- 1School of Mathematical Sciences, Ocean University of China, Qingdao, China
- 2Department of Respiratory and Critical Care, Emergency General Hospital, Beijing, China
- 3Geneis Beijing Co., Ltd., Beijing, China
- 4Qingdao Geneis Institute of Big Data Mining and Precision Medicine, Qingdao, China
- 5School of Life Sciences, Jiangsu University, Zhenjiang, Jiangsu, China
- 6Chifeng Municipal Hospital, Chifeng, China
Background: Local recurrence and distant metastasis are the main causes of death in patients with lung cancer. Multiple studies have described the recurrence or metastasis of lung cancer at the genetic level. However, association between the microbiome of lung cancer tissue and recurrence or metastasis remains to be discovered. Here, we aimed to identify the bacterial biomarkers capable of distinguishing patients with lung cancer from recurrence or metastasis, and how it related to the severity of patients with lung cancer.
Methods: We applied microbiome pipeline to bacterial communities of 134 non-recurrence and non-metastasis (non-RM) and 174 recurrence or metastasis (RM) samples downloaded from The Cancer Genome Atlas (TCGA). Co-occurrence network was built to explore the bacterial interactions in lung cancer tissue of RM and non-RM. Finally, the Kaplan–Meier survival analysis was used to evaluate the association between bacterial biomarkers and patient survival.
Results: Compared with non-RM, the bacterial community of RM had lower richness and higher Bray–Curtis dissimilarity index. Interestingly, the co-occurrence network of non-RM was more complex than RM. The top 500 genera in relative abundance obtained an area under the curve (AUC) of 0.72 when discriminating between RM and non-RM. There were significant differences in the relative abundances of Acidovorax, Clostridioides, Succinimonas, and Shewanella, and so on between RM and non-RM. These biomarkers played a role in predicting the survival of lung cancer patients and were significantly associated with lung cancer stage.
Conclusion: This study provides the first evidence for the prediction of lung cancer recurrence or metastasis by bacteria in lung cancer tissue. Our results highlights that bacterial biomarkers that distinguish RM and non-RM are also associated with patient survival and disease severity.
Introduction
Lung cancer is still the leading cause of cancer deaths worldwide. Local recurrence and distant metastasis are the primary causes of morbidity and mortality, and account for up to 95% of deaths related to lung cancer (Seyfried and Huysentruyt, 2013). Despite advances in therapeutic strategies, especially targeted therapy and immunotherapy, the prognosis remains poor because most patients have extensive metastases at diagnosis (Herbst et al., 2018; Liu et al., 2021). Clinically, a large number of patients with early-stage lung cancer relapse after surgery due to the neglected distant metastasis (Lu Y. et al., 2021). Thus, capturing the signal of metastasis in patients with early-stage lung cancer and continuously monitoring cancer progression after surgery is of great significance for reducing patient mortality.
Growing research has suggested that microbial communities influence the occurrence, progression, metastasis, and response to therapy of multiple cancers (Cullin et al., 2021; Yang M. et al., 2022). For example, studies have shown that Fusobacterium nucleatum may trigger cancer through multiple ways, and is related to cancer cell invasion and metastasis (Bullman et al., 2017). Recently, Bertocchi et al. found that intratumoral CRC-associated Escherichia coli could migrate to the liver following gut vascular barrier disruption and then prime the liver microenvironment to directly promote metastasis (Bertocchi et al., 2021). In addition, multiple studies have shown that enterotoxigenic Bacteroides fragilis could encode a toxin that ultimately induces chronic intestinal inflammation and tissue damage in colorectal cancer by targeting intestinal cells (Boleij et al., 2015; Cheng et al., 2020). However, the potential association between microbial communities of cancer tissue and lung cancer metastasis remains a knowledge gap.
A prominent reason for the high mortality rate of lung cancer is that it is initially asymptomatic and typically discovered at advanced stages (Nasim et al., 2019). Therefore, it is urgent to accurately identify the biomarkers in each stage of lung cancer and adjust the treatment measures for different stages (Yang et al., 2021). Zheng et al. identified 13 gut microbes as biomarkers with high accuracy in predicting early-stage lung cancer by 16 s rRNA sequencing analysis (Zheng et al., 2020). A survey of the gut and sputum microbiota of lung cancer patients at different stages by Lu et al. revealed that these two microbiomes were associated with distant metastasis and that microbial biomarkers across disease stages were largely shared (Lu H. et al., 2021). However, although the potential relationship between gut microbes and non-gut-related cancers is largely unraveled (Erdman and Poutahidis, 2015; Kwa et al., 2016; Zhao F. et al., 2021), the microbes at the original site of cancer development, the cancer tissue, deserve further exploration. The unclear mechanism of tissue microbiome in distant metastasis and lung cancer stage urgently needs to be investigated.
In this study, 174 samples of patients with recurrence or metastasis (RM) and 134 samples of patients without recurrence or metastasis (non-RM) were collected, and the tissue microbiome of all patients with lung cancer was characterized. The main objectives of this study were (1) to identify the bacterial biomarkers capable of discriminating between RM and non-RM, (2) to investigate the effect of smoking on RM and non-RM differential bacteria, and (3) to correlate bacterial biomarkers with survival and disease stage in lung cancer patients. Our study sheds light on the ability of tissue microbial markers of lung cancer to predict recurrence or metastasis and that these biomarkers are strongly associated with the survival and stage of patients with lung cancer.
Materials and methods
Patient cohorts and data preparation
Rob Knight’s team rechecked the microbial readings from 18,116 cancer tissue samples included 10,481 patients and 33 cancers in The Cancer Genome Atlas1 (TCGA; Poore et al., 2020). Of the 6.4′1012 sequencing readings in TCGA, 7.2% were classified as non-human, of which 35.2% were assigned to bacteria, archaea, or viruses; the sequencing readings were clustered into operational taxonomic units (OTUs) to the genus level by Kraken (Wood and Salzberg, 2014). Microbial sequencing technologies included whole-genome sequencing (WGS) and whole-transcriptome sequencing (RNA-seq). To obtain more tissue samples from lung cancer patients, we downloaded the microbial data obtained by RNA-seq in TCGA database, and obtained clinical indicators and patient information of all samples.
In total, we obtained 308 lung tissue samples from 298 patients with lung cancer. We divided the samples into two groups based on the presence of recurrence or metastasis within 3 years after the initial diagnosis of lung cancer. Specifically, we defined patient samples without recurrence or metastasis within 3 years as non-RM, and defined patient samples with recurrence or metastasis, or both recurrence and metastasis as RM. We obtained 174 RM samples and 134 non-RM samples. We also collected related important clinical indicators of the patients, such as age, gender, TNM stage, smoking history, etc. Specific information for all samples is provided in Table 1.
Network analyses and keystone taxa
We performed network analysis to assess microbiome complexity and identify potential keystone genera for RM and non-RM. We used Spearman’s rank correlation to assess the association among genera. We used the Hmisc package for calculating correlation coefficients and p values. Correlation coefficients greater than 0.7 with a corresponding p-value less than 0.001 were considered statistically significant. Eligible correlations are used to generate the networks. The undirected networks were explored and visualized with the interactive platform Gephi (Bastian et al., 2009), using the Fruchterman-Reingold layout. Some important topological parameters and node scores of the resulting network are obtained through Gephi (Newman, 2006). In our networks, nodes represented the genera, and the edges represented Spearman’s rank correlations. The average degree is the number of edges on each node. Path length and diameter, respectively, represent the nearest distance and the largest distance between two nodes in a network. Clustering coefficient indicates the extent a node is connected to its neighbors. We used high degree to statistically identify the keystone taxa (Banerjee et al., 2019).
Machine-learning classification model and biomarkers identification
We used the microbiome at the genus level as a feature to predict the possibility of recurrence or metastasis of patients in the future. We labeled the patients of RM as “0,” and the patients of non-RM as “1.” Thus, this problem can be considered a binary classification task. We selected Random Forest (RF) to complete our classification task. RF is used for classification purposes and it had a good performance in recent years. This model was implemented by Python’s Sklearn module. We estimated the performance of the classification algorithms using the 5-fold cross-validation (5-fold-cv) procedure. We evaluated the predicted goodness at each abundance level in steps of one hundred. The performance of the classification algorithm was estimated by averaging the area under the curve (AUC) in the 5 test datasets. To ensure comparability, the division of the datasets on each abundance was consistent.
