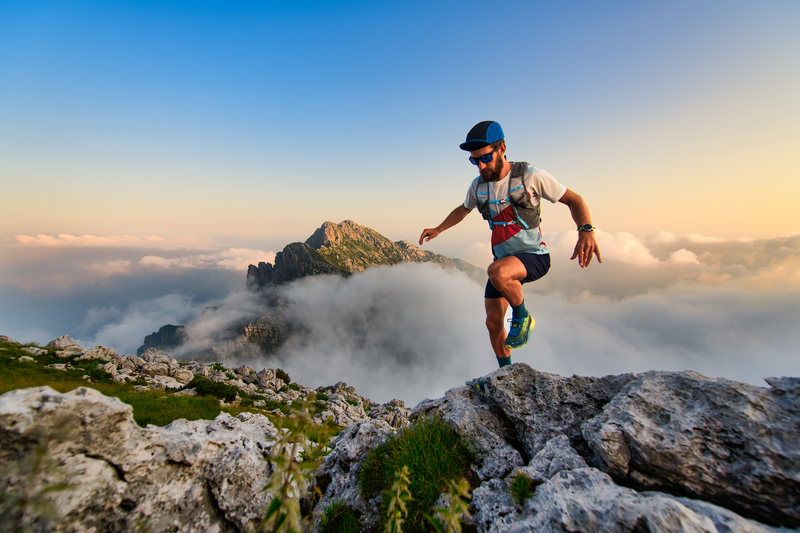
94% of researchers rate our articles as excellent or good
Learn more about the work of our research integrity team to safeguard the quality of each article we publish.
Find out more
ORIGINAL RESEARCH article
Front. Microbiol. , 02 December 2021
Sec. Systems Microbiology
Volume 12 - 2021 | https://doi.org/10.3389/fmicb.2021.749760
This article is part of the Research Topic A Focus on Actinobacteria: Diversity, Distribution, and Secondary Metabolites View all 10 articles
Actinorhizal plants host mutualistic symbionts of the nitrogen-fixing actinobacterial genus Frankia within nodule structures formed on their roots. Several plant-growth-promoting bacteria have also been isolated from actinorhizal root nodules, but little is known about them. We were interested investigating the in planta microbial community composition of actinorhizal root nodules using culture-independent techniques. To address this knowledge gap, 16S rRNA gene amplicon and shotgun metagenomic sequencing was performed on DNA from the nodules of Casuarina glauca. DNA was extracted from C. glauca nodules collected in three different sampling sites in Tunisia, along a gradient of aridity ranging from humid to arid. Sequencing libraries were prepared using Illumina NextEra technology and the Illumina HiSeq 2500 platform. Genome bins extracted from the metagenome were taxonomically and functionally profiled. Community structure based off preliminary 16S rRNA gene amplicon data was analyzed via the QIIME pipeline. Reconstructed genomes were comprised of members of Frankia, Micromonospora, Bacillus, Paenibacillus, Phyllobacterium, and Afipia. Frankia dominated the nodule community at the humid sampling site, while the absolute and relative prevalence of Frankia decreased at the semi-arid and arid sampling locations. Actinorhizal plants harbor similar non-Frankia plant-growth-promoting-bacteria as legumes and other plants. The data suggests that the prevalence of Frankia in the nodule community is influenced by environmental factors, with being less abundant under more arid environments.
Casuarinaceae family comprises four plant genera (Allocasuarina, Casuarina, Ceuthostoma, and Gymnostoma) and approximately 86 species and 13 subspecies indigenous to Australia (Steane et al., 2003; Gtari and Dawson, 2011; Diagne et al., 2013; Normand et al., 2014) that are frequently introduced into approximately 150 tropical and subtropical countries where they play an important role for land reclamation, crop protection and as windbreaks (Diagne et al., 2013; Potgieter et al., 2014). Large-scale planting of casuarinas has proven to have a strong impact especially in China, Senegal, Egypt, and Tunisia (Gtari and Dawson, 2011). In arid and semi-arid areas, salinization of soils and groundwater is a serious problem causing a drastic reduction in agricultural production (Munns, 2005; Rengasamy, 2006)1. Over 800 million hectares of land throughout the world are salt affected. A common method for dealing with salt stress problems is to reclaim saline soils with multipurpose, fast-growing salt-tolerant tree species such as Casuarina tree. Casuarina are notable for high salt tolerance (Tani and Sasakawa, 2003) and have been used as a green barrier. Despite the applied interests, substantial global invasion consequences for Casuarina has noted in Florida and the Mascarene Islands (Potgieter et al., 2014).
Actinorhizal plants, like Casuarina, form a nitrogen-fixing symbiosis with the Actinobacteria Frankia that results in the formation of root nodule structures where the bacteria are located (Chaia et al., 2010; Normand et al., 2014). Besides Frankia, actinorhizal plants are known to associate with mycorrhizae including both ectomycorrhizal or endomycorrhizal fungi (Zhong et al., 1995; Duponnois et al., 2003; Diagne et al., 2013; Zhou et al., 2017). The symbioses with Frankia and mycorrhizae allow actinorhizal host plants to colonize harsh environmental terrains including highly contaminated, dry, poorly drained, nutrient-poor, and salinized soils (Diagne et al., 2013, 2015).
Besides Frankia, several other non-Frankia bacteria have been isolated as a by-product of Frankia isolation attempts (Ghodhbane-Gtari and Tisa, 2014). Large numbers of other bacteria were collected from these actinorhizal nodules, occupying the same microniche as Frankia. Most of these isolates were ignored or discarded as being irrelevant to the plants. However, these non-Frankia Actinobacteria have consistently been isolated from several actinorhizal plants including Casuarina (Guillén et al., 1993; Niner et al., 1996; Valdes et al., 2005; Ghodhbane-Gtari et al., 2010), and consist of Actinobacteria from Micromonospora (Valdes et al., 2005; Ghodhbane-Gtari et al., 2010), Nocardia (Ghodhbane-Gtari et al., 2010, 2019) and Streptomyces genera (Ghodhbane-Gtari et al., 2010). Similarly, non-symbiotic bacteria, such as Azospirillum, Bacillus, Pseudomonas, and Streptomyces, are known to be auxiliary bacteria in several Rhizobium-legume symbioses (Iruthayathas et al., 1983; Grimes and Mount, 1984; Li and Alexander, 1990). In this case, both wild and cultivated legume nodules are not exclusively inhabited by rhizobia, but contain diverse assemblages of non-rhizobial bacteria (Dudeja et al., 2012; Aserse et al., 2013; Martínez-Hidalgo and Hirsch, 2017). These nodule non-rhizobial communities may be influenced more by soil type rather plant genotype (Leite et al., 2017).
During the last decade, culture-dependent and –independent approaches have been used to intensively investigate these effects. For legume nodules, rhizobial, and non-rhizobial endophytes communities have been initiated (Martínez-Hidalgo and Hirsch, 2017; Zhang et al., 2018; Mayhood and Mirza, 2021), but very little is known about microbial communities and their structure associated with actinorhizal nodules. Endophytic bacteria can be found between cells, while others are associated in an intimate relation with the plant. For that intimate association to occur, complex relationships are required during the colonization of plant tissues. Plant endophytes are described as having the ability to restrain pathogen infection, to accelerate seedling emergence, and to promote plant growth. Thus, they can be considered as biocontrol agents (Ryan et al., 2008; Eljounaidi et al., 2016; Santoyo et al., 2016).
In this study, we investigated the structure and function of bacterial communities found in Casuarina glauca nodules and associated rhizosphere soils and bulk soils from three different bioclimates in Tunisia. We used both high-throughput amplicon (targeting of the 16S rRNA gene) and whole metagenome shotgun sequencing of C. glauca nodules found from the different bioclimates: humid, semi-arid and arid condition. For comparative purposes, high-throughput amplicon sequencing was performed on rhizosphere soils, and bulk soils for these three sites.
Samples of Casuarina glauca root nodules, rhizosphere, and bulk soils. Samples were collected in February 2015 from three sites in Tunisia that represented different bioclimatic regions (Figure 1). Sample sites were in Tamra (37°3′26″ N 9°14′17″ E), Sidi Bouzid (35°2′ 7.58″ N 9° 29′2.18″ E), and Medenine (33°21′17′′ N 10°30′19′′ E), representing Mediterranean-humid, semi-arid and arid bioclimatic regions, respectively. Three to four root nodules were used for each replicate and three replicate samples were taken for each site. For the soil and rhizosphere samples, triplicate samples of 5 g soil were collected from each site. Bulk soil was soil that was not in contact with the root system of any plant. Rhizosphere soil was collected by removing organic litter and 15 cm of the surrounding soil from around the C. glauca roots. Soil that was attached to roots after removal of the surrounding soil was collected. The samples were placed in sterile plastic tubes and stored at −20°C until analysis. DNA extractions were performed within 24 h of sampling.
Figure 1. Map and schematic of amplicon survey sampling design: For each sample type from each site, 3 replicates were sampled. Modified from Hénia (2008).
