- 1Canadian Food Inspection Agency, Dartmouth, NS, Canada
- 2Public Health Agency of Canada, Winnipeg, MB, Canada
- 3Canadian Food Inspection Agency, Ottawa, ON, Canada
- 4Canadian Food Inspection Agency, Lethbridge, AB, Canada
- 5Agriculture and Agri-Food Canada, Lethbridge, AB, Canada
- 6Environment and Climate Change Canada, Ottawa, ON, Canada
The increasing prevalence of antimicrobial resistance (AMR) in Campylobacter spp. is a global concern. This study evaluated the use of whole-genome sequencing (WGS) to predict AMR in Campylobacter jejuni and C. coli. A panel of 271 isolates recovered from Canadian poultry was used to compare AMR genotype to antimicrobial susceptibility testing (AST) results (azithromycin, ciprofloxacin, erythromycin, gentamicin, tetracycline, florfenicol, nalidixic acid, telithromycin, and clindamycin). The presence of antibiotic resistance genes (ARGs) was determined for each isolate using five computational approaches to evaluate the effect of: ARG screening software, input data (i.e., raw reads, draft genome assemblies), genome coverage and genome assembly software. Overall, concordance between the genotype and phenotype was influenced by the computational pipelines, level of genome coverage and the type of ARG but not by input data. For example, three of the pipelines showed a 99% agreement between detection of a tet(O) gene and tetracycline resistance, whereas agreement between the detection of tet(O) and TET resistance was 98 and 93% for two pipelines. Overall, higher levels of genome coverage were needed to reliably detect some ARGs; for example, at 15X coverage a tet(O) gene was detected in >70% of the genomes, compared to <60% of the genomes for bla(OXA). No genes associated with florfenicol or gentamicin resistance were found in the set of strains included in this study, consistent with AST results. Macrolide and fluoroquinolone resistance was associated 100% with mutations in the 23S rRNA (A2075G) and gyrA (T86I) genes, respectively. A lower association between a A2075G 23S rRNA gene mutation and resistance to clindamycin and telithromycin (92.8 and 78.6%, respectively) was found. While WGS is an effective approach to predicting AMR in Campylobacter, this study demonstrated the impact that computational pipelines, genome coverage and the genes can have on the reliable identification of an AMR genotype.
Introduction
Antimicrobial resistance (AMR) is an increasing global concern to public health, as resistance to common antimicrobials is making it more difficult or impossible to effectively treat human infectious diseases (World Health Organization, 2014). Development of AMR is a natural process in bacteria; occurring through spontaneous mutations in chromosomal genes or by horizontal transfer of genes from one bacteria to another. Acquisition of antibiotic resistance through both point mutations and transfer of antibiotic resistance genes (ARGs) can result in strains with multidrug resistances, leading to very limited treatment options. The emergence or increased prevalence of AMR to critically important antibiotics for human health in the bacterial population has been associated with the unnecessary use of antimicrobial drugs (e.g., over-prescribing in human and animal health, use of antimicrobials for growth-promotion or prophylaxis in animal health) (Inglis et al., 2005; Government of Canada, 2018). In recent years, the need to address increasing AMR in bacterial pathogens has become a global priority (World Health Organization, 2017a,b; Haldenby et al., 2020). This has led to increased surveillance and a reduction in the use of antibiotics, particularly those highly important to human health.
Campylobacter spp. are currently the leading cause of food-borne bacterial illnesses in Canada (Government of Canada, 2020b), the United States (Tack et al., 2019), Europe (European Centre for Disease Prevention and Control, 2019), and in many other countries worldwide (Kaakoush et al., 2015). In general, Campylobacter jejuni is responsible for >90% of reported cases of campylobacteriosis, followed by C. coli (Public Health Agency of Canada, 2018), and together they can represent >99% of the reported illnesses. Campylobacteriosis is typically self-limiting and often characterized by diarrhea, fever and abdominal pain (Skirrow, 1990). In some cases, however, infections by C. jejuni can be severe, leading to hospitalization, serious post-infection sequelae such as Guillain-Barré Syndrome (GBS), which results in limb weakness and, in rare cases, total paralysis and death (Skirrow, 1990; Fitzgerald et al., 2021). Antibiotic treatment is usually only given to patients exhibiting severe forms of the disease, or to high-risk individuals (e.g., neonates, pregnant women, and immunocompromised individuals) (Yang et al., 2019). For Campylobacter spp., there are increasing rates of fluoroquinolone resistance, specifically, ciprofloxacin resistance being observed in many countries throughout the world (World Health Organization, 2017a,b), including Canada (Inglis et al., 2021). In Canada, ciprofloxacin is classified as an antibiotic of “very high importance” by Health Canada, and it is one of the most prescribed antibiotics for the treatment of campylobacteriosis (Agunos et al., 2013; Inglis et al., 2020). The reason for an increasing prevalence of fluoroquinolone resistance is not known; however, with more extensive surveillance of AMR in Campylobacter, potential routes of transmission could become more apparent [reviewed in Sproston et al. (2018)].
Traditional methods for identifying AMR in bacteria include antimicrobial susceptibility testing (AST) via disk diffusion, E-test and broth/agar dilution assays. However, as these methods are often time-consuming and laborious, it can be difficult to undertake large scale studies and surveillance programs (Azrad et al., 2018). A relatively recent alternative to these methods is to use whole-genome sequencing (WGS) to screen for the presence of known genetic markers of AMR (Zhao et al., 2016; Whitehouse et al., 2018; Feldgarden et al., 2019; Marotta et al., 2019). Decreasing costs for WGS has led to widespread use of this technology for surveillance and routine testing, in addition to outbreak investigations, as the need for multiple tests can be eliminated when results are inferred from WGS data (e.g., AMR, isolate subtyping, virulence, etc.) (Carrillo et al., 2019). Although the effectiveness of this approach has been previously demonstrated (Page et al., 2015; Zhao et al., 2016; Whitehouse et al., 2018; Feldgarden et al., 2019; Elhadidy et al., 2020; Painset et al., 2020; Rokney et al., 2020; Dahl et al., 2021), to our knowledge, this is the first study that also investigated the impact of bioinformatics tools (e.g., ARG screening and genome assembly software) and level of genome coverage on the ability to predict AMR in Campylobacter spp. The objectives of this study were to: (1) compare the WGS-based AMR predictions for C. jejuni and C. coli isolates to the antimicrobial susceptibility profiles and (2) evaluate how the accuracy of the genotype identification is affected by the choice of computational pipelines and databases used for ARG detection.
Materials and Methods
Isolate Collection
All Campylobacter jejuni and C. coli isolates used in this study were collected between December 2012 and December 2013 as part of the National Microbiological Baseline Study (MBS) conducted by the Canadian Food Inspection Agency in collaboration with industry and government partners (Canadian Food Inspection Agency, 2016). All isolates used in the study were recovered from both federally registered chicken establishments and retail outlets. Antimicrobial susceptibility testing (AST) was conducted as described in below. A subset of 271 isolates (229 C. jejuni and 42 C. coli) was selected for this study (Supplementary Table 1). The panel consisted of 109 isolates that were phenotypically sensitive to azithromycin (AZM), ciprofloxacin (CIP), erythromycin (ERY), gentamicin (GEN), tetracycline (TET), florfenicol (FLR), nalidixic acid (NAL), telithromycin (TEL), and clindamycin (CLI) and 162 isolates that were resistant to at least one of these antibiotics. Although none of the isolates used in this panel were phenotypically resistant to telithromycin, florfenicol, or gentamicin, the panel did include 37 isolates with an intermediate level of resistance to telithromycin (Supplementary Table 1).
Antimicrobial Susceptibility Testing
In the case of disagreements between the AST profile and the WGS-derived profile, the AST profile of the isolate was verified as described in Dramé et al. (2020). Briefly, the minimum inhibitory concentration (MIC) for each of the nine antibiotics was determined by the broth dilution method in a microtitre plate format (Public Health Agency of Canada, 2015) and antibiotic susceptibility breakpoints were as described in Dramé et al. (2020) (Supplementary Table 1).