We calculated the variable importance of the top 100 bacteria in relative abundance for identifying RM and non-RM using the Random Forest algorithm. We identified 15 bacterial biomarkers that best discriminated between RM and non-RM based on two variable importance metrics from Random Forest, mean decrease accuracy (MDA) and mean decrease gini (MDGini). Further, Wilcoxon rank-sum test was used to compare the differences of these 15 biomarkers between RM and non-RM. p-value <0.05 was considered statistically significant.
Validation of predictions on survival
From the 15 bacterial biomarkers that discriminate between RM and non-RM, we used the bacteria with the top 6 variables in importance to predict all samples into two groups, recurrence or metastasis (Pred_lable = RM) and without recurrence or metastasis (Pred_lable = non-RM), respectively. Then, overall survival time and status were used to evaluate the prognosis of lung cancer patients in the two groups. The survival curve was performed by using the Kaplan–Meier method and the log-rank test was used to compare the difference in survival probability with R package “survival.” p-value <0.05 was considered statistically significant.
Analysis of tissue microbes in different stages of lung cancer
The TNM staging system was first proposed by the French Pierre Denoix between 1943 and 1952(Asare et al., 2019), and later the American Joint Committee on Cancer (AJCC) and the Union for International Cancer Control (UICC) gradually began to establish an international system. In 1968, the first edition of the ‘TNM Classification of Malignant Tumors’ manual was officially published. It has become the standard method for staging malignant tumors by clinicians and medical scientists.
In the TNM staging system: (1) T refers to the condition of the primary tumor. With the increase in tumor volume and the increase in the extent of adjacent tissue involvement, it is represented by T1 ~ T4 in turn. (2) N refers to the involvement of regional lymph nodes. When the lymph nodes are not involved, it is indicated by N0. With the increase in the degree and scope of lymph node involvement, it is represented by N1 ~ N3 in turn. (3) M refers to distant metastasis (usually blood duct metastasis), M0 is used for those without distant metastasis, and M1 is used for those with distant metastasis. On this basis, use the combination of the three indicators of TNM to draw a specific stage. To investigate changes in bacterial composition at different stages of lung cancer, we compared the relative abundances of 15 bacterial biomarkers capable of distinguishing metastatic and non-metastatic between different stages. Wilcoxon rank-sum test was used to compare the differences between groups. Besides, taking the T stage as an example, we constructed five-fold cross-validation random forest models with features from the combinations of three bacterial biomarkers (Dickeya, Lactococcus, and Pseudogulbenkiania) to validate the performance of bacterial biomarkers in predicting the tumor stage.
Identification of the patient’s smoking history
Based on the smoking history information of patients provided by TCGA, we divided all patients into four groups: smoking history >15 years, smoking history ≤15 years, current smokers, and unknown. Among them, the smoking age of current smoker is not clear, so we focused on comparing the relative abundance of bacterial biomarkers between the two groups of samples with a smoking history of more than 15 years and less than 15 years. Wilcoxon rank-sum test was used to compare the differences between groups.
Statistical analysis
All analyses were implemented with R version 4.1.32 and its appropriate packages. Principal coordinate analysis (PCoA) was performed with R package ‘ape’ based on the Bray-Curtis distance matrix. The Shannon index and Bray–Curtis dissimilarity index were calculated by using the R package “vegan.” Non-metric multidimensional scaling (NMDS) was performed with the R package “vegan.” Comparison between groups was conducted utilizing Wilcoxon rank-sum test. p-value <0.05 was set as the threshold.
Results
Characteristics of the lung cancer datasets in meta-analysis
A total of 308 lung tissue samples from 298 patients with lung cancer were obtained. We determined the recurrence or metastasis of patients based on the follow-up information provided by TCGA. Detailedly, we defined patients without recurrence or metastasis within three years after the initial diagnosis of lung cancer as non-RM samples, and patients with recurrence, metastasis, and simultaneous recurrence and metastasis within 3 years as RM samples. The demographics and clinical characteristics are provided in Table 1.
Bacterial profile of the lung cancer microbiome is dominated by proteobacteria
Previous microbial studies of lung cancer have shown that bacterial composition of cancerous lungs shifts compared to non-cancerous lungs (Huang et al., 2011); however, these compositional changes have not been examined in distant metastatic lung cancer. To obtain a comprehensive characteristic of the bacterial community of metastatic lung cancer, we thoroughly compared the bacterial compositions of RM and non-RM. As shown in Figure 1, Proteobacteria dominated the tissue microbiome of lung cancer with an average relative abundance of 52.3%, followed by Firmicutes (21.8%) and Actinobacteria (16.0%). Importantly, Proteobacteria was generally more dominant in non-RM (Wilcoxon p = 0.041), indicating that this is a recurrent phenomenon in lung cancer (Woerner et al., 2022).
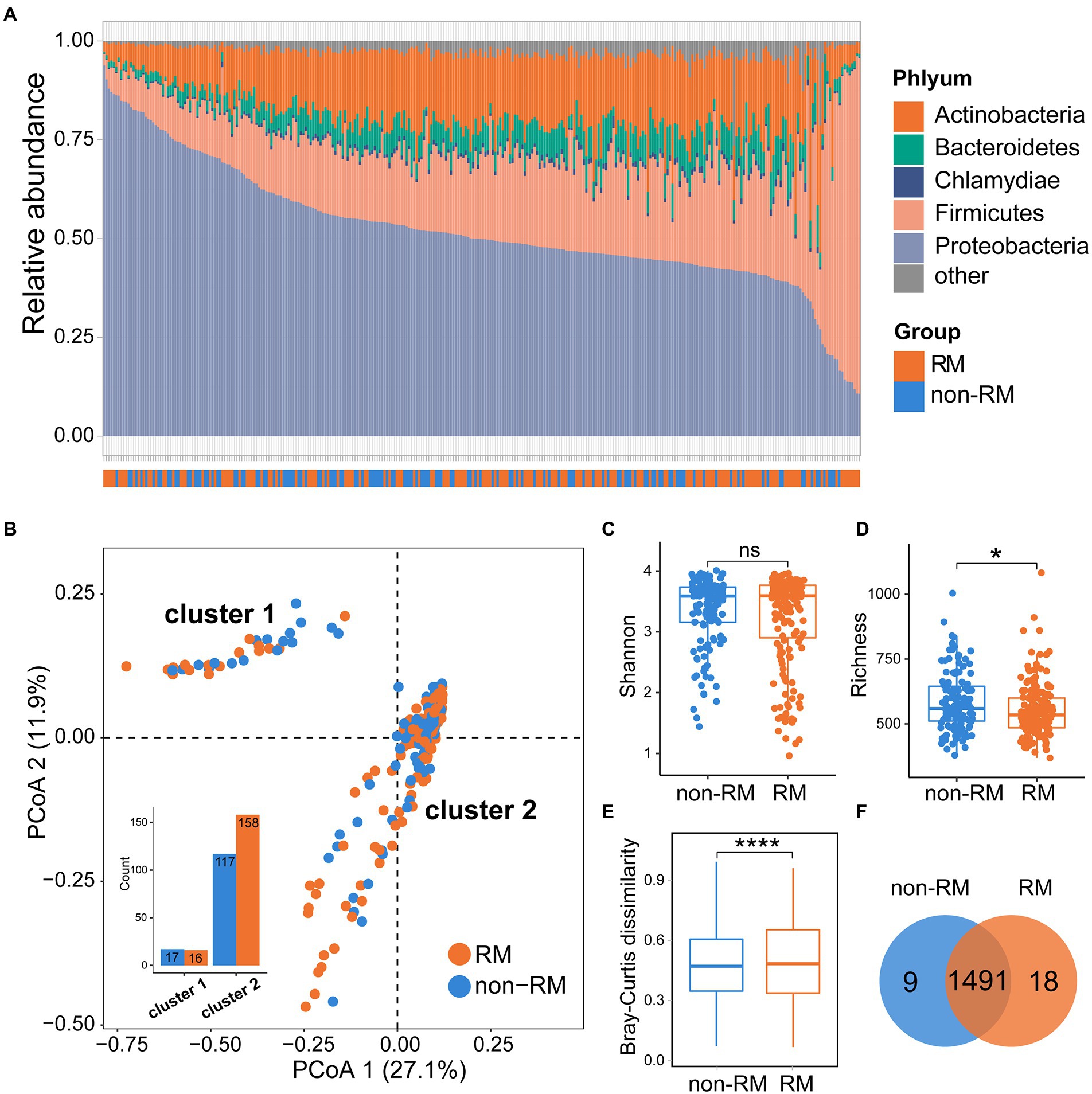
Figure 1. Bacterial community structures of RM and non-RM patients with lung cancer. (A) Bacteria composition at phylum level for all samples; (B) The PCoA plot, on the genus level, colored by group as in panel; Comparisons of (C) Shannon index and (D) richness of bacterial communities between RM and non-RM; (E) Bray–Curtis dissimilarity measures, on the genus level, based for all pairs of samples; (F) Common and unique genera between RM and non-RM. Wilcoxon test, * p < 0.05, **** p < 0.0001.
