- 1State Key Laboratory of Bio-Control, School of Life Sciences, Southern Marine Science and Engineering Guangdong Laboratory (Zhuhai), Sun Yat-sen University, Guangzhou, China
- 2Laboratory for Marine Fisheries Science and Food Production Processes, Qingdao National Laboratory for Marine Science and Technology, Qingdao, China
Bacterial metabolism is related to resistance and susceptibility to antibiotics. Fumarate and nitrate reduction regulatory protein (FNR) is a global transcriptional regulator that regulates metabolism. However, the role of FNR in antibiotic resistance is elusive. Here, fnr deletion mutant was constructed and used to test the role in Edwardsiella tarda EIB202 (EIB202). Δfnr exhibited elevated sensitivity to aminoglycosides. The mutant had a globally enhanced metabolome, with activated alanine, aspartate, and glutamate metabolism and increased abundance of glutamic acid as the most impacted pathway and crucial biomarker, respectively. Glutamate provides a source for the pyruvate cycle (the P cycle) and thereby relationship between exogenous glutamate-activated P cycle and gentamicin-mediated killing was investigated. The activated P cycle elevated proton motive force (PMF). Consistently, exogenous glutamate potentiated gentamicin-mediated killing to EIB202 as the similarity as the loss of FNR did. These findings reveal a previously unknown regulation by which FNR downregulates glutamate and in turn inactivates the P cycle, which inhibits PMF and thereby exhibits the resistance to aminoglycosides.
Introduction
Bacterial antimicrobial resistance (AMR) poses a major threat to human and animal health around the world (Evangelista et al., 2022; Murray et al., 2022). It has been revealed that four mechanisms play a role in the resistance, including reduction of membrane permeability, activation of enzymatic degradation, elevation of efflux pumping, and down-regulation of drug-binding target (Sun et al., 2014). Clarification of these resistance mechanisms improves understanding of bacterial antibiotic resistance, but antibiotic-resistant pathogens are still out of control. Therefore, a further understanding for the mechanisms of antibiotic resistance is needed.
Recent studies have shown that bacterial metabolism is related to susceptibility and resistance to antibiotics (Lee and Collins, 2011; Peng et al., 2015a; Ye et al., 2018a; Zhang et al., 2019; Li et al., 2020), where the pyruvate cycle (the P cycle) plays a role (Su et al., 2018; Zhang et al., 2019; Li et al., 2020). The P cycle is a recently clarified metabolic pathway, which rather than the TCA cycle provides respiratory energy in bacteria (Su et al., 2018). Inactivation of the P cycle is related to antibiotic resistance (Yin et al., 2022). This is because the inactivation affects the redox state and purine biosynthesis, leading to reduction of antibiotic uptake (Ye et al., 2018b; Zhang et al., 2019; Zhao et al., 2021). On the contrary, bacteria become sensitive to antibiotics when the P cycle is activated (Li et al., 2020), especially in response to aminoglycosides antibiotics (Zhang et al., 2019). Therefore, exogenous metabolites that promote the P cycle such as alanine, glucose, and fructose can potentiate the antibiotic-mediated killing (Su et al., 2015, 2018; Peng et al., 2015b). This is because the activated P cycle elevates proton motive force, which is required for aminoglycosides uptake.
Transcriptional regulators that integrate cellular and environmental signals to control cell activity are well known in bacteria (Liao et al., 2021). Among the regulators, fumarate and nitrate reduction regulatory protein (FNR) plays a central role in bacterial oxygen response. FNR regulates multiple biological processes through monitoring environmental oxygen via iron–sulfur cluster assembly-disassembly in a facultative anaerobe (Crack and Le Brun, 2018). These biological processes include the switch between aerobic/anaerobic metabolism (Crack et al., 2012), nitrogen fixation (Rutten and Poole, 2019), bioluminescence (Shi et al., 2020), infection (Kuntumalla et al., 2011), proliferation (Kado et al., 2017), virulence (Barbieri et al., 2014), the structure of the gut microbiome for adapting to the gut environment (Azcarate-Peril et al., 2018), and interactions between host and pathogen (Wang et al., 2019). Most researches about FNR focus on facultative bacteria under anaerobic conditions (Lin et al., 2019; Jiang et al., 2021), but a recent study has shown that FNR may regulate antibiotic synthesis in Serratia in the presence of oxygen (Sun et al., 2021). However, whether the transcriptional regulator that plays a role in metabolism contributes to antibiotic resistance is elusive.
Here, the role of FNR in aminoglycoside antibiotics was investigated in an EIB202 fnr-deleted mutant and the underlying mechanisms are explored. EIB202 is a highly pathogenic Edwardsiella tarda with available genome and causes hemorrhagic septicemia in fish and gastro- and extraintestinal infections in humans (Wang et al., 2009). The bacterium has been used a bacterial model for the study of bacterial antibiotic resistance (Su et al., 2015; Peng et al., 2015b). Since FNR regulates central carbon metabolism (Kargeti and Venkatesh, 2017), which is closely related to bacterial susceptibility to aminoglycosides (Peng et al., 2015b; Zhang et al., 2019), the present study explored whether FNR plays a role in aminoglycoside resistance. The loss of fnr caused elevated sensitivity to aminoglycosides. This is linked to the most elevated glutamate, the most impacted alanine, aspartate, and glutamate metabolism, and activation of the P cycle in Δfnr, which promoted proton motive force (PMF). Exogenous glutamate had a similar potential to E. tarda EIB202 (EIB202) to elevate sensitivity to aminoglycosides. These results indicate that fnr may regulate glutamate metabolism to play a role in the resistance to aminoglycosides in EIB202.
Materials and methods
Bacterial strains and culture conditions
EIB202 used in this study was obtained from professor Yuanxing Zhang, East China University of Science and Technology. EIB202 and its FNR gene deletion strain Δfnr was grown at 30°C for 24 h in 50 ml Luria-Bertani (LB) broth in 250-mL flasks.
Construction and complementation of FNR gene-deletion mutant
FNR gene deletion strain was constructed with one-step inactivation of chromosomal gene as described previously (Jiang et al., 2020). In brief, recombinant fragment was amplified from pKD13 using a pair of primer fnr-1F and fnr-1R (fnr-1F: AAAAGATGTTAAAATTGACCGATATCAATATTATTTAGGCAACACCTATGATTCCGGGGATCCGTCGACC, fnr-1R: AAAACGGCCCGCAGGCCGTCTTCTTTA TTCGCAGCGGGCGTTCATTCGCCTGTAGGCTGGAGCTGCTTCG. Sequence without underscore was fnr homologous sequence; Underline part was kanamycin gene homologous sequence). Then, the purified product was transformed into EIB202 component harboring pSIM6 plasmids, which express lambda red recombinase. Recombinants were selected by agar plate with 50 μg/ml kanamycin and validated by PCR amplification of kanamycin gene and fnr gene using two pairs of primers Kan-F/Kan-R (Kan-F:TGTAGGCTGGAGCTGCTT; Kan-R:ATTCCGGGGATCCG TCGA) and fnr-2F/ fnr-2R (fnr-2F: ATTCCGGAAAAACGTGTCAT; fnr-2R: GCA GGGCGTACGCGGATTAC), and western blotting. For gene complementation, the entire coding regions of fnr were amplified by PCR using primers (fnr-2F/ fnr-2R) and cloned into the modified pACYC184 plasmid. The recombinant plasmids were transformed into the Δfnr and selected on Luria broth with 100 μg/ml ampicillin to construct the complemented mutant strains Δfnr-resure.
Growth curve analysis
EIB202 and its mutant Δfnr were separately cultured in LB medium overnight and were diluted 1:100 (v/v) in fresh LB broth. These bacteria were cultured at 30°C with shaking 200 rpm and measured OD600 at every 2 h. All experiments were carried out in biological triplicates.
Survival capability assay
Bacterial survival capability assay was carried out as described previously with a modification (Lin et al., 2012). To test the survival capability of bacteria in indicated antibiotic concentration, the inoculums of EIB202 and Δfnr were separately cultured in 5 ml LB medium at 30°C overnight, and then the bacteria were diluted into a 5 ml fresh LB medium at a ratio of 1:1,000. Half of them contained indicated concentration antibiotic and the other was control without any antibiotic. These tubes were incubated at 30°C for 8 h. Bacterial growth was determined by measurement of OD600. The ability for survival was characterized by comparison between experimental and control groups and was termed as percent survival. At least three biologic replicates were performed.
