA-mRNA network identifies circ-YOD1 as a biomarker for coronary artery disease. Sci. Rep. 9:18314. doi: 10.1038/s41598-019-54603-2
Middleton, S., Steinbach, S., Coad, M., McGill, K., Brady, C., Duignan, A., et al. (2021). A molecularly defined skin test reagent for the diagnosis of bovine tuberculosis compatible with vaccination against Johne’s disease. Sci. Rep. 11:2929. doi: 10.1038/s41598-021-82434-7
Moguche, A. O., Musvosvi, M., Penn-Nicholson, A., Plumlee, C. R., Mearns, H., Geldenhuys, H., et al. (2017). Antigen availability shapes T cell differentiation and function during tuberculosis. Cell Host Microbe 21, 695–706.e5. doi: 10.1016/j.chom.2017.05.012
Mogues, T., Goodrich, M. E., Ryan, L., LaCourse, R., and North, R. J. (2001). The relative importance of T cell subsets in immunity and immunopathology of airborne Mycobacterium tuberculosis infection in mice. J. Exp. Med. 193, 271–280. doi: 10.1084/jem.193.3.271
Mohareer, K., Asalla, S., and Banerjee, S. (2018). Cell death at the cross roads of host-pathogen interaction in Mycobacterium tuberculosis infection. Tuberculosis 113, 99–121. doi: 10.1016/j.tube.2018.09.007
Mukund, K., and Subramaniam, S. (2015). Dysregulated mechanisms underlying Duchenne muscular dystrophy from co-expression network preservation analysis. BMC. Res. Notes 8:182. doi: 10.1186/s13104-015-1141-9
Naeem, M. A., Ahmad, W., Tyagi, R., Akram, Q., Younus, M., and Liu, X. (2021). Stealth strategies of Mycobacterium tuberculosis for immune evasion. Curr. Issues Mol. Biol. 41, 597–616. doi: 10.21775/cimb.041.597
Nagabhushanam, V., Solache, A., Ting, L.-M., Escaron, C. J., Zhang, J. Y., and Ernst, J. D. (2003). Innate inhibition of adaptive immunity: Mycobacterium tuberculosis-induced IL6 inhibits macrophage responses to IFN-γ. J. Immunol. 171, 4750–4757. doi: 10.4049/jimmunol.171.9.4750
Nager, M., Tarr, P. E., Haack, H. G., Martius, F., Stoebe, C., Frei, R., et al. (2009). Reactivation of bovine tuberculosis in patient treated with infliximab, Switzerland. Emerg. Infect. Dis. J. 15:1132, –1133. doi: 10.3201/eid1507.090024
Nalpas, N. C., Magee, D. A., Conlon, K. M., Browne, J. A., Healy, C., McLoughlin, K. E., et al. (2015). RNA sequencing provides exquisite insight into the manipulation of the alveolar macrophage by tubercle bacilli. Sci. Rep. 5:13629. doi: 10.1038/srep13629
Nalpas, N. C., Park, S. D. E., Magee, D. A., Taraktsoglou, M., Browne, J. A., Conlon, K. M., et al. (2013). Whole-transcriptome, high-throughput RNA sequence analysis of the bovine macrophage response to Mycobacterium bovis infection in vitro. BMC Genomics 14:230. doi: 10.1186/1471-2164-14-230
Ndlovu, H., and Marakalala, M. J. (2016). Granulomas and inflammation: host-directed therapies for tuberculosis. Front. Immunol. 7:434. doi: 10.3389/fimmu.2016.00434
Netea, M. G., Van der Meer, J. W. M., and Kullberg, B.-J. (2004). Toll-like receptors as an escape mechanism from the host defense. Trends Microbiol. 12, 484–488. doi: 10.1016/j.tim.2004.09.004
Ní Cheallaigh, C., Keane, J., Lavelle, E. C., Hope, J. C., and Harris, J. (2011). Autophagy in the immune response to tuberculosis: clinical perspectives. Clin. Exp. Immunol. 164, 291–300. doi: 10.1111/j.1365-2249.2011.04381.x
Noss, E. H., Pai, R. K., Sellati, T. J., Radolf, J. D., Belisle, J., Golenbock, D. T., et al. (2001). Toll-like receptor 2-dependent inhibition of macrophage class II MHC expression and antigen processing by 19-kDa lipoprotein of Mycobacterium tuberculosis. J. Immunol. 167, 910–918. doi: 10.4049/jimmunol.167.2.910
Nougayrède, J.-P., Taieb, F., Rycke, J. D., and Oswald, E. (2005). Cyclomodulins: bacterial effectors that modulate the eukaryotic cell cycle. Trends Microbiol. 13, 103–110. doi: 10.1016/j.tim.2005.01.002
Olea-Popelka, F., Muwonge, A., Perera, A., Dean, A. S., Mumford, E., Erlacher-Vindel, E., et al. (2017). Zoonotic tuberculosis in human beings caused by Mycobacterium bovis—a call for action. Lancet Infect. Dis. 17, e21–e25. doi: 10.1016/S1473-3099(16)30139-6
O'Leary, S., O'Sullivan, M. P., and Keane, J. (2011). IL-10 blocks phagosome maturation in Mycobacterium tuberculosis–infected human macrophages. Am. J. Respir. Cell Mol. Biol. 45, 172–180. doi: 10.1165/rcmb.2010-0319OC
Padhi, A., Pattnaik, K., Biswas, M., Jagadeb, M., Behera, A., and Sonawane, A. (2019). Mycobacterium tuberculosis LprE suppresses TLR2-dependent cathelicidin and autophagy expression to enhance bacterial survival in macrophages. J. Immunol. 203, 2665–2678. doi: 10.4049/jimmunol.1801301