Bacterial composition carry information on recurrence or metastasis in lung cancer
We next computed the firth two principal coordinates based on the Bray–Curtis dissimilarity and the PCoA plot showed two distinct clusters (Figure 1B). The two groups (RM and non-RM) were not randomly dispersed between the two clusters. Instead, enrichment was observed in specific clusters for certain groups, providing further evidence that the bacterial composition may carry RM/non-RM information in lung cancer. Then, we examined the alpha diversity (Shannon) and richness of the microbiome within samples of RM and non-RM. Specifically, there was no significant difference in the Shannon index between RM and non-RM; however, we observed a significant increase in richness in non-RM as compared to RM tissue (Figures 1C,D). Further, we calculated the Bray–Curtis dissimilarity for each pair of samples to measure how different each pair is regarding bacterial composition. Non-RM samples were far more similar to one another than to the RM samples (Wilcoxon p < 0.0001; Figure 1E). We detected 1,509 and 1,500 genera in non-RM and RM, respectively, indicating that the vast majority of genera were shared in lung cancer tissues regardless of recurrence or metastasis (Figure 1F).
Co-occurrence networks and keystone taxa of RM and non-RM
We know that bacterial composition in the tissues of lung cancer patients with and without metastasis is different; however, the interaction pattern of bacterial communities in lung cancer tissues has not been disclosed. To reveal the underlying patterns, based on genus pairs with significant positive correlations screened by thresholds, we mapped co-occurrence networks for RM and non-RM, respectively (Figures 2A,B). Network complexity varied considerably between the two groups. Specifically, compared to non-RM, microbial communities in RM had a less complex network with fewer edges (6286), fewer nodes (738), a lower average degree (17.04), and a lower average clustering coefficient (0.78, Figure 2C). In the RM, the keystone genera we detected was Azoarcus, while in the non-RM, it was Variovorax, Ramlibacter, and Sphaerotilus. Although limited studies have directly linked these genera to lung cancer metastasis, the difference in keystone certainly implies a divergence in bacterial interactions between RM and non-RM.
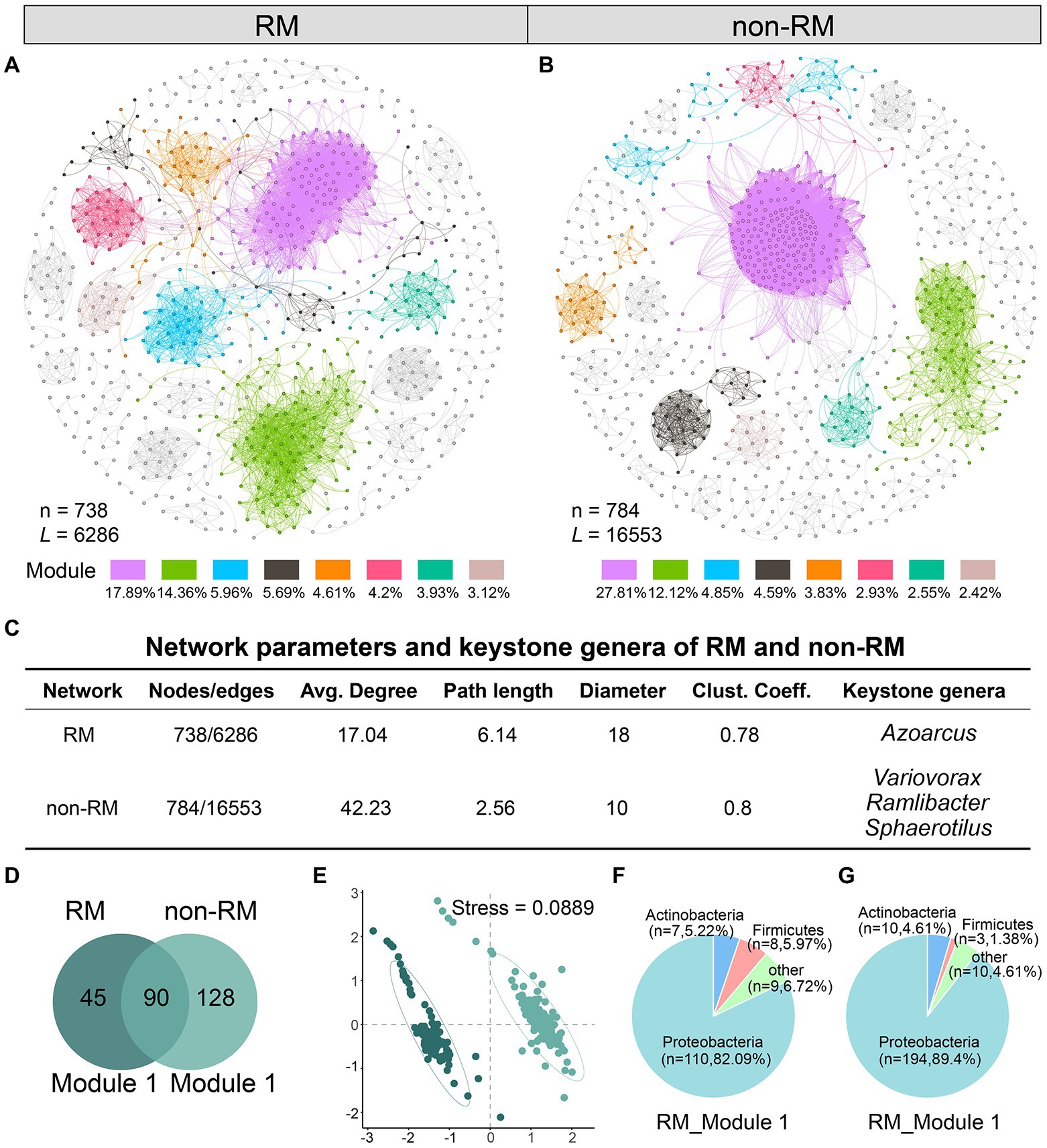
Figure 2. Network analysis reveals distinct bacterial community interaction patterns between RM and non-RM. Network of co-occurring bacteria of (A) RM and (B) non-RM. Only Spearman’s correlation coefficient (r > 0.7 significant at p < 0.001) is shown. The nodes are colored according to module. The percentage indicates the ratio of the number of nodes in the module to the total number; (C) Network parameters and the potential keystone genera of RM and non-RM. Average degree is the number of edges on each node. Path length and diameter, respectively, represent the nearest distance and the largest distance between two nodes in a network. Clustering coefficient indicates the extent a node is connected to its neighbors; (D) Common and unique genera in the largest modules of RM and non-RM; (E) NMDS analysis shows significant differences in the largest modules of RM and non-RM; Phylum-level bacterial composition in the largest modules of (F) RM and (G) non-RM.
Further, we drilled down into the largest module in the network, i.e., with the most nodes, which we called Module 1 in this study. Module1 of RM and non-RM contained 135 and 218 genera, respectively, of which 90 were shared (Figure 2D). NMDS analysis showed that the bacterial composition in Module 1 of the two groups was significantly different (Figure 2E; stress = 0.0889). Detailedly, Proteobacteria were the core phylum in these two modules, accounting for 82.1 and 89.4%, respectively (Figures 2F,G), further indicating that Proteobacteria dominated the lung cancer tissue bacterial community.