Metabolomic analysis
Metabolomic analysis was performed as described previously (Peng et al., 2015b). In brief, 10 ml OD600 1.0 bacteria were quenched with 60% (v/v) cold of methanol (Sigma) and centrifuged at 6,297 rcf at 4°C for 5 min, followed by extraction of metabolites using 1 ml of cold methanol. To normalize variations across samples, 10 μl ribitol (0.1 mg per ml, Sigma-Aldrich, United States) was added into each sample as an internal standard. The supernatants were concentrated drying in a rotary vacuum centrifuge device (LABCONCO). The dried extracts were then incubated with 80 μl methoxyamine hydrochloride (20 mg/ml, Sigma–Aldrich) in pyridine (Sigma–Aldrich) for 180 min at 37°C, and then metabolite derivatization was done with an identical volume of N-methyl-N(trimethylsilyl)trifluoroacetamide (Sigma–Aldrich) for another 30 min. Samples were centrifuged at 14,167 rcf for 10 min, and the supernatant was transferred into new tubes for gas chromatography–mass spectrometry (GC–MS) analysis (Agilent).
Initial peak detection and mass spectral deconvolution were performed using Agilent Technologies MSD Productivity ChemStation Software (E.02.02.1431, 2011). Metabolites were identified using spectral matching and retention indexes from the National Institute of Standards and Technology (NIST) library using the NIST MS search 2.0. After the removal of any known artificial peaks and merger of the same compounds, the internal standard allows normalization of the resulting data. The peak intensities were normalized to form a single matrix with Rt-m/z pairs (retention time-mass charge ratio pairs) for each file in the data set. Data matrix was normalized according to the internal standard (ribitol). Normalized peak intensities formed a single matrix with Rt-m/z pairs (retention time-mass charge ratio pairs) for each file in the data set. The matrix can be used for further analysis. According to a reference distribution, Z score analysis scaled each metabolite. Statistical difference was obtained by Kruskal–Wallis test and Mann–Whitney test using SPSS 13.0. A p-value < 0.01 was considered significant. Hierarchical clustering was completed in the R platform1 with the function “heatmap. 2” of “gplots library.” Multivariate statistical analysis included principal component analysis (PCA) and orthogonal partial least square discriminant analysis (OPLS-DA) implemented with SIMCA 12.0 (Umetrics, Umeå, Sweden). Control scaling was selected prior to fitting. All variables were mean-centered and scaled to Pareto variance of each variable. PCA was used to reduce the high dimension of the data set. We analyzed the differential metabolites to their respective biochemical pathways as outlined in the MetaboAnalyst 5.0.2 Pathways were enriched by raw p value <0.05. To explore a global view of bacterial metabolism of Δfnr, Interactive Pathways (iPath) analysis using iPath3.03 was carried out as described previously (Yamada et al., 2011).
Real-time quantitative PCR
Total RNA samples were prepared from indicated strains using TRIzol reagent (Invitrogen). Double-stranded cDNA was synthesized from total RNA using the SYBR Perfect real-time series kits (Takara). cDNA was analyzed by real-time RT-PCR using Roche’s LightCycler 480 real-time PCR system. Quantitative PCR was performed on each cDNA sample in triplicate. 16 s rRNA was used as an internal control to normalize the expression level. The expression level was calculated using the comparative 2–ΔΔCt method. The primers used for amplification are listed in Supplementary Table 1.
Measurement of membrane potential
Measurement of membrane potential was described previously (Zhao et al., 2021). Bacteria were collected and strained with 10 μl of 3 mM DiOC2 (BacLight Bacterial Membrane Potential Kit, Invitrogen) for 30 min at 30°C. Aliquots with 1 ml of culture were added into flow tubes before analysis. Samples were analyzed on a FACSCalibur flow cytometer (Becton Dickinson, San Jose, CA, United States) at 30°C. Each sample was observed with forwarding versus side scatter and gated before the acquisition of data. Settings were optimized according to the manual. The computational formula of membrane potential: Log[103/2 × (red fluorescence/green fluorescence)]. Experiments were repeated at least in three independent biological replicates.
Measurement of enzyme activity
Measurement of enzyme activity was performed as previously described with a few modifications (Jiang et al., 2020). A volume of 30 ml bacterial cultures at OD600 = 1.0 were collected by centrifugation. After washing, bacterial cells were crushed by ultrasonic crusher for 10 min at a 200-W on ice. Supernatants containing 200 μg of total proteins were transferred to a pyruvate dehydrogenase (PDH) reaction mix (0.15 mM MTT, 1 mM MgCl2, 0.5 mM PMS, 0.2 mM TPP, 2 mM sodium pyruvate, and 50 mM PBS), an α-ketoglutarate dehydrogenase (KGDH) reaction mix (0.15 mM MTT, 1 mM MgCl2, 0.5 mM PMS, 0.2 mM TPP, 50 mM α-ketoglutaric acid potassium salt, and 50 mM PBS), a succinate dehydrogenase (SDH) reaction mix (0.15 mM MTT, 1 mM PMS, 5 mM sodium succinate, and 50 mM PBS), and a malate dehydrogenase (MDH) reaction mix (0.15 mM MTT, 1 mM PMS, 50 mM PBS, and 50 mM malate) to a final volume of 200 μl in a 96-well plate. Subsequently, the plate was incubated at 30°C for 30 min for PDH/KGDH/MDH activity and at 30°C for 5 min for SDH activity and then measured at 566 nm for colorimetric readings. The plates were protected from light during the incubation. Experiments were repeated at least four independent biological replicates.
Bactericidal assay
Bactericidal assay was carried out as described previously (Allison et al., 2011; Peng et al., 2015b). After cultured at 30°C for 24 h, bacterial cells were collected by centrifugation at 6,297 rcf for 5 min. The samples were washed with sterile saline three times and then resuspended in M9 minimal media containing 10 mM acetate, 1 mM MgSO4, and 100 μM CaCl2 to arrive at OD600 0.2. Aliquot of 5 ml bacterial suspension with desired glutamate or/and gentamicin was incubated at 30°C and 200 rpm for 6 h. To determine bacterial count, 100 μl of cultures were obtained and then serially diluted. An aliquot of 5 μl of each dilution was plated on LB agar.
Results
Resistance of Δfnr to aminoglycoside antibiotics
To understand the role of FNR in resistance to aminoglycoside antibiotics, fnr deletion mutant was constructed (Supplementary Figure 1). First, the growth curve of EIB202 and Δfnr was detected, showing that the loss of fnr caused a higher growth during 2–12 h but not later (Figure 1A). Then, survival capability assay was used to test bacterial sensitivity to aminoglycosides gentamicin, micronomicin, tobramycin, and amikacin. The loss of fnr caused elevated sensitivity to these antibiotics (Figure 1B). These results indicate that the absence of fnr increases bacterial sensitivity to aminoglycoside antibiotics.