For the rhizosphere and bulk soil samples, genomic DNA (gDNA) was extracted from 0.5 g of soil using the Qiagen DNA extraction kit as described previously (Ghodhbane-Gtari et al., 2010, 2014). For the C. glauca root nodule samples, three to four nodules were used per gDNA extraction and nodules were surface sterilized as described previously (Ghodhbane-Gtari et al., 2019). Root nodules were thoroughly rinsed with water. Surface sterilization conducted by shaking in 30% (v/v) H2O2 for 30 min. Sterilized nodules were rinsed thoroughly several times with sterile water. The final wash liquid was tested for microbial growth and only nodules resulting in a sterile final washing liquid were further considered for DNA extraction. The nodule was then aseptically powdered in liquid nitrogen using a sterile mortar and the resulting powder was used for DNA extraction using Qiagen DNA Plant Mini-kit following the manufacturer’s protocol.
To investigate the prokaryotic community profiles of different DNA extracted, sequences corresponding to the V4 hypervariable region the 16S subunit rRNA gene were amplified by PCR using the Earth Microbiome Project 515F/806R universal primers under conditions described previously (Caporaso et al., 2012). For each sample, triplicate amplifications were performed. The triplicates were pooled, and all samples were normalized to equal DNA concentrations. Paired-end sequencing of the amplification product was performed using the Illumina HiSeq 2500 platform at the Hubbard Center for Genome Studies (University of New Hampshire, Durham, NH, United States). Read lengths of 250 bp were obtained and processed as described below.
To generate metagenomes for the C. glauca root nodule samples, whole genome shotgun sequencing was performed. Sequencing libraries for the samples were prepared using the Illumina NextEra Library Preparation protocol according to the manufacturer’s instructions and were sequenced on an Illumina HiSeq 2500. The same triplicate nodule DNA extractions were used for both the metabarcoding and metagenome shotgun sequencing methods, and metagenome libraries for both methods were sequenced at 150 bp read lengths at the Hubbard Center for Genome Studies (University of New Hampshire, Durham, NH, United States).
The 16S rRNA gene amplicon sequences for each sample were initially processed with Quantitative Insights into Microbial Ecology (QIIME) pipeline (Caporaso et al., 2010b). Paired-end reads were joined using Fastq-join (Aronesty, 2011). Paired-end reads were truncated from positions where nucleotides received a Phred score of 20 or less. Reads with more than three consecutive low-quality nucleotides were discarded. Reads with less than 75% high-quality base-call scores were also discarded. This processing resulted in a total of 176,319 reads with an average length of 253 base pairs.
The reads were clustered as described in the open reference-based protocol provided in QIIME (version 1.9.0-dev) (Rideout et al., 2014). OTUs were clustered by UCLUST, using a 97% percent identity threshold to define an OTU (Edgar, 2010). Taxonomy was assigned to each OTU cluster using the UCLUST consensus taxonomy assigner by aligning the centroid sequence of each cluster to the Green Genes 13_8 rRNA database with PyNAST (DeSantis et al., 2006; Caporaso et al., 2010a; Edgar, 2010). OTUs that were unable to be aligned to the Green Genes 13_8 database were excluded from downstream analysis. OTUs that only contained one sequence (singletons) were also excluded from downstream analysis. The resulting OTU tables were filtered to exclude all unassigned sequences, and sequences that assigned to mitochondrial or chloroplast taxonomy.
The OTU table resulting from the process above was rarified to an equal sampling depth based on the smallest library size (1,762 reads) by random sub-sampling of all samples. Alternatively, Cumulative Sum Scaling (CSS), available through the metagenomeSeq R package and facilitated through QIIME, was used to normalize the OTU table (Paulson et al., 2013). Because choice of normalization method has been shown to effect downstream analysis (McMurdie and Holmes, 2014), both methods were used to compare results of normalization techniques. For Alpha diversity analysis, the original OTU table was converted to closed-reference format and corrected for variation in 16S rRNA copy number using the online Galaxy server version of PICRUSt (Langille et al., 2013). This correction was also performed on CSS normalized OTU tables and raw OTU tables in order to assess effects on ordination results.
Alpha diversity for each sample was calculated based on the Shannon Diversity Index (Shannon, 1948) and significant differences in diversity based on sample type (Nodule, Rhizosphere, or Soil) were determined using ANOVA and the t-test in Microsoft Excel. Alpha diversity was also calculated in the similar manner for nodule samples from individual sampling locations. Community beta diversity among samples was measured by the UniFrac distance (Lozupone and Knight, 2005). Both the weighted (quantitative) and unweighted (qualitative) versions of the distance metric were used. Both of these metrics can yield different but complementary results from the same dataset (Lozupone et al., 2007). Distance matrices produced using these metrics were ordinated using Principle Coordinates Analysis (PCoA). Ordinations were created for distance matrices produced by CSS (for both 16S copy number corrected and not corrected), for raw OTU tables with 16S operon copy number correction and for evenly rarefied OTU tables.
Jackknifing, which is the repeated subsampling of a dataset, was used to determine the confidence level in Beta diversity analysis. This technique was performed by randomly subsampling 100 sequences per sample from the rarefied OTU table. For each subsampling, an Unweighted Pair Group Method with Arithmetic Mean (UPGMA) tree was created using the unweighted UniFrac distance matric between samples, based on those 100 randomly subsampled reads. This process was repeated 100 times. A consensus tree was built from the 100 trees with jackknife values for each node representing the number of times, out of the 100 jackknifed trees, that the consensus configuration was observed.
Nodule samples were analyzed by two-way cluster analysis to investigate the difference in nodule community structure across the environmental gradient in the sampling design. An OTU table was constructed that was corrected for 16S operon copy number abundance by PICRUSt and summarized to relative abundance at the genus level. The samples were grouped by hierarchical clustering using the average linkage method. The Bray-Curtis distance between each sample based on taxonomic composition was used for sample clustering. The taxa were clustered by their abundance patterns across samples using the Bray-Curtis distance between metric. Clustering was performed using the data for all genera present. However, for visualization purposes, only taxa that made up greater than 1% of the community were displayed.
Multi-Response Permutation Procedures (MRPP) were performed to determine if there were significant differences in phylogenetic distances based on the sample type (Nodule, Soil, or Rhizosphere). MRPP analysis was performed in R (Version 3.2.1) using the Vegan Library (Oksanen et al., 2016). MRPP To determine if nodules formed a statistically significant group based on the weighted and unweighted UniFrac distance matrices, the samples were partitioned into two categories, nodule (n = 9) and non-nodule (n = 18). Significance was calculated by permutation of the distance matrices 999 times to determine if the groups had within-group distances that were different to what would be expected by chance. All MRPP analysis was performed on the weighted and unweighted UniFrac distance matrices produced by the different normalization methods described previously (Lozupone et al., 2007; McMurdie and Holmes, 2014).
Soil and Rhizosphere samples were analyzed to test the hypothesis that C. glauca maintains a unique microbial community within the rhizosphere at the different sampling locations. Soil (n = 9) and Rhizosphere (n = 9) samples from all sites were pooled together and analyzed independently of nodule samples in the same fashion as described above. To determine if there were significant groupings between soil and rhizosphere by climate type, the soil and rhizosphere samples were grouped into a humid group (n = 6) and non-humid group (n = 18). MRPP was performed as above with this grouping using the unweighted UniFrac distance matrix. This metric calculates phylogenetic distance between samples based off of the presence or absence of taxa and is thus useful for identifying differences between samples that come from environments that host distinct communities.
Trimmomatic was used to remove Nextera adapters and filter the raw reads based on quality (Bolger et al., 2014). The following parameters were used. Three base-pairs were trimmed from the tailing and leading ends of each read. The reads were scanned with a sliding window of four base-pairs, and sections of reads were discarded when the average quality score of the base-pairs was below 15. After this process, only reads that retained a minimum length of 36 base pairs were used for downstream processes. Of these resulting reads, only reads that retained both forward and reverse pairs were used for assembly.
In order to perform differential coverage binning of draft genomes, the read sets from each of the three sampling locations were combined in silico and a composite co-assembly was created. SPAdes (version 3.6.0) was used to assemble the combined reads using the metagenomic setting, without error correction (Bankevich et al., 2012). The assembly process used Khmer lengths of 22, 33, and 55 to produce the final assembled contigs.
Metagenomic Assembled Genomes (MAGs) of individual members of the metagenomic community were binned using the mmgenome package (Albertsen et al., 2013) for R2 using the Rstudio IDE3. This package facilitates distinguishing individual genomes from metagenomes primarily by the unique coverage values of assembled sequences belonging to organisms present in multiple samples, but at different abundances. Coverage values for each contig per-site was performed by individually aligning each read-set to the co-assembled contigs using the Bowtie2 alignment tool (Version 2.2.5) (Langmead and Salzberg, 2012). The resulting Sequence Alignment Map (SAM) files were converted to binary format and sorted to the left-most coordinates on the assembled sequences and depth of coverage per-base was calculated using SAMtools (Version: 0.1.19-96b5f2294a) (Li et al., 2009). The resulting files containing coverage per-base per-contig were converted to average coverage per-contig scripts in the Mmgenome package.
Additional information for assembled contigs was generated following the workflow as described by the mmgenome package developers (Albertsen et al., 2013). Open Reading Frames (ORFs) within the assembled metagenomic contigs were predicted and translated to amino acid sequence using the metagenomic setting of Prodigal (Version 2.6.2) (Hyatt et al., 2010). The translated amino acid fasta file of predicted ORFs was searched against Hidden Markov Models (HMMs) of 111 essential prokaryotic single copy genes provided in the mmgenome package using hmmsearch (Eddy, 1998). ORFs identified as essential genes were extracted from the co-assembly using Perl scripts provided by the mmgenome package.