Bacterial biomarkers for differentiating RM and non-RM are associated with patient outcomes in lung cancer
Given the observed differences in bacterial content between RM and non-RM (Figures 2A,B,3), we reasoned that bacteria might be able to classify recurrence or metastasis of patients with lung cancer. To this end, we constructed a machine-learning classifier to identify recurrence or metastasis from tissue bacteria. The top 500 genera in relative abundance were chosen as a compromise between reduced resolution with more genera and decreased representation of bacterial community with fewer genera. The average AUC of the classifier using bacterial content reached 0.72 (Figure 3A). The performance of our machine-learning classifier provides evidence that bacterial composition contains a signal that tracks recurrence or metastasis.
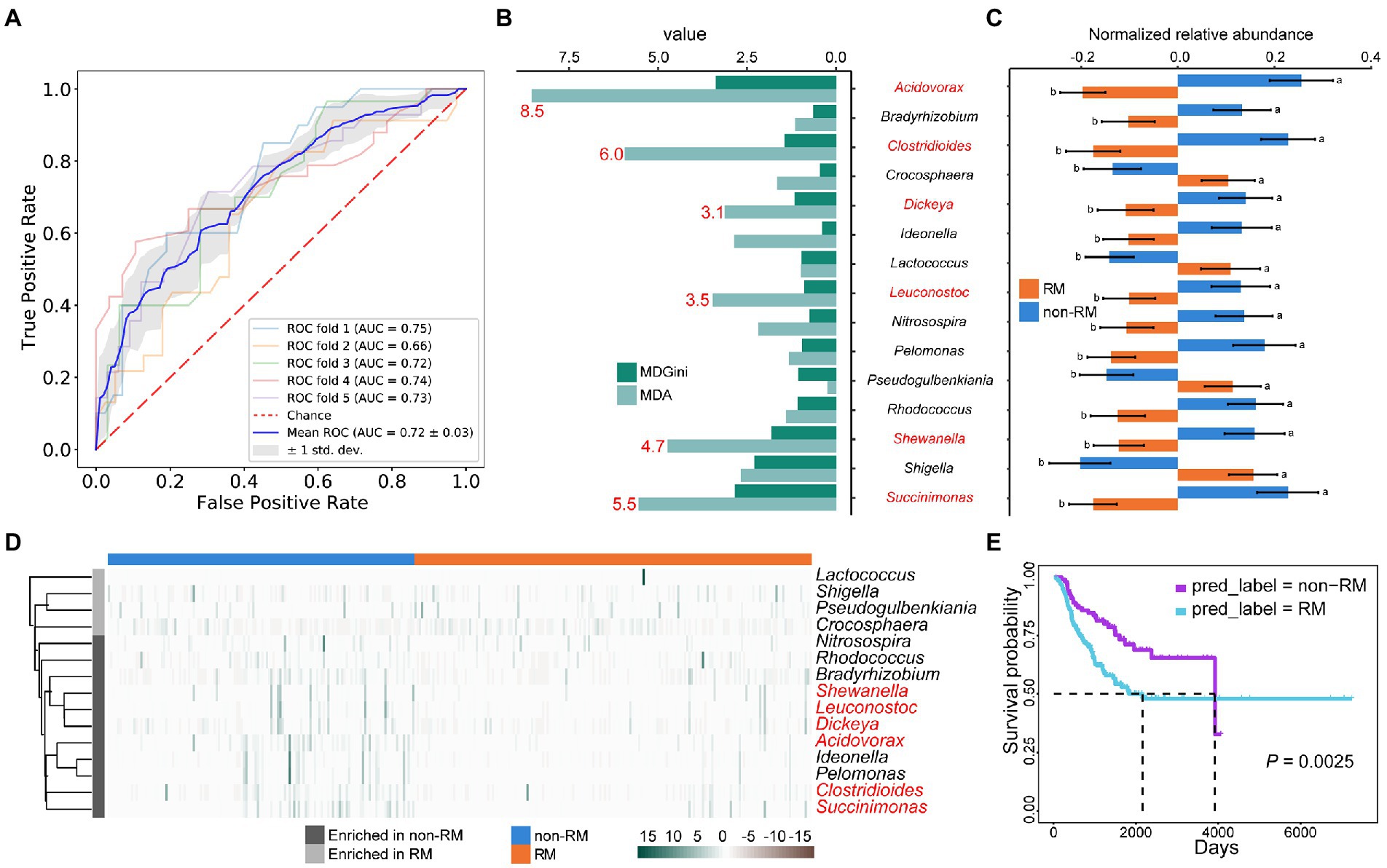
Figure 3. Metastasis and survival in lung cancer patients share some bacterial biomarkers. (A) Fivefold cross-validation random forest models with feature from top 500 genera in relative abundance; (B) The top 15 genera of variable importance predicted by random forest. The top 6 genera of MDA are shown in red; (C) The relative abundances of these 15 genera were significantly different between RM and non-RM; (D) Heatmap shows the enrichment of these 15 genera in RM and non-RM; (E) Kaplan–Meier survival curve shows that non-RM showed a significant overall survival benefit as compared with RM.
In addition to providing an algorithm to assign classes (RM/non-RM in our case) based on features (relative abundance of bacterial genera). Random Forest also assigns variable importance to each categorical feature. Based on the MDA value, we identified the top 15 genera with variable importance and significant differences between RM and non-RM (Figures 3B,C). Acidovorax has been reported to develop as a panel of sputum biomarkers that could diagnose lung squamous cell carcinoma (Leng et al., 2021). It is currently known that patients with lung cancer are at high risk of developing Clostridium difficile infection (CDI) due to continued chemotherapy, prolonged hospital stay, and general debility (Hwang et al., 2013). However, we detected a reduced relative abundance of Clostridium in recurrence or metastasis lung cancer patients, suggesting that its mechanism in recurrence or metastasis remains to be elucidated. Many other genera that contribute to discrimination between RM and non-RM (Figure 3D) are known to be associated with lung disease or lung cancer chemotherapy outcomes, e.g., Leuconostoc (Zhao Z. et al., 2021), Shigella (Zhang et al., 2018), Rhodococcus (Haramati and Jenny-Avital, 1998), and Bradyrhizobium (Jin et al., 2019).
Given the significance of predicting the prognosis of lung cancer patients and the relationship between bacteria and lung cancer patient survival demonstrated by multiple studies (Salazar et al., 2020; Tomita et al., 2020; Zhao Y. et al., 2021), we tried to correlate these bacterial biomarkers with patient survival. First, we selected the top 6 genera of variable importance as biomarkers (Figure 3D), then used these biomarkers to predict the recurrence or metastasis of all lung cancer patients, and finally performed survival analysis on the predicted two groups. As shown in Figure 3E, non-RM showed a significant overall survival benefit as compared with RM (p = 0.0025). Our results further prove the accuracy and clinical significance of the bacterial biomarkers we identified, as well as the fact that the patients with lung cancer recurrence or metastasis have reduced survival.
Smoking history influences bacteria that distinguish recurrence or metastasis in lung cancer patients
Smoking is the greatest risk factor for lung cancer, up to 90% of lung cancers can be attributable to smoking (de Groot et al., 2018). Previous studies have demonstrated that nicotine-induced N2-neutrophils have a pro-metastatic role in lung cancer cell colonization (Tyagi et al., 2021). However, whether bacteria are mediators linking smoking and lung cancer recurrence or metastasis is still unknown.
Thus, we associated smoking history with recurrence or metastasis-related bacterial biomarkers (Figure 3B) in lung cancer patients. Coincidentally, we found that the relative abundance of most bacterial biomarkers was reduced in patients with a longer smoking history (Figure 4A). The relative abundances of the genera Acidovorax, Clostridioides, and Lactococcus varied with smoking history (Figures 4B–D). In particular, the relative abundance of the genus Acidovorax was significantly higher in patients with a smoking history of less than 15 years than in patients with a smoking history of more than 15 years (Figure 4B). Similarly, we also detected a reduced relative abundance of Acidovorax in RM compared to non-RM (Figure 3C). Naturally, we speculate that excessive smoking can cause changes in the content of certain bacteria, which, in turn, promotes the recurrence or metastasis of lung cancer patients.