The AST was verified for 13 isolates by broth dilution, based on the methods described by the Public Health Agency of Canada (2015) and National Antimicrobial Resistance Monitoring System (2016) (Supplementary Table 2). Briefly, isolates were streaked for purity on Brucella agar from frozen stocks stored in Brucella broth (Oxoid) with 20% glycerol at −80°C. Isolates were cultured on Mueller Hinton agar (BD Difco) with 5% sheep blood (Cedarlane) at 42°C for 24 h in a microaerobic atmosphere. Cell were harvested from agar plates and cell density was to adjusted to a 0.5 McFarland standard in cation-adjusted Mueller Hinton broth with TES buffer (CAMHB+TES) (Thermo Scientific) using a DensiCHEK Plus instrument (bioMérieux). The cell suspension was then diluted 1:100 in CAMHB+TES with sheep blood (Thermo Scientific) and used as the inoculum for a broth dilution assay. The range of antibiotic concentration tested were as described by Public Health Agency of Canada (2015). Cultures were incubated in the microaerobic atmosphere at 42°C for 24 h. The minimum inhibitory concentration (MIC) was the lowest concentration at which no growth occurred, break-points for each antimicrobial were as described by Public Health Agency of Canada (2015) (Supplementary Table 1).
Whole-Genome Sequencing and Genome Assembly
Isolates were grown from frozen glycerol stocks by streaking for single colonies on 5% horse blood agar. Cultures were incubated for 24 to 48 h at 42°C in microaerobic conditions. DNA was extracted from single colonies using the EZ1 DNA tissue kit (Qiagen) according to the manufacturer’s instructions. Genomic DNA was quantified using the Quant-it High-Sensitivity DNA Assay Kit (Life Technologies Inc., Burlington, ON, United States) and DNA libraries were prepared from 1 ng of genomic DNA with Illumina Nextera XT DNA sample preparation kit (Illumina, Inc., San Diego, CA, United States) according to manufacturers’ instructions. Whole-genome sequencing was performed on the Illumina MiSeq platform (Illumina Inc.), using a 600 cycle MiSeq reagent kit (v3) generating 2 × 300 bp paired-end reads.
Basic metrics of FASTQ-formatted sequencing reads were determined with FastQC version 0.11.6 (Andrews, 2010). Contamination was detected in sequencing reads using ConFindr (Low et al., 2019). Quality and adapter trimming of reads was performed with the BBduk script from the BBTools suite version 37.78 (Bushnell, 2014) with the following parameters: trim quality of 20, and remove reads below 50 bp long. Error correction was performed using tadpole version 37.78 (Bushnell, 2014) in “correct” mode with default parameters. Trimmed and corrected reads were assembled using SKESA v2.3.0 (Souvorov et al., 2018) and SPAdes v3.13.0 (Bankevich et al., 2012) with shovill 1.0.41. Pilon version 1.2.2 (Walker et al., 2014) was used to automatically improve assembly, and assembly metrics were calculated with a custom Python script2, and Qualimap version 2.2.2 (Okonechnikov et al., 2016). Contigs shorter than 500 bp were removed from assemblies. Sequencing was repeated for strains with poor assembly metrics (e.g., low N50, coverage) or when contamination was detected.
Nucleotide Sequence Accession Numbers
Raw data have been deposited at DDBJ/EMBL/GenBank under BioProject PRJNA600010. The accession numbers and strain descriptions are listed in the Supplementary Table 1.
Identification of Antimicrobial Resistance Markers in Raw Reads and Assembled Genomes
Identification of antibiotic resistance genes (ARGs) in the raw reads and draft genome assemblies (contig files) of each isolate was completed using the bioinformatics tools and databases listed in Table 1. Genes were deemed to be present based on a minimum sequence identity of 90%. Currently, PointFinder is unable to detect the gyrA gene of C. coli and at the time analysis the program was unable to reliably detect mutations in the 23S rRNA gene. As such, known point mutations in these two genes were screened for manually. Point mutations conferring AMR in the gyrA and the 23S rRNA genes were identified as follows. The gyrA and 23S rRNA gene sequences were obtained from the assemblies using GeneSeekr3 or from the raw reads using Sipprverse (Lambert et al., 2015; Table 1). Reference sequences for the target genes were from C. jejuni NCTC 11168 (accession number AL111168) and C. coli RM 4661 (accession number CP007181). Query gene sequences were identified by GeneSeekr through BLASTn (Altschul et al., 1990) sequence comparisons against the reference genes, and enabling the “–fasta_output” option. Sipprverse mapping of raw FASTQ reads to reference gene sequences with a kmer size of 11 and soft-clips enabled, allowed the extraction of the gene sequences. Sipprverse read-baiting was performed with BBduk version 37.78 (Bushnell, 2014). FASTQ reads were mapped to reference files using BOWTIE2 version 2.3.5 (Langmead and Salzberg, 2012), with “–local” alignment, “–very-sensitive-local” scoring, and “–all” alignments reported. BAM files were indexed and sorted with samtools version 1.9 (Li et al., 2009). Alignments were parsed from sorted BAM files using pysam version 0.15.24. Sequence alignments of reference and query genes were done using Geneious Prime (version 2019.2) with nucleotide position numbering of the 23S rRNA gene based on the C. jejuni NCTC 11168 reference sequence. Mutations in the gyrA gene (amino acid position 86, Thr to Ile) which confer resistance to quinolone and the 23S rRNA gene (positions 2074, A to T, and 2075, A to G), which confer resistance to macrolides/lincosamides/ketolides, were identified.
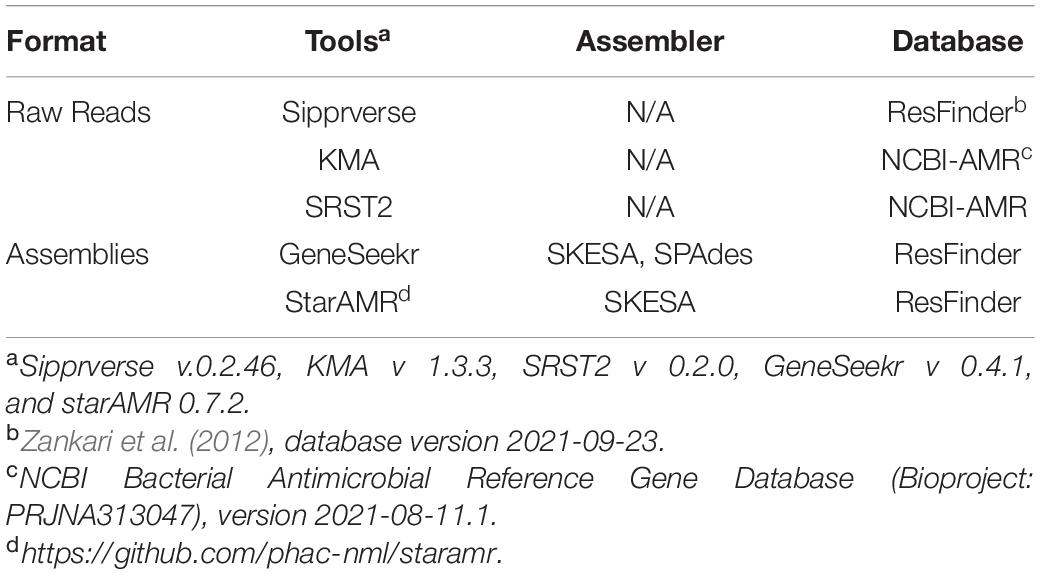
Table 1. Bioinformatics tools used to identify antimicrobial resistance genes from assemblies and WGS raw reads.
For isolates with an identified 23S rRNA gene mutation (n = 42), sequences of cmeR, cmeABC operon, L4 (rspD), and L22 (rspV) were investigated for potential mutations relative to reference sequences from C. jejuni and C. coli. In addition, the presence of base substitutions (relative to C. jejuni NCTC 11168) in the CmeR binding site identified by Lin et al. (2005) were also investigated. Sequences for the genes of interest, including the tet(O) gene for isolates carrying the gene, were obtained using Sipprverse and aligned against reference sequences using Geneious (as described above). Isolates carrying a 23S rRNA gene mutation were screened for additional AMR markers using the Comprehensive Antibiotic Resistance Database (CARD) (Alcock et al., 2020).
To evaluate the accuracy of using WGS data to predict AMR in Campylobacter the following calculations were made using the phenotype profile as the reference method; overall association ([(a+d)/(a+b+c+d)]x100)), sensitivity ([a/(a+c)] × 100), specificity ([d/(b+d)] × 100), positive predictive value (PPV) ([a/(a+b)] × 100), and negative predictive value (NPV) ([d/(c+d)] × 100), where a = true positives, b = false positives, c = false negatives, and d = true negatives.