Taxonomic information was assigned to each ORF identified as essential gene by searching against the RefSeq protein database using BlastP with an E value cutoff of 1e–5, with five maximum target sequences. The resulting BlastP output in XML format was uploaded into MEGAN (Version 5) (Huson et al., 2007). The Lowest Common Ancestor (LCA) algorithm was used to make a consensus taxonomic assignment for each ORF based off of the top five BlastP hits using the following settings: Top Percent = 5, Minimum Support = 1, Rank = Species. The resulting file was parsed using the hmm.majority.vote.pl script to derive a consensus taxonomic assignment for each assembled contig based off of the taxonomy of the ORFs that it contains.
The coverage and taxonomic information produced above was loaded into the Rstudio environment along with the composite metagenomic co-assembly using BioStrings4. The GC content and tetranucleotide frequency distribution of each assembled contig was calculated using the mmgenome R package. A principle component analysis (PCA) of normalized tetranucleotide frequencies of all contigs was performed in R using the mmgenome package. The composite co-assembly was visualized in Rstudio and binning was performed using the tools available in the mmgenome R package. The data that was produced as described above and accompanying rmarkdown files allow the reproduction of the binned draft genomes.
Reads from each site were assembled individually using SPAdes-3.6.0 using the metagenomic setting (Bankevich et al., 2012). The resulting assembled contigs were annotated using the Prokka annotation pipeline using the metagenomic settings (Seemann, 2014).
Coverage profiles of individual genomes with PCR duplicates removed were calculated to compare genome coverages at different sites. Reads were aligned to the metagenome assembly using the Bowtie2 alignment tool (Version 2.2.5) (Langmead and Salzberg, 2012). The SAM file resulting from alignment with Bowtie2 was converted to BAM format. The aligned reads were sorted to the left-most coordinate on the indexed metagenome assembly using SAMtools (Version: 0.1.19-96b5f2294a) (Li et al., 2009). Duplicate reads were removed using Picard Tools MarkDuplicates5. Coverage for the contigs was calculated from the resulting SAM file using Bedtools (v2.17.0) (Quinlan and Hall, 2010). The coverage calculations per-contig were averaged for all the contigs within a given genome bin. The average coverage for the entire genome was normalized by the amount of reads per data set corresponding to the different sampling sites. The percentage of the given read sets that aligned to plant-derived DNA was used to normalize each genome coverage value based on the differing amounts of plant-derived DNA in the different read sets.
The read sets, with plant-derived reads removed, were aligned to the Frankia genome using Bowtie2. The percentage of the reads that aligned to the Frankia genome bin were recorded from the standard output statistics in order to compare the relative abundance of Frankia in the shotgun data in comparison to the replicated amplicon data.
The Genome Feature File (GFF) produced by Prokka was parsed using a custom python script to evaluate the presence and taxonomy of plant-growth-promoting genes and genes involved in cellulose, chitin, and pectin degradation within the nodule community. Genes known for plant auxin production (Indole acetic acid and Phenyl acetic acid), phosphate solubilization (alkaline phosphatases and phytases), ACC Deaminase activity and degradation of chitin, cellulose and pectin were identified for each of the three data sets. The presence of these enzymes was identified by parsing the GFF file for the Enzyme Commission (EC) number assigned to a given ORF by the Prokka pipeline. Enzymes that were identified by their EC numbers were annotated by aligning the amino acid sequence of the entry against the RefSeq protein database using BlastP with an E value cutoff of 1e–5 and a maximum output of five entries. Cladograms of the BlastP output were constructed using the Lowest Common Ancestor (LCA) algorithm in MEGAN (Version 5.0) using the following settings: Top Percent = 5, Minimum Support = 1, Rank = Genus. A summary of the EC numbers used in this analysis is in Supplementary Tables 1–3. The antiSMASH pipeline (version 3.0) was used to identify secondary metabolites in the binned genomes (Weber et al., 2015). antiSMASH was run using the metagenomic setting to improve gene prediction for highly fragmented metagenomic assemblies.
Three distinct sites in Tunisia (Figure 1) were chosen to assess the effect of environmental conditions on the microbial community structure and diversity for Casuarina nodules, rhizosphere and surrounding soil. These sites represented a humid well-developed forest at Tamra, a semi-arid, poorly evolved saline soils of Sidi Bouzid and an arid, poorly evolved saline soils of Medenine. Figure 2 shows representative Casuarina stands from each of these sites and nodules from those stands. There were no obvious differences in nodule shape or size among the three sites and the plants exhibited the same level of general health.
Figure 2. Photograph of Casuarina tree stands at the sampling and representative nodules for those trees.
Preprocessing of the 16S rRNA gene amplicon sequences resulted in a total of 7,855 OTUs from the 149,879 paired reads. Individual library sizes ranged from 1,762 to 11,542 reads per sample, with a mean of 5,551 reads per sample. Rarefaction plots were performed in order to estimate the completeness of community sampling at the sequencing depth performed. The rarefaction plots for average observed OTUs per sample type is shown in Supplementary Figure 1. These plots show that the nodule samples were sequenced close to saturation, while increased sequencing depth could improve the documentation of rare OTUs for rhizosphere and soil samples.
The Shannon Diversity Index (SDI) was used to measure alpha diversity. This metric was measured on the relative abundance after correction for 16S operon copy number. Diversity measures were grouped by sample type and are as follows; Nodules: 4.47 ± 2.29 (SD), Rhizosphere: 9.33 ± 0.233 (SD) and Soil: 9.52 ± 0.69 (SD), displayed in Supplementary Figure 2A. The alpha diversity was significantly less for nodules than for rhizosphere and soil samples (Students t-test, p = 0.00001). Alpha diversity was calculated for the nodule samples at the three different samples locations. Nodules from Tamra had an average SDI of 2.28 (SD = 0.584), nodules from Sidi Bouzid had an average SDI of 7.32 (SD = 0.389) and nodules from Medenine had an SDI of 3.82 (SD = 0.394) (Supplementary Figure 2B).
After correction for 16S operon copy number, the relative abundance of taxa present in the root nodules was analyzed. At the humid sampling site, the relative abundance of Frankia was an average of 80% of the nodule community. This prevalence dropped to 1.5% and 0.5% and the semi-arid and arid sampling sites, respectively. These data are like the shotgun sequencing data (Supplementary Figure 3), where reads mapping to the binned Frankia genome comprised 60% of the humid site read set and that level dropped to 1.71% and 1.63% for the semi-arid and arid sampling sites, respectively. Supplementary Figure 3 shows the relative abundance of Frankia at the three sampling sites using the shotgun and amplicon data. Figure 3A gives a taxonomic summary of the nodule samples at the class level. The taxonomic summary of the rhizosphere and bulk soils is shown in Figure 3B.
Figure 3. Class level taxonomic summary of nodule, Rhizosphere and soil samples. (A) Nodules grouped by site. The relative abundance of the nodule samples was summarized at the class level after corrected for 16S operon copy number variation. The humid and arid samples are significantly less diverse than the semi-arid samples, as can be seen in (A). This can be seen here, as the humid site is dominated by Actinobacteria (the genus Frankia), and the arid site is dominated by Acinetobacter and Pseudomonas. (B) Rhizosphere and soil samples grouped by sampling site. These charts show relative abundance of taxa at the class level after correction for 16S operon copy number.
Beta diversity was analyzed by measuring the weighted and unweighted UniFrac distances (Lozupone and Knight, 2005). An Unweighted Pair Ground Method with Arithmetic Mean (UPGMA) consensus tree was built from the unweighted UniFrac distance matrix (Figure 4). Numbers on the nodes of the tree represent the percentage of trees out of 100 bootstrapped trees that had the configuration of the final consensus tree. The grouping on the tree shows that nodule samples from all sites form one clade when analyzed by the presence or absence of taxa in the samples, after removing one outlier (Sample ID: N3SIDI). This result shows that although the relative abundance of taxa within the nodule differed from sampling location (Figure 3 and Supplementary Figure 3), the nodules are distinct based on what taxa they host when compared to the soil and rhizosphere samples. The rhizosphere and soil primarily cluster based off the sampling location, this trend is also evident in ordinations of the data using the unweighted UniFrac distance metric (Supplementary Figure 4).
Figure 4. Unweighted pair group method with Arithmetic Mean (UPGMA) Consensus Tree. The tree was constructed from the rarefied unweighted UniFrac distance Matrix. The consensus tree was built by creating 100 trees from 100 randomly subsampled reads from each sample. The numbers on the nodes represent the percentage of the 100 trees that conformed to this configuration. The red box outlines that all nodule samples form one cluster on the tree. The other clades on the tree show that the soil and rhizosphere communities cluster mostly by sampling site.
The beta diversity distance matrices were ordinated using PCoA. Distance matrices were constructed using the weighted and unweighted UniFrac distances on OTU tables that had been normalized by rarefaction, a raw OTU table with ribosomal operon copy number correction and by cumulative sum-scaling (with and without ribosomal operon copy number correction). PCoA ordinations of these distance matrixes can be seen in Figure 5 and Supplementary Figures 4, 5. For the sake of clarity, Figure 5 represents weighted UniFrac distances normalized by cumulative sum-scaling without ribosomal operon copy number correction. All of the methods gave similar results (Supplementary Figure 4). In all ordinations, nodule samples are separated from the soil and rhizosphere samples along the first Principal Coordinate, which explains the most phylogenetic variation in the data. Multi-response Permutation Procedures (MRPP) agreed with the results seen in the UPGMA tree, with significant grouping of nodules for every normalization type using the both forms of the UniFrac distance metric. Supplementary Table 4 summarizes the chance-corrected results of the MRPP analysis. The A Statistics, the chance-corrected within-group agreement, is a value between 1 and 0 that describes the within-group heterogeneity of a given group. If all samples in a group are identical A = 1, if the heterogeneity in a group is equal to what would be expected by chance A = 0 (Mielke and Kenneth, 2007).