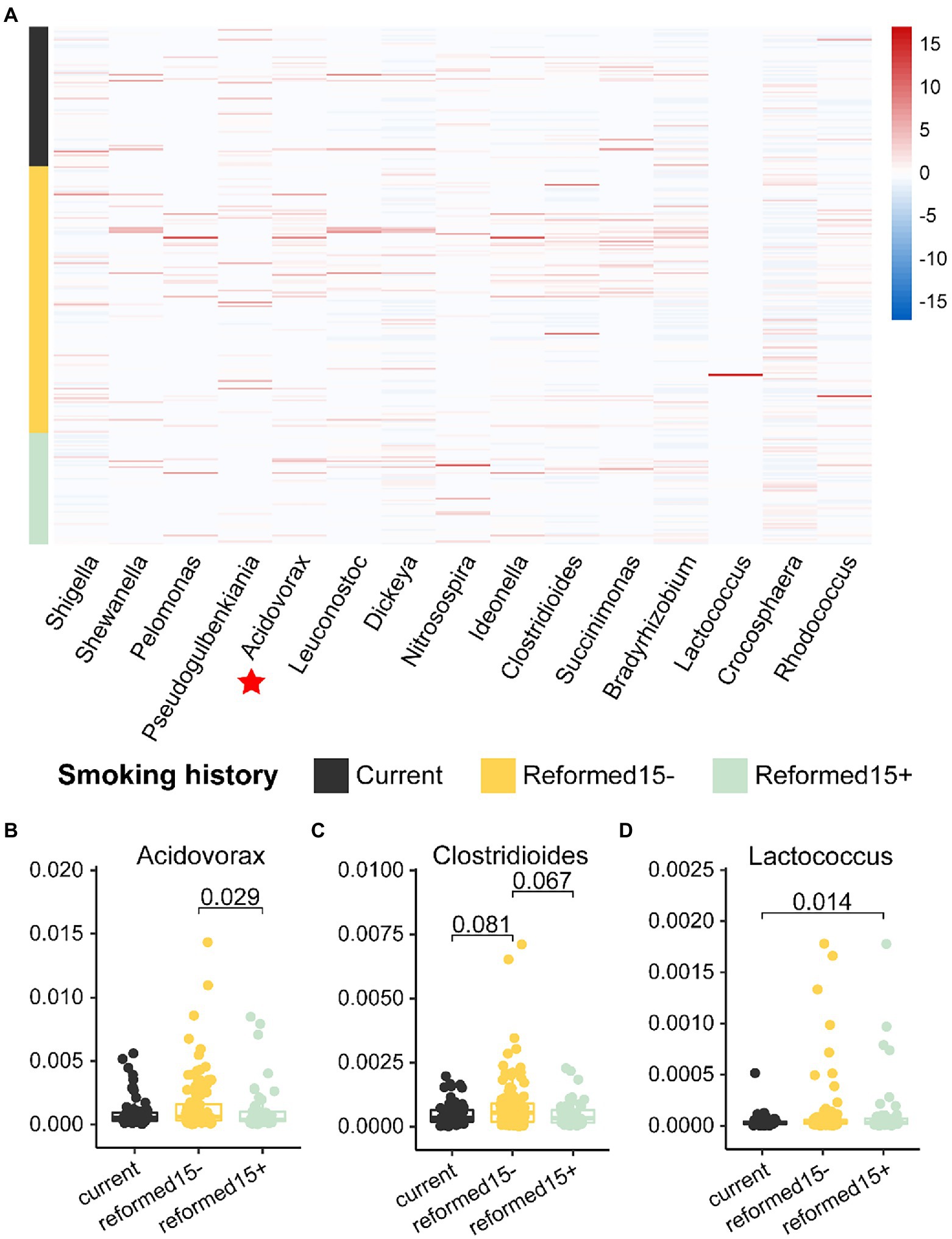
Figure 4. Relative abundances of bacterial biomarkers vary in patients with different smoking histories. (A) Heatmap shows the relative abundance of 15 bacterial biomarkers in patients with different smoking histories. The relative abundances of (B) Acidovorax, (C) Clostridioides, and (D) Lactococcus are significantly different among patients with different smoking histories.
Bacterial biomarkers of disease stage and lung cancer recurrence or metastasis intersect
It has long been recognized that regional and metastatic cancers have a worse prognosis, and many cancers can be traced back to this gradual progression (Cserni et al., 2018). This has become the basis for cancer staging, including lung cancer. The Tumor-Node-Metastasis (TNM) system established by the Union for International Cancer Control (UICC) has become a worldwide means of describing the anatomical extent of cancer and determining its stage.
We have known that bacterial biomarkers that can distinguish recurrence or metastasis of lung cancer are related to patient survival (Figure 3E), and then we wondered whether these biomarkers also carry disease stage information. Interestingly, we found that the relative abundances of some of these 15 bacterial markers (Figure 2B) varied significantly between stages (Figures 5A–I). For example, the relative abundance of Dickeya was significantly lower in T4 patients compared to T2 patients (Figure 5A). Rhodococcus has the lowest relative abundance in N3 stage patients compared to other stages (Figure 5F). Then, taking the T stage as an example, we constructed five-fold cross-validation Random Forest models with features from these three biomarkers (Figures 5A–C). As expected, features from the combination of these three bacteria showed capabilities for identifying the T stage for patients with lung cancer (Figure 5J). The genera Dickeya, Lactococcus, and Pseudogulbenkiania displayed the strongest ability to identify the T stage with an average AUC of 0.84.
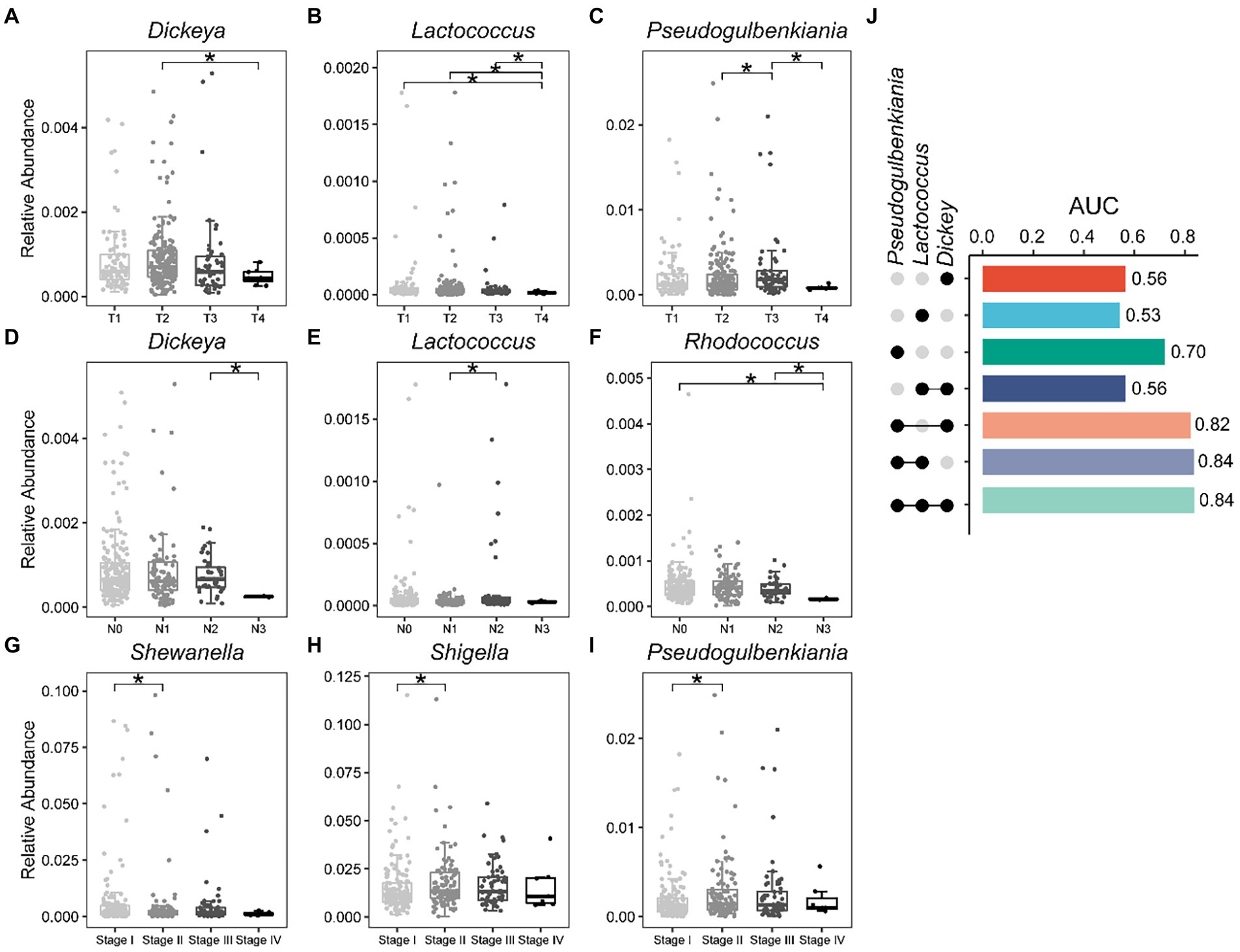
Figure 5. Bacterial biomarkers can identify disease stage in lung cancer patients. Relative abundance of specific genera varies significantly between different stages of lung cancer. (A–C) T stage; (D–F) N stage; (G–I) Stage; (J) Fivefold cross-validation random forest models with features from Dickeya, Lactococcus, and Pseudogulbenkiania to predict the T stage of lung cancer patients. Wilcoxon test, *p < 0.05.
