- Department of Biological Sciences, University of Southern California, Los Angeles, CA, United States
With developing understanding that host-associated microbiota play significant roles in individual health and fitness, taking an interdisciplinary approach combining microbiome research with conservation science is increasingly favored. Here we establish the scat microbiome of the imperiled Channel Island fox (Urocyon littoralis) and examine the effects of geography and captivity on the variation in bacterial communities. Using high throughput 16S rRNA gene amplicon sequencing, we discovered distinct bacterial communities in each island fox subspecies. Weight, timing of the sample collection, and sex contributed to the geographic patterns. We uncovered significant taxonomic differences and an overall decrease in bacterial diversity in captive versus wild foxes. Understanding the drivers of microbial variation in this system provides a valuable lens through which to evaluate the health and conservation of these genetically depauperate foxes. The island-specific bacterial community baselines established in this study can make monitoring island fox health easier and understanding the implications of inter-island translocation clearer. The decrease in bacterial diversity within captive foxes could lead to losses in the functional services normally provided by commensal microbes and suggests that zoos and captive breeding programs would benefit from maintaining microbial diversity.
Introduction
The gut microbiome plays an essential role in the health and fitness of its host (Mcfall-Ngai et al., 2013; McKenney et al., 2018). As such, it is becoming increasingly clear that to fully understand a host one must examine their associated microbiome and the forces driving variation among microbial communities. This approach is burgeoning in the field of conservation biology, where anthropogenic effects such as habitat fragmentation (Barelli et al., 2015) and increasing temperatures (Fontaine et al., 2018) have been shown to alter host-associated microbial communities. Integrating microbiome research into conservation science is beneficial at multiple levels from health monitoring (Kreisinger et al., 2015; Bragg et al., 2020) to microbiome transplants in captive individuals (Trevelline et al., 2019; Guo et al., 2020). Therefore, characterizing the current bacterial communities in endangered host species is crucial to identify future changes in microbial diversity.
Here we investigated the gut microbiome of the Channel Island fox, Urocyon littoralis, a charismatic species that was recently rescued from the brink of local extinction and that still faces continuing threats (Coonan et al., 2010). U. littoralis is a dwarfed insular canid with a distinct subspecies on six of the Channel Islands off the coast of southern California, United States (Coonan et al., 2010). Formerly endangered, four of the six inhabited islands went through extensive population crashes losing upward of 90–96% of foxes, but have since recovered in population size (Coonan et al., 2010). Only one subspecies is still federally listed as threatened but all island fox populations face continued threats of human activity and climate change, including introduced animals, novel pathogens, and worsening prolonged droughts (Doak et al., 2013; Gould and Andelt, 2013; Gustafson, 2020; Sanchez and Hudgens, 2020; Bakker et al., 2021). With low genetic diversity within populations (Robinson et al., 2016), the microbiome may be particularly important in disease identification and protection. In fact, changes in the microbiome have been linked to mange and ear tumors in U. littoralis (DeCandia et al., 2019, 2020). Potential differences in microbiomes between populations (signifying underlying adaptive differences) could have profound conservation implications considering proposed inter-island translocation of foxes (Funk et al., 2016).
In addition to being of conservation concern, this system presents a natural experiment to test the effect of geography on the gut microbiome because the foxes originated from one mainland source, and migrated to the other islands in a known pattern with little further movement between islands (Hofman et al., 2016). Variation in the size and topography of the islands cause differences in temperature, precipitation, and wind (Schoenherr et al., 2003) and likely has contributed to the divergence of the gut microbiome. These geographically differing variables, as well as differences in anthropogenic impacts, across the islands influence the diet (Cypher et al., 2011, 2014) and pathogen prevalence (Clifford et al., 2006; Harris et al., 2013; Gaffney et al., 2015; Vickers et al., 2015) across fox populations. Knowing that diet and the immune system affect the gut microbiome, this system provides the opportunity to identify a potential correlation between geography and gut bacterial communities.