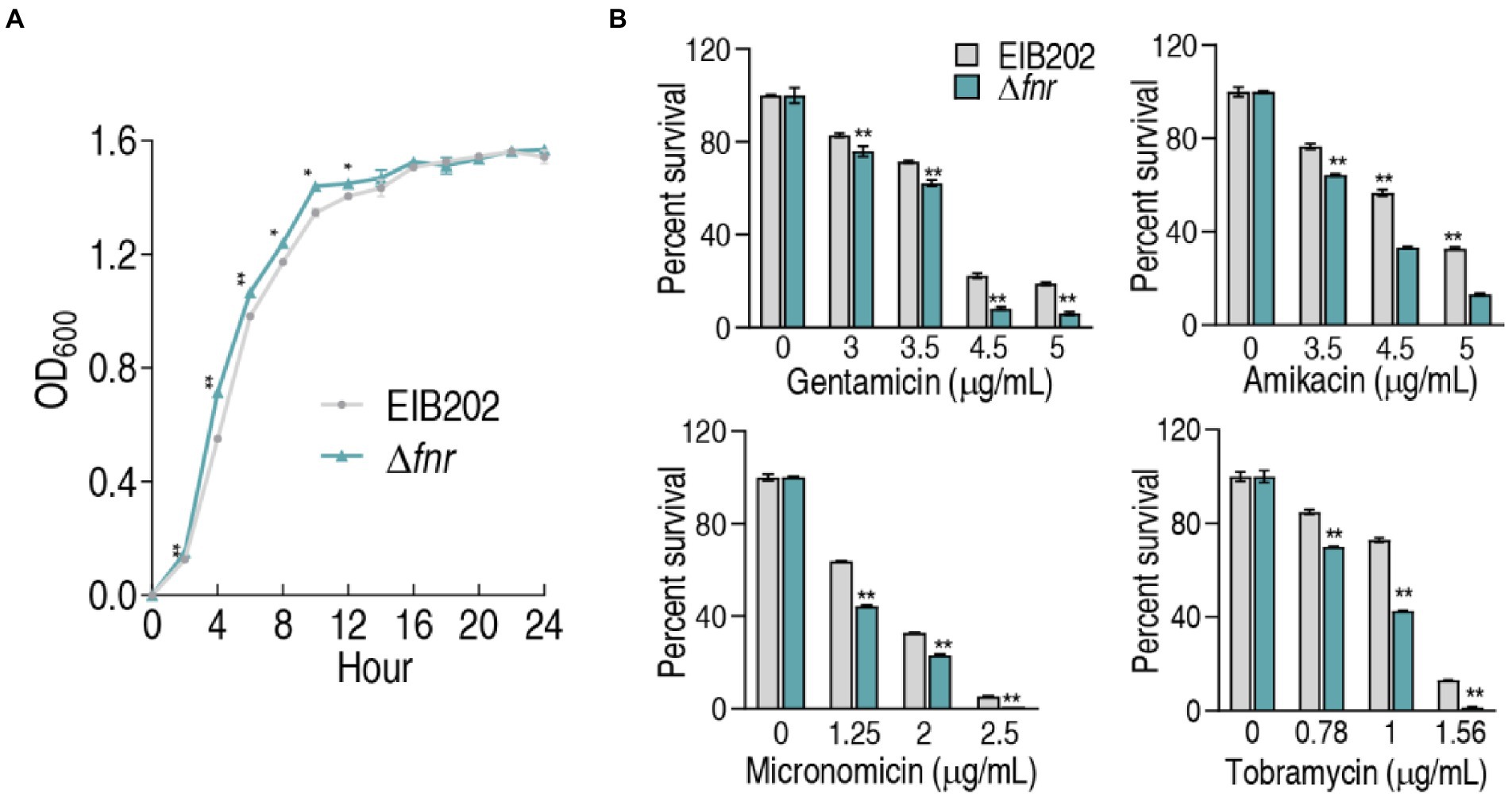
Figure 1. Growth and survival of genetically modified strains of fnr deletion. (A) Growth curve of EIB202 and Δfnr. (B) Survival of Δfnr and its parent strain EIB202 to aminoglycoside antibiotics. Results are displayed as means ± SEM, and significant differences are identified (*p < 0.05; **p < 0.01) as determined by Student’s t-test. At least three biological repeats were carried out.
Metabolomic profiling of Δfnr
Reports have shown that bacterial metabolomes contribute to antibiotic efficacy (Peng et al., 2015b; Zhao et al., 2021), and gene-deleted mutants exhibit differential metabolomes (Jiang et al., 2020). Therefore, GC–MS-based metabolomics was used to investigate whether the loss of fnr affects metabolic profiles. Four biological replicates with two technical repeats in each group were performed, yielding a total of 16 data sets. The high reproducibility of the identification in the discovery phase is shown in (Supplementary Figure 2A). This led to identification of 74 metabolites each sample (Supplementary Figure 2B). Biological categories of the identified metabolites were searched against the Kyoto Encyclopedia of Genes and Genomes (KEGG). The categories showed that 29.72% (22), 27.02% (20), 24.32% (18), 10.81% (8), and 8.10% (6) of metabolites belong to carbohydrate, amino acid, fatty acid, nucleotide, and other, respectively (Supplementary Figure 2C).
Compared with the metabolome of EIB202, 45 (60.8%) metabolites showed differential abundances (p < 0.05) in Δfnr (Figure 2A). Z-value based on the control group was calculated, showing that it spanned from −10.23 to 18.17 in Δfnr (Figure 2B). We further examined the metabolic categories of these differential metabolites. They showed differential percentages with a ranking of carbohydrate > amino acid > fatty acid > other > nucleotide in Δfnr (Figure 2C). The numbers of up- and down-regulated metabolites in these categories were listed in (Figure 2D). These results indicate that the loss of fnr affects bacterial metabolic profile.
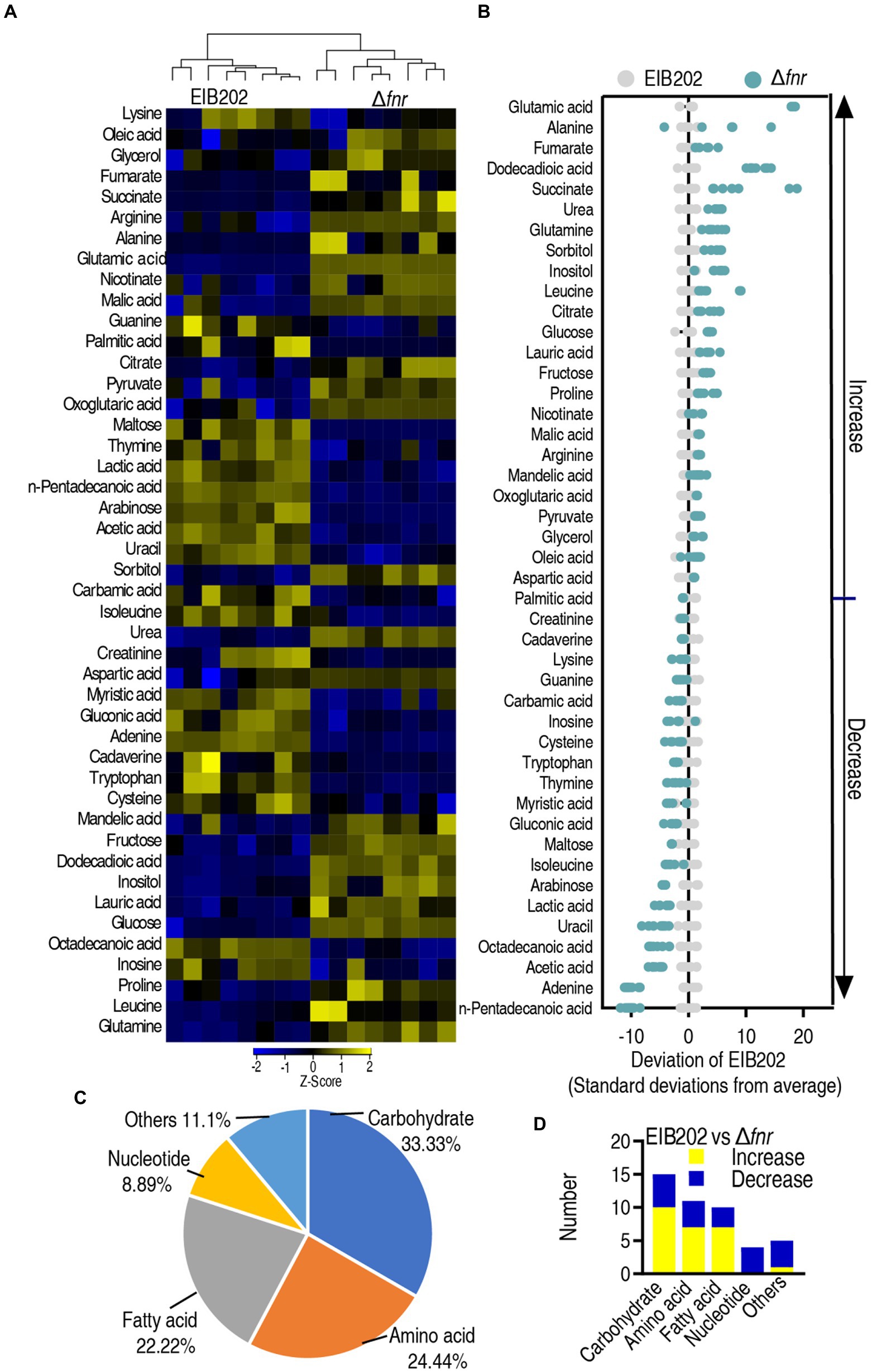
Figure 2. Differential abundance of metabolites in EIB202 and Δfnr. (A) Heat map showing relative abundance of different metabolites (row). Heat map scale (blue to yellow, low to high abundance) is shown below data. (B) Z score plots of differential abundances of metabolites based on control. Data from tested groups are separately scaled to the mean and standard deviation of the control (EIB202). Each point represents one metabolite in one technical repeat and is colored by sample type (gray, control; green, Δfnr). (C) Category of detected different metabolites in Δfnr. (D) The number of changed metabolites in each category of Δfnr and EIB202.