Estimation of the Minimum Read Coverage Required for Detection of ARG
An estimate of the average genome coverage needed to reliably detect an ARG was determined by screening at increasing levels of read coverage. For each isolate, the raw reads were randomly sampled to genome coverage levels of 1, 2.5, 5, 10, 15, 20, 25, and 30X, using the reformat.sh script (version 37.61) provided with the BBMap suite (Bushnell, 2014). Each set of subsampled raw reads was then screened for ARGs using sipprverse (v 0.0.76) with the ResFinder database (2019-04-26), and SRST2 (v 0.2.0) (Inouye et al., 2014), and KMA (v 1.3.3) (Clausen et al., 2018) with the NCBI-AMR database (2019-04-29.1) (Table 1). For all three tools, default settings were used, with a minimum gene coverage was set to 90%. For each isolate, at each genome coverage level, the subsampling and ARG screening was repeated 100X. The expected ARG profile of each isolate was determined by running each of the tools against the raw reads without subsampling. A false positive result was defined as an ARG detected in the subsampled raw reads but not detected using all available raw reads.
Results
Concordance Between AST and WGS-Based Predictions
Overall, there was a high concordance between the phenotypic susceptibility and the corresponding genotype (96.3–100%) (Table 2). Of the 271 isolates tested, discrepancies were observed in 13 isolates which were retested using the broth dilution method (Supplementary Table 2). Most of the discrepancies were due to discordance between the CLI and/or TEL genotype and the reported phenotype, i.e., presence or absence of the A2075G 23S rRNA gene mutation but lack of the expected susceptibility profile (Supplementary Table 2). Repeat testing led to the amendment of AST profiles for three of the isolates. Isolates CJ-MBS3796A and CC-MBS0767R were amended as being susceptible to CLI and CJ-MBS3139A was amended to being susceptible to AZM, ERY, TEL, and CLI (Supplementary Table 2). The amended phenotypes were used in subsequent analyses. The remaining isolates which were phenotypically susceptible to CLI and/or TEL but carried the A2075G 23S rRNA gene mutation, were confirmed as being susceptible to the antibiotic.
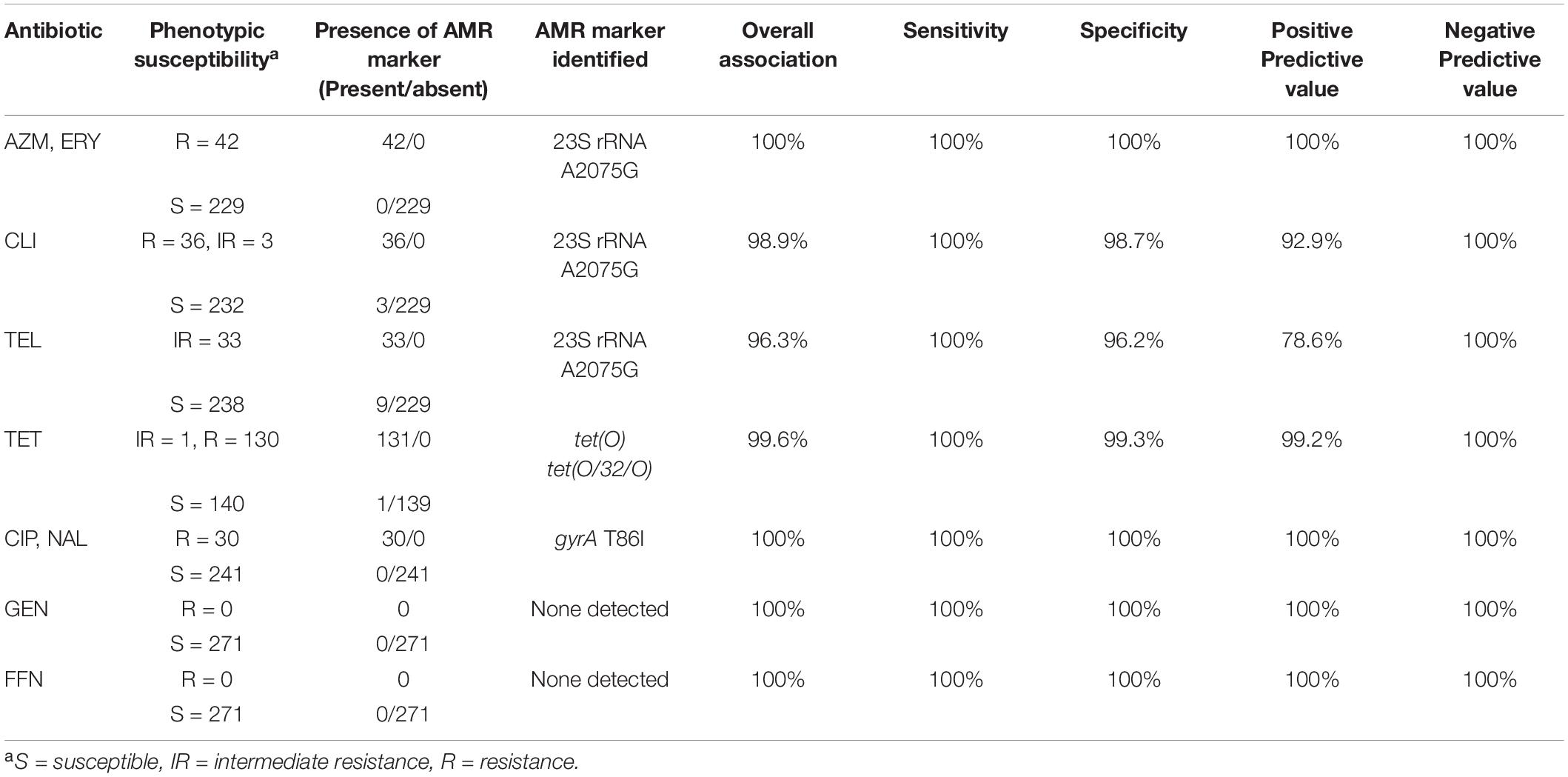
Table 2. Concordance of C. jejuni and C. coli resistance phenotypes to resistance genotypes predicted using GeneSeekr and manual alignment.
There was a 99.6% association between tetracycline resistance and the detection of a tet(O) determinant. The only discordance was a single isolate (CC-MBS7487A), in which a tet(O) gene was detected by all of the pipelines but the isolate was susceptible to TET (Supplementary Table 2). The sequences of the tet(O) gene from this isolate and all other isolates positive for a tet(O) gene (n = 128) were aligned against a tet(O) reference sequence (accession number M18896). The tet(O) sequence of CC-MBS7487A was the only sequence to have a substitution at nucleotide position 1609 (G → T) which resulted in a premature stop codon at amino acid residue 537.
Point mutation in the gyrA (T86I) or the 23S rRNA (A2075G) gene was found to correspond 100% to phenotypic resistance to quinolones (CIP and NAL) and macrolides (AZI and ERY), respectively (Table 2). In contrast, there was a lower association between a A2075G 23S rRNA gene mutation and increased resistance to TEL and CLI, with an overall 96.3 and 98.9% association between the predicted AMR and its phenotype for TEL and CLI, respectively. For both TEL and CLI, the ability of WGS to accurately predict sensitivity to each antibiotic was 100%, (i.e., isolates that do not carry a A2075G 23S rRNA gene mutation always corresponded to a susceptible phenotype). In contrast, the PPV (i.e., the accuracy of detecting a mutation and the isolate being resistant to either TEL or CLI) was only 92.9 and 78.6% for CLI and TEL, respectively.
No genes currently known to confer resistance to gentamycin or florfenicol were observed in any of the isolates, resulting in a 100% concordance between genotype and phenotype.
Effect of Bioinformatics Tools, Genome Assembly and Input Data on AMR Predictions
Overall, the screening software used to identify ARGs (Table 1) had the most impact on both the detection of a gene and the type of gene identified, whereas WGS input (i.e., raw reads or draft assemblies) did not appear to affect the genotype. For example, sipprverse, a software which uses raw reads as input, performed similarly to starAMR and GeneSeekr, software which use draft assemblies as input. While all three pipelines detected the same number of tetracycline resistance genes, there were discrepancies in the detection of two beta-lactam resistance genes; two blaOXA genes were not detected by GeneSeekr using SKESA assemblies but were detected in the SPAdes assemblies and by Sipprverse. An investigation into the SKESA assemblies of CJ-MBS1118R and CJ-MBS1595R found that the blaOXA gene had not assembled (CJ-MBS1118R) or had only partially assembled (CJ-MBS1595R).