Broadly, geographic isolation of populations can lead to divergence in microbiota over time due to drift and/or local adaptation. While geography has been shown to play a role in shaping the gut microbiome in a number of taxa (Linnenbrink et al., 2013; Moeller et al., 2017; Grieneisen et al., 2019; Colborn et al., 2020), few studies have looked at these effects in intraspecific island populations. Relative to interspecific comparisons, intraspecific comparisons can be expected to minimize differences in host factors such as physiology, behavior and ecology, and therefore allow better resolution of factors such as host sex and condition, as well as the effects of geography. Here we investigate a species with substantial geographic subdivision, allowing a strong test of the correspondence between geography and microbial variation.
Captivity, often a tool in conservation management, has also been shown to alter gut microbial diversity (Villers et al., 2008; Kohl et al., 2014; Cheng et al., 2015; Clayton et al., 2016; McKenzie et al., 2017; Wasimuddin et al., 2017). Conditions in captivity are markedly different than those in the wild including changes in diet, interspecific interactions, and health care, any of which can impact microbial community makeup. Across multiple taxa, studies have shown a decrease in microbial diversity in captive mammals compared to wild individuals (Kohl et al., 2014; Cheng et al., 2015; Clayton et al., 2016; McKenzie et al., 2017; Wasimuddin et al., 2017), which could impact individual health and/or release success (Wienemann et al., 2011). However, a recent study showed that the microbiome of Tasmanian devils was restored upon release in the wild (Chong et al., 2019). The effects of captivity on the microbial community appear to affect diet specialists more than generalists (Kohl et al., 2014) and carnivores more than herbivores (McKenzie et al., 2017). Currently, there are island foxes in multiple mainland zoos, and previously four wild populations went through captive breeding programs, yet it remains unknown how captivity affects the microbiome of U. littoralis.
The objective of this study is to characterize the bacterial communities in island fox scat and to test for associations between geography and bacterial composition. We also compare natural populations to captive populations. We predict that geography and captivity will correlate with shifts in bacterial community composition. For the effects of captivity our predictions are twofold: (1) we predict that bacterial diversity will decrease in captive foxes compared to their wild counterparts, as shown in other canids (McKenzie et al., 2017) and (2) we predict that bacterial communities from captive foxes will be more similar to other captive foxes than they are to those from the host source population. Understanding the drivers of the microbiome will provide additional tools for the conservation of these threatened animals and potentially provide a minimally invasive way to gauge health by relating microbial community profiles with fox health status.
Materials and Methods
Sampling and DNA Extraction
Scat samples were collected during annual trap monitoring of U. littoralis during the fall and winter of 2014–2015 by managers or contractors on the Channel Islands off the coast of southern California, United States (San Miguel Island, SMI; Santa Rosa Island, SRI; Santa Cruz Island, SCZ; Santa Catalina Island, CAT; San Clemente Island, SCL; San Nicolas Island, SNI; Figure 1). See Supplementary Table 1 for sample details. Samples were collected from traps of individual foxes, so the identity of the foxes were known. Scat samples from captive U. littoralis were collected opportunistically in 2014 (Orange County Zoo, OCZ; Santa Barbara Zoo, SBZ). Scat samples were collected in ethanol and then stored at −80°C until DNA extraction could be performed. Total genomic DNA was extracted from the scat samples using the E.Z.N.A Genomic DNA Isolation Kit for stool samples (Omega Bio-Tek, Norcross, GA, United States) following the manufacturer’s instructions with minor changes. Modifications to the protocol included incubating with Proteinase K for 20 min (an increase of 10 min) and eluting the DNA with 100 μL of elution buffer. Glass beads were used during the extractions to ensure bacteria were lysed, per the extraction kit’s instructions. Each batch was extracted alongside an experimental blank containing molecular grade water instead of sample to monitor potential contamination, and samples were extracted no more than 8 months after being collected in the field. Genomic DNA was quantified using an Invitrogen Qubit 2.0 Fluorometer (Carlsbad, CA, United States).