Enrichment of metabolic pathways involved in Δfnr
Enriched metabolic pathways are important for understanding the metabolome alteration due to the loss of fnr. A total of 12 pathways were enriched after analyzing differential metabolites between Δfnr and EIB202. The 12 pathways were alanine, aspartate, and glutamate metabolism; pyruvate metabolism; TCA cycle; nitrogen metabolism; butanoate metabolism; arginine and proline metabolism; D-glutamine and D-glutamate metabolism; lysine degradation, sulfur metabolism; arginine biosynthesis; nicotinate and nicotinamide metabolism; and glyoxylate and dicarboxylate metabolism (Figure 3A). Except for lysine degradation and sulfur metabolism, in which part metabolites detected were increased or decreased in abundance, the other pathways showed that all metabolites were increased in abundance (Figure 3B). Notably, the top three enriched metabolic pathways are closely interrelated. Specifically, parts of pyruvate metabolism (pyruvate → acetyl-CoA and → oxaloacetate) plus the TCA cycle belong to the P cycle (Su et al., 2018), while alanine, aspartate and glutamate metabolism provides fuels for the P cycle.
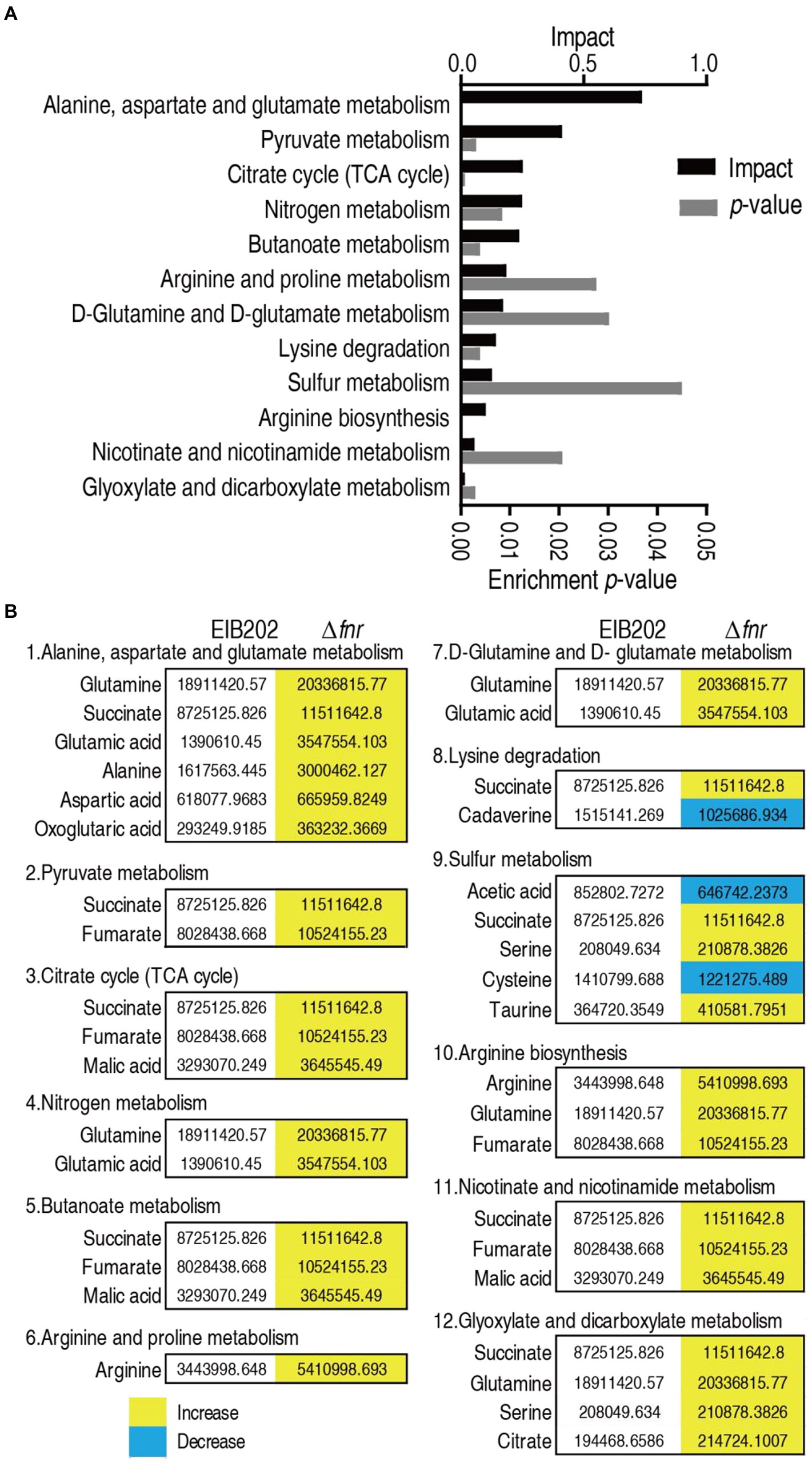
Figure 3. Pathway enrichment. (A) Pathway enrichment of varied metabolites in Δfnr. (B) Integrative analysis of metabolites in significantly enriched pathways. Yellow color and green color indicate increased and decreased metabolites, respectively.
Identification of crucial biomarkers using multivariate data analysis
To identify crucial biomarkers representing differential metabolomes in Δfnr, orthogonal partial least-squares discriminant analysis (OPLS-DA) was applied for the recognition of the sample patterns, followed by ranking the altered abundance of metabolites in loading. Δfnr was separated from the control group EIB202 by predictive component [1] (Figure 4A). Discriminating variables were present with S-plot when cut-off values were set as greater or equal to 0.05 and 0.5 for the absolute value of covariance p and correlation p (corr), respectively. Glutamic acid, leucine, succinate, fumarate, alanine, arginine, glutamine, glycerol, nicotinate, inositol, proline, adenine, pentadecanoic acid, inosine, lysine, and octadecanoic acid are identified as biomarkers in the predictive component [1] between the mutant and control (Figure 4B). Among the 16 metabolites, 11 (glycerol, inositol, glutamine, glutamic acid, leucine, fumarate, alanine, succinate, nicotinate, arginine, proline) were increased and 5 (adenine, inosine, lysine, octadecanoic acid, and pentadecanoic acid) were decreased (Figures 4C,D). Out of these metabolites, glutamic acid belongs to alanine, aspartate, and glutamate metabolism, the biggest impact pathway. Glutamic acid has the most absolute value of covariance p and the most difference in abundance between EIB202 and Δfnr. Therefore, glutamic acid is identified as the most crucial biomarker.
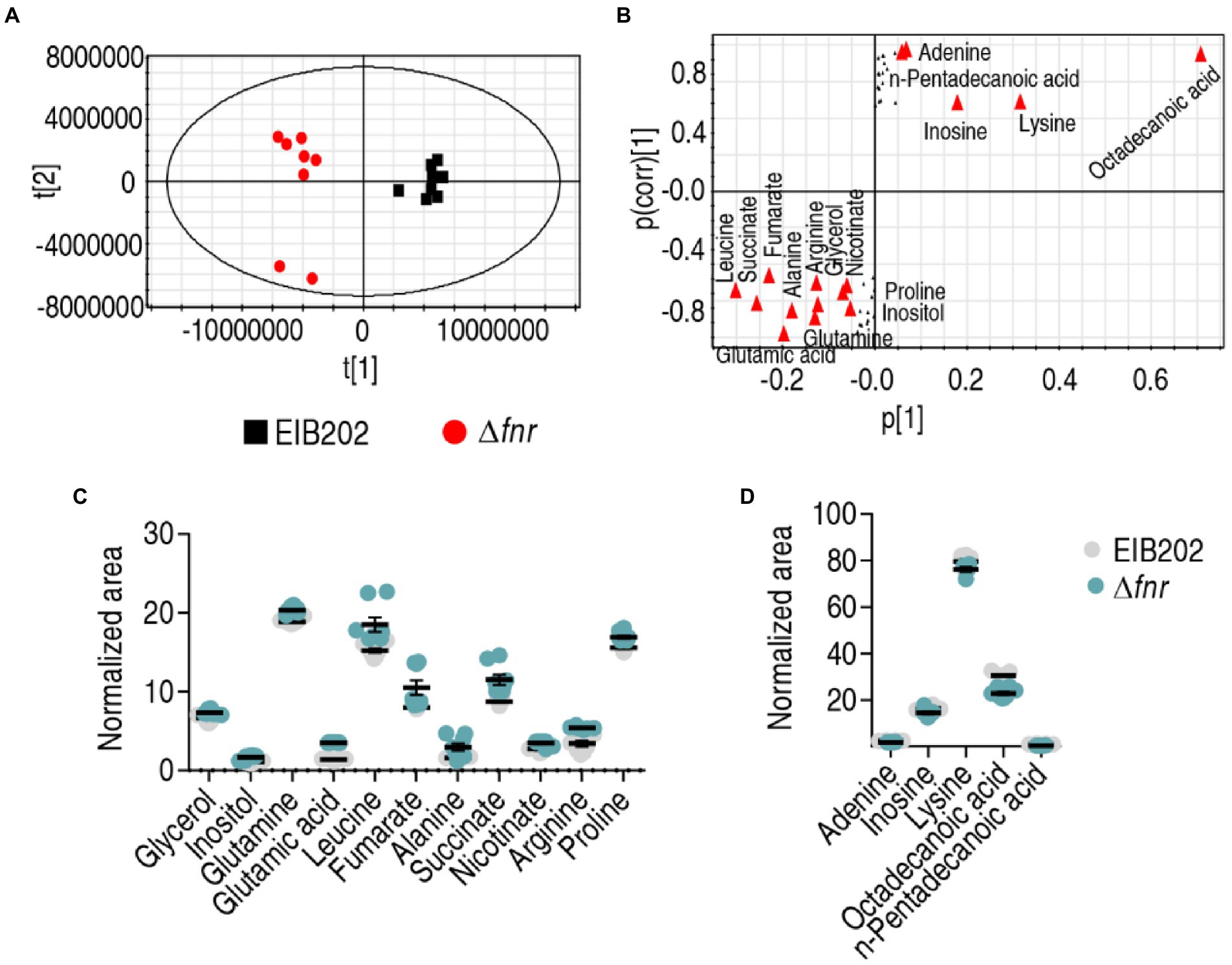
Figure 4. Identification of crucial metabolites. (A) The PCA analysis of EIB202 and Δfnr. Each dot represents the technical replicate of samples in the plot. (B) S-plot generated from OPLS-DA. Predictive component p[1] and correlation p(corr)[1] differentiate Δfnr from EIB202. The triangle represents metabolites in which candidate biomarkers are marked. (C) and (D) Scatter diagram of 16 biomarkers.