Pahari, S., Kaur, G., Aqdas, M., Negi, S., Chatterjee, D., Bashir, H., et al. (2017). Bolstering immunity through pattern recognition receptors: A unique approach to control tuberculosis. Front. Immunol. 8:906. doi: 10.3389/fimmu.2017.00906
Paik, S., Kim, J. K., Chung, C., and Jo, E.-K. (2019). Autophagy: A new strategy for host-directed therapy of tuberculosis. Virulence 10, 448–459. doi: 10.1080/21505594.2018.1536598
Pajuelo, D., Gonzalez-Juarbe, N., and Niederweis, M. (2020). NAD hydrolysis by the tuberculosis necrotizing toxin induces lethal oxidative stress in macrophages. Cell. Microbiol. 22:e13115. doi: 10.1111/cmi.13115
Palmer, M. V., Thacker, T. C., Rabideau, M. M., Jones, G. J., Kanipe, C., Vordermeier, H. M., et al. (2020). Biomarkers of cell-mediated immunity to bovine tuberculosis. Vet. Immunol. Immunopathol. 220:109988. doi: 10.1016/j.vetimm.2019.109988
Papp, A. C., Azad, A. K., Pietrzak, M., Williams, A., Handelman, S. K., Igo, R. P. Jr., et al. (2018). AmpliSeq transcriptome analysis of human alveolar and monocyte-derived macrophages over time in response to Mycobacterium tuberculosis infection. PLoS One 13:e0198221. doi: 10.1371/journal.pone.0198221
Parasa, V. R., Muvva, J. R., Rose, J. F., Braian, C., Brighenti, S., and Lerm, M. (2017). Inhibition of tissue matrix metalloproteinases interferes with Mycobacterium tuberculosis-induced granuloma formation and reduces bacterial load in a human lung tissue model. Front. Microbiol. 8:2370. doi: 10.3389/fmicb.2017.02370
Park, S. K., Park, C.-S., Lee, H.-S., Park, K. S., Park, B. L., Cheong, H. S., et al. (2014). Functional polymorphism in aldehyde dehydrogenase-2 gene associated with risk of tuberculosis. BMC Med. Genet. 15:40. doi: 10.1186/1471-2350-15-40
Pathak, S. K., Basu, S., Basu, K. K., Banerjee, A., Pathak, S., Bhattacharyya, A., et al. (2007). Direct extracellular interaction between the early secreted antigen ESAT-6 of Mycobacterium tuberculosis and TLR2 inhibits TLR signaling in macrophages. Nat. Immunol. 8, 610–618. doi: 10.1038/ni1468
Pawar, K., Sharbati, J., Einspanier, R., and Sharbati, S. (2016). Mycobacterium bovis BCG interferes with miR-3619-5p control of cathepsin S in the process of autophagy. Front. Cell. Infect. Microbiol. 6:27. doi: 10.3389/fcimb.2016.00027
Petrilli, J. D., Araújo, L. E., da Silva, L. S., Laus, A. C., Müller, I., Reis, R. M., et al. (2020). Whole blood mRNA expression-based targets to discriminate active tuberculosis from latent infection and other pulmonary diseases. Sci. Rep. 10:22072. doi: 10.1038/s41598-020-78793-2
Piercy, J., Werling, D., and Coffey, T. J. (2007). Differential responses of bovine macrophages to infection with bovine-specific and non-bovine specific mycobacteria. Tuberculosis 87, 415–420. doi: 10.1016/j.tube.2007.06.001
Pires, D., Bernard, E. M., Pombo, J. P., Carmo, N., Fialho, C., Gutierrez, M. G., et al. (2017). Mycobacterium tuberculosis modulates miR-106b-5p to control cathepsin S expression resulting in higher pathogen survival and poor T-cell activation. Front. Immunol. 8:1819. doi: 10.3389/fimmu.2017.01819
Placido, R., Mancino, G., Amendola, A., Mariani, F., Vendetti, S., Piacentini, M., et al. (1997). Apoptosis of human monocytes/macrophages in Mycobacterium tuberculosis infection. J. Pathol. 181, 31–38. doi: 10.1002/(SICI)1096-9896(199701)181:1<31::AID-PATH722>3.0.CO;2-G
Pollock, J. M., and Neill, S. D. (2002). Mycobacterium bovis infection and tuberculosis in cattle. Vet. J. 163, 115–127. doi: 10.1053/tvjl.2001.0655
Pollock, J. M., Pollock, D. A., Campbell, D. G., Girvin, R. M., Crockard, A. D., Neill, S. D., et al. (1996). Dynamic changes in circulating and antigen-responsive T-cell subpopulations post-Mycobacterium bovis infection in cattle. Immunology 87, 236–241. doi: 10.1046/j.1365-2567.1996.457538.x
Pollock, J. M., Rodgers, J. D., Welsh, M. D., and McNair, J. (2006). Pathogenesis of bovine tuberculosis: the role of experimental models of infection. Vet. Microbiol. 112, 141–150. doi: 10.1016/j.vetmic.2005.11.032
Qualls, J. E., and Murray, P. J. (2016). Immunometabolism within the tuberculosis granuloma: amino acids, hypoxia, and cellular respiration. Semin. Immunopathol. 38, 139–152. doi: 10.1007/s00281-015-0534-0
Quesnel-Vallières, M., Weatheritt, R. J., Cordes, S. P., and Blencowe, B. J. (2019). Autism spectrum disorder: insights into convergent mechanisms from transcriptomics. Nat. Rev. Genet. 20, 51–63. doi: 10.1038/s41576-018-0066-2
Riquelme Medina, I., and Lubovac-Pilav, Z. (2016). Gene co-expression network analysis for identifying modules and functionally enriched pathways in type 1 diabetes. PLoS One 11:e0156006. doi: 10.1371/journal.pone.0156006