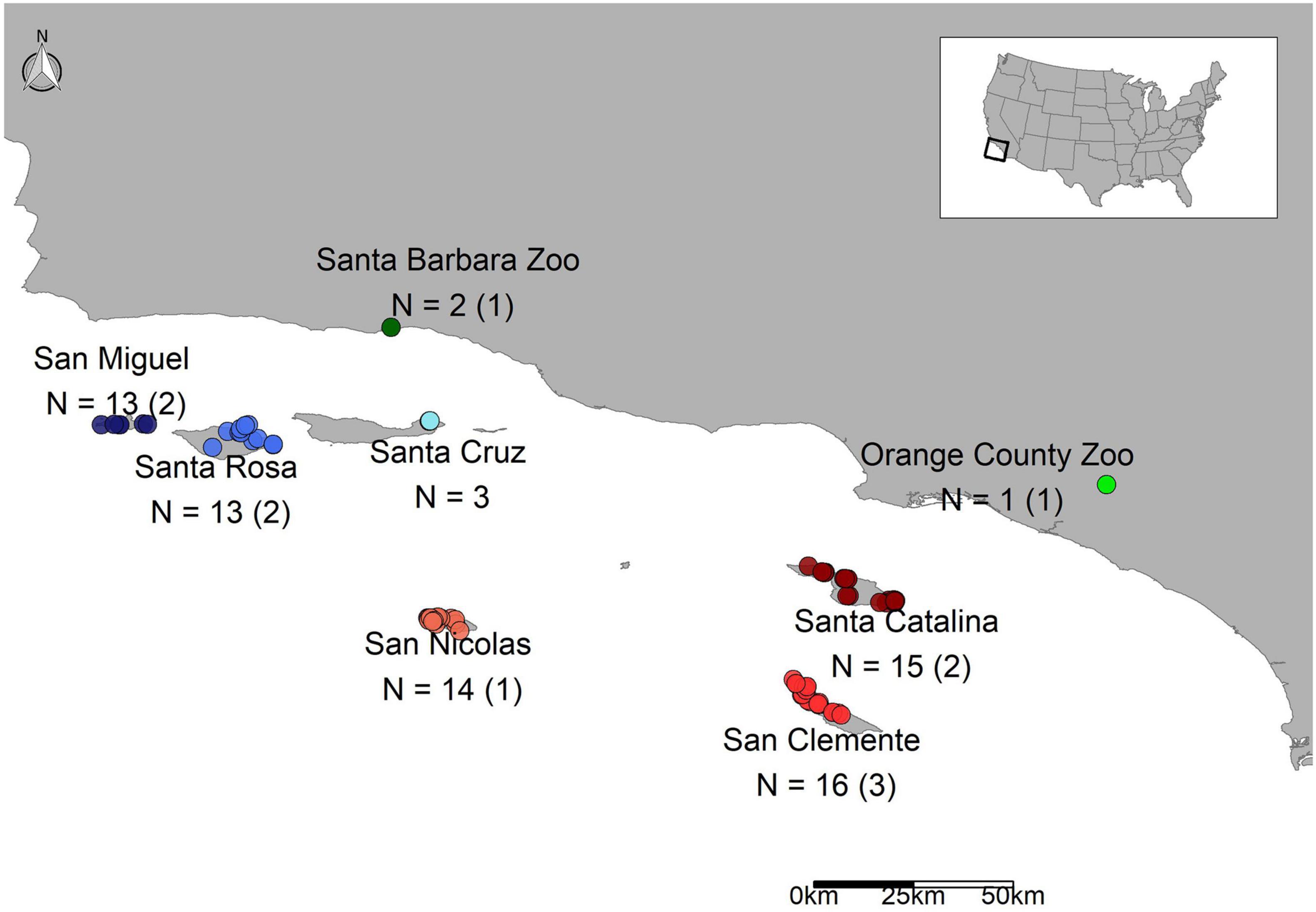
Figure 1. Map of scat samples across the Channel Islands and the mainland. The sample sizes are listed followed by the number of replicates, which are listed in parentheses.