iPath analysis
Comparative metabolic pathway analysis between Δfnr and EIB202 was carried out in iPath. The resulting global overview map provides a better insight into the effects of the fnr loss, where brown line represents increased pathways and blue line represents decreased pathways in Δfnr (Figure 5A). TCA cycle and energy metabolism were elevated (Figure 5A), implying the importance of the activation of the pyruvate cycle since the pyruvate rather than the TCA cycle provides respiratory energy (Su et al., 2018). To validate the contributing role of the pyruvate cycle in Δfnr, expression of 15 genes and activity of four key enzymes in the pyruvate cycle were measured. The four key enzymes include PDH that transforms pyruvate into acetyl-CoA, KGDH that converts a-ketoglutarate to succinyl-CoA, SDH that catalyzes the oxidation of succinate to fumarate, and MDH that catalyzes the interconversion of L-malate and oxaloacetate. Among the 15 genes, 13 were elevated and the others were not changed in Δfnr (Figure 5B). Consistently, activity of the four enzymes was elevated due to the loss of fnr (Figure 5C). Therefore, proton motive force (PMF) was promoted (Figure 5D). These results indicate that the loss of fnr promotes the pyruvate cycle to elevate PMF.
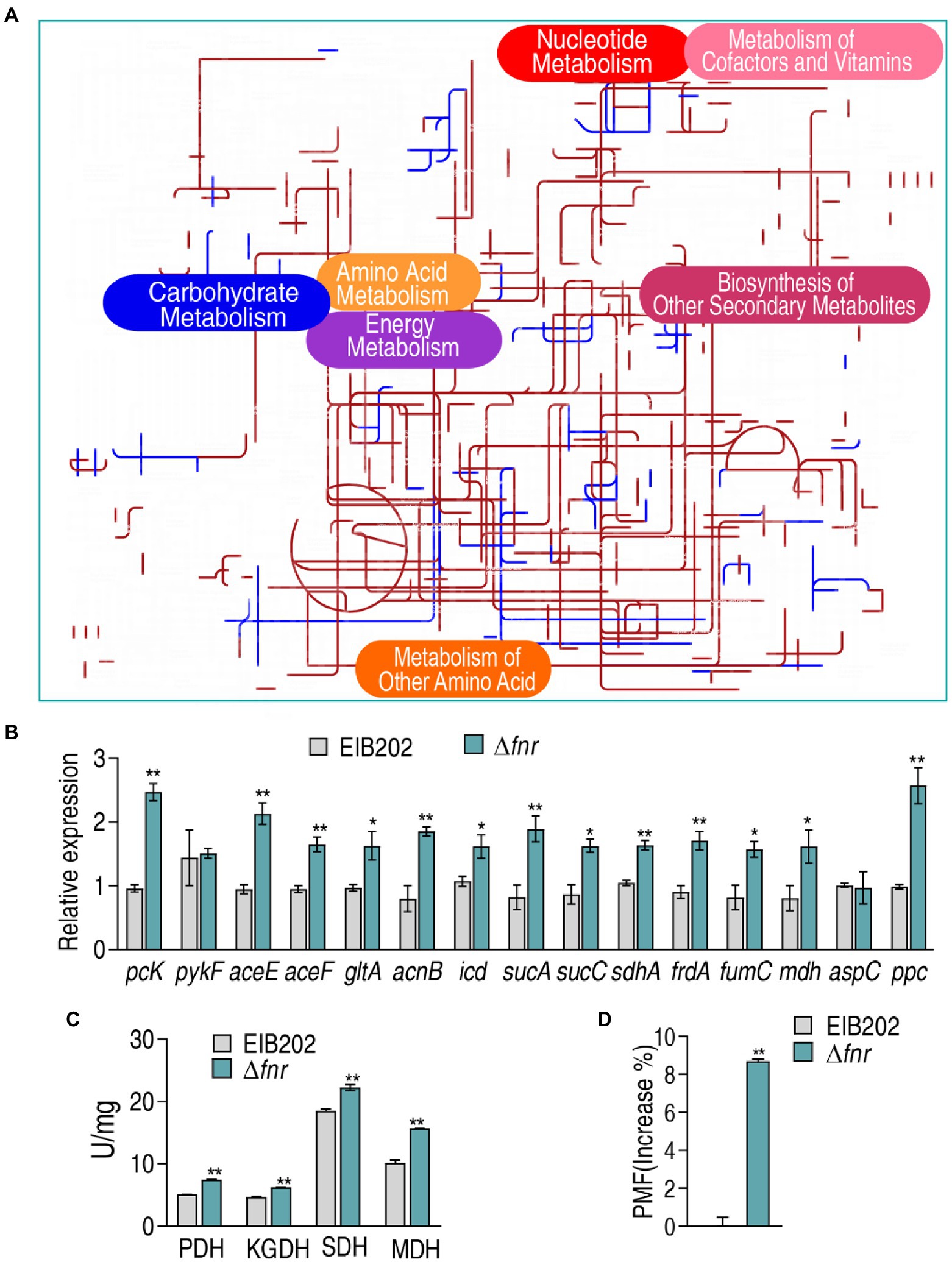
Figure 5. Comparative metabolic pathway analysis and enzyme activity measurement between Δfnr and its parent strain EIB202. (A) Analysis of the metabolic profiles resulting from Δfnr provides a better insight into the effects of 45 significant metabolites (p < 0.05). Based on the KEGG compound (http://www.kegg.jp/kegg/compound/), metabolic network pathways in Δfnr are further analyzed with iPath3.0 (https://pathways.embl.de/). Purple line represents increase and blue line represents decrease in Δfnr. (B) qRT-PCR for expression of pyruvate cycle genes. (C) Activity of PDH, KGDH, SDH and MDH in EIB202. (D) Membrane potential of EIB202 and Δfnr. Results are displayed as means ± SEM, and significant differences are identified (*p < 0.05; **p < 0.01) as determined by Student’s t-test. At least three biological repeats were carried out.
Exogenous glutamate promotes gentamicin-mediated killing to EIB202
Glutamate is linked to the P cycle and thereby the most elevated glutamate can fuel the P cycle, which is responsible for the elevated sensitivity to aminoglycosides in Δfnr. Logically, when glutamate is added to a certain extent, similar sensitivity to the antibiotics may be obtained in EIB202. To explore this idea, viability of EIB202 and Δfnr was measured in different doses of gentamicin using bactericide assay. Lower survival was detected in Δfnr than EIB202 in a gentamicin dose-dependent manner (Figure 6A). However, the difference of the survival between Δfnr and EIB202 disappeared with the increasing glutamate concentrations. Specifically, survival was lower in Δfnr than EIB202 in medium with 0.15 mM glutamate, but similar and higher viability was detected in medium with 0.3125 mM and 0.625–1.25 mM glutamate, respectively in EIB202 than Δfnr without gluamate (Figure 6B). Moreover, when 0.3125 mM glumate was supplemented, an increased sensitivity in EIB202 was detected in a manner of gentamicin-dependence and time-dependence, which was the same as Δfnr did without glutamate (Figures 6C,D). These results indicate that the elevated glutamate may be a reason why Δfnr is sensitive to aminoglycosides.