In contrast, KMA, which uses raw reads as WGS input, detected the fewest isolates carrying tet(O) and blaOXA, with 14 fewer tet(O) genes detected than the other pipelines. The pipelines also differed in the detection of genes associated with resistance to various aminoglycosides, as KMA and SRST2 were the only pipelines to detect the sat4 gene while GeneSeekr detected four additional isolates carrying the aadE gene (Table 3). The failure to detect a sat4 gene by sipprverse was due at least in part to the ARG not found in the ResFinder database. The association between phenotypic resistance and the detection of genes for resistance to beta-lactam or aminoglycosides other than gentamycin was not verified in this study. While all of the pipelines detected tetracycline and beta-lactam resistance [i.e., tet(O) and blaOXA, respectively], the ability to distinguish between alleles also varied among the pipelines (Table 3). For example, not all pipelines reported a predicted class of blaOXA, whereas KMA and SRST2 were the only pipelines that did not identify the mosaic allele tet(O/32/O), which was found in four isolates. With the exception of two blaOXA genes, assemblies generated using SKESA and SPAdes reported the same genotype when screened with the same software (i.e., GeneSeekr).
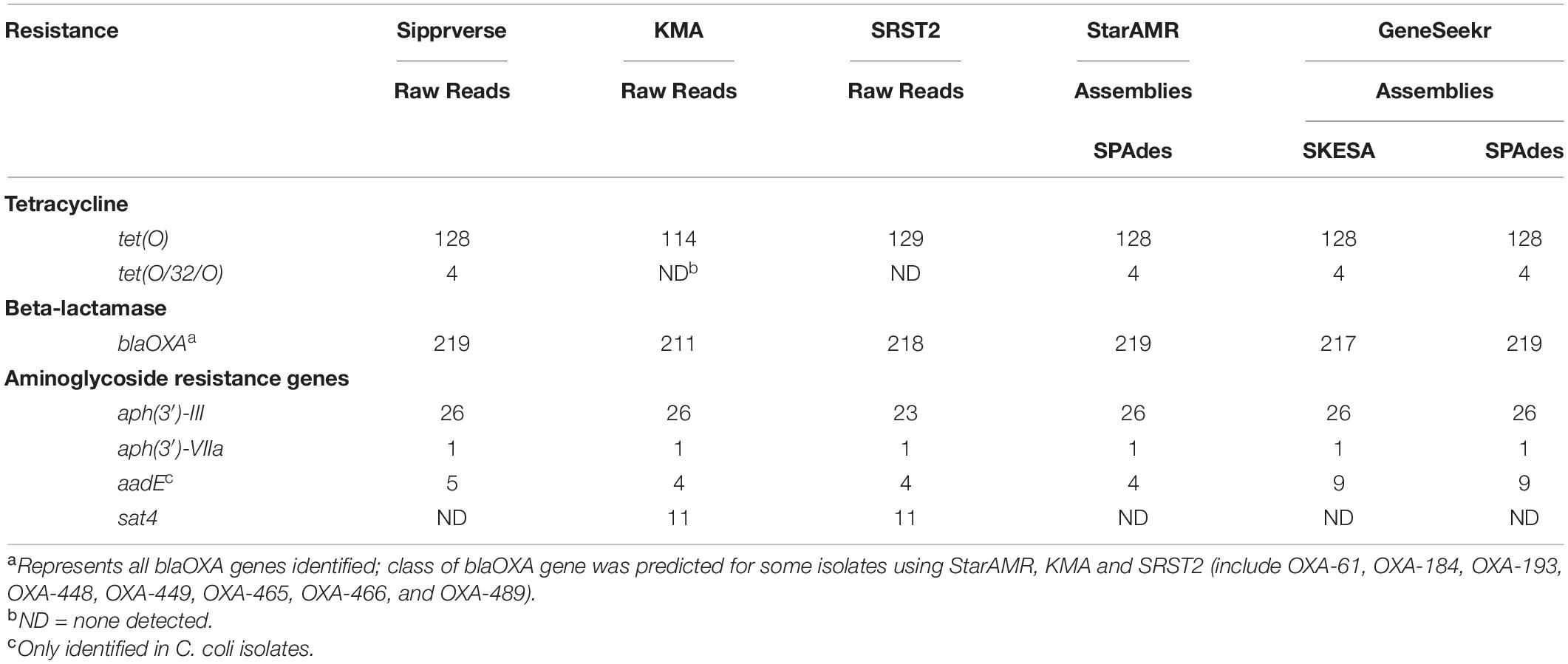
Table 3. A comparison of AMR gene detection pipelines (n = 5) using WGS raw reads and/or assemblies (n = 271).
Effect of Genome Coverage on AMR Predictions
The impact of genome coverage on the ability to reliably detect ARGs from raw reads was evaluated using sipprverse, KMA and SRST2. Detection of an ARG was found to be dependent on the screening software, the ARG and the level of genome coverage (Figure 1). Although KMA and SRST2 could detect blaOXA genes at lower coverage levels compared to sipprverse, all three pipelines failed to detect the gene 100% of the time for a proportion of isolates using 30X average genome coverage. For example, SRST2 was able to detect the gene in >90% of the subsamples for 89.5% of the isolates (n = 194/217) at 30X genome coverage and the gene was missed in only one isolate (0.5%) where it could not be detected in at least 50% of the subsample. In contrast, at 30X genome coverage, the blaOXA gene was detected in <50% of the replicates in 3.8% (n = 8/217) and 5.5% (n = 12/217) of isolates using KMA and sipprverse, respectively (Figure 2).
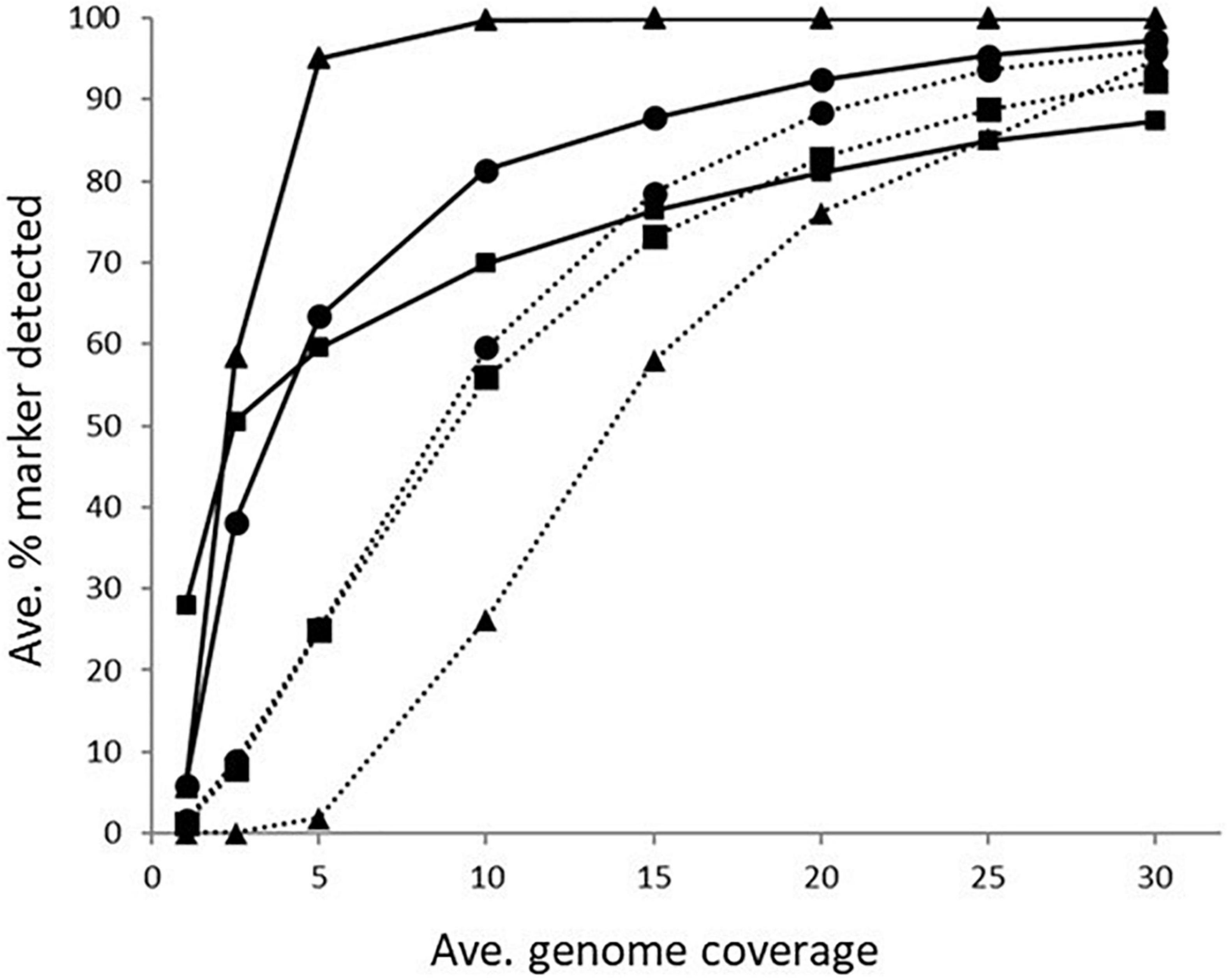
Figure 1. The average percentage of subsampled reads in which blaOXA gene (dotted line) and tet(O) gene (solid line) was detected in the WGS data at increasing raw read coverage levels. Data points represent the average proportion of subsamples in which a gene was observed that was also observed when each sample was screened using unfiltered raw reads. Symbols represent ■KMA, •SRST2, and ▲sipprverse.