Rivero-Lezcano, O. M., González-Cortés, C., Reyes-Ruvalcaba, D., and Diez-Tascón, C. (2010). CCL20 is overexpressed in Mycobacterium tuberculosis-infected monocytes and inhibits the production of reactive oxygen species (ROS). Clin. Exp. Immunol. 162, 289–297. doi: 10.1111/j.1365-2249.2010.04168.x
Rohde, K., Yates, R. M., Purdy, G. E., and Russell, D. G. (2007). Mycobacterium tuberculosis and the environment within the phagosome. Immunol. Rev. 219, 37–54. doi: 10.1111/j.1600-065X.2007.00547.x
Rojas, M., Barrera, L. F., and García, L. F. (1998). Induction of apoptosis in murine macrophages by Mycobacterium tuberculosis is reactive oxygen intermediates-independent. Biochem. Biophys. Res. Commun. 247, 436–442. doi: 10.1006/bbrc.1998.8802
Roy, A., Díez-Guerrier, A., Ortega, J., de la Cruz, M. L., Sáez, J. L., Domínguez, L., et al. (2019). Evaluation of the McLintock syringe as a cause of non-specific reactions in the intradermal tuberculin test used for the diagnosis of bovine tuberculosis. Res. Vet. Sci. 122, 175–178. doi: 10.1016/j.rvsc.2018.11.025
Rusk, R. A., Palmer, M. V., Waters, W. R., and McGill, J. L. (2017). Measuring bovine γδ T cell function at the site of Mycobacterium bovis infection. Vet. Immunol. Immunopathol. 193–194, 38–49. doi: 10.1016/j.vetimm.2017.10.004
Russell, D. G., Cardona, P.-J., Kim, M.-J., Allain, S., and Altare, F. (2009). Foamy macrophages and the progression of the human tuberculosis granuloma. Nat. Immunol. 10, 943–948. doi: 10.1038/ni.1781
Sabio y García, J., Bigi, M. M., Klepp, L. I., García, E. A., Blanco, F. C., and Bigi, F. (2020). Does Mycobacterium bovis persist in cattle in a non-replicative latent state as Mycobacterium tuberculosis in human beings? Vet. Microbiol. 247:108758. doi: 10.1016/j.vetmic.2020.108758
Sakowski, E. T., Koster, S., Portal Celhay, C., Park, H. S., Shrestha, E., Hetzenecker, S. E., et al. (2015). Ubiquilin 1 promotes IFN-γ-induced xenophagy of Mycobacterium tuberculosis. PLoS Pathog. 11:e1005076. doi: 10.1371/journal.ppat.1005076
Salgame, P. (2005). Host innate and Th1 responses and the bacterial factors that control Mycobacterium tuberculosis infection. Curr. Opin. Immunol. 17, 374–380. doi: 10.1016/j.coi.2005.06.006
Salgame, P. (2011). MMPs in tuberculosis: granuloma creators and tissue destroyers. J. Clin. Invest. 121, 1686–1688. doi: 10.1172/JCI57423
Sambarey, A., Devaprasad, A., Baloni, P., Mishra, M., Mohan, A., Tyagi, P., et al. (2017). Meta-analysis of host response networks identifies a common core in tuberculosis. NPJ Syst. Biol. Appl. 3:4. doi: 10.1038/s41540-017-0005-4
Samten, B., Wang, X., and Barnes, P. F. (2009). Mycobacterium tuberculosis ESX-1 system-secreted protein ESAT-6 but not CFP10 inhibits human T-cell immune responses. Tuberculosis 89, S74–S76. doi: 10.1016/S1472-9792(09)70017-4
Sánchez-Soto, E., Ponce-Ramos, R., Hernández-Gutiérrez, R., Gutiérrez-Ortega, A., Álvarez, A. H., Martínez-Velázquez, M., et al. (2017). Colostrum proinflammatory cytokines as biomarkers of bovine immune response to bovine tuberculosis (bTB). Microb. Pathog. 103, 57–64. doi: 10.1016/j.micpath.2016.12.007
Sande, O. J., Karim, A. F., Li, Q., Ding, X., Harding, C. V., Rojas, R. E., et al. (2016). Mannose-capped lipoarabinomannan from Mycobacterium tuberculosis induces CD4+ T cell anergy via GRAIL. J. Immunol. 196, 691–702. doi: 10.4049/jimmunol.1500710
Schaale, K., Neumann, J., Schneider, D., Ehlers, S., and Reiling, N. (2011). Wnt signaling in macrophages: augmenting and inhibiting mycobacteria-induced inflammatory responses. Eur. J. Cell Biol. 90, 553–559. doi: 10.1016/j.ejcb.2010.11.004
Schiller, I., Oesch, B., Vordermeier, H. M., Palmer, M. V., Harris, B. N., Orloski, K. A., et al. (2010). Bovine tuberculosis: A review of current and emerging diagnostic techniques in view of their relevance for disease control and eradication. Transbound. Emerg. Dis. 57, 205–220. doi: 10.1111/j.1865-1682.2010.01148.x
Schneider, H., Downey, J., Smith, A., Zinselmeyer, B. H., Rush, C., Brewer, J. M., et al. (2006). Reversal of the TCR stop signal by CTLA-4. Science 313, 1972–1975. doi: 10.1126/science.1131078
Seto, S., Morimoto, K., Yoshida, T., Hiramatsu, M., Hijikata, M., Nagata, T., et al. (2020). Proteomic profiling reveals the architecture of granulomatous lesions caused by tuberculosis and Mycobacterium avium complex lung disease. Front. Microbiol. 10:3081. doi: 10.3389/fmicb.2019.03081