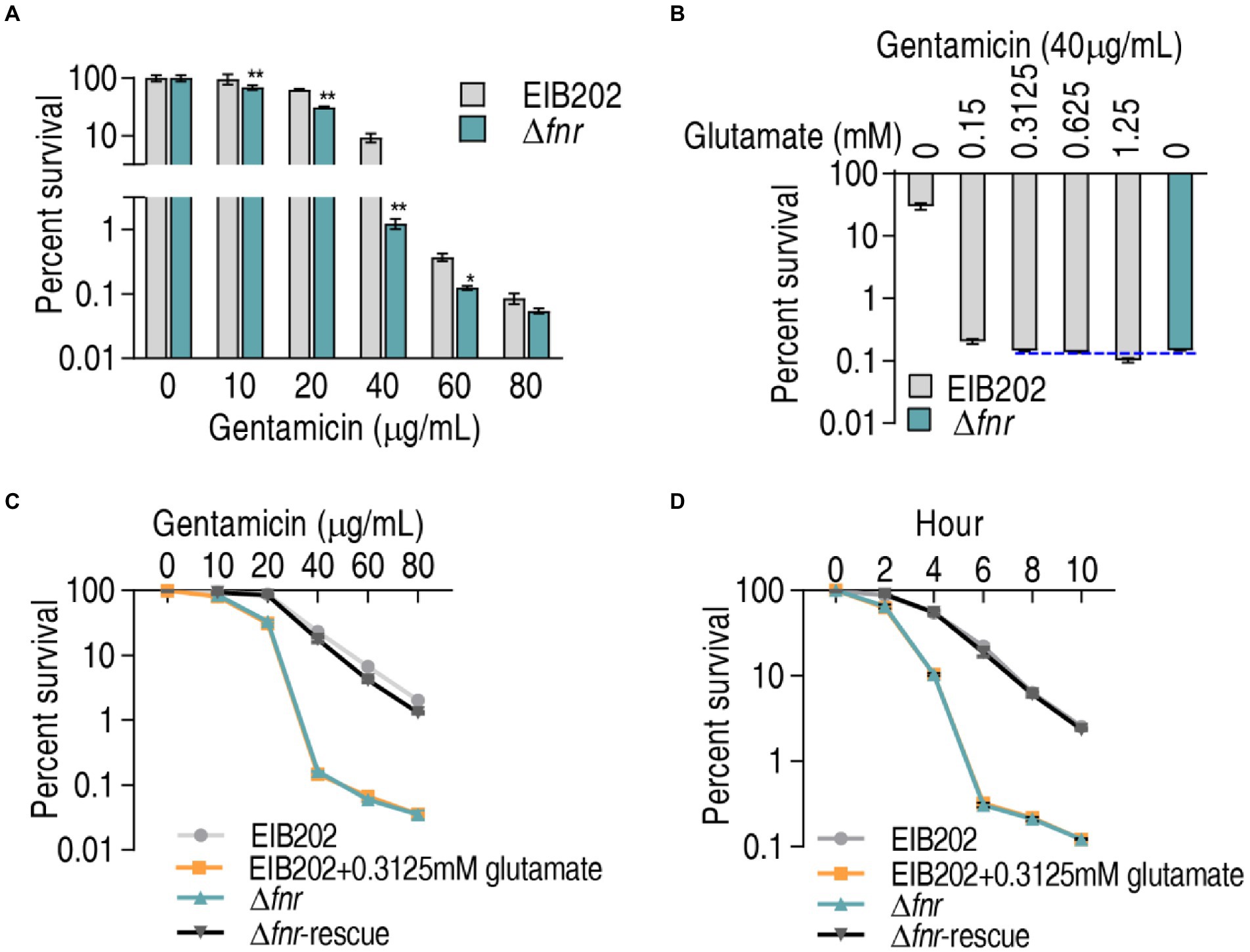
Figure 6. Exogenous glutamate elevates sensitivity of EIB202 to gentamicin. (A) Percent survival of EIB202 and Δfnr in the indicated concentration of gentamicin. (B) Percent survival of EIB202 and Δfnr in the indicated concentration of glutamate plus 40 μg/ml gentamicin. (C) Percent survival of EIB202 and Δfnr in the indicated concentration of gentamicin plus 0.3125 mM glutamate. (D) Percent survival of EIB202 and Δfnr in the indicated times with 0.3125 mM glutamate plus 40 μg/ml gentamicin. Results are displayed as means ± SEM, and significant differences are identified (*p < 0.05; **p < 0.01) as determined by Student’s t-test. At least three biological repeats were carried out.
Exogenous glutamate promotes gentamicin-mediated killing to EIB202 via activating the pyruvate cycle
Further experiments were focused whether exogenous glutamate promotes the pyruvate cycle for more PMF to elevate the sensitivity to gentamycin in EIB202 as Δfnr did. To test this, qRT-PCR was used to quantify the gene expression of the pyruvate cycle in EIB202. Among the detected 15 genes, 13 were increased when exogenous glutamate was added (Figure 7A). Consistently, exogenous glutamate promoted activity of PDH, KGDH, and SDH, which led to the similar PMF as Δfnr did (Figures 7B,C). These results indicate that the elevated glutamate is a reason why Δfnr is sensitive to aminoglycosides.
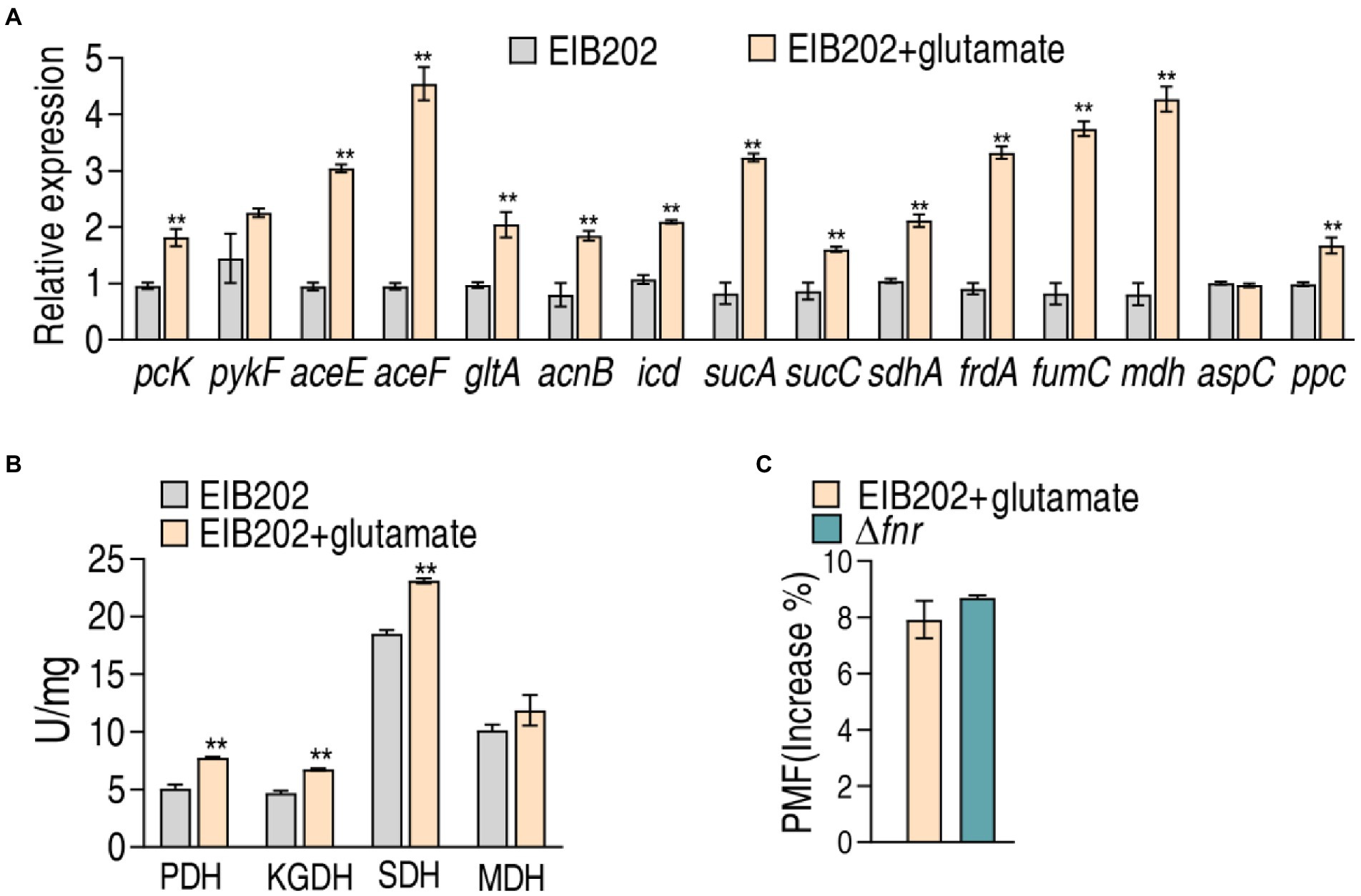
Figure 7. Effect of exogenous glutamate on gene expression, enzyme activity, and PMF in EIB202. (A) qRT-PCR for expression of genes of the P cycle in EIB202 with or without 0.3125 mM glutamate. (B) Activity of enzymes of the P cycle in EIB202 with or without 0.3125 mM glutamate. (C) Measurement of PMF in EIB202 with 0.3125 mM glutamate and Δfnr. Results are displayed as means ± SEM, and significant differences are identified (**p < 0.01) as determined by Student’s t-test. At least three biological repeats were carried out.