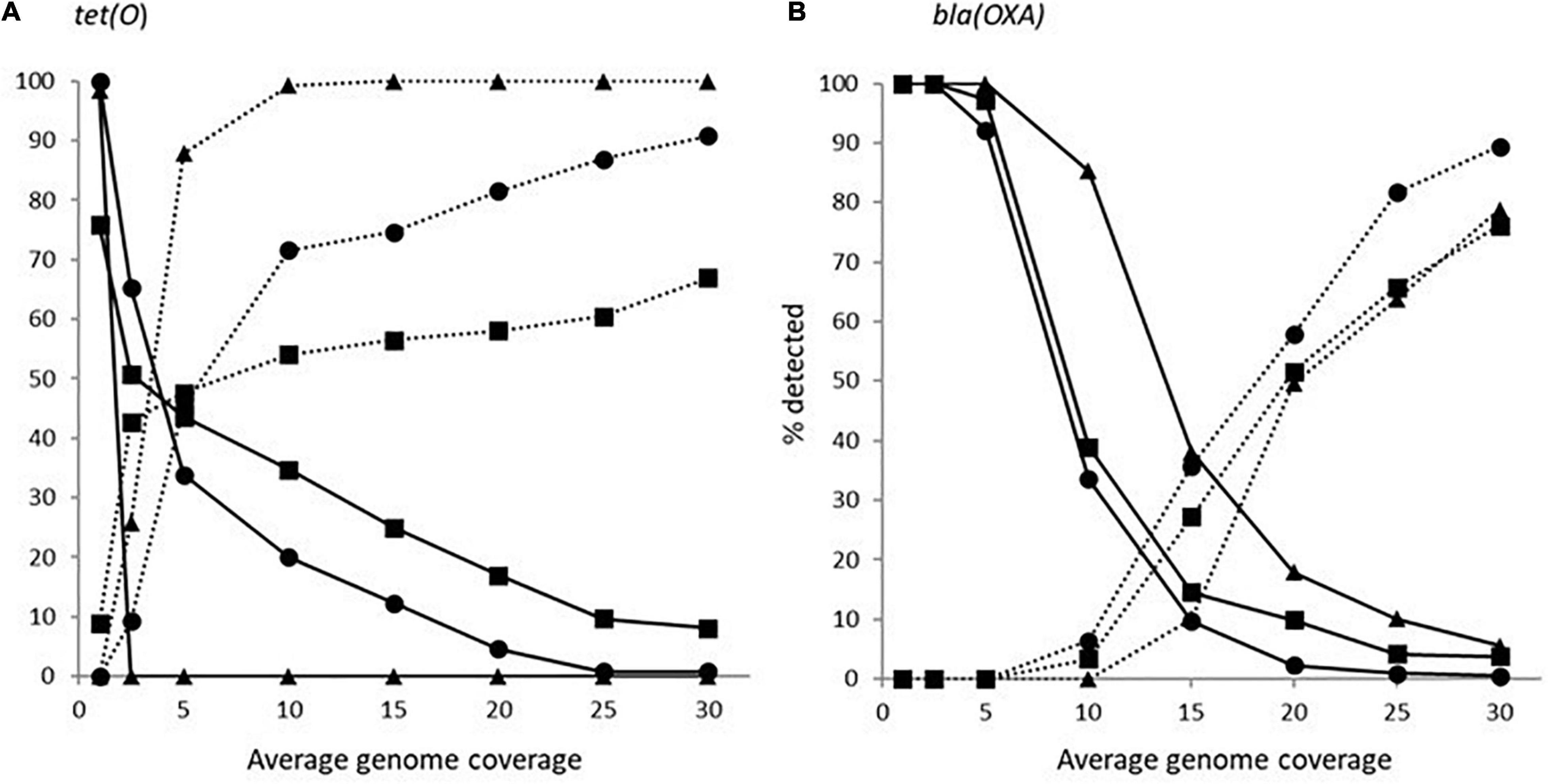
Figure 2. The proportion of Campylobacter isolates found to have a tet(O) (panel A) or a beta-lactamase (panel B) marker that had a <50% probability of being detected (solid line) and >90% of being detected (dotted line) using the detection tools KMA (■), SRTS2 (•), and sipprverse (▲) for each level of genome coverage.
In comparison to blaOXA, a tet(O) or tet(O/32/O) gene was not only more likely to be detected but it could be detected using significantly lower genome coverage (Figure 1). Of the three software, sipprverse correctly detected a tet(O) gene determinant in the most isolates, using the least amount of genome coverage. For example, there was a 95% likelihood of detecting a tet(O) gene at only 5X coverage, compared to a likelihood of 60 and 63% using KMA and SRST2, respectively (Figure 1). For example, using KMA false positives were identified in an increasing number of subsamples as genome coverages increased for three isolates, i.e., gene detected in the subsample but not using all available reads. Although the presence of a tet(O) gene was not detected using KMA and all available reads, all three isolates were phenotypically tetracycline resistant and a tet(O) gene was detected using other pipelines. Using sipprverse, one isolate was reported as a false positive in a proportion of the subsampled reads, identified in up to 20% of the subsamples between one times and 20X coverage. The false positive was not detected by any other pipeline (Table 1) and further investigation using both the assemblies and raw reads suggests the gene is non-functional. The result of a BLAST search of the gene indicates this was likely due to the insertion of a transposon, which corresponded with the isolate being phenotypically susceptible to TET.
Discussion
In this study, the ability to accurately predict antimicrobial susceptibility using WGS data was evaluated using a panel of 271 C. jejuni and C. coli isolates recovered from broiler chickens collected in abattoirs and food retail outlets across Canada. While all of the pipelines used in our study were able to identify ARGs associated with a variety of antibiotics (e.g., beta-lactams and tetracycline), the primary focus of this study was on the detection of ARGs and point mutations conferring resistance to the nine antibiotics currently monitored by the national AMR programs in Canada (Government of Canada, 2020a) and in the United States (National Antimicrobial Resistance Monitoring System, 2021).
In addition to the numerous bioinformatics tools that have been developed to screen WGS data for the presence of ARGs (using genome assemblies and/or raw reads), several databases are available that these tools can utilize in order to identify ARGs of interest, leading to a wide range of choices for identifying ARGs in WGS data (Hendriksen et al., 2019; Doyle et al., 2020). Here we have shown that predicting an AMR phenotype from WGS data was dependant on the choice of screening tool, the level of genome coverage and on the specific ARG itself. Of the bioinformatics tools we used, GeneSeekr, sipprverse, and starAMR were found to produce similar results and generated the most accurate profiles with regards to the selected antibiotics. While the completeness of an AMR database used to screen the genome is important for identifying known resistance markers, this was not a significant factor in our study as genes conferring resistance to the priority antibiotics were well represented in both the ResFinder database (Zankari et al., 2012) and the NCBI Bacterial Antimicrobial Resistance Reference Gene Database (Feldgarden et al., 2019). It may, however, explain why KMA and SRST2 detected the presence of an additional sat4 gene using the more comprehensive NCBI database. Moreover, we observed that software using the same database occasionally produced different results suggesting that the choice of screening tool has a greater impact on results than the choice of database.