Figure 5. Principal coordinates ordination of CSS normalized UniFrac distance matrices: Ordinations where normalized using Cumulative Sum Scaling. Ordinations was made using the weighted UniFrac distance. Symbols represent: nodules (•), rhizosphere (■), and soil (▲). For all ordinations MRPP showed a statistically significant grouping of the nodule samples, as outlined in Supplementary Table 4.
The results of the UPGMA Tree (Figure 4) and the PCoA ordinations (Figure 5 and Supplementary Figures 4, 5) show that nodules host a distinct prokaryotic community compared to the soil and rhizosphere samples. Ordination and MRPP results show that this grouping is statistically significant whether the unweighted UniFrac or weighted UniFrac is used.
Nodules form a distinct group based on the taxa they host, but the relative composition of this group of taxa changes drastically along the environmental gradient sampled (Figure 3A). In Figure 5, the ordinations show the grouping of nodule samples by location along the second principal coordinate, which explains the second largest amount of taxonomic variation in the dataset.
To understand differences in the rhizosphere and soil communities across the sampling sites, these samples were analyzed separately from the nodule samples. Rhizosphere soils from all sites were analyzed as one sample category to test the hypothesis that C. glauca hosts a distinct microbial community within the rhizosphere across environmental gradients. When soil and rhizosphere samples from all sites were grouped together by type, the MRPP results were inconsistent in their significance, depending on the OTU table normalization strategy. A summary of these results is found in Supplementary Table 5. Statistical significance (P < 0.05) was only observed with the evenly rarefied OTU table and the raw OUT table that corrected for 16S operon copy number Variation. Ordinations of these data (Supplementary Figures 4, 5) show that the rhizosphere and soil samples formed two clusters only on the raw OTU table and the evenly rarefied OTU table. CSS normalization produces different results with the soil and rhizosphere samples not forming distinct groups.
The ordinations using the unweighted UniFrac distance, which considers the presence and absence of taxa, show that soil and rhizosphere samples clustered based on climate. When the data is ordinated using this metric, two distinct clusters of rhizosphere and soil samples formed Supplementary Figure 6. These clusters correspond to the humid sampling site (Tamra) and the two arid sampling sites (Sidi Bouzid and Medenine). For all normalization types, a significant grouping was identified by MRPP (Supplementary Table 6), showing that the environmental conditions significantly change the taxa present between the humid and arid soils that were sampled. A taxonomic summary of these samples at the class level are presented in Figure 3B.
Quality filtering of shotgun metagenomic reads resulted in a total of 83,027,118 paired end reads. The library for Sites 1, 2, and 3 were comprised of 33,051,352, 34,239,886, and 15,735,880 reads, respectively. In silico co-assembly of the reads resulted in an assembly contained 3,153,711 contigs totaling 56,964,841 base pairs in length. The statistics for the assembly produced by Quast (Gurevich et al., 2013) are outlined in Supplementary Table 7.
Seven predominant genomes were binned using the mmgenome R package. A plot of the metagenomic assembly can be seen in Figure 6A. The binned genomes were identified being in the genera Frankia, Micromonospora, Bacillus (two isolates), Phyllobacterium, Paenibacillus, and Afipia.
Figure 6. (A) Plot of metagenomic contigs larger than 5 kbp produced by the mmgenome R Package. The seven dominant genomes that were binned using the mmgenome R package are labeled with their annotated genus. The purple and black cluster in the center of the image are contigs derived from C. glauca DNA. (B) Normalized genome coverage of genome bins. Reads from each of the three sampling sites were aligned to the co-assembled contigs. SAMtools was used to sort and index the coordinates of the aligned reads and Picard MarkDuplicates was used to remove duplicate reads with identical coordinates. Bedtools was then used to calculate coverage per contig per site. Coverage values were normalized by millions of reads per data set and the relative amount of plant DNA that comprised each data set.
Two of the binned genomes annotated to the phylum Actinobacteria. The first genome bin contained 4,111 ORFs that annotated to the genus Frankia. The annotations for the ORFs in this bin had top hits on many of the Frankia clade 1c Casuarina infective strains. The most top annotations that hit on a single sequenced strain was 775 of the 4,111 ORFs annotated to Frankia sp. BMG5.23, a salt-tolerant Tunisian isolate (Ghodhbane-Gtari et al., 2014). Reads mapping to the binned Frankia draft genome comprised 60% of the reads in from the humid sampling site, Tamra. Of the reads from semiarid Sidi Bouzid and arid Gabes, 1.7% and 1.6% of the reads mapped to the Frankia genome bin, respectively. Genome bin two resulted in an assembly that contained 6,522 ORFs with 6,053 annotating to the genus Micromonospora. The ORFs that annotated to Micromonospora were mainly comprised of annotations to Micromonospora purpureochromogenes, with 4,565 ORFs. Three genomes that were binned annotated to the phylum Firmicutes. The first contained 6,268 ORFs with 5,866 of them annotating to the genus Paenibacillus. The second Firmicutes genome bin contained 4,275 ORFs, 4,060 of which annotated to the genus Bacillus. Of those ORFs annotating to the genus Bacillus, 3,416 of them annotated to B. simplex. The last Firmicutes genome had 4,215 ORFs that also annotated to the genus Bacillus with 3,313 of them annotating to B. aquimaris and 569 annotating to B. vietnamensis. Two of the binned genomes annotated to the phylum proteobacteria. The first binned genome contained 4,370 ORFs that annotated to the genus Afipia. The second proteobacteria genome bin contained 3,068 ORFs that annotated to the genus Phyllobacterium. General genome characteristics of the binned genomes are summarized in Table 1.
Genome coverage for binned genomes was calculated in order to compare coverage patterns across sites to the replicated 16S rRNA gene amplicon survey. The only binned genome which followed the same patterns as the replicated analysis was the genome bin that annotated as a member of Frankia (Figure 6A) compared to the relative abundance of Frankia between the two datasets. For those calculations, reads that aligned to plant-derived DNA was first removed. The resulting read sets for each site were aligned to the reassembled Frankia genome bin using Bowtie2. The percentage of those reads that aligned to the genome bin was recorded. The Frankia genome bin was the dominant member of the humid site, but coverage values were much lower for the semi-arid and arid sampling sites (Figure 6B).
The MAGs reconstructed from the metagenomic dataset were analyzed for the presence of secondary metabolite biosynthetic pathways using AnitSMASH (Weber et al., 2015). The secondary metabolite biosynthetic clusters that were identified in the binned genomes are outlined in Table 2. Predicted biosynthetic clusters from the binned genomes were predicted to produce siderophores, terpenes, non-ribosomal peptide synthases and other secondary metabolites. The genome Frankia and Micromonospora genome bins contained the most secondary metabolite biosynthetic clusters about 25 for each bin genome, although only clusters which have a predicted product are listed here.
The shotgun metagenomic data was mined for genes of interest with potential functional importance to the actinorhizal symbiosis and C. glauca health. Genes potentially responsible for plant–growth-promoting traits were mined from GFF files produced by the Prokka Annotation pipeline (Seemann, 2014). Figures 7–9 show the results of this analysis as outlined by site. Cladograms for the taxonomy of the genes identified are presented. The number of genes for each function is reported in each figure. The sampling sites 1, 2, and 3 had 159, 183, and 202 genes of interest, respectively. Since shotgun sequencing was not performed with replicates, these results are presented qualitatively as presence or absence data.
Figure 7. Cladograms of functional genes from Site 1 (Tamra), that were produced by the LCA Algorithm in MEGAN (Version 5). The sizes of colored circles represent that relative contribution of a taxa to the functional genes analyzed in a given cladogram. Numbers in Brackets represent the number of genes that were annotated to a given taxon for that set of genes. (A) Potential indole acetic acid biosynthetic genes. Total genes, 24. (B) Cellulose degrading genes. Total genes identified, 89. (C) Alkaline phosphatase genes. Total genes identified, 26. (D) ACC deaminase genes. Total genes Identified, 2. (E) Chitin degrading genes. Total genes identified, 14. (F) Pectinase genes. Total genes identified, 2. (G) Phytase genes. Total genes identified, 2.
Figure 8. Cladograms of functional genes from Site 2 (Sidi Bouzid), that were produced by the LCA Algorithm in MEGAN (Version 5). The sizes of colored circles represent that relative contribution of a taxa to the functional genes analyzed in a given cladogram. Numbers in Brackets represent that number of genes that annotated to a given taxon for that set of genes. (A) Phytase genes. Total genes identified, 8. (B) Pectinase enzymes. Total genes identified, 5. (C) ACC deaminase genes. Total genes identified, 3. (D) Potential indole acetic acid biosynthesis genes. Total genes identified, 27. (E) Cellulose degrading enzymes. Total genes identified, 97. (F) Chitin degrading enzymes. Total genes identified, 25. (G) Potential phenyl acetic acid biosynthesis genes. Total genes identified, 3. (H) Alkaline phosphatase genes. Total genes identified, 15.