Library Preparation and Sequencing
The V4 hypervariable region of the 16S rRNA gene was amplified from DNA extracted from U. littoralis scat using bacterial “universal” primers 515F and 806R, to which 4 ambiguous bases and variable barcodes on the forward primer and variable indexes on the reverse primers were added (Supplementary Table 2; Bartram et al., 2011; Caporaso et al., 2011). We followed a modified Earth Microbiome Project protocol (Gilbert et al., 2014). Briefly, the 16S V4 region was amplified in triplicate reactions using Phusion High-Fidelity DNA Polymerase (New England Biolabs, Ipswich, MA, United States) and then the reactions were cleaned with AMPure XP beads (Beckman Coulter, Brea, CA, United States) at a 1:1 ratio of beads to PCR product volume. The amplicon quantity was again evaluated with the Qubit and the quality was further evaluated with a Bioanalyzer analysis (Agilent Technologies, Santa Clara, CA, United States). Finally, samples were pooled equimolarly for sequencing. We sequenced 95 samples (Supplementary Table 1) as 300 bp paired-end reads on an Illumina MiSeq at UCLA’s Genotyping Core (Los Angeles, CA, United States). Blank samples (N = 5), technical replicates (repeat sequencing library preparations on the same DNA extraction; N = 10), and a positive control (an even mock community) were sequenced as part of the 95 samples. For the Orange County Zoo samples (sample 1 = OCZ.1a and OCZ.1b) and one Santa Barbara Zoo sample (sample 102003 = SBZ.2a and SBZ.2b) biological replicates were sequenced (meaning two different scat samples from the same organism). These two zoo individuals (SBZ.2a/b and OCZ.1a/b) were born in captivity but are descended from San Clemente Island foxes. One zoo sample (SBZ.1) was born on San Clemente Island but was brought into captivity.
Sequence Processing
Leading ambiguous bases were trimmed from forward reads with Trimmomatic v. 0.33 (Bolger et al., 2014). Barcodes were extracted with extract_barcodes.py, and samples were demultiplexed in QIIME1 v. 1.9.1 (Caporaso et al., 2010). Demultiplexed reads were merged into one artifact with QIIME1 and then imported back into QIIME2. Sequences were processed using denoise-paired in the DADA2 v. 2018.6.0 plugin (Callahan et al., 2016) in QIIME2. Processing included the following steps with default settings unless otherwise noted: denoising, trimming primers (–p-trim-left-f 19, –p-trim-left-r 20), removing chimeras and dereplicate reads, trimming forward reads to a minimum of 270 bp and reverse reads to a minimum of 210 bp based on quality scores, filtering out targets below those length cutoffs, and inferring amplicon sequence variants (ASVs) for both forward and reverse reads. Non-clustering methods that generate sequence variants are convincingly argued to be preferred over traditional 97% sequence clustering approaches due to their higher resolution and reproducibility (Callahan et al., 2017). Taxonomy was assigned using feature-classifier classify-sklearn (Pedregosa et al., 2011) in QIIME2 using the Greengenes database v. 13-8 (DeSantis et al., 2006), then non-target ASVs (mitochondria, archaea, and chloroplasts) were removed. The mock community sample was removed from the dataset for analysis.
Decontamination, Filtering, and Transformation
The data were imported into RStudio v. 1.1.456 (RStudio Team, 2018) with R v. 3.5.1 (R Core Team, 2016) using the Qiime2r v. 0.99.1 package (Bisanz, 2018) creating an object to use with the Bioconductor R package Phyloseq v. 1.24.2 (McMurdie and Holmes, 2013). Extraction blanks were addressed first by identifying potential contaminants using Decontam v. 1.0.0 (Davis et al., 2018). We used the “combined” method, which is based on frequency (variation based on DNA concentration) and prevalence (increased prevalence in negative controls) probabilities in tandem with a Fisher’s combined probabilities test. This method identified 12 ASVs as potential contaminants which were then removed from the dataset (Supplementary Table 3). After decontamination, extraction blanks were also removed from the dataset. Then technical replicates were merged using mean counts in Phyloseq [see Supplementary Figure 1 for principal coordinates analysis (PCoA) of technical replicates]. The ASV count table was transformed using a variance stabilizing transformation (Anders and Huber, 2010; McMurdie and Holmes, 2014) in DESeq2 v. 1.20.0 (type = “poscounts” to estimate size factors; Love et al., 2014) for beta diversity assessment. For use in Phyloseq (McMurdie and Holmes, 2013), negative transformed values were set to zero as advised by the authors1.