Whitehouse et al. (2018) suggested a potential issue with using genome assemblies instead of the raw reads, would be that the software could report the isolate as susceptible when an ARG was split across two contigs. Here, we have shown using two different assembly software, SKESA and SPAdes, that discrepancies between the AMR profiles generated were more likely to be influenced by the screening software and the gene, than by using assemblies generated by using different assembly software. Overall, genotypes generated by GeneSeekr using SKESA and SPAdes assemblies only differed by two blaOXA genes. This due to the gene not fully assembling using SKESA, rather than the gene assembling on two contigs. In contrast, it is apparent that the screening tools have considerable impact when using raw reads as the WGS input. For example, of the three pipelines, KMA detected 18 fewer tet(O) genes and seven fewer blaOXA genes. While the accuracy of detecting aminoglycoside resistance genes or detecting a blaOXA gene and predicting the allelic type was not assessed in our study, these results highlight how the choice of screening tool can have a significant impact on the genotype determination.
In our study, we also examined how genome coverage can influence the detection of various ARGs and estimated the minimum level of coverage needed to accurately predict AMR. As expected, the likelihood of detecting an ARG increased with increasing genome coverage. However, each of the three software used (sipprverse, KMA, and SRST2) to map raw reads to the AMR databases was affected differently by the level of coverage in an ARG-specific manner. For example, sipprverse was able to consistently and accurately identify a tet(O) marker at very low coverage levels, with an almost 100% detection rate at 5X coverage, whereas using 30X coverage, KMA could not detect the gene in 50% of the subsamples in nearly 10% of isolates. The considerably higher level of genome coverage required by KMA may explain why the pipeline failed to predict tetracycline resistance in 19 isolates that were correctly identified by sipprverse. In contrast, the consistent detection of a blaOXA gene required considerably more genome coverage than for a tet(O) gene for all the pipelines. In contrast, a similar study performed using KMA with Salmonella enterica indicated that ARGs could be accurately identified for more genes with 20 coverage (Cooper et al., 2020). These findings demonstrate that the genetic sequence of a gene also has a significant impact on its detectability, possibly due to the ability of the software algorithms to correctly map the reads to a gene with difficult regions to map (e.g., repetitive regions, multiple copies) and to reach the level of coverage needed to be able to distinguish it from other closely related sequences. This may explain why the use of raw reads as WGS input resulted in considerably more variability in the AMR genotypes between screening software than was observed between software which use draft assemblies as WGS input.
Overall, while it is more cost and time effective to be able to generate profiles with a lower level of coverage, it comes with an increased risk of falsely detecting or not detecting an ARG. For example, in up to 20% of the subsampled reads for one isolate, sipprverse identified a tet(O) gene that was non-functional (i.e., insertion of transposon). By ensuring an adequate level of genome coverage and by maintaining stringent cut-off values for detection, the likelihood of a false-positive ARG identification, could be reduced. Due influence that individual genes can play on accurate AMR predictions, our results suggest that the minimum amount of coverage required for these pipelines will largely depend on the organism and the genes of interest.
Consistent with Whitehouse et al. (2018) and Zhao et al. (2016) we observed a strong concordance between the genotype and phenotypic resistance to macrolides (i.e., AZM and ERY), quinolones (i.e., NAL and CIP), and tetracycline. All of the isolates resistant to fluoroquinolone carried a T86I mutation in the gyrA gene, consistent with previous studies (Zhao et al., 2016; Whitehouse et al., 2018; Painset et al., 2020), additional substitutions were observed in the gene but were not further investigated. However, a recent study by Webb et al. (2018) identified other base substitutions in the gyrA gene which were associated with isolates having a higher level of resistance to ciprofloxacin. In our study, all isolates phenotypically resistant to macrolides were found to have an A2075G mutation in the 23S rRNA gene. In Campylobacter spp., the A2075G is the most commonly identified mutation among macrolide-resistant isolates, followed by an A2074T mutation (Bolinger and Kathariou, 2017; Whitehouse et al., 2018). Recently, Campylobacter strains carrying an erm(B) marker, another macrolide marker, have also been reported although, this is a rare occurrence (Wang et al., 2014). It is often suggested that these two point mutations also confer resistance to lincosamides and ketolides in Campylobacter spp.; however, as observed our study and previous studies, the association between these mutations and resistance to lincosamides and ketolides is not conclusive (Whitehouse et al., 2018; Feldgarden et al., 2019). In our study, isolates that did not have an A2075G mutation in the 23S rRNA gene were phenotypically sensitive to both TEL and CLI as was predicted (i.e., negative predictive value), however, the predictability of having a mutation and being resistant to clindamycin or telithromycin was only 92.9 and 78.6%, respectively (i.e., positive predictive value). These rates are similar to those of Whitehouse et al. (2018) who reported rates of 93 and 63% for clindamycin and telithromycin, respectively. Suggested reasons for differences in resistance between strains include insertions, deletions or base substitutions in the L22, L4, cmeABC (an efflux pump associated with C. jejuni), cmeR (regulator of cmeABC) and the binding site of CmeR (Lin et al., 2005). However, we were unable to identify mutations in any of these genes or sites which were specific to either resistant or susceptible isolates, as polymorphisms in the sequences were observed in both resistant and susceptible isolates (data not shown). Isolates with an A2075G mutation in the 23S rRNA gene were screened for additional AMR markers using CARD (Alcock et al., 2020), which screens for AMR markers known to cause resistance and genes/markers that may be potential markers of resistance. We did not find an association between any known or suspected AMR marker and resistance to TEL or CLI that could possibly explain why a small number of isolates carry a A2075G mutation in the 23S rRNA gene but are not resistant to either TEL or CLI. The lower accuracy of predicting resistance to lincosamides and ketolides highlights a limitation due to gaps in our understanding of the resistance mechanisms of Campylobacter that are reflected in the reference ARG databases, resulting in a risk of either over- or under-estimating the prevalence of AMR in a population.
This study demonstrates the significant impact that the choice of ARG screening software, genome coverage and the gene targets themselves have on the accurate identification of an AMR genotype. Therefore, these factors need to be taken into consideration when screening tools are being developed or are selected for use in a study and/or surveillance pipeline. The genomic data generated for this study can be used as a verified benchmark dataset for assessment of the performance of new computational tools for predicting AMR in C. jejuni and C. coli. In fact, the genotypes generated by the computational pipelines were in some cases more accurate than those generated by phenotypic susceptibility testing. While the screening tools influence our ability to detect known AMR markers, the accuracy of a genotype is also limited by the comprehensiveness of the reference database. It is important that we continue to study how Campylobacter gains resistance to antimicrobials and to understand the mechanisms for AMR in Campylobacter spp.
Data Availability Statement
Raw data have been deposited at https://www.ncbi.nlm.nih.gov/bioproject/PRJNA600010. The accession numbers and strain descriptions are listed in the Supplementary Table 1.
Author Contributions
LH and CC were responsible for conception and design. LH was responsible for data analysis and preparation of the manuscript. ET, DI, and SM were responsible for all sequencing related to this project, using funding acquired by ET and DI. AK was responsible for bioinformatic support. DL provided all isolates and associated metadata used in this study. CC and BB was responsible for overseeing the bioinformatic support and sequence data. All authors contributed to manuscript revision, read, and approved the submitted version.
Funding
This work was funded through the Canadian Food Inspection Agency. Funds to whole genome sequence C. jejuni isolates was provided in part by grants from the Public Health Agency of Canada, the Genome Alberta Applied Agricultural Genomic Program (A3GP60), and the Canadian Poultry Research Council (1374 Activity 11). The Canadian Food Inspection Agency approved publication of the manuscript. The funders had no role in study design, data collection and analysis, decision to publish, or preparation of the manuscript.
Conflict of Interest
The authors declare that the research was conducted in the absence of any commercial or financial relationships that could be construed as a potential conflict of interest.
Publisher’s Note
All claims expressed in this article are solely those of the authors and do not necessarily represent those of their affiliated organizations, or those of the publisher, the editors and the reviewers. Any product that may be evaluated in this article, or claim that may be made by its manufacturer, is not guaranteed or endorsed by the publisher.
Acknowledgments
We would like to thank the Canadian Food Inspection Agency - St. Hyacinthe Laboratory for providing us with the isolates used for confirmation of the phenotypic susceptibility and to thank Andrew Low and Ashley Cooper for their assistance with bioinformatics analyses.