Figure 9. Cladograms of functional genes from Site 3 (Medenine), that were produced by the LCA Algorithm in MEGAN (Version 5). The sizes of colored circles represent that relative contribution of a taxa to the functional genes analyzed in a given cladogram. Numbers in Brackets represent that actual number of genes that annotated to a given taxon for that set of genes. (A) Cellulose degrading genes. Total genes identified, 108. (B) Alkaline phosphatase genes. Total genes identified, 19. (C) Potential indole acetic acid biosynthetic genes. Total genes identified, 30. (D) Potential phenyl acetic acid biosynthesis genes. Total genes identified, 5. (E) ACC deaminase genes. Total genes identified, 6. (F) Chitin degrading genes. Total genes identified, 24. (G) Pectinase genes. Total genes identified, 8. (H) Phytase genes. Total genes identified, 2.
The 16S rRNA gene amplicon analysis of the taxonomic community in nodules showed that they were largely dominated by Actinobacteria, Alphaproteobacteria, and Gammaproteobacteria (Figure 3). The remaining sequences matched with Bacilli, Planctomycetia and Deltaproteobacteria. A remarkable change in microbial community dominance was observed across climate stages. In the humid climate zone, represented by Tamra site, Actinobacteria was the most dominant class, while the semiarid (Sidi Bouzid) and arid (Medenine) environments had Alphaproteobacteria and Gammaproteobacteria, respectively, as the most prominent class. This result was surprising because Frankia is Actinobacteria and would be expected to dominate the nodule environment.
However, the relative abundance of Frankia was drastically affected by the environmental gradient. Nodule occupancy was highest (80%) in plants at the Tamra (humid zone); but was drastically reduced in nodules from the semi-arid Sidi Bouzid (1.5%) and arid Medenine (0.5%). One possible explanation of this phenomenon is that the physical and chemical characteristics of semi-arid and arid environments are not beneficial for the maintenance of the actinorhizal symbiosis or Frankia persistence. Soils in the semi-arid and arid regions of Tunisia are poorly evolved calcareous soils (Mtimet, 2001). In these soils, phosphorous is known to be immobilized by formation of complexes with calcium cations (Tunesi et al., 1999). Low phosphorus levels decrease the frequency of C. cunninghamiana nodulation by Frankia (Yang, 1995) and nodule weight of Frankia-C. equisetifolia (Sanginga et al., 1989). Similarly, drying and heat are known to decrease the ability of some Frankia strains to infect C. equisetifolia (Sayed, 2011). Dryness, high temperature and low levels of available phosphorous are characteristics of soils in arid environments and these factors could be influencing the low abundance and absence of Frankia in nodules at these environments. Detecting Casuarina infective clades of Frankia through plant-trapping assays have proven difficult (Gtari et al., 2002, 2007) and the scarcity of detection of Casuarinaceae-infective Frankia outside of the plants native range has been demonstrated (Mirza et al., 2007). One possible explanation is that Casuarina-infective Frankia are more persistent in humid environments that are more similar to the riparian habitat that is preferred by Casuarina within their host range. These results are congruent with the need for intentional inoculation with a compatible Frankia strain necessary for nodulation in some land reclamation projects in Africa (Gauthier et al., 1985). However, this reasoning does not explain the absence of Frankia within mature nodules. The absence of detected Frankia in four of the nine nodule samples leads to one hypothesis that another member of the community may be responsible for the formation of nodules on C. glauca. Agrobacterium rhizogenes will induce pseudonodules on Elaeagnus angustifolia that are indistinguishable from Frankia – induced nodules (Berg et al., 1992). Our results show an OTU of genus Agrobacterium that was as differentially abundant in nodule samples. The genus Agrobacterium was present in eight of the nine nodule samples ranging from 0.01% to 2.8% relative abundance. This suggests that a member of this genus could play a role in nodule formation when Frankia is in low abundance. Another hypothesis is that this absence is a seasonal variation and part of the life cycle of Frankia under semi-arid and arid conditions. As the levels of Frankia decreases, other microbes could establish more dominance within the nodule. A seasonal study of the changes in community structure could address this hypothesis.
Multi-response permutation procedures analysis found that nodule samples formed a statistically significant group compared to the rhizosphere and soil samples (Figure 5 and Supplementary Figures 4–6). Nodule samples are separated from the rhizosphere and soil samples by the first principal coordinate axis, which explains the most taxonomic information in the samples. This result is also confirmed with the jackknifed UPGMA tree built from the unweighted UniFrac distance matrix (Figure 4), which shows a distinct clade where all nodule samples clustered, despite location. Alpha diversity of the nodule samples was also significantly lower than for the rhizosphere or soil samples (Supplementary Figure 1). These observations are similar to comparative analysis of the root microbiome of the model plant Arabidopsis thaliana, which has shown that endophytic communities from plants growing in chemically distinct soil types are highly overlapping and less diverse than surrounding soils (Lundberg et al., 2012). The community selected by C. glauca was distinct from the soil and rhizosphere communities across a steep environmental gradient and was significantly less diverse, which is like the pattern of the A. thaliana microbiome. The similar patterns of microbiome recruitment by C. glauca and A. thaliana suggests that phylogenetically distant plant groups tend to select for a particular endophytic community across environmental gradients.
From metagenome analysis, seven predominant genomes were identified by binning (Figure 6) and were assigned to six different genera: Frankia, Micromonospora, Bacillus (two isolates), Phyllobacterium, Paenibacillus, and Afipia. Reads from the humid sampling site Tamra, contains the most reads mapping to the binned Frankia draft genome (60%), then Sidi Bouzid (1.7%) and Medenine (1.6%). The less abundance of Frankia in semi-arid and arid sites can be related to the harsh conditions, dryness and poor composition of soils. Not surprisingly, the Frankia MAG closely matched the Frankia sp. strain BMG5.23 genome (Ghodhbane-Gtari et al., 2014). This strain was isolated from Tunisian soils and probably represents the local strain in this region.
By the use of the antiSMASH program (Blin et al., 2017), secondary metabolite biosynthetic pathways were predicted from the draft genomes rebuilt from the metagenomic data set (Table 2). Not surprisingly, the Actinobacteria, Frankia and Micromonospora, genomes contained the most secondary metabolite biosynthetic clusters. Actinobacteria are well known for their large assembly of secondary metabolic pathways producing a wide array of natural products.
The presence of non-Frankia bacteria within the nodules confirms other observations (Guillén et al., 1993; Niner et al., 1996; Solans and Vobis, 2003; Valdes et al., 2005; Trujillo et al., 2006; Solans, 2007; Ghodhbane-Gtari et al., 2010, 2014, 2019; Solans et al., 2011). In particular, the presence of Micromonospora is noteworthy having been identified as an endophyte of actinorhizal and leguminous plants (Trujillo et al., 2006, 2007; Solans et al., 2011) and considered a normal occupant of actinorhizal nodules (Carro et al., 2013). Two different Bacillus genomes were identified with one being closely related to B. simplex and the other being closely related to B. aquimaris and B. vietnamensis. Bacillus simplex is known for its adaptations to arid environments (Sikorski and Nevo, 2005) and has been shown to acts as a helper-bacteria for the symbiosis between Pisum sativum and Rhizobium leguminosarum bv. viciae (Schwartz et al., 2013). Bacillus isolates are helper-bacteria for the Frankia-Casuarina symbiosis (Echbab et al., 2004).
Paenibacillus genome revealed secondary metabolic clusters for siderophore production, ectoine, (an osmoprotectant), and bacillaene (an antibiotic). In a nursery trail, co-inoculation of C. equisetifolia with Paenibacillus polymyxa and Glomus geosporum results in the highest seedling quality (Muthukumar and Udaiyan, 2010), showing the potential benefits of this nodule occupant. One hypothesis is that these non-Frankia Actinobacteria act as helper-bacteria in the formation of the actinorhizal symbiosis (Ghodhbane-Gtari et al., 2010, 2019; Solans et al., 2011; Ghodhbane-Gtari and Tisa, 2014). The absence of Nocardia within these nodule communities was surprising. Nocardia strains (BMG51109 and BMG111209) were isolated from Casuarina glauca in Tunisia (Ghodhbane-Gtari et al., 2010) and shown to have plant-growth promoting properties (Ghodhbane-Gtari et al., 2019). Co-infection studies showed that Nocardia casuarinae strain BMG51109 plays a role as a “helper bacteria” promoting an earlier onset of nodulation (Ghodhbane-Gtari et al., 2019).
Besides generating metagenomes, the shotgun data set was functionally mined to identify potential plant-growth-promoting genes (PGPG) and to phylogenetically analyze these data (Figures 7–9) suggesting a wide berth of potential PGPG genetic capability for plant growth promotion. Besides this phylogenetic analysis, these coding regions were predicted to produce enzymes and metabolites of relevance to the benefiting plant health. All three metagenomes contained the 1-aminocyclopropane-1-carboxylate (ACC) deaminase gene. ACC deaminase plays an important role in the nodulation process of leguminous plants (Nascimento et al., 2019) and has been shown to improve plant growth under arid (Bessadok et al., 2020) or saline regions (Orozco-Mosqueda et al., 2020). Other major functions involved in nutrient recycling, suppressing phytopathogens and mineralization of organic matter were also highly abundant within all three metagenomes. The enzymes, chitinase, phytase, cellulase, pectinase and alkaline phosphatase are well known to be involved in the preservation of the plant fertility and maintaining the healthy plant growth (Neeraja et al., 2010; Maksimov et al., 2011; Jain and Singh, 2017). These hydrolytic enzymes serve as an important defense strategy to defend against phytopathogenic fungi (Lynd et al., 2002). These enzymes may serve to help the Casuarina to survive from fungal pathogens.