Shapira, T., Rankine-Wilson, L., Chao, J. D., Pichler, V., Rens, C., Pfeifer, T., et al. (2020). High-content screening of eukaryotic kinase inhibitors identify CHK2 inhibitor activity against Mycobacterium tuberculosis. Front. Microbiol. 11:553962. doi: 10.3389/fmicb.2020.553962
Sharifi, S., Pakdel, A., Ebrahimie, E., Aryan, Y., Ghaderi Zefrehee, M., and Reecy, J. M. (2019). Prediction of key regulators and downstream targets of E. coli induced mastitis. J. Appl. Genet. 60, 367–373. doi: 10.1007/s13353-019-00499-7
Shariq, M., Quadir, N., Sharma, N., Singh, J., Sheikh, J. A., Khubaib, M., et al. (2021). Mycobacterium tuberculosis RipA dampens TLR4-mediated host protective response using a multi-pronged approach involving autophagy, apoptosis, metabolic repurposing, and immune modulation. Front. Immunol. 12:636644. doi: 10.3389/fimmu.2021.636644
Sharma, S., Ryndak, M. B., Aggarwal, A. N., Yadav, R., Sethi, S., Masih, S., et al. (2017). Transcriptome analysis of mycobacteria in sputum samples of pulmonary tuberculosis patients. PLoS One 12:e0173508. doi: 10.1371/journal.pone.0173508
Sharma, M., Sharma, S., Roy, S., Varma, S., and Bose, M. (2007). Pulmonary epithelial cells are a source of interferon-γ in response to Mycobacterium tuberculosis infection. Immunol. Cell Biol. 85, 229–237. doi: 10.1038/sj.icb.7100037
Sharma, G., Sowpati, D. T., Singh, P., Khan, M. Z., Ganji, R., Upadhyay, S., et al. (2016). Genome-wide non-CpG methylation of the host genome during M. tuberculosis infection. Sci. Rep. 6:25006. doi: 10.1038/srep25006
Sheridan, M. P., Browne, J. A., Doyle, M. B., Fitzsimons, T., McGill, K., and Gormley, E. (2017). IL-10 suppression of IFN-γ responses in tuberculin-stimulated whole blood from Mycobacterium bovis infected cattle. Vet. Immunol. Immunopathol. 189, 36–42. doi: 10.1016/j.vetimm.2017.06.003
Sheybani, N., Bakhtiarizadeh, M. R., and Salehi, A. (2021). An integrated analysis of mRNAs, lncRNAs, and miRNAs based on weighted gene co-expression network analysis involved in bovine endometritis. Sci. Rep. 11:18050. doi: 10.1038/s41598-021-97319-y
Shi, L., Salamon, H., Eugenin, E. A., Pine, R., Cooper, A., and Gennaro, M. L. (2015). Infection with Mycobacterium tuberculosis induces the Warburg effect in mouse lungs. Sci. Rep. 5:18176. doi: 10.1038/srep18176
Shi, L., Wen, Z., Li, H., and Song, Y. (2021). Identification of hub genes associated with tuberculous pleurisy by integrated bioinformatics analysis. Front. Genet. 12:730491. doi: 10.3389/fgene.2021.730491
Shukla, S. K., Shukla, S., Chauhan, A., Sarvjeet,, Khan, R., Ahuja, A., et al. (2017). Differential gene expression in Mycobacterium bovis challenged monocyte-derived macrophages of cattle. Microb. Pathog. 113, 480–489. doi: 10.1016/j.micpath.2017.11.030
Shukla, S. K., Shukla, S., Khan, R., Ahuja, A., Singh, L. V., Kamble, N. M., et al. (2018). Pathway analysis of differentially expressed genes in Mycobacterium bovis challenged bovine macrophages. Microb. Pathog. 115, 343–352. doi: 10.1016/j.micpath.2017.11.065
Silva, C. A., Ribeiro-dos-Santos, A., Gonçalves, W. G., Pinto, P., Pantoja, R. P., Vinasco-Sandoval, T., et al. (2021). Can miRNA indicate risk of illness after continuous exposure to M. tuberculosis? Int. J. Mol. Sci. 22:3674. doi: 10.3390/ijms22073674
Silveira-Mattos, P. S., Barreto-Duarte, B., Vasconcelos, B., Fukutani, K. F., Vinhaes, C. L., Oliveira-De-Souza, D., et al. (2019). Differential expression of activation markers by Mycobacterium tuberculosis-specific CD4+ T cell distinguishes extrapulmonary from pulmonary tuberculosis and latent infection. Clin. Infect. Dis. 71, 1905–1911. doi: 10.1093/cid/ciz1070
Simmons, D. P., Canaday, D. H., Liu, Y., Li, Q., Huang, A., Boom, W. H., et al. (2010). Mycobacterium tuberculosis and TLR2 agonists inhibit induction of type I IFN and class I MHC antigen cross processing by TLR9. J. Immunol. 185, 2405–2415. doi: 10.4049/jimmunol.0904005
Skinner, M. A., Parlane, N., McCarthy, A., and Buddle, B. M. (2003). Cytotoxic T-cell responses to Mycobacterium bovis during experimental infection of cattle with bovine tuberculosis. Immunology 110, 234–241. doi: 10.1046/j.1365-2567.2003.01731.x
Smith, N. H., Gordon, S. V., de la Rua-Domenech, R., Clifton-Hadley, R. S., and Hewinson, R. G. (2006). Bottlenecks and broomsticks: the molecular evolution of Mycobacterium bovis. Nat. Rev. Microbiol. 4, 670–681. doi: 10.1038/nrmicro1472
Smyth, G. K. (2005). “Limma: linear models for microarray data” in Bioinformatics and Computational Biology Solutions Using R and Bioconductor. eds. R. Gentleman, V. J. Carey, W. Huber, R. A. Irizarry, and S. Dudoit (New York, NY: Springer New York), 397–420.