Wu and his colleagues found that mannan exopolysaccharides (EPS) produced by a subsp. of Lactococcus lactis affected the production of inflammatory cytokine (Wu et al., 2016). Similarly, we also detected a decrease in the relative abundance of Lactococcus in advanced patients (Figure 5B). In general, our results demonstrate that bacteria capable of discriminating recurrence or metastasis from lung cancer also carry disease stage information, thereby assisting clinicians and medical scientists in staging malignancies.
Discussion
Genetic and environmental factors have long been recognized as contributors to cancer recurrence or metastasis (Bhujwalla et al., 2001; Rosell and Karachaliou, 2015; Song et al., 2020). Recently, histopathological images are also been found capable of predicting cancer recurrence or metastasis (Yang J. et al., 2022; Ye et al., 2022); however, little is known about the tissue microbiome that promotes cancer recurrence or metastasis. We demonstrate that recurrence or metastasis in lung cancer patients is associated with specific bacteria and that smoking significantly affects the relative abundance of these bacteria. In-depth, by building machine-learning classifiers, we found that six recurrence- or metastasis-distinguishing bacterial biomarkers (Acidovorax, Clostridioides, Succinimonas, Shewanella, Leuconostoc, and Dickeya) were associated with survival in lung cancer patients. Further, we verified that bacteria capable of discriminating recurrence or metastasis also carry information on tumor stage in lung cancer, and three genera, Dickeya, Lactococcus, and Pseudogulbenkiania, can accurately predict tumor T stage. Collectively, the above results support our proposal that smoking can lead to changes in the bacterial community in lung cancer tissue, which, in turn, affects tumor metastasis in patients, and the bacteria closely associated with recurrence or metastasis are inseparable from patient prognosis and tumor stage.
The number one risk factor for lung cancer development is tobacco exposure, which outweighs all other factors that lead to lung cancer (Bade and Dela Cruz, 2020). Tobacco smoke contains many potential carcinogens and bacterial products, which can induce epithelial cells to secrete inflammatory cytokines, cause barrier function impairment, and even alter the microbiome to influence lung carcinogenesis (Sapkota et al., 2010; Pauly and Paszkiewicz, 2011; Heijink et al., 2012; Checa et al., 2016). We observed significantly lower relative abundances of certain bacteria, such as Acidovorax, in patients with a smoking history of more than 15 years compared with patients with a smoking history of less than 15 years. Similarly, in a study of tumor (n = 143) and non-tumor adjacent tissues (n = 144), they observed a significant difference in the relative abundance of Acidovorax among smokers as compared to non-smokers (Greathouse et al., 2018). In addition, a study of non-malignant lung tissue showed that a greater abundance of Acidovorax was specifically found in the extracellular vesicles of smokers (Kim et al., 2017). Innovatively, we are the first to suggest that the relative abundance of Acidovorax is reduced in recurrence or metastasis patients compared to without recurrence or metastasis patients, echoing the reduced relative abundance of Acidovorax in patients with a longer smoking history. Nevertheless, future studies should mechanistically elucidate the role of Acidovorax between tobacco exposure and lung cancer metastasis.
Lung cancer patients face severe mortality even when detected in the early stages of cancer. Different from other types of cancers that are detected early and have obvious survival advantages, about 35–45% of patients with stage I lung cancer will die due to recurrence within 5 years even if the operation is successful (Molina et al., 2008; Zhao et al., 2017). We have verified that lung cancer patients with recurrence or metastasis are associated with lower survival rates. Further, our machine-learning classifier with features of Acidovorax, Clostridioides, Succinimonas, Shewanella, Leuconostoc, and Dickeya predicted recurrence or metastasis information in lung cancer patients, and patients predicted to be recurrence or metastasis had lower survival rates. Although the mechanism remains to be elucidated by more evidence, the current findings undoubtedly provide guidance for clinicians to preliminarily judge patient survival.
Our study showed that bacteria capable of distinguishing recurrence or metastasis can predict tumor stage in patients. In a study of 156 incident lung cancer cases and 156 individually matched controls, they found that species Lactococcus lactis was associated with decreased lung cancer risk (Shi et al., 2021). We also detected a decrease in the relative abundance of Lactococcus from the T1–T4 stages. Dickeya, Lactococcus, and Pseudogulbenkiania outperformed in predicting T4 and T1 stages in lung cancer patients.
The strength of our findings includes two accurately divided lung cancer cohorts with and without recurrence or metastasis within 3 years, the microbiome at the site of initial cancer development, and detailed follow-up information for nearly all patients. But our research still has some limitations. The distribution of samples in different stages is not uniform; for example, the number of samples in the T4 stage is much smaller than that in the T2 stage, we admit that this may skew the results. Although we comprehensively compared the tissue microbiome of patients without and those with recurrence or metastasis, the absence of healthy controls is a pity. Functional experiments are needed in the future to determine if and how bacteria influence the progression of lung cancer. Such experiments will reveal the potential of bacteria as biomarkers in lung cancer recurrence or metastasis and may provide treatment options for patients. Functional experiments to further provide treatment assistance for lung cancer patients is the focus of our future work.
Conclusion
Through a comprehensive comparison of tissue microbes in recurrence or metastasis and without recurrence or metastasis lung cancer patients, we identified 15 bacterial biomarkers that differentiate between RM and non-RM lung cancer, with the relative abundance of most bacteria decreasing in recurrence or metastasis patients. Besides, six recurrence or metastasis-distinguishing bacterial biomarkers (Acidovorax, Clostridioides, Succinimonas, Shewanella, Leuconostoc, and Dickeya) were associated with survival in lung cancer patients. Further, we found that patients with longer smoking history were associated with lower abundances of these biomarkers, such as the genus Acidovorax. Finally, these bacterial biomarkers (Dickeya, Lactococcus, and Pseudogulbenkiania) accurately predicted the tumor T stage in lung cancer patients. We propose that smoking induces tissue microbial changes in lung cancer patients, which, in turn, promotes recurrence or metastasis in lung cancer patients, and the altered bacteria are associated with patient prognosis and tumor progression. With these results, we foresee a new avenue for mechanistic studies to address the role of microbes in the recurrence or metastasis of lung cancer patients, patient prognosis, and tissue tumor progression monitoring.
Data availability statement
The original contributions presented in the study are included in the article/supplementary material, further inquiries can be directed to the corresponding authors.
Ethics statement
Written informed consent was obtained from the individual(s) for the publication of any potentially identifiable images or data included in this article.