Genes for production of phytohormones were also present at all three sites in variable proportions. Genes for IAA and PAA production were significantly enriched in the semi-arid and arid climates. IAA and PAA play a key role in many aspects of plant growth and cell elongation and division (Champion et al., 2015; Sugawara et al., 2015). The increased number of IAA and PAA genes suggest that these phytohormones may alter the plant physiology to respond to these arid conditions.
The microbial community of the nodules appears to be shaped by the different bioclimates found in Tunisia. Beside Frankia microsymbiont, the niche builder of root nodules, other bacteria may occur within. However, further work is required to understand the PGP mechanisms of the different co-inhabitants of the nodule and more diverse samples from a wide range of actinorhizal plant nodules is needed to deepen this study.
The datasets presented in this study can be found in online repositories. The names of the repository/repositories and accession number(s) can be found below: https://www.ncbi.nlm.nih.gov/bioproject/, PRJNA482626.
FG-G, MG, and LT conceived the study. FG-G and TD’A performed the research. FG-G, TD’A, AG, SG, MG, and LT analyzed the data. FG-G, TD’A, MG, and LT wrote the manuscript. All authors approved the manuscript.
This material is based upon work supported by the New Hampshire Agricultural Experiment Station, through joint funding of the National Institute of Food and Agriculture, United States Department of Agriculture, and State of New Hampshire. This is Scientific Contribution Number 2913. This project (LT) was supported by the USDA National Institute of Food and Agriculture Hatch 1019869 (LT), College of Life Science and Agriculture at the University of New Hampshire, Durham, and Ministère de l’Enseignement Supérieur et de la Recherche Scientifique, Tunisia (LR03ES03) (FG-G).
The authors declare that the research was conducted in the absence of any commercial or financial relationships that could be construed as a potential conflict of interest.
All claims expressed in this article are solely those of the authors and do not necessarily represent those of their affiliated organizations, or those of the publisher, the editors and the reviewers. Any product that may be evaluated in this article, or claim that may be made by its manufacturer, is not guaranteed or endorsed by the publisher.
The Supplementary Material for this article can be found online at: https://www.frontiersin.org/articles/10.3389/fmicb.2021.749760/full#supplementary-material
Albertsen, M., Hugenholtz, P., Skarshewski, A., Nielsen, K. L., Tyson, G. W., and Nielsen, P. H. (2013). Genome sequences of rare, uncultured bacteria obtained by differential coverage binning of multiple metagenomes. Nat. Biotechnol. 31, 533–538. doi: 10.1038/nbt.2579
Aronesty, E. (2011). ea-utils: “Command-Line Tools for Processing Biological Sequencing Data”. Available online at: http://code.google.com/p/ea-utils
Aserse, A. A., Rasanen, L. A., Aseffa, F., Hailemariam, A., and Lindstrom, K. (2013). Diversity of sporadic symbionts and nonsymbiotic endophytic bacteria isolated from nodules of woody, shrub, and food legumes in Ethiopia. Appl. Microbiol. Biotechnol. 97, 10117–10134. doi: 10.1007/s00253-013-5248-4
Bankevich, A., Nurk, S., Antipov, D., Gurevich, A. A., Dvorkin, M., Kulikov, A. S., et al. (2012). SPAdes: a new genome assembly algorithm and its applications to single-cell sequencing. J. Comput. Biol. 19, 455–477. doi: 10.1089/cmb.2012.0021
Berg, R. H., Liu, L. X., Dawson, J. O., Savka, M. A., and Farrand, S. K. (1992). Induction of Pseudoactinorhizae by the plant pathogen agrobacterium-rhizogenes. Plant Physiol. 98, 777–779. doi: 10.1104/pp.98.2.777
Bessadok, K., Navarro-Torre, S., Pajuelo, E., Mateos-Naranjo, E., Redondo-Gomez, S., Caviedes, M. A., et al. (2020). The ACC-deaminase producing bacterium Variovorax sp. CT7.15 as a tool for improving Calicotome villosa nodulation and growth in Arid regions of Tunisia. Microorganisms 8:541. doi: 10.3390/microorganisms8040541
Blin, K., Medema, M. H., Kottmann, R., Lee, S. Y., and Weber, T. (2017). The antiSMASH database, a comprehensive database of microbial secondary metabolite biosynthetic gene clusters. Nucleic Acids Res. 45, D555–D559.
Bolger, A. M., Lohse, M., and Usadel, B. (2014). Trimmomatic: a flexible trimmer for illumina sequence data. Bioinformatics 30, 2114–2120. doi: 10.1093/bioinformatics/btu170
Caporaso, J. G., Bittinger, K., Bushman, F. D., DeSantis, T. Z., Andersen, G. L., and Knight, R. (2010a). PyNAST: a flexible tool for aligning sequences to a template alignment. Bioinformatics 26, 266–267. doi: 10.1093/bioinformatics/btp636
Caporaso, J. G., Kuczynski, J., Stombaugh, J., Bittinger, K., Bushman, F. D., Costello, E. K., et al. (2010b). QIIME allows analysis of high-throughput community sequencing data. Nat. Methods 7, 335–336.
Caporaso, J. G., Lauber, C. L., Walters, W. A., Berg-Lyons, D., Huntley, J., Fierer, N., et al. (2012). Ultra-high-throughput microbial community analysis on the illumina HiSeq and MiSeq platforms. ISME J. 6, 1621–1624. doi: 10.1038/ismej.2012.8
Carro, L., Pujic, P., Trujillo, M. E., and Normand, P. (2013). Micromonospora is a normal occupant of actinorhizal nodules. J. Biosciences 38, 685–693. doi: 10.1007/s12038-013-9359-y
Chaia, E. E., Wall, L. G., and Huss-Danell, K. (2010). Life in soil by the actinorhizal root nodule endophyte Frankia. A review. Symbiosis 51, 201–226. doi: 10.1007/s13199-010-0086-y
Champion, A., Lucas, M., Tromas, A., Vaissayre, V., Crabos, A., Diedhiou, I., et al. (2015). Inhibition of auxin signaling in Frankia species-infected cells in Casuarina glauca nodules leads to increased nodulation. Plant Physiol. 167, 1149–1157. doi: 10.1104/pp.114.255307
DeSantis, T. Z., Hugenholtz, P., Larsen, N., Rojas, M., Brodie, E. L., Keller, K., et al. (2006). Greengenes, a chimera-checked 16S rRNA gene database and workbench compatible with ARB. Appl. Environ. Microb 72, 5069–5072. doi: 10.1128/AEM.03006-05
Diagne, N., Diouf, D., Svistoonoff, S., Kane, A., Noba, K., Franche, C., et al. (2013). Casuarina in Africa: distribution, role and importance of arbuscular mycorrhizal, ectomycorrhizal fungi and Frankia on plant development. J. Environ. Manage 128, 204–209. doi: 10.1016/j.jenvman.2013.05.009
Diagne, N., Ngom, M., Djighaly, P. I., Ngom, D., Ndour, B., Cissokho, M., et al. (2015). “Remediation of heavy-metal-contaminated soils and enhancement of their fertility with actinorhizal plants,” in Heavy Metal Contamination of Soils, Soil Biology, eds I. Sherameti and A. Varma (Cham: Springer International Publishing), 355–366. doi: 10.1007/978-3-319-14526-6_19
Dudeja, S. S., Giri, R., Saini, R., Suneja-Madan, P., and Kothe, E. (2012). Interaction of endophytic microbes with legumes. J. Basic Microbiol. 52, 248–260. doi: 10.1002/jobm.201100063
Duponnois, R., Di dhiou, S., Chotte, J. L., and Sy, M. O. (2003). Relative importance of the endomycorrhizal and (or) ectomycorrhizal associations in Allocasuarina and Casuarina genera. Can. J. Microbiol. 49, 281–287. doi: 10.1139/w03-038
Echbab, H., Prin, Y., Ducousso, M., Nourissier-Mountou, S., Lahlou, H., and Arahou, M. (2004). Helper bacteria associated with Casuarina cunninghamiana-Frankia symbiosis: selection of isolates for their effects on plant growth in axenic conditions. Acta Bot. Gallica 151, 429–440. doi: 10.1080/12538078.2004.10515445
Edgar, R. C. (2010). Search and clustering orders of magnitude faster than BLAST. Bioinformatics 26, 2460–2461. doi: 10.1093/bioinformatics/btq461
Eljounaidi, K., Lee, S. K., and Bae, H. (2016). Bacterial endophytes as potential biocontrol agents of vascular wilt diseases – Review and future prospects. Biol. Control 103, 62–68. doi: 10.1016/j.biocontrol.2016.07.013
Gauthier, D., Diem, H. G., Dommergues, Y. R., and Ganry, F. (1985). Assessment of N-2 fixation by Casuarina-equisetifolia inoculated with Frankia Ors021001 Using N-15 Methods. Soil Biol. Biochem. 17, 375–379. doi: 10.1016/0038-0717(85)90076-8
Ghodhbane-Gtari, F., Essoussi, I., Chattaoui, M., Chouaia, B., Jaouani, A., Daffonchio, D., et al. (2010). Isolation and characterization of non-Frankia actinobacteria from root nodules of Alnus glutinosa, Casuarina glauca and Elaeagnus angustifolia. Symbiosis 50, 51–57. doi: 10.1007/s13199-009-0029-7
Ghodhbane-Gtari, F., Hurst, S. G. IV, Oshone, R., Morris, K., Abebe-Akele, F., Thomas, W. K., et al. (2014). Draft genome sequence of Frankia sp. Strain BMG5.23, a salt-tolerant nitrogen-fixing actinobacterium isolated from the root nodules of Casuarina glauca grown in Tunisia. Genome Announc. 2:e00520-14. doi: 10.1128/genomeA.00520-14
Ghodhbane-Gtari, F., Nouioui, I., Hezbri, K., Lundstedt, E., D’Angelo, T., McNutt, Z., et al. (2019). The plant-growth-promoting actinobacteria of the genus Nocardia induces root nodule formation in Casuarina glauca. Antonie Van Leeuwenhoek 112, 75–90. doi: 10.1007/s10482-018-1147-0
Ghodhbane-Gtari, F., and Tisa, L. S. (2014). “Ecology and physiology of Non-Frankia actinobacteria from actinorhizal plants,” in Plasticity in Plant-Growth-Promoting and Phytopathogenic Bacteria, ed. E. I. Katsey (New York, NY: Springer), 27–42.