Supplementary Material
The Supplementary Material for this article can be found online at: https://www.frontiersin.org/articles/10.3389/fmicb.2021.776967/full#supplementary-material
Footnotes
- ^ https://github.com/tseemann/shovill
- ^ https://github.com/OLC-Bioinformatics/COWBAT
- ^ https://github.com/OLC-Bioinformatics/GeneSeekr
- ^ https://github.com/pysam-developers/pysam
References
Agunos, A., Léger, D., Avery, B. P., Parmley, E. J., Deckert, A., Carson, C. A., et al. (2013). Ciprofloxacin-resistant Campylobacter spp. in retail chicken, western Canada. Emerg. Infect. Dis. 19, 1121–1124. doi: 10.3201/eid1907.111417
Alcock, B. P., Raphenya, A. R., Lau, T. T., Tsang, K. K., Bouchard, M., Edalatmand, A., et al. (2020). CARD 2020: antibiotic resistome surveillance with the comprehensive antibiotic resistance database. Nucleic Acids Res. 48, D517–D525. doi: 10.1093/nar/gkz935
Altschul, S. F., Gish, W., Miller, W., Myers, E. W., and Lipman, D. J. (1990). Basic local alignment search tool. J. Mol. Biol. 215, 403–410.
Andrews, S. (2010). FastQC: A Quality Control Tool for High Throughput Sequence Data. Available online at: http://www.bioinformatics.babraham.ac.uk/projects/fastqc (accessed September 1, 2020).
Azrad, M., Tkhawkho, L., Isakovich, N., Nitzan, O., and Peretz, A. (2018). Antimicrobial susceptibility of Campylobacter jejuni and Campylobacter coli: comparison between Etest and a broth dilution method. Ann. Clin. Microbiol. Antimicrob. 17:23. doi: 10.1186/s12941-018-0275-8
Bankevich, A., Nurk, S., Antipov, D., Gurevich, A. A., Dvorkin, M., Kulikov, A. S., et al. (2012). SPAdes: a new genome assembly algorithm and its applications to single-cell sequencing. J. Comput. Biol. 19:22. doi: 10.1089/cmb.2012.0021
Bolinger, H., and Kathariou, S. (2017). The current state of macrolide resistance in Campylobacter spp.: trends and impacts of resistance mechanisms. Appl. Environ. Microbiol. 83:e00416-17.
Bushnell, B. (2014). BBMap: A Fast, Accurate, Splice-Aware Aligner. Berkeley, CA: Lawrence Berkeley National Lab.(LBNL)
Canadian Food Inspection Agency (2016). National Microbiological Baseline Study in Broiler Chicken, December 2012 – December 2013. Available online at: https://www.inspection.gc.ca/DAM/DAM-food-aliments/STAGING/text-texte/chem_testing_report_2012-2013_broiler_chicken_1471382238248_eng.pdf (accessed September 2020).
Carrillo, C. D., Koziol, A., Vary, N., and Blais, B. W. (2019). “Applications of genomics in regulatory food safety testing in Canada,” in New Insight into Brucella Infection Foodborne Diseases, eds M. Ranjbar, M. Nojomi, and M. T. Mascellino (London: InTechOpen).
Clausen, P. T., Aarestrup, F. M., and Lund, O. (2018). Rapid and precise alignment of raw reads against redundant databases with KMA. BMC Bioinformatics 19:307. doi: 10.1186/s12859-018-2336-6
Cooper, A. L., Low, A. J., Koziol, A. G., Thomas, M. C., Leclair, D., Tamber, S., et al. (2020). Systematic evaluation of whole genome sequence-based predictions of Salmonella serotype and antimicrobial resistance. Front. Microbiol. 11:549. doi: 10.3389/fmicb.2020.00549
Dahl, L. G., Joensen, K. G., Østerlund, M. T., Kiil, K., and Nielsen, E. M. (2021). Prediction of antimicrobial resistance in clinical Campylobacter jejuni isolates from whole-genome sequencing data. Eur. J. Clin. Microbiol. Infect. Dis. 40, 673–682.
Doyle, R. M., O’sullivan, D. M., Aller, S. D., Bruchmann, S., Clark, T., Pelegrin, A. C., et al. (2020). Discordant bioinformatic predictions of antimicrobial resistance from whole-genome sequencing data of bacterial isolates: an inter-laboratory study. Microb. Genomics 6:e000335. doi: 10.1099/mgen.0.000335
Dramé, O., Leclair, D., Parmley, E. J., Deckert, A., Ouattara, B., Daignault, D., et al. (2020). Antimicrobial resistance of Campylobacter in broiler chicken along the food chain in Canada. Foodborne Pathog. Dis. 17, 512–520. doi: 10.1089/fpd.2019.2752
Elhadidy, M., Ali, M. M., El-Shibiny, A., Miller, W. G., Elkhatib, W. F., Botteldoorn, N., et al. (2020). Antimicrobial resistance patterns and molecular resistance markers of Campylobacter jejuni isolates from human diarrheal cases. PLoS One 15:e0227833. doi: 10.1371/journal.pone.0227833
European Centre for Disease Prevention and Control (2019). Campylobacteriosis in ECDC. Annual Epidemiological Report for 2017. Stockholm: ECDC.
Feldgarden, M., Brover, V., Haft, D. H., Prasad, A. B., Slotta, D. J., Tolstoy, I., et al. (2019). Validating the AMRFinder tool and resistance gene database by using antimicrobial resistance genotype-phenotype correlations in a collection of isolates. Antimicrob. Agents Chemother. 63:e00483-19. doi: 10.1128/AAC.00483-19
Fitzgerald, C., Pruckler, J., Karlsson, M., Kwan, P., Joseph, L., Caidi, H., et al. (2021). “The Genus Campylobacter,” in Practical Handbook of Microbiology, eds L. H. Green and E. Goldman (Boca Raton: CRC Press), 297–310.
Government of Canada (2018). Canadian Integrated Program for Antimicrobial Resistance Surveillance System (CIPARS) – 2016 Report. Guelph, ON: Public Health Agency of Canada.
Government of Canada (2020b). Notifiable Diseases Online. Available online at: https://diseases.canada.ca/notifiable/ (accessed September, 2020).
Government of Canada (2020a). Canadian Integrated Program for Antimicrobial Resistance Surveillance (CIPARS) 2018: Design and Methods. Ottawa, ON: Public Health Agency of Canada.
Haldenby, S., Bronowski, C., Nelson, C., Kenny, J., Martinez-Rodriguez, C., Chaudhuri, R., et al. (2020). Increasing prevalence of a fluoroquinolone resistance mutation amongst Campylobacter jejuni isolates from four human infectious intestinal disease studies in the United Kingdom. PLoS One 15:e0227535. doi: 10.1371/journal.pone.0227535
Hendriksen, R. S., Bortolaia, V., Tate, H., Tyson, G. H., Aarestrup, F. M., and Mcdermott, P. F. (2019). Using genomics to track global antimicrobial resistance. Front. Public Health 7:242. doi: 10.3389/fpubh.2019.00242
Inglis, G. D., Boras, V. F., and Taboada, E. N. (2020). Rates of fluoroquinolone resistance in domestically acquired Campylobacter jejuni are increasing in people living within a model study location in Canada. Can. J. Microbiol. (Early Release) 67, 37–52. doi: 10.1139/cjm-2020-0146
Inglis, G. D., Mcallister, T. A., Busz, H. W., Yanke, L. J., Morck, D. W., Olson, M. E., et al. (2005). Effects of subtherapeutic administration of antimicrobial agents to beef cattle on the prevalence of antimicrobial resistance in Campylobacter jejuni and Campylobacter hyointestinalis. Appl. Environ. Microbiol. 71, 3872–3881.
Inglis, G. D., Taboada, E. N., and Boras, V. F. (2021). Rates of fluoroquinolone resistance in domestically acquired Campylobacter jejuni are increasing in people living within a model study location in Canada. Can. J. Microbiol. 67, 37–52.