Discussion
It has been documented that bacterial metabolic environment confounds antibiotic susceptibility (Peng et al., 2015b; Zhao et al., 2021), but the role of global transcriptional regulator FNR that regulates oxygen response in antibiotic resistance is largely unknown. The present study uses EIB202, a model E. tarda that causes a great loss in aquaculture, to explore whether FNR plays a role in the resistance aminoglycosides. To do this, fnr deletion mutant was constructed to test sensitivity to aminoglycoside antibiotics. The absence of fnr causes elevated sensitivity to the four types of aminoglycoside antibiotics tested. For understanding whether fnr mediates the resistance via managing metabolism, GC–MS-based metabolomics is used to characterize the altered metabolic profile caused by the loss of fnr. The loss leads to an activated metabolome, characterizing the most elevated glutamate and the most impactful alanine, aspartate, and glutamate metabolism as the most consequence of the fnr absence. The dominantly enhanced metabolome and the most elevated glutamate and impactful metabolic pathway suggest that the P cycle plays a crucial role in the resistance. This is demonstrated by elevated gene expression, enzymatic activity, and PMF, which supports the conclusion on the PMF-potentiated killing of aminoglycoside antibiotics. It proceeds by increasing glutamate to promote the P cycle and thereby elevate PMF. These results indicate that FNR plays a role in the aminoglycosides resistance via metabolic regulation. This is first time to reveal an FNR-related antibiotic resistance mechanism.
FNR regulates metabolism has been reported (Crack and Le Brun, 2018; Jiang et al., 2021), but information regarding its influence on metabolic profile is not available. The present study shows that the loss of fnr causes globally metabolic activation, indicating that the global transcriptional regulator depresses global metabolism. This may be related to its role in regulating respiratory metabolism that produces the energy and substrate molecules to influence other metabolisms. In bacteria, the P cycle instead of the TCA cycle provides respiratory energy (Su et al., 2018). Thus, the present study demonstrates that the loss of FNR leads to the elevation of gene expression and enzymatic activity of the P cycle and then shows that the resulting PMF contributes to the sensitivity to gentamicin. These results not only provide a solid proof that FNR regulates global metabolism, but also reveal that the regulation contributes to aminoglycosides resistance.
Another interesting finding is that the loss of fnr elevates glutamate, which, in turn, increases the sensitivity to aminoglycosides. The finding is consistent with the reports that reduced glutamate is a characteristic feature in kanamycin-resistant E. tarda and complemented glutamate potentiates kanamycin-mediated killing (Peng et al., 2015b; Su et al., 2018). The present study demonstrates that exogenous glutamate promotes bacterial sensitivity to gentamicin in a dose-dependent manner in EIB202. The resulting effect can be equal to that caused by the absence of fnr, which supports the conclusion that FNR regulates resistance to gentamicin through modulation of glutamate level. Furthermore, the modulation influences the P cycle and PMF. These results indicate that the regulation of glutamate is a mechanism by which FNR plays a role in aminoglycosides resistance.
Conclusion
An fnr-deleted mutant is constructed to explore a role of the gene in aminoglycoside antibiotics. The mutant shows an elevated sensitivity to gentamicin, micronomicin, tobramycin, and amikacin, which is linked to the most activated alanine, aspartate, and glutamate metabolism and the P cycle and the most elevated glutamic acid. These promote PMF that contributes to aminoglycosides-mediated killing. Further evidence show that exogenous glutamate plays the same role as fnr deletion, including activation of the P cycle, promotion of PMF, and elevation of gentamicin-mediated killing. Therefore, FNR plays a role in aminoglycoside resistance by modulation of glutamate metabolism. These findings reveal the underlying mechanism by which FNR regulates aminoglycoside antibiotic resistance.
Data availability statement
The original contributions presented in the study are included in the article/Supplementary material, further inquiries can be directed to the corresponding author.
Author contributions
HL conceptualized the project and designed the protocol. LM and SL performed the experiments and interpreted the data. HL and XP wrote the manuscript. All authors contributed to the article and approved the submitted version.
Funding
This work was sponsored by the National Natural Science Foundation of China (31930115 and 31902414), International Exchanges Scheme (NSFC-RS; 32061133007), Innovation Group Project of Southern Marine Science and Engineering Guangdong Laboratory (Zhuhai; no. 311020006).
Conflict of interest
The authors declare that the research was conducted in the absence of any commercial or financial relationships that could be construed as a potential conflict of interest.
Publisher’s note
All claims expressed in this article are solely those of the authors and do not necessarily represent those of their affiliated organizations, or those of the publisher, the editors and the reviewers. Any product that may be evaluated in this article, or claim that may be made by its manufacturer, is not guaranteed or endorsed by the publisher.
Supplementary material
The Supplementary material for this article can be found online at: https://www.frontiersin.org/articles/10.3389/fmicb.2022.1003586/full#supplementary-material
Footnotes
1. ^https://cran.r-project.org/ (Accessed August 20, 2022).
2. ^http://www.metaboanalyst.ca/ (Accessed August 20, 2022).
3. ^https://pathways.embl.de/ (Accessed August 20, 2022).