Song, L., Langfelder, P., and Horvath, S. (2012). Comparison of co-expression measures: mutual information, correlation, and model based indices. BMC Bioinf. 13:328. doi: 10.1186/1471-2105-13-328
Songane, M., Kleinnijenhuis, J., Netea, M. G., and van Crevel, R. (2012). The role of autophagy in host defence against Mycobacterium tuberculosis infection. Tuberculosis 92, 388–396. doi: 10.1016/j.tube.2012.05.004
Strong, E. J., Wang, J., Ng, T. W., Porcelli, S. A., Lee, S., and Siegrist, M. S. (2022). Mycobacterium tuberculosis PPE51 inhibits autophagy by suppressing toll-like receptor 2-dependent signaling. MBio 13, e02974–e02921. doi: 10.1128/mbio.02974-21
Subuddhi, A., Kumar, M., Majumder, D., Sarkar, A., Ghosh, Z., Vasudevan, M., et al. (2020). Unraveling the role of H3K4 trimethylation and lncRNA HOTAIR in SATB1 and DUSP4-dependent survival of virulent Mycobacterium tuberculosis in macrophages. Tuberculosis 120:101897. doi: 10.1016/j.tube.2019.101897
Sun, Y., Chen, G., Liu, Z., Yu, L., and Shang, Y. (2020). A bioinformatics analysis to identify novel biomarkers for prognosis of pulmonary tuberculosis. BMC Pulm. Med. 20:279. doi: 10.1186/s12890-020-01316-2
Szklarczyk, D., Gable, A. L., Lyon, D., Junge, A., Wyder, S., Huerta-Cepas, J., et al. (2018). STRING v11: protein–protein association networks with increased coverage, supporting functional discovery in genome-wide experimental datasets. Nucleic Acids Res. 47, D607–D613. doi: 10.1093/nar/gky1131
Tang, D., Kang, R., Coyne, C. B., Zeh, H. J., and Lotze, M. T. (2012). PAMPs and DAMPs: signal 0s that spur autophagy and immunity. Immunol. Rev. 249, 158–175. doi: 10.1111/j.1600-065X.2012.01146.x
Thakur, A., Andrea, A., Mikkelsen, H., Woodworth, J. S., Andersen, P., Jungersen, G., et al. (2018). Targeting the Mincle and TLR3 receptor using the dual agonist cationic adjuvant formulation 9 (CAF09) induces humoral and polyfunctional memory T cell responses in calves. PLoS One 13:e0201253. doi: 10.1371/journal.pone.0201253
Trinchieri, G., and Sher, A. (2007). Cooperation of toll-like receptor signals in innate immune defence. Nat. Rev. Immunol. 7, 179–190. doi: 10.1038/nri2038
Tripathi, D., Welch, E., Cheekatla, S. S., Radhakrishnan, R. K., Venkatasubramanian, S., Paidipally, P., et al. (2018). Alcohol enhances type 1 interferon-α production and mortality in young mice infected with Mycobacterium tuberculosis. PLoS Pathog. 14:e1007174. doi: 10.1371/journal.ppat.1007174
Tsai, K.-N., Chan, E.-C., Tsai, T.-Y., Chen, K.-T., Chen, C.-Y., Hung, K., et al. (2009). Cytotoxic effect of recombinant Mycobacterium tuberculosis CFP-10/ESAT-6 protein on the crucial pathways of WI-38 cells. J. Biomed. Biotechnol. 2009:917084. doi: 10.1155/2009/917084
Vainchenker, W., and Constantinescu, S. N. (2013). JAK/STAT signaling in hematological malignancies. Oncogene 32, 2601–2613. doi: 10.1038/onc.2012.347
van Dam, S., Võsa, U., van der Graaf, A., Franke, L., and de Magalhães, J. P. (2017). Gene co-expression analysis for functional classification and gene–disease predictions. Brief. Bioinform. 19, bbw139–bbw592. doi: 10.1093/bib/bbw139
Vayr, F., Martin-Blondel, G., Savall, F., Soulat, J.-M., Deffontaines, G., and Herin, F. (2018). Occupational exposure to human Mycobacterium bovis infection: A systematic review. PLoS Negl. Trop. Dis. 12:e0006208. doi: 10.1371/journal.pntd.0006208
Vega-Manriquez, X., López-Vidal, Y., Moran, J., Adams, L. G., and Gutiérrez-Pabello, J. A. (2007). Apoptosis-inducing factor participation in bovine macrophage Mycobacterium bovis-induced caspase-independent cell death. Infect. Immun. 75, 1223–1228. doi: 10.1128/IAI.01047-06
Vegh, P., Magee, D. A., Nalpas, N. C., Bryan, K., McCabe, M. S., Browne, J. A., et al. (2015). MicroRNA profiling of the bovine alveolar macrophage response to Mycobacterium bovis infection suggests pathogen survival is enhanced by microRNA regulation of endocytosis and lysosome trafficking. Tuberculosis 95, 60–67. doi: 10.1016/j.tube.2014.10.011
Vereecke, L., Beyaert, R., and van Loo, G. (2009). The ubiquitin-editing enzyme A20 (TNFAIP3) is a central regulator of immunopathology. Trends Immunol. 30, 383–391. doi: 10.1016/j.it.2009.05.007
Villarino, A. V., Kanno, Y., and O'Shea, J. J. (2017). Mechanisms and consequences of Jak–STAT signaling in the immune system. Nat. Immunol. 18, 374–384. doi: 10.1038/ni.3691
Villarreal-Ramos, B., Berg, S., Whelan, A., Holbert, S., Carreras, F., Salguero, F. J., et al. (2018). Experimental infection of cattle with Mycobacterium tuberculosis isolates shows the attenuation of the human tubercle bacillus for cattle. Sci. Rep. 8:894. doi: 10.1038/s41598-017-18575-5