Inouye, M., Dashnow, H., Raven, L.-A., Schultz, M. B., Pope, B. J., Tomita, T., et al. (2014). SRST2: rapid genomic surveillance for public health and hospital microbiology labs. Genome Med. 6:90. doi: 10.1186/s13073-014-0090-6
Kaakoush, N. O., Castaño-Rodríguez, N., Mitchell, H. M., and Man, S. M. (2015). Global epidemiology of Campylobacter infection. J. Clin. Microbiol. Rev. 28, 687–720. doi: 10.1128/CMR.00006-15
Lambert, D., Carrillo, C. D., Koziol, A. G., Manninger, P., and Blais, B. W. (2015). GeneSippr: a rapid whole-genome approach for the identification and characterization of foodborne pathogens such as priority Shiga toxigenic Escherichia coli. PLoS One 10:0122928. doi: 10.1371/journal.pone.0122928
Langmead, B., and Salzberg, S. L. (2012). Fast gapped-read alignment with Bowtie 2. Nat. Methods 9:357. doi: 10.1038/nmeth.1923
Li, H., Handsaker, B., Wysoker, A., Fennell, T., Ruan, J., Homer, N., et al. (2009). The sequence alignment/map format and SAMtools. Bioinformatics 25, 2078–2079. doi: 10.1093/bioinformatics/btp352
Lin, J., Akiba, M., Sahin, O., and Zhang, Q. (2005). CmeR functions as a transcriptional repressor for the multidrug efflux pump CmeABC in Campylobacter jejuni. Antimicrob. Agents Chemother. 49, 1067–1075. doi: 10.1128/AAC.49.3.1067-1075.2005
Low, A. J., Koziol, A. G., Manninger, P. A., Blais, B., and Carrillo, C. D. (2019). ConFindr: rapid detection of intraspecies and cross-species contamination in bacterial whole-genome sequence data. PeerJ 7:e6995. doi: 10.7717/peerj.6995
Marotta, F., Garofolo, G., Di Marcantonio, L., Di Serafino, G., Neri, D., Romantini, R., et al. (2019). Antimicrobial resistance genotypes and phenotypes of Campylobacter jejuni isolated in Italy from humans, birds from wild and urban habitats, and poultry. PLoS One 14:e0223804.
National Antimicrobial Resistance Monitoring System (2021). National Antimicrobial Resistance Monitoring System. Available online at: https://www.cdc.gov/narms/antibiotics-tested.html (accessed July, 2021).
National Antimicrobial Resistance Monitoring System (2016). The National Antimicrobial Resistance Monitoring System: Manual of Laboratory Methods, 3rd Edn. Available online at: https://www.fda.gov/media/101423/download (accessed May, 2019).
Okonechnikov, K., Conesa, A., and García-Alcalde, F. (2016). Qualimap 2: advanced multi-sample quality control for high-throughput sequencing data. Bioinformatics 32, 292–294. doi: 10.1093/bioinformatics/btv566
Page, A. J., Cummins, C. A., Hunt, M., Wong, V. K., Reuter, S., Holden, M. T., et al. (2015). Roary: rapid large-scale prokaryote pan genome analysis. Bioinformatics 31, 3691–3693. doi: 10.1093/bioinformatics/btv421
Painset, A., Day, M., Doumith, M., Rigby, J., Jenkins, C., Grant, K., et al. (2020). Comparison of phenotypic and WGS-derived antimicrobial resistance profiles of Campylobacter jejuni and Campylobacter coli isolated from cases of diarrhoeal disease in England and Wales, 2015–16. J. Antimicrob. Chemother. 75, 883–889. doi: 10.1093/jac/dkz539
Public Health Agency of Canada (2015). Canadian Integrated Program for Antimicrobial Resistance Surveillance (CIPARS) 2013 Annual Report – Chapter 1. Design and Methods. Available online at: http://publications.gc.ca/collections/collection_2015/aspc-phac/HP2-4-2013-1-eng.pdf (accessed September, 2020).
Public Health Agency of Canada (2018). Foodnet Canada Annual Report 2017. Available online at: https://www.canada.ca/content/dam/phac-aspc/documents/services/surveillance/foodnet-canada/publications/FNC-Annual-Report-2017-en.pdf (accessed September, 2020).
Rokney, A., Valinsky, L., Vranckx, K., Feldman, N., Agmon, V., Moran-Gilad, J., et al. (2020). WGS-Based prediction and analysis of antimicrobial resistance in Campylobacter jejuni isolates from Israel. Front. Cell. Infect. Microbiol 10:365.
Souvorov, A., Agarwala, R., and Lipman, D. J. (2018). SKESA: strategic k-mer extension for scrupulous assemblies. Genome Biol. 19:153. doi: 10.1186/s13059-018-1540-z
Sproston, E. L., Wimalarathna, H. M. L., and Sheppard, S. K. (2018). Trends in fluoroquinolone resistance in Campylobacter. Microb. Genomics 4:e000198. doi: 10.1099/mgen.0.000198
Tack, D. M., Marder, E. P., Griffin, P. M., Cieslak, P. R., Dunn, J., Hurd, S., et al. (2019). Preliminary incidence and trends of infections with pathogens transmitted commonly through food—Foodborne Diseases Active Surveillance Network, 10 US sites, 2015–2018. Morb. Mortal Weekly Rep. 68, 369–371. doi: 10.15585/mmwr.mm6917a1
Walker, B. J., Abeel, T., Shea, T., Priest, M., Abouelliel, A., Sakthikumar, S., et al. (2014). Pilon: an integrated tool for comprehensive microbial variant detection and genome assembly improvement. PLoS One 9:e112963. doi: 10.1371/journal.pone.0112963
Wang, Y., Zhang, M., Deng, F., Shen, Z., Wu, C., Zhang, J., et al. (2014). Emergence of multidrug-resistant Campylobacter species isolates with a horizontally acquired rRNA methylase. Antimicrob. Agents Chemother. 58, 5405–5412. doi: 10.1128/AAC.03039-14
Webb, A. L., Selinger, L. B., Taboada, E. N., and Inglis, G. D. (2018). Subtype-specific selection for resistance to fluoroquinolones but not to tetracyclines is evident in Campylobacter jejuni isolates from beef cattle in confined feeding operations in Southern Alberta, Canada. Appl. Environ. Microbiol. 84: e02713-17.
Whitehouse, C. A., Young, S., Li, C., Hsu, C.-H., Martin, G., and Zhao, S. (2018). Use of whole-genome sequencing for Campylobacter surveillance from NARMS retail poultry in the United States in 2015. Food Microbiol. 73, 122–128. doi: 10.1089/fpd.2017.2283
World Health Organization (2014). Antimicrobial Resistance: Global Report on Surveillance 2014. Available online at: https://www.who.int/drugresistance/documents/surveillancereport/en/ (accessed September, 2020).
World Health Organization (2017a). Prioritization of Pathogens to Guide Discovery, Research and Development of New Antibiotics for Drug-Resistant Bacterial Infections, Including Tuberculosis. Available online at: https://www.who.int/medicines/areas/rational_use/prioritization-of-pathogens/en/ (accessed September, 2020).
World Health Organization (2017b). WHO publishes List of Bacteria for Which New Antibiotics are Urgently Needed. Available online at: https://www.who.int/news-room/detail/27-02-2017-who-publishes-list-of-bacteria-for-which-new-antibiotics-are-urgently-needed (accessed September, 2017).
Yang, Y., Feye, K. M., Shi, Z., Pavlidis, H. O., Kogut, M., Ashworth, J. A., et al. (2019). A historical review on antibiotic resistance of foodborne Campylobacter. Front. Microbiol. 10:1509. doi: 10.3389/fmicb.2019.01509
Zankari, E., Hasman, H., Cosentino, S., Vestergaard, M., Rasmussen, S., Lund, O., et al. (2012). Identification of acquired antimicrobial resistance genes. J. Antimicrob. Chemother. 67, 2640–2644. doi: 10.1093/jac/dks261
Keywords: Campylobacter, antimicrobial resistance, whole-genome sequence (WGS), bioinformatic tools, AMR surveillance
Citation: Hodges LM, Taboada EN, Koziol A, Mutschall S, Blais BW, Inglis GD, Leclair D and Carrillo CD (2021) Systematic Evaluation of Whole-Genome Sequencing Based Prediction of Antimicrobial Resistance in Campylobacter jejuni and C. coli. Front. Microbiol. 12:776967. doi: 10.3389/fmicb.2021.776967
Received: 14 September 2021; Accepted: 25 October 2021;
Published: 16 November 2021.
Edited by:
Yuji Morita, Meiji Pharmaceutical University, JapanReviewed by:
José F. Cobo-Díaz, University of León, SpainPhilippe Lehours, Université Bordeaux Segalen, France
Julio Alvarez, VISAVET Health Surveillance Centre (UCM), Spain
Copyright © 2021 Hodges, Taboada, Koziol, Mutschall, Blais, Inglis, Leclair and Carrillo. This is an open-access article distributed under the terms of the Creative Commons Attribution License (CC BY). The use, distribution or reproduction in other forums is permitted, provided the original author(s) and the copyright owner(s) are credited and that the original publication in this journal is cited, in accordance with accepted academic practice. No use, distribution or reproduction is permitted which does not comply with these terms.
*Correspondence: Lisa M. Hodges, Lisa.Hodges@inspection.gc.ca