References
Allison, K. R., Brynildsen, M. P., and Collins, J. J. (2011). Metabolite-enabled eradication of bacterial persisters by aminoglycosides. Nature 473, 216–220. doi: 10.1038/nature10069
Azcarate-Peril, M., Butz, N., Cadenas, M., Koci, M., Ballou, A., Mendoza, M., et al. (2018). An attenuated salmonella enterica serovar Typhimurium strain and galacto-oligosaccharides accelerate clearance of salmonella infections in poultry through modifications to the gut microbiome. Appl. Environ. Microbiol. 84, e02526–e02517. doi: 10.1128/AEM.02526-17
Barbieri, N., Nicholson, B., Hussein, A., Cai, W., Wannemuehler, Y., Dell'Anna, G., et al. (2014). FNR regulates expression of important virulence factors contributing to pathogenicity of uropathogenic Escherichia coli. Infect. Immun. 82, 5086–5098. doi: 10.1128/IAI.02315-14
Crack, J., Green, J., Hutchings, M., Thomson, A., and Le Brun, N. E. (2012). Bacterial iron–sulfur regulatory proteins as biological sensor-switches. Antioxid. Redox Signal. 17, 1215–1231. doi: 10.1089/ars.2012.4511
Crack, J., and Le Brun, N. E. (2018). Redox-sensing iron–sulfur cluster regulators. Antioxid. Redox Signal. 29, 1809–1829. doi: 10.1089/ars.2017.7361
Evangelista, A. G., Corrêa, J. A. F., Pinto, A. C. S. M., and Luciano, F. B. (2022). The impact of essential oils on antibiotic use in animal production regarding antimicrobial resistance – a review. Crit. Rev. Food Sci. Nutr. 62, 5267–5283. doi: 10.1080/10408398.2021.1883548
Jiang, F., Huang, X., Barbieri, N. L., Logue, C. M., Nolan, L. K., and Li, G. (2021). Citrate utilization under anaerobic environment in Escherichia coli is under direct control of Fnr and indirect control of arc A and Fnr via Cit A-Cit B system. Environ. Microbiol. 23, 1496–1509. doi: 10.1111/1462-2920.15357
Jiang, M., Kuang, S., Lai, S., Zhang, S., Yang, J., Peng, B., et al. (2020). Na+-NQR confers aminoglycoside resistance via the regulation of l-alanine metabolism. MBio 11, e02086–e02020. doi: 10.1128/mBio.02086-20
Kado, T., Kashimoto, T., Yamazaki, K., and Ueno, S. (2017). Importance of fumarate and nitrate reduction regulatory protein for intestinal proliferation of Vibrio vulnificus. FEMS Microbiol. Lett. 364:fnw274. doi: 10.1093/femsle/fnw274
Kargeti, M., and Venkatesh, K. V. (2017). The effect of global transcriptional regulators on the anaerobic fermentative metabolism of Escherichia coli. Mol. BioSyst. 13, 1388–1398. doi: 10.1039/c6mb00721j
Kuntumalla, S., Zhang, Q., Braisted, J., Fleischmann, R., Peterson, S., Donohue-Rolfe, A., et al. (2011). In vivo versus in vitro protein abundance analysis of Shigella dysenteriaetype 1 reveals changes in the expression of proteins involved in virulence, stress and energy metabolism. BMC Microbiol. 11, 1–13. doi: 10.1186/1471-2180-11-147
Lee, H., and Collins, J. (2011). Microbial environments confound antibiotic efficacy. Nat. Chem. Biol. 8, 6–9. doi: 10.1038/nchembio.740
Li, L., Su, Y., Peng, B., Peng, X., and Li, H. (2020). Metabolic mechanism of colistin resistance and its reverting in vibrio alginolyticus. Environ. Microbiol. 22, 4295–4313. doi: 10.1111/1462-2920.15021
Liao, Y., Vogel, V., Hauber, S., Bartel, J., Alkhnbashi, O., Maaß, S., et al. (2021). Cdr S is a global transcriptional regulator influencing cell division in Haloferax volcanii. MBio 12, e01416–e01421. doi: 10.1128/mBio.01416-21
Lin, X. M., Wang, C., Guo, C., Tia, N. Y. M., Li, H., and Peng, X. X. (2012). Differential regulation of Omp C and Omp F by Atp B in Escherichia coli exposed to nalidixic acid and chlortetracycline. J. Proteome 75, 5898–5910. doi: 10.1016/j.jprot.2012.08.016
Lin, T. H., Wu, C. C., Kuo, J. T., Chu, H. F., Lee, D. Y., and Lin, C. T. (2019). FNR-dependent Rmp A and Rmp A2 regulation of capsule polysaccharide biosynthesis in Klebsiella pneumoniae. Front. Microbiol. 10:2436. doi: 10.3389/fmicb.2019.02436
Murray, C., Ikuta, K., Sharara, F., Swetschinski, L., Aguilar, G., Gray, A., et al. (2022). Global burden of bacterial antimicrobial resistance in 2019: A systematic analysis. Lancet 399, 629–655. doi: 10.1016/S0140-6736(21)02724-0
Peng, B., Li, H., and Peng, X. (2015a). Functional metabolomics: from biomarker discovery to metabolome reprogramming. Protein Cell 6, 628–637. doi: 10.1007/s13238-015-0185-x
Peng, B., Su, Y. B., Li, H., Han, Y., Guo, C., Tian, Y. M., et al. (2015b). Exogenous alanine and/or glucose plus kanamycin kills antibiotic-resistant bacteria. Cell Metab. 21, 249–262. doi: 10.1016/j.cmet.2015.01.008
Rutten, P. J., and Poole, P. S. (2019). Oxygen regulatory mechanisms of nitrogen fixation in rhizobia. Adv. Microb. Physiol. 75, 325–389. doi: 10.1016/bs.ampbs.2019.08.001
Shi, H., Li, Y., Hao, T., Liu, X., Zhao, X., and Chen, S. (2020). The role of Fnr paralogs in controlling anaerobic metabolism in the diazotroph Paenibacillus polymyxa WLY78. Appl. Environ. Microbiol. 86, e03012–e03019. doi: 10.1128/AEM.03012-19
Su, Y. B., Peng, B., Han, Y., Li, H., and Peng, X. X. (2015). Fructose restores susceptibility of multidrug-resistant Edwardsiella tarda to kanamycin. J. Proteome Res. 14, 1612–1620. doi: 10.1021/pr501285f
Su, Y., Peng, B., Li, H., Cheng, Z., Zhang, T., Zhu, J., et al. (2018). Pyruvate cycle increases aminoglycoside efficacy and provides respiratory energy in bacteria. Proc. Nat. Acad. Sci. 115, E1578–E1587. doi: 10.1073/pnas.1714645115
Sun, J., Deng, Z., and Yan, A. (2014). Bacterial multidrug efflux pumps: mechanisms, physiology and pharmacological exploitations. Biochem. Biophys. Res. Commun. 453, 254–267. doi: 10.1016/j.bbrc.2014.05.090
Sun, D., Zhou, X., Liu, C., Zhu, J., Ru, Y., Liu, W., et al. (2021). Fnr negatively regulates prodigiosin synthesis in Serratia sp. ATCC 39006 during aerobic fermentation. Front. Microbiol. 12:734854. doi: 10.3389/fmicb.2021.734854
Wang, Z., Sun, J., Tian, M., Xu, Z., Liu, Y., Fu, J., et al. (2019). Proteomic analysis of FNR-regulated anaerobiosis in salmonella Typhimurium. J. Am. Soc. Mass Spectrom. 30, 1001–1012. doi: 10.1007/s13361-019-02145-2
Wang, Q. Y., Yang, M. J., Xiao, J. F., Wu, H. Z., Wang, X., Lv, Y. Z., et al. (2009). Genome sequence of the versatile fish pathogen Edwardsiella tarda provides insights into its adaptation to broad host ranges and intracellular niches. PLoS One 4:e7646. doi: 10.1371/journal.pone.0007646
Yamada, T., Letunic, I., Okuda, S., Kanehisa, M., and Bork, P. (2011). iPath2.0: interactive pathway explorer. Nucleic Acids Res. 39, W412–W415. doi: 10.1093/nar/gkr313
Ye, J., Lin, X., Cheng, Z., Su, Y., Li, W., Ali, F., et al. (2018a). Identification and efficacy of glycine, serine and threonine metabolism in potentiating kanamycin-mediated killing of Edwardsiella piscicida. J. Proteome 183, 34–44. doi: 10.1016/j.jprot.2018.05.006
Ye, J., Su, Y., Lin, X., Lai, S., Li, W., Ali, F., et al. (2018b). Alanine enhances aminoglycosides-induced ROS production as revealed by proteomic analysis. Front. Microbiol. 9:29. doi: 10.3389/fmicb.2018.00029
Yin, Y., Yang, H., Chen, Z., Zheng, J., and Peng, B. (2022). Vibrio alginolyticus survives from ofloxacin stress by metabolic adjustment. Front. Microbiol. 13:818923. doi: 10.3389/fmicb.2022.818923
Zhang, S., Wang, J., Jiang, M., Xu, D., Peng, B., Peng, X., et al. (2019). Reduced redox-dependent mechanism and glucose-mediated reversal in gentamicin-resistant vibrio alginolyticus. Environ. Microbiol. 21, 4724–4739. doi: 10.1111/1462-2920.14811
Keywords: FNR, metabolomics, alanine-aspartate-glutamate metabolism, glutamate, aminoglycoside antibiotics, the pyruvate cycle, PMF
Citation: Mao L-C, Li S-H, Peng X-X and Li H (2022) Global transcriptional regulator FNR regulates the pyruvate cycle and proton motive force to play a role in aminoglycosides resistance of Edwardsiella tarda. Front. Microbiol. 13:1003586. doi: 10.3389/fmicb.2022.1003586
Edited by:
Xiangmin Lin, Fujian Agriculture and Forestry University, ChinaCopyright © 2022 Mao, Li, Peng and Li. This is an open-access article distributed under the terms of the Creative Commons Attribution License (CC BY). The use, distribution or reproduction in other forums is permitted, provided the original author(s) and the copyright owner(s) are credited and that the original publication in this journal is cited, in accordance with accepted academic practice. No use, distribution or reproduction is permitted which does not comply with these terms.
*Correspondence: Hui Li, bGlodWkzMkBzeXN1LmVkdS5jbg==
†These authors have contributed equally to this work