Author contributions
JY, LJ, and CL conceived this study. XY and ZW performed the study and experiments. KL, GT, and MT collected the data. XY and LJ wrote the manuscript. All authors contributed to the interpretation of data and to the revision of the manuscript.
Conflict of interest
GT, LJ, and JY were employed by Geneis Beijing Co., Ltd.
The remaining authors declare that the research was conducted in the absence of any commercial or financial relationships that could be construed as a potential conflict of interest.
Publisher’s note
All claims expressed in this article are solely those of the authors and do not necessarily represent those of their affiliated organizations, or those of the publisher, the editors and the reviewers. Any product that may be evaluated in this article, or claim that may be made by its manufacturer, is not guaranteed or endorsed by the publisher.
Footnotes
References
Asare, E. A., Grubbs, E. G., Gershenwald, J. E., Greene, F. L., and Aloia, T. A. (2019). Setting the “stage” for surgical oncology fellows: Pierre Denoix and TNM staging. J. Surg. Oncol. 119:823. doi: 10.1002/jso.25404
Bade, B. C., and Dela Cruz, C. S. (2020). Lung cancer 2020: epidemiology, etiology, and prevention. Clin. Chest Med. 41, 1–24. doi: 10.1016/j.ccm.2019.10.001
Banerjee, S., Walder, F., Büchi, L., Meyer, M., Held, A. Y., Gattinger, A., et al. (2019). Agricultural intensification reduces microbial network complexity and the abundance of keystone taxa in roots. ISME J. 13, 1722–1736. doi: 10.1038/s41396-019-0383-2
Bastian, M., Heymann, S., and Jacomy, M. (2009). “Gephi: an open source software for exploring and manipulating networks.” in Proceedings of the International AAAI Conference on Web and Social Media, San Jose, CA, 361–362.
Bertocchi, A., Carloni, S., Ravenda, P. S., Bertalot, G., Spadoni, I., Lo Cascio, A., et al. (2021). Gut vascular barrier impairment leads to intestinal bacteria dissemination and colorectal cancer metastasis to liver. Cancer Cell 39, 708.e11–724.e11. doi: 10.1016/j.ccell.2021.03.004
Bhujwalla, Z. M., Artemov, D., Aboagye, E., Ackerstaff, E., Gillies, R. J., Natarajan, K., et al. (2001). The physiological environment in cancer vascularization, invasion and metastasis. Novartis Found. Symp. 240:23–38; discussion 38–45, 152–153. doi: 10.1002/0470868716.ch3
Boleij, A., Hechenbleikner, E. M., Goodwin, A. C., Badani, R., Stein, E. M., Lazarev, M. G., et al. (2015). The Bacteroides fragilis toxin gene is prevalent in the colon mucosa of colorectal cancer patients. Clin. Infect. Dis. 60, 208–215. doi: 10.1093/cid/ciu787
Bullman, S., Pedamallu, C. S., Sicinska, E., Clancy, T. E., Zhang, X., Cai, D., et al. (2017). Analysis of Fusobacterium persistence and antibiotic response in colorectal cancer. Science 358, 1443–1448. doi: 10.1126/science.aal5240
Checa, M., Hagood, J. S., Velazquez-Cruz, R., Ruiz, V., Garcia-De-Alba, C., Rangel-Escareno, C., et al. (2016). Cigarette smoke enhances the expression of Profibrotic molecules in alveolar epithelial cells. PLoS One 11:e0150383. doi: 10.1371/journal.pone.0150383
Cheng, W. T., Kantilal, H. K., and Davamani, F. (2020). The mechanism of Bacteroides fragilis toxin contributes to colon cancer formation. Malays J. Med. Sci. 27, 9–21. doi: 10.21315/mjms2020.27.4.2
Cserni, G., Chmielik, E., Cserni, B., and Tot, T. (2018). The new TNM-based staging of breast cancer. Virchows Arch. 472, 697–703. doi: 10.1007/s00428-018-2301-9
Cullin, N., Azevedo Antunes, C., Straussman, R., Stein-Thoeringer, C. K., and Elinav, E. (2021). Microbiome and cancer. Cancer Cell 39, 1317–1341. doi: 10.1016/j.ccell.2021.08.006
de Groot, P. M., Wu, C. C., Carter, B. W., and Munden, R. F. (2018). The epidemiology of lung cancer. Transl. Lung. Cancer Res. 7, 220–233. doi: 10.21037/tlcr.2018.05.06
Erdman, S. E., and Poutahidis, T. (2015). Gut bacteria and cancer. Biochim. Biophys. Acta 1856, 86–90. doi: 10.1016/j.bbcan.2015.05.007
Greathouse, K. L., White, J. R., Vargas, A. J., Bliskovsky, V. V., Beck, J. A., von Muhlinen, N., et al. (2018). Interaction between the microbiome and TP53 in human lung cancer. Genome Biol. 19:123. doi: 10.1186/s13059-018-1501-6
Haramati, L. B., and Jenny-Avital, E. R. (1998). Approach to the diagnosis of pulmonary disease in patients infected with the human immunodeficiency virus. J. Thorac. Imaging 13, 247–260. doi: 10.1097/00005382-199810000-00005
Heijink, I. H., Brandenburg, S. M., Postma, D. S., and van Oosterhout, A. J. (2012). Cigarette smoke impairs airway epithelial barrier function and cell-cell contact recovery. Eur. Respir. J. 39, 419–428. doi: 10.1183/09031936.00193810
Herbst, R. S., Morgensztern, D., and Boshoff, C. (2018). The biology and management of non-small cell lung cancer. Nature 553, 446–454. doi: 10.1038/nature25183
Huang, Y. J., Nelson, C. E., Brodie, E. L., DeSantis, T. Z., Baek, M. S., Liu, J. N., et al. (2011). Airway microbiota and bronchial hyperresponsiveness in patients with suboptimally controlled asthma. J. Allergy Clin. Immun. 127, 372.e3–381.e3. doi: 10.1016/j.jaci.2010.10.048
Hwang, K. E., Hwang, Y. R., Seol, C. H., Chul-Park, P. S. H., Yoon, K. H., Park, D. S., et al. (2013). Clostridium difficile infection in lung cancer patients. Jpn. J. Infect. Dis. 66, 379–382. doi: 10.7883/yoken.66.379
Jin, J., Gan, Y. C., Liu, H. Y., Wang, Z. R., Yuan, J. Y., Deng, T. B., et al. (2019). Diminishing microbiome richness and distinction in the lower respiratory tract of lung cancer patients: a multiple comparative study design with independent validation. Lung Cancer 136, 129–135. doi: 10.1016/j.lungcan.2019.08.022
Kim, H. J., Kim, Y. S., Kim, K. H., Choi, J. P., Kim, Y. K., Yun, S., et al. (2017). The microbiome of the lung and its extracellular vesicles in nonsmokers, healthy smokers and COPD patients. Exp. Mol. Med. 49:e316. doi: 10.1038/emm.2017.7
Kwa, M., Plottel, C. S., Blaser, M. J., and Adams, S. (2016). The intestinal microbiome and estrogen receptor-positive female breast cancer. J. Natl. Cancer Inst. 108:djw029. doi: 10.1093/jnci/djw029
Leng, Q. X., Holden, V. K., Deepak, J., Todd, N. W., and Jiang, F. (2021). Microbiota biomarkers for lung cancer. Diagnostics 11:407. doi: 10.3390/diagnostics11030407
Liu, H., Qiu, C., Wang, B., Bing, P., Tian, G., Zhang, X., et al. (2021). Evaluating DNA methylation, gene expression, somatic mutation, and their combinations in inferring tumor tissue-of-origin. Front. Cell Develop. Biol. 9:619330. doi: 10.3389/fcell.2021.619330
Lu, H., Gao, N. L., Tong, F., Wang, J. J., Li, H. H., Zhang, R. G., et al. (2021). Alterations of the human lung and gut microbiomes in non-small cell lung carcinomas and distant metastasis. Microbiol. Spectr. 9:e0080221. doi: 10.1128/Spectrum.00802-21
Lu, Y., Zheng, Y. S., Wang, Y. H., Gu, D. M., Zhang, J., Liu, F., et al. (2021). FlowCell-enriched circulating tumor cells as a predictor of lung cancer metastasis. Hum. Cell 34, 945–951. doi: 10.1007/s13577-021-00500-8