Vinayagam, A., Gibson, T. E., Lee, H.-J., Yilmazel, B., Roesel, C., Hu, Y., et al. (2016). Controllability analysis of the directed human protein interaction network identifies disease genes and drug targets. Proc. Natl. Acad. Sci. 113, 4976–4981. doi: 10.1073/pnas.1603992113
Vordermeier, H. M., Chambers, M. A., Cockle, P. J., Whelan, A. O., Simmons, J., and Hewinson, R. G. (2002). Correlation of ESAT-6-specific gamma interferon production with pathology in cattle following Mycobacterium bovis BCG vaccination against experimental bovine tuberculosis. Infect. Immun. 70, 3026–3032. doi: 10.1128/IAI.70.6.3026-3032.2002
Walzl, G., Ronacher, K., Hanekom, W., Scriba, T. J., and Zumla, A. (2011). Immunological biomarkers of tuberculosis. Nat. Rev. Immunol. 11, 343–354. doi: 10.1038/nri2960
Wan, Q., Tang, J., Han, Y., and Wang, D. (2018). Co-expression modules construction by WGCNA and identify potential prognostic markers of uveal melanoma. Exp. Eye Res. 166, 13–20. doi: 10.1016/j.exer.2017.10.007
Wang, Z., Arat, S., Magid-Slav, M., and Brown, J. R. (2018). Meta-analysis of human gene expression in response to Mycobacterium tuberculosis infection reveals potential therapeutic targets. BMC Syst. Biol. 12:3. doi: 10.1186/s12918-017-0524-z
Wang, J., Hussain, T., Yue, R., Liao, Y., Li, Q., Yao, J., et al. (2018). MicroRNA-199a inhibits cellular autophagy and downregulates IFN-β expression by targeting TBK1 in Mycobacterium bovis infected cells. Front. Cell. Infect. Microbiol. 8:238. doi: 10.3389/fcimb.2018.00238
Wang, L.-X., Li, Y., and Chen, G.-Z. (2018). Network-based co-expression analysis for exploring the potential diagnostic biomarkers of metastatic melanoma. PLoS One 13:e0190447. doi: 10.1371/journal.pone.0190447
Wang, J., Li, B.-X., Ge, P.-P., Li, J., Wang, Q., Gao, G. F., et al. (2015). Mycobacterium tuberculosis suppresses innate immunity by coopting the host ubiquitin system. Nat. Immunol. 16, 237–245. doi: 10.1038/ni.3096
Wang, C., Li, Y.-Y., Li, X., Wei, L.-L., Yang, X.-Y., Xu, D.-D., et al. (2014). Serum complement C4b, fibronectin, and prolidase are associated with the pathological changes of pulmonary tuberculosis. BMC Infect. Dis. 14:52. doi: 10.1186/1471-2334-14-52
Wang, X., Mehra, S., Kaushal, D., Veazey, R. S., and Xu, H. (2021). Abnormal tryptophan metabolism in HIV and Mycobacterium tuberculosis infection. Front. Microbiol. 12:666227. doi: 10.3389/fmicb.2021.666227
Wang, Y., Wang, J., Tang, Q., and Ren, G. (2021). Identification of UBE2C as hub gene in driving prostate cancer by integrated bioinformatics analysis. PLoS One 16:e0247827. doi: 10.1371/journal.pone.0247827
Wang, Y., Zhou, X., Lin, J., Yin, F., Xu, L., Huang, Y., et al. (2011). Effects of Mycobacterium bovis on monocyte-derived macrophages from bovine tuberculosis infection and healthy cattle. FEMS Microbiol. Lett. 321, 30–36. doi: 10.1111/j.1574-6968.2011.02304.x
Wang, J., Zhou, X., Pan, B., Wang, H., Shi, F., Gan, W., et al. (2013). Expression pattern of interferon-inducible transcriptional genes in neutrophils during bovine tuberculosis infection. DNA Cell Biol. 32, 480–486. doi: 10.1089/dna.2012.1941
Waters, W. R., Maggioli, M. F., McGill, J. L., Lyashchenko, K. P., and Palmer, M. V. (2014). Relevance of bovine tuberculosis research to the understanding of human disease: historical perspectives, approaches, and immunologic mechanisms. Vet. Immunol. Immunopathol. 159, 113–132. doi: 10.1016/j.vetimm.2014.02.009
Waters, W. R., Palmer, M. V., Buddle, B. M., and Vordermeier, H. M. (2012). Bovine tuberculosis vaccine research: historical perspectives and recent advances. Vaccine 30, 2611–2622. doi: 10.1016/j.vaccine.2012.02.018
Wedlock, D. N., Denis, M., Painter, G. F., Ainge, G. D., Vordermeier, H. M., Hewinson, R. G., et al. (2008). Enhanced protection against bovine tuberculosis after coadministration of Mycobacterium bovis BCG with a mycobacterial protein vaccine-adjuvant combination but not after coadministration of adjuvant alone. Clin. Vaccine Immunol. 15, 765–772. doi: 10.1128/CVI.00034-08
Wei, S.-N., Zhao, W.-J., Zeng, X.-J., Kang, Y.-M., Du, J., and Li, H.-H. (2015). Microarray and co-expression network analysis of genes associated with acute doxorubicin cardiomyopathy in mice. Cardiovasc. Toxicol. 15, 377–393. doi: 10.1007/s12012-014-9306-7