Grimes, H. D., and Mount, M. S. (1984). Influence oF Pseudomonas putida on nodulation of Phaseolus vulgaris. Soil Biol. Biochem. 16, 27–30.
Gtari, M., Brusetti, L., Aouani, M. E., Daffonchio, D., and Boudabous, A. (2002). Frankia nodulating Alnus glutinosa and Casuarinaceae in Tunisia. Ann. Microbiol. 52, 145–153.
Gtari, M., Brusetti, L., Hassen, A., Mora, D., Daffonchio, D., and Boudabous, A. (2007). Genetic diversity among Elaeagnus compatible Frankia strains and sympatric-related nitrogen-fixing actinobacteria revealed by nifH sequence analysis. Soil Biol. Biochem. 39, 372–377. doi: 10.1016/j.soilbio.2006.07.005
Gtari, M., and Dawson, J. O. (2011). An overview of actinorhizal plants in Africa. Funct. Plant Biol. 38, 653–661. doi: 10.1071/FP11009
Guillén, G. M., Valdès, M., Liao, J., and Hisch, A. M. (1993). Identificacion de actinobacterias aisladas de nodulos de Casuarina, por tecnicas tradicionales y moleculares. Rev. Lat. Amer. Microbiol. 35, 195–200.
Gurevich, A., Saveliev, V., Vyahhi, N., and Tesler, G. (2013). QUAST: quality assessment tool for genome assemblies. Bioinformatics 29, 1072–1075. doi: 10.1093/bioinformatics/btt086
Hénia, L. (2008). Atlas De L’eau en Tunisie. Tunis: Université de Tunis, Faculté des Sciences Humaines et Sociales.
Huson, D. H., Auch, A. F., Qi, J., and Schuster, S. C. (2007). MEGAN analysis of metagenomic data. Genome Res. 17, 377–386.
Hyatt, D., Chen, G. L., LoCascio, P. F., Land, M. L., Larimer, F. W., and Hauser, L. J. (2010). Prodigal: prokaryotic gene recognition and translation initiation site identification. BMC Bioinformatics 11:119. doi: 10.1186/1471-2105-11-119
Iruthayathas, E. E., Gunasekaran, S., and Vlassak, K. (1983). Effect of combined inoculation of Azospirillum and Rhizobium on nodulation and N2-fixation of winged bean and soybean. Sci. Hortic. 20, 231–240.
Jain, J., and Singh, B. (2017). Phytase production and development of an ideal dephytinization process for amelioration of food nutrition using microbial phytases. Appl. Biochem. Biotech. 181, 1485–1495. doi: 10.1007/s12010-016-2297-z
Langille, M. G. I., Zaneveld, J., Caporaso, J. G., McDonald, D., Knights, D., Reyes, J. A., et al. (2013). Predictive functional profiling of microbial communities using 16S rRNA marker gene sequences. Nat. Biotechnol. 31, 814–821. doi: 10.1038/nbt.2676
Langmead, B., and Salzberg, S. L. (2012). Fast gapped-read alignment with Bowtie 2. Nat. Methods 9, 357–359. doi: 10.1038/nmeth.1923
Leite, J., Fischer, D., Rouws, L. F. M., Fernandes, P. I., Hofmann, A., Kublik, S., et al. (2017). Cowpea Nodules Harbor Non-rhizobial bacterial communities that are shaped by soil type rather than plant genotype. Front. Plant Sci. 7:2064. doi: 10.3389/fpls.2016.02064
Li, D.-M., and Alexander, M. (1990). Factors affecting co-inoculation with antibiotic-producing bacteria to enhance rhizobial colonization and nodulation. Plant Soil 129, 195–201. doi: 10.1007/bf00032413
Li, H., Handsaker, B., Wysoker, A., Fennell, T., Ruan, J., Homer, N., et al. (2009). The sequence alignment/map format and SAMtools. Bioinformatics 25, 2078–2079. doi: 10.1093/bioinformatics/btp352
Lozupone, C., and Knight, R. (2005). UniFrac: a new phylogenetic method for comparing microbial communities. Appl. Environ. Microbiol. 71, 8228–8235. doi: 10.1128/aem.71.12.8228-8235.2005
Lozupone, C. A., Hamady, M., Kelley, S. T., and Knight, R. (2007). Quantitative and qualitative beta diversity measures lead to different insights into factors that structure microbial communities. Appl. Environ. Microbiol. 73, 1576–1585. doi: 10.1128/AEM.01996-06
Lundberg, D. S., Lebeis, S. L., Paredes, S. H., Yourstone, S., Gehring, J., Malfatti, S., et al. (2012). Defining the core Arabidopsis thaliana root microbiome. Nature 488, 86–90. doi: 10.1038/nature11237
Lynd, L. R., Weimer, P. J., van Zyl, W. H., and Pretorius, I. S. (2002). Microbial cellulose utilization: fundamentals and biotechnology. Microbiol. Mol. Biol. Rev. 66, 506–577. doi: 10.1128/mmbr.66.3.506-577.2002
Maksimov, I. V., Abizgil’dina, R. R., and Pusenkova, L. I. (2011). Plant growth promoting rhizobacteria as alternative to chemical crop protectors from pathogens (review). Appl. Biochem. Microbiol. 47, 333–345. doi: 10.1134/s0003683811040090
Martínez-Hidalgo, P., and Hirsch, A. M. (2017). The nodule microbiome: N2-fixing rhizobia do not live alone. Phytobiomes 1, 70–82. doi: 10.1094/pbiomes-12-16-0019-rvw
Mayhood, P., and Mirza, B. S. (2021). Soybean root nodule and rhizosphere microbiome: distribution of rhizobial and nonrhizobial endophytes. Appl. Environ. Microbiol. 87:e02884-20. doi: 10.1128/AEM.02884-20
McMurdie, P. J., and Holmes, S. (2014). Waste not, want not: why rarefying microbiome data is inadmissible. PLos Comput. Biol. 10:e1003531. doi: 10.1371/journal.pcbi.1003531
Mielke, P. W. B. Jr., and Kenneth, J. (2007). Permutation Methods: A Distance Function Approach. New York, NY: Springer.
Mirza, B. S., Welsh, A., and Hahn, D. (2007). Saprophytic growth of inoculated Frankia sp in soil microcosms. FEMS Microbiol. Ecol. 62, 280–289. doi: 10.1111/j.1574-6941.2007.00382.x
Munns, R. (2005). Genes and salt tolerance: bringing them together. New Phytol. 167, 645–663. doi: 10.1111/j.1469-8137.2005.01487.x
Muthukumar, T., and Udaiyan, K. (2010). Growth response and nutrient utilization of Casuarina equisetifolia seedlings inoculated with bioinoculants under tropical nursery conditions. New For. 40, 101–118. doi: 10.1007/s11056-009-9186-z
Nascimento, F. X., Tavares, M. J., Franck, J., Ali, S., Glick, B. R., and Rossi, M. J. (2019). ACC deaminase plays a major role in Pseudomonas fluorescens YsS6 ability to promote the nodulation of alpha- and Betaproteobacteria rhizobial strains. Arch. Microbiol. 201, 817–822. doi: 10.1007/s00203-019-01649-5
Neeraja, C., Anil, K., Purushotham, P., Suma, K., Sarma, P., Moerschbacher, B. M., et al. (2010). Biotechnological approaches to develop bacterial chitinases as a bioshield against fungal diseases of plants. Crit. Rev. Biotechnol. 30, 231–241. doi: 10.3109/07388551.2010.487258
Niner, B. M., Brandt, J. P., Villegas, M., Marshall, C. R., Hirsch, A. M., and Valdes, M. (1996). Analysis of partial sequences of genes coding for 16S rRNA of actinomycetes isolated from Casuarina equisetifolia nodules in Mexico. Appl. Environ. Microbiol. 62, 3034–3036. doi: 10.1128/aem.62.8.3034-3036.1996
Normand, P., Benson, D. R., Berry, A. M., and Tisa, L. S. (2014). “Family Frankiaceae,” in The Prokaryote – Actinobacteria, eds E. Rosenberg, E. F. DeLong, S. Lory, E. Stackebrandt, and F. Thompson (Berlin: Springer-Verlag), 339–356. doi: 10.1007/978-3-642-30138-4_183
Oksanen, J., Kindt, R., Legendre, P., O’Hara, B., Stevens, M. H. H., and Oksanen, M. J. (2016). The vegan package. Commun. Ecol. Pack. 10:719.