Molina, J. R., Yang, P. G., Cassivi, S. D., Schild, S. E., and Adjei, A. A. (2008). Non-small cell lung cancer: epidemiology, risk factors, treatment, and survivorship. Mayo Clin. Proc. 83, 584–594. doi: 10.1016/S0025-6196(11)60735-0
Nasim, F., Sabath, B. F., and Eapen, G. A. (2019). Lung cancer. Med. Clin. N. Am. 103:463. doi: 10.1016/j.mcna.2018.12.006
Newman, M. E. (2006). Modularity and community structure in networks. Proc. Natl. Acad. Sci. 103, 8577–8582. doi: 10.1073/pnas.0601602103
Pauly, J. L., and Paszkiewicz, G. (2011). Cigarette smoke, bacteria, mold, microbial toxins, and chronic lung inflammation. J. Oncol. 2011:819129. doi: 10.1155/2011/819129
Poore, G. D., Kopylova, E., Zhu, Q., Carpenter, C., Fraraccio, S., Wandro, S., et al. (2020). Microbiome analyses of blood and tissues suggest cancer diagnostic approach. Nature 579, 567–574. doi: 10.1038/s41586-020-2095-1
Rosell, R., and Karachaliou, N. (2015). Relationship between gene mutation and lung cancer metastasis. Cancer Metast Rev. 34, 243–248. doi: 10.1007/s10555-015-9557-1
Salazar, Y., Zheng, X., Brunn, D., Raifer, H., Picard, F., Zhang, Y. J., et al. (2020). Microenvironmental Th9 and Th17 lymphocytes induce metastatic spreading in lung cancer. J. Clin. Invest. 130, 3560–3575. doi: 10.1172/JCI124037
Sapkota, A. R., Berger, S., and Vogel, T. M. (2010). Human pathogens abundant in the bacterial metagenome of cigarettes. Environ. Health Perspect. 118, 351–356. doi: 10.1289/ehp.0901201
Seyfried, T. N., and Huysentruyt, L. C. (2013). On the origin of cancer metastasis. Crit. Rev. Oncog. 18, 43–73. doi: 10.1615/CritRevOncog.v18.i1-2.40
Shi, J. J., Yang, Y. H., Xie, H., Wang, X. F., Wu, J., Long, J. R., et al. (2021). Association of oral microbiota with lung cancer risk in a low-income population in the southeastern USA. Cancer Cause Control 32, 1423–1432. doi: 10.1007/s10552-021-01490-6
Song, Z., Chen, X., Shi, Y., Huang, R., Wang, W., Zhu, K., et al. (2020). Evaluating the potential of T cell receptor repertoires in predicting the prognosis of resectable non-small cell lung cancers. Mol. Ther. Methods Clin. Dev. 18, 73–83. doi: 10.1016/j.omtm.2020.05.020
Tomita, Y., Ikeda, T., Sakata, S., Saruwatari, K., Sato, R., Iyama, S., et al. (2020). Association of Probiotic Clostridium butyricum therapy with survival and response to immune checkpoint blockade in patients with lung cancer. Cancer Immunol. Res. 8, 1236–1242. doi: 10.1158/2326-6066.CIR-20-0051
Tyagi, A., Sharma, S., Wu, K., Wu, S. Y., Xing, F., Liu, Y., et al. (2021). Nicotine promotes breast cancer metastasis by stimulating N2 neutrophils and generating pre-metastatic niche in lung. Nat. Commun. 12:474. doi: 10.1038/s41467-020-20733-9
Woerner, J., Huang, Y. D., Hutter, S., Gurnari, C., Sanchez, J. M. H., Wang, J., et al. (2022). Circulating microbial content in myeloid malignancy patients is associated with disease subtypes and patient outcomes. Nat. Commun. 13:1038. doi: 10.1038/s41467-022-28678-x
Wood, D. E., and Salzberg, S. L. (2014). Kraken: ultrafast metagenomic sequence classification using exact alignments. Genome Biol. 15:R46. doi: 10.1186/gb-2014-15-3-r46
Wu, Z., Wang, G., Pan, D., Guo, Y., Zeng, X., Sun, Y., et al. (2016). Inflammation-related pro-apoptotic activity of exopolysaccharides isolated from Lactococcus lactis subsp lactis. Benef Microbes 7, 761–768. doi: 10.3920/BM2015.0192
Yang, J., Hui, Y., Zhang, Y., Zhang, M., Ji, B., Tian, G., et al. (2021). Application of circulating tumor DNA as a biomarker for non-small cell lung cancer. Front. Oncol. 11:725938. doi: 10.3389/fonc.2021.725938
Yang, J., Ju, J., Guo, L., Ji, B., Shi, S., Yang, Z., et al. (2022). Prediction of HER2-positive breast cancer recurrence and metastasis risk from histopathological images and clinical information via multimodal deep learning. Comput. Struct. Biotechnol. J. 20, 333–342. doi: 10.1016/j.csbj.2021.12.028
Yang, M., Yang, H., Ji, L., Hu, X., Tian, G., Wang, B., et al. (2022). A multi-omics machine learning framework in predicting the survival of colorectal cancer patients. Comput. Biol. Med. 146:105516. doi: 10.1016/j.compbiomed.2022.105516
Ye, Z., Zhang, Y., Liang, Y., Lang, J., Zhang, X., Zang, G., et al. (2022). Cervical cancer metastasis and recurrence risk prediction based on deep convolutional neural network. Curr. Bioinforma. 17, 164–173. doi: 10.2174/1574893616666210708143556
Zhang, W. Q., Zhao, S. K., Luo, J. W., Dong, X. P., Hao, Y. T., Li, H., et al. (2018). Alterations of fecal bacterial communities in patients with lung cancer. Am. J. Transl. Res. 10, 3171–3185.
Zhao, F., An, R., Wang, L. Q., Shan, J. K., and Wang, X. J. (2021). Specific gut microbiome and serum Metabolome changes in lung cancer patients. Front. Cell Infect. Microbiol. 11:725284. doi: 10.3389/fcimb.2021.725284
Zhao, Z., Fei, K. L., Bai, H., Wang, Z. J., Duan, J. C., and Wang, J. (2021). Metagenome association study of the gut microbiome revealed biomarkers linked to chemotherapy outcomes in locally advanced and advanced lung cancer. Thorac. Cancer 12, 66–78. doi: 10.1111/1759-7714.13711
Zhao, Y., Liu, Y. X., Li, S., Peng, Z. Y., Liu, X. T., Chen, J., et al. (2021). Role of lung and gut microbiota on lung cancer pathogenesis. J. Cancer Res. Clin. 147, 2177–2186. doi: 10.1007/s00432-021-03644-0
Zhao, J., Zhang, X. L., Gong, C. J., and Zhang, J. L. (2017). Targeted therapy with apatinib in a patient with relapsed small cell lung cancer a case report and literature review. Medicine 96:e9259. doi: 10.1097/MD.0000000000009259
Keywords: lung cancer, recurrence or metastasis, bacterial community, random forest, survival
Citation: Yuan X, Wang Z, Li C, Lv K, Tian G, Tang M, Ji L and Yang J (2022) Bacterial biomarkers capable of identifying recurrence or metastasis carry disease severity information for lung cancer. Front. Microbiol. 13:1007831. doi: 10.3389/fmicb.2022.1007831
Edited by:
Fei Ma, Chinese Academy of Medical Sciences and Peking Union Medical College, ChinaReviewed by:
Min Chen, Hunan Institute of Technology, ChinaCangzhi Jia, Dalian Maritime University, China
Copyright © 2022 Yuan, Wang, Li, Lv, Tian, Tang, Ji and Yang. This is an open-access article distributed under the terms of the Creative Commons Attribution License (CC BY). The use, distribution or reproduction in other forums is permitted, provided the original author(s) and the copyright owner(s) are credited and that the original publication in this journal is cited, in accordance with accepted academic practice. No use, distribution or reproduction is permitted which does not comply with these terms.
*Correspondence: Changjun Li, licj@ouc.edu.cn; Lei Ji, jilei123@hust.edu.cn; Jialiang Yang, yangjl@geneis.cn
†These authors have contributed equally to this work