Weiner, J. 3rd, Parida, S. K., Maertzdorf, J., Black, G. F., Repsilber, D., Telaar, A., et al. (2012). Biomarkers of inflammation, immunosuppression and stress are revealed by metabolomic profiling of tuberculosis patients. PLoS One 7:e40221. doi: 10.1371/journal.pone.0040221
White, M. J., He, H., Penoske, R. M., Twining, S. S., and Zahrt, T. C. (2010). PepD participates in the mycobacterial stress response mediated through MprAB and SigE. J. Bacteriol. 192, 1498–1510. doi: 10.1128/JB.01167-09
Wiarda, J. E., Boggiatto, P. M., Bayles, D. O., Waters, W. R., Thacker, T. C., and Palmer, M. V. (2020). Severity of bovine tuberculosis is associated with innate immune-biased transcriptional signatures of whole blood in early weeks after experimental Mycobacterium bovis infection. PLoS One 15:e0239938. doi: 10.1371/journal.pone.0239938
Widdison, S., and Coffey, T. J. (2011). Cattle and chemokines: evidence for species-specific evolution of the bovine chemokine system. Anim. Genet. 42, 341–353. doi: 10.1111/j.1365-2052.2011.02200.x
Widdison, S., Watson, M., and Coffey, T. J. (2009). Correlation between lymph node pathology and chemokine expression during bovine tuberculosis. Tuberculosis 89, 417–422. doi: 10.1016/j.tube.2009.09.003
Widdison, S., Watson, M., and Coffey, T. J. (2011). Early response of bovine alveolar macrophages to infection with live and heat-killed Mycobacterium bovis. Dev. Comp. Immunol. 35, 580–591. doi: 10.1016/j.dci.2011.01.001
Widdison, S., Watson, M., Piercy, J., Howard, C., and Coffey, T. J. (2008). Granulocyte chemotactic properties of M. tuberculosis versus M. bovis-infected bovine alveolar macrophages. Mol. Immunol. 45, 740–749. doi: 10.1016/j.molimm.2007.06.357
Wilburn, K. M., Fieweger, R. A., and VanderVen, B. C. (2018). Cholesterol and fatty acids grease the wheels of Mycobacterium tuberculosis pathogenesis. Pathog. Dis. 76:fty021. doi: 10.1093/femspd/fty021
Wong, D., Bach, H., Sun, J., Hmama, Z., and Av-Gay, Y. (2011). Mycobacterium tuberculosis protein tyrosine phosphatase (PtpA) excludes host vacuolar-H+–ATPase to inhibit phagosome acidification. Proc. Natl. Acad. Sci. 108, 19371–19376. doi: 10.1073/pnas.1109201108
Wong, D., Chao, J. D., and Av-Gay, Y. (2013). Mycobacterium tuberculosis-secreted phosphatases: from pathogenesis to targets for TB drug development. Trends Microbiol. 21, 100–109. doi: 10.1016/j.tim.2012.09.002
Wong, K.-W., and Jacobs, W. R. Jr. (2011). Critical role for NLRP3 in necrotic death triggered by Mycobacterium tuberculosis. Cell. Microbiol. 13, 1371–1384. doi: 10.1111/j.1462-5822.2011.01625.x
Wu, S., Wang, M.-G., Wang, Y., and He, J.-Q. (2019). Polymorphisms of cytokine genes and tuberculosis in two independent studies. Sci. Rep. 9:2507. doi: 10.1038/s41598-019-39249-4
Wu, S., Wang, Y., Zhang, M., Shrestha, S. S., Wang, M., and He, J.-Q. (2018). Genetic polymorphisms of IL1B, IL6, and TNFα in a Chinese Han population with pulmonary tuberculosis. Biomed. Res. Int. 2018:3010898. doi: 10.1155/2018/3010898
Xaus, J., Besalduch, N., Comalada, M., Marcoval, J., Pujol, R., Mañá, J., et al. (2003). High expression of p21Waf1 in sarcoid granulomas: a putative role for long-lasting inflammation. J. Leukoc. Biol. 74, 295–301. doi: 10.1189/jlb.1202628
Xaus, J., Cardó, M., Valledor, A. F., Soler, C., Lloberas, J., and Celada, A. (1999). Interferon γ induces the expression of p21waf-1 and arrests macrophage cell cycle, preventing induction of apoptosis. Immunity 11, 103–113. doi: 10.1016/S1074-7613(00)80085-0
Xie, X., Li, F., Chen, J.-W., and Wang, J. (2014). Risk of tuberculosis infection in anti-TNF-α biological therapy: from bench to bedside. J. Microbiol. Immunol. Infect. 47, 268–274. doi: 10.1016/j.jmii.2013.03.005
Xin, P., Xu, X., Deng, C., Liu, S., Wang, Y., Zhou, X., et al. (2020). The role of JAK/STAT signaling pathway and its inhibitors in diseases. Int. Immunopharmacol. 80:106210. doi: 10.1016/j.intimp.2020.106210
Xiong, W., Wen, Q., Du, X., Wang, J., He, W., Wang, R., et al. (2018). Novel function of cyclooxygenase-2: suppressing mycobacteria by promoting autophagy via the protein kinase B/mammalian target of rapamycin pathway. J. Infect. Dis. 217, 1267–1279. doi: 10.1093/infdis/jiy033
Xu, D., Li, Y., Li, X., Wei, L.-L., Pan, Z., Jiang, T.-T., et al. (2015). Serum protein S100A9, SOD3, and MMP9 as new diagnostic biomarkers for pulmonary tuberculosis by iTRAQ-coupled two-dimensional LC-MS/MS. Proteomics 15, 58–67. doi: 10.1002/pmic.201400366
Yang, D., Fu, X., He, S., Ning, X., and Ling, M. (2017). Analysis of differentially expressed proteins in Mycobacterium avium-infected macrophages comparing with Mycobacterium tuberculosis-infected macrophages. Biomed. Res. Int. 2017:5103803. doi: 10.1155/2017/5103803