Orozco-Mosqueda, M. D., Glick, B. R., and Santoyo, G. (2020). ACC deaminase in plant growth-promoting bacteria (PGPB): an efficient mechanism to counter salt stress in crops. Microbiol. Res. 235:126439. doi: 10.1016/j.micres.2020.126439
Paulson, J. N., Stine, O. C., Bravo, H. C., and Pop, M. (2013). Differential abundance analysis for microbial marker-gene surveys. Nat. Methods 10, 1200–1202. doi: 10.1038/nmeth.2658
Potgieter, L. J., Richardson, D. M., and Wilson, J. R. U. (2014). Casuarina: biogeography and ecology of an important tree genus in a changing world. Biol. Invas. 16, 609–633. doi: 10.1007/s10530-013-0613-x
Quinlan, A. R., and Hall, I. M. (2010). BEDTools: a flexible suite of utilities for comparing genomic features. Bioinformatics 26, 841–842. doi: 10.1093/bioinformatics/btq033
Rengasamy, P. (2006). World salinization with emphasis on Australia. J. Exp. Bot. 57, 1017–1023. doi: 10.1093/jxb/erj108
Rideout, J. R., He, Y., Navas-Molina, J. A., Walters, W. A., Ursell, L. K., Gibbons, S. M., et al. (2014). Subsampled open-reference clustering creates consistent, comprehensive OTU definitions and scales to billions of sequences. PeerJ 2:e545. doi: 10.7717/peerj.545
Ryan, R. P., Germaine, K., Franks, A., Ryan, D. J., and Dowling, D. N. (2008). Bacterial endophytes: recent developments and applications. FEMS Microbiol. Lett. 278, 1–9. doi: 10.1111/j.1574-6968.2007.00918.x
Sanginga, N., Danso, S. K. A., and Bowen, G. D. (1989). Nodulation and growth-response of Allocasuarina and Casuarina Species to phosphorus fertilization. Plant Soil 118, 125–132. doi: 10.1007/bf02232797
Santoyo, G., Moreno-Hagelsieb, G., Orozco-Mosqueda Mdel, C., and Glick, B. R. (2016). Plant growth-promoting bacterial endophytes. Microbiol. Res. 183, 92–99.
Sayed, W. F. (2011). Improving Casuarina growth and symbiosis with Frankia under different soil and environmental conditions-review. Folia Microbiol. 56, 1–9. doi: 10.1007/s12223-011-0002-8
Schwartz, A. R., Ortiz, I., Maymon, M., Herbold, C. W., Fujishige, N. A., Vijanderan, J. A., et al. (2013). Bacillus simplex—a little known PGPB with anti-fungal activity—alters pea legume root architecture and nodule morphology when coinoculated with Rhizobium leguminosarum bv. viciae. Agronomy 3, 595–620. doi: 10.3390/agronomy3040595
Seemann, T. (2014). Prokka: rapid prokaryotic genome annotation. Bioinformatics 30, 2068–2069. doi: 10.1093/bioinformatics/btu153
Sikorski, J., and Nevo, E. (2005). Adaptation and incipient sympatric speciation of Bacillus simplex under microclimatic contrast at “Evolution Canyons” I and II, Israel. Proc. Natl. Acad. Sci. U.S.A. 102, 15924–15929. doi: 10.1073/pnas.0507944102
Solans, M. (2007). Discaria trinervis – Frankia symbiosis promotion by saprophytic actinomycetes. J. Basic Microbiol. 47, 243–250. doi: 10.1002/jobm.200610244
Solans, M., and Vobis, G. (2003). Actinomycetes saprophíticos asociados a la rizósfera de Discaria trinervis. Ecol. Aust. 13, 97–107.
Solans, M., Vobis, G., Cassan, F., Luna, V., and Wall, L. G. (2011). Production of phytohormones by root-associated saprophytic actinomycetes isolated from the actinorhizal plant Ochetophila trinervis. World J. Microbiol. Biotechnol. 27, 2195–2202. doi: 10.1007/s11274-011-0685-7
Steane, D. A., Wilson, K. L., and Hill, R. S. (2003). Using matK sequence data to unravel the phylogeny of Casuarinaceae. Mol. Phylogenet. Evol. 28, 47–59. doi: 10.1016/s1055-7903(03)00028-9
Sugawara, S., Mashiguchi, K., Tanaka, K., Hishiyama, S., Sakai, T., Hanada, K., et al. (2015). Distinct characteristics of indole-3-acetic acid and phenylacetic acid, two common auxins in plants. Plant Cell Physiol. 56, 1641–1654. doi: 10.1093/pcp/pcv088
Tani, C., and Sasakawa, H. (2003). Salt tolerance of Casuarina equisetifolia and Frankia Ceq1 strain isolated from the root nodules of C-equisetifolia. Soil Sci. Plant Nutr. 49, 215–222.
Trujillo, M. E., Kroppenstedt, R. M., Fernandez-Molinero, C., Schumann, P., and Martinez-Molina, E. (2007). Micromonospora lupini sp nov and Micromonospora saelicesensis sp nov., isolated from root nodules of Lupinus angustifolius. Int. J. Syst. Evol. Micr. 57, 2799–2804. doi: 10.1099/ijs.0.65192-0
Trujillo, M. E., Kroppenstedt, R. M., Schumann, P., Carro, L., and Martinez-Molina, E. (2006). Micromonospora coriariae sp nov., isolated from root nodules of Coriaria myrtifolia. Int. J. Syst. Evol. Micr. 56, 2381–2385. doi: 10.1099/ijs.0.64449-0
Tunesi, S., Poggi, V., and Gessa, C. (1999). Phosphate adsorption and precipitation in calcareous soils: the role of calcium ions in solution and carbonate minerals. Nutr. Cycling Agroecosyst. 53, 219–227.
Valdes, M., Perez, N. O., Estrada de los Santos, P., Caballero-Mellado, J., Pena-Cabriales, J. J., Normand, P., et al. (2005). Non-Frankia actinomycetes isolated from surface-sterilized roots of Casuarina equisetifolia fix nitrogen. Appl. Environ. Microbiol. 71, 460–466. doi: 10.1128/AEM.71.1.460-466.2005
Weber, T., Blin, K., Duddela, S., Krug, D., Kim, H. U., Bruccoleri, R., et al. (2015). antiSMASH 3.0-a comprehensive resource for the genome mining of biosynthetic gene clusters. Nucleic Acids Res. 43, W237–W243. doi: 10.1093/nar/gkv437
Yang, Y. (1995). The effect of phosphorus on nodule formation and function in the Casuarina-Frankia symbiosis. Plant Soil 176, 161–169. doi: 10.1007/bf00017686
Zhang, B., Du, N., Li, Y., Shi, P., and Wei, G. (2018). Distinct biogeographic patterns of rhizobia and non-rhizobial endophytes associated with soybean nodules across China. Sci. Total Environ. 643, 569–578. doi: 10.1016/j.scitotenv.2018.06.240
Zhong, C. L., Gong, M. Q., Chen, Y., and Wang, F. Z. (1995). Inoculation of Casuarina with Ectomycorrhizal fungi, vesicular-arbuscular Mycorrhizal fungi and Frankia. Aciar. Proc. 62, 122–126.
Keywords: actinorhizal symbiosis, microbiome, endophyte, symbiont, plant-growth-promoting bacteria
Citation: Ghodhbane-Gtari F, D’Angelo T, Gueddou A, Ghazouani S, Gtari M and Tisa LS (2021) Alone Yet Not Alone: Frankia Lives Under the Same Roof With Other Bacteria in Actinorhizal Nodules. Front. Microbiol. 12:749760. doi: 10.3389/fmicb.2021.749760
Received: 29 July 2021; Accepted: 08 November 2021;
Published: 02 December 2021.
Edited by:
F. L. Consoli, University of São Paulo, BrazilReviewed by:
Monali C. Rahalkar, Agharkar Research Institute, IndiaCopyright © 2021 Ghodhbane-Gtari, D’Angelo, Gueddou, Ghazouani, Gtari and Tisa. This is an open-access article distributed under the terms of the Creative Commons Attribution License (CC BY). The use, distribution or reproduction in other forums is permitted, provided the original author(s) and the copyright owner(s) are credited and that the original publication in this journal is cited, in accordance with accepted academic practice. No use, distribution or reproduction is permitted which does not comply with these terms.
*Correspondence: Louis S. Tisa, bG91aXMudGlzYUB1bmguZWR1
†Present address: Timothy D’Angelo, Bigelow Laboratory for Ocean Sciences, East Boothbay, ME, United States
Disclaimer: All claims expressed in this article are solely those of the authors and do not necessarily represent those of their affiliated organizations, or those of the publisher, the editors and the reviewers. Any product that may be evaluated in this article or claim that may be made by its manufacturer is not guaranteed or endorsed by the publisher.
Research integrity at Frontiers
Learn more about the work of our research integrity team to safeguard the quality of each article we publish.