Yang, H., Wang, Y., Zhang, Z., and Li, H. (2020). Identification of KIF18B as a hub candidate gene in the metastasis of clear cell renal cell carcinoma by weighted gene co-expression network analysis. Front. Genet. 11:905. doi: 10.3389/fgene.2020.00905
Yao, K., Chen, Q., Wu, Y., Liu, F., Chen, X., and Zhang, Y. (2017). Unphosphorylated STAT1 represses apoptosis in macrophages during mycobacterium tuberculosis infection. J. Cell Sci. 130, 1740–1751. doi: 10.1242/jcs.200659
Yarilina, A., Park-Min, K.-H., Antoniv, T., Hu, X., and Ivashkiv, L. B. (2008). TNF activates an IRF1-dependent autocrine loop leading to sustained expression of chemokines and STAT1-dependent type I interferon–response genes. Nat. Immunol. 9, 378–387. doi: 10.1038/ni1576
Yi, F., Hu, J., Zhu, X., Wang, Y., Yu, Q., Deng, J., et al. (2021). Transcriptional profiling of human peripheral blood mononuclear cells stimulated by Mycobacterium tuberculosis PPE57 identifies characteristic genes associated with type I interferon signaling. Front. Cell. Infect. Microbiol. 11:716809. doi: 10.3389/fcimb.2021.716809
Yu, J. S. L., and Cui, W. (2016). Proliferation, survival and metabolism: the role of PI3K/AKT/mTOR signalling in pluripotency and cell fate determination. Development 143, 3050–3060. doi: 10.1242/dev.137075
Zhang, B., and Horvath, S. (2005). A general framework for weighted gene co-expression network analysis. Stat. Appl. Genet. Mol. Biol. 4:Article17. doi: 10.2202/1544-6115.1128
Zhang, X., Huang, T., Wu, Y., Peng, W., Xie, H., Pan, M., et al. (2017). Inhibition of the PI3K-Akt-mTOR signaling pathway in T lymphocytes in patients with active tuberculosis. Int. J. Infect. Dis. 59, 110–117. doi: 10.1016/j.ijid.2017.04.004
Zhang, T., Rao, G., and Gao, X. (2021). Identification of hub genes in tuberculosis via bioinformatics analysis. Comput. Math. Methods Med. 2021:8159879. doi: 10.1155/2021/8159879
Zhang, Y., Zhang, X., Zhao, Z., Zheng, Y., Xiao, Z., and Li, F. (2019). Integrated bioinformatics analysis and validation revealed potential immune-regulatory miR-892b, miR-199b-5p and miR-582–5p as diagnostic biomarkers in active tuberculosis. Microb. Pathog. 134:103563. doi: 10.1016/j.micpath.2019.103563
Zhao, J., Gao, S., Chen, C., Li, H., Wang, S., Yu, Y., et al. (2022). Screening and identification of differentially expressed long non-coding RNAs in multidrug-resistant tuberculosis. PeerJ 10:e12776. doi: 10.7717/peerj.12776
Zhou, Y., Shah, S. Z. A., Yang, L., Zhang, Z., Zhou, X., and Zhao, D. (2016). Virulent Mycobacterium bovis Beijing strain activates the NLRP7 inflammasome in THP-1 macrophages. PLoS One 11:e0152853. doi: 10.1371/journal.pone.0152853
Keywords: bovine tuberculosis, hub-central gene, maximal clique centrality, Mycobacterium bovis, RNA-seq, systems biology, weighted gene co-expression network analysis
Citation: Hasankhani A, Bahrami A, Mackie S, Maghsoodi S, Alawamleh HSK, Sheybani N, Safarpoor Dehkordi F, Rajabi F, Javanmard G, Khadem H, Barkema HW and De Donato M (2022) In-depth systems biological evaluation of bovine alveolar macrophages suggests novel insights into molecular mechanisms underlying Mycobacterium bovis infection. Front. Microbiol. 13:1041314. doi: 10.3389/fmicb.2022.1041314
Edited by:
Bernat Pérez de Val, Centre for Research on Animal Health, SpainReviewed by:
Cristina Lourdes Vazquez, Instituto Nacional de Tecnología Agropecuaria (INTA), Oliveros, ArgentinaHugo Esquivel-Solís, CONACYT Centro de Investigación y Asistencia en Tecnología y Diseño del Estado de Jalisco (CIATEJ), Mexico
Copyright © 2022 Hasankhani, Bahrami, Mackie, Maghsoodi, Alawamleh, Sheybani, Safarpoor Dehkordi, Rajabi, Javanmard, Khadem, Barkema and De Donato. This is an open-access article distributed under the terms of the Creative Commons Attribution License (CC BY). The use, distribution or reproduction in other forums is permitted, provided the original author(s) and the copyright owner(s) are credited and that the original publication in this journal is cited, in accordance with accepted academic practice. No use, distribution or reproduction is permitted which does not comply with these terms.
*Correspondence: Aliakbar Hasankhani, QS5oYXNhbmtoYW5pNzRAdXQuYWMuaXI=; Abolfazl Bahrami, QS5CYWhyYW1pQHV0LmFjLmly; Farhad Safarpoor Dehkordi, Ri5zYWZhcnBvb3JAdXQuYWMuaXI=
†These authors have contributed equally to this work and share first authorship