General Taxonomy and Beta Diversity Visualization
The dominant taxa present in the scat bacterial microbiome were identified based on ASV proportions (not transformed), and differences among sources were evaluated with analysis of variance (ANOVA) and Tukey HSD post hoc tests. First we agglomerated the count table at the phylum level for all phyla except for the Proteobacteria phylum which was split into its major classes for analysis due to its complexity (Hug et al., 2016), and phyla representing less than 5% of the ASVs were grouped together as “other.” With similar methods, we looked at the top genera with the largest proportions. To address beta diversity, a Bray–Curtis dissimilarity matrix was calculated on variance-stabilizing transformed data and used with hclust (method = ward.D2) to generate a hierarchical clustering dendrogram. The transformed data were also used to conduct a principal coordinates analysis (PCoA) based on Bray–Curtis dissimilarities with Phyloseq.
Testing for Group Differences and Differential Abundance
Testing for statistical differences in scat bacterial communities was done on the transformed ASV counts with Vegan v. 2.5-3 (Oksanen et al., 2020). Permutational ANOVAs were calculated using adonis (permutations = 99999) based on Bray–Curtis dissimilarity (Anderson, 2006, 2017) only on samples with no missing data (Supplementary Table 4A). The data were transformed separately for each dataset after samples with missing data were removed. We did this across all samples and then again for each island and the captive versus wild dataset. The assumption of homogeneity in dispersion within groups was tested with betadisper. We evaluated the best models by including all variables regardless of dispersion and then we sequentially removed variables with low variance explained (R2). The models were evaluated with Akaike information criterion (AIC), taking the lowest value as the best model that was used in the permutational ANOVA. The source code for the AIC method is available at Kdyson (2020). For the combined dataset that included all islands, we tested the effects of age, body condition, month and year collected, sex, source (each island or zoo), weight, and the extraction group in which the sample belonged. For each island, we evaluated the same variables excluding source and year collected when it did not vary from month collected (SNI). For the captive dataset, we included the following variables: captivity status, age, month and year collected, sex, and if the individual was born in captivity or born on an island then taken into captivity. Body condition is a common measurement scale used to gauge animal health (Coonan et al., 2014) that goes from emaciated (body condition = 1) to obese (body condition = 5) and was recorded by wildlife managers in the field. We then examined differential abundances in ASVs for comparisons with significant permutational ANOVA results using the R package DESeq2 and a significance level of 0.01 (with the input being the untransformed, but filtered, count table). We also looked at differentially abundant genera by first agglomerating the count table at the genus level. We looked at alpha diversity in comparisons with significant permutational ANOVA results by calculating Chao1 richness index and Shannon diversity index using untransformed count tables and plot_richness in Phyloseq to understand relative differences.
Results
Taxonomy
Bacteroidetes, Firmicutes, Fusobacteria, and Proteobacteria (classes Gammaproteobacteria and Betaproteobacteria) were the dominant phyla in the scat samples; the first two accounting for over 67% of the recovered sequences and the top 6 major taxa collectively accounting for about 94% of the total sequences in each group (Figure 2A). Seven major taxa were significantly different between sources. Firmicutes had the highest number of significant pairwise comparisons and was mainly elevated in zoo foxes compared to island foxes (Supplementary Table 5A and Supplementary Figure 2). Foxes from SMI and SNI were significantly different between 3 out of 7 major taxa, the most of any pairwise comparison (Supplementary Table 5A). Largely foxes from SMI had significantly elevated proportions of Fusobacteria (Supplementary Table 5A and Supplementary Figure 2). Bacteroidia made up the largest average proportion (37.8 ± 12.2%; mean ± 1SD) of identified classes followed by Clostridia (27.4 ± 10.0%). The same pattern held for order; Bacteroidales and Clostridiales made up the largest represented orders at comparable percentages to their respective classes. The top genera were Bacteroides and Fusobacterium accounting for over 38% of the recovered sequences (Supplementary Table 5B and Figure 2B). Of the top genera, 6 were significantly different between sources. Escherichia was elevated in foxes from CAT compared to SRI and the other southern islands of SCL and SNI. Most notably, however, Clostridium and Streptococcus were significantly elevated in captive foxes from OCZ compared to every other source (Supplementary Table 5B and Figure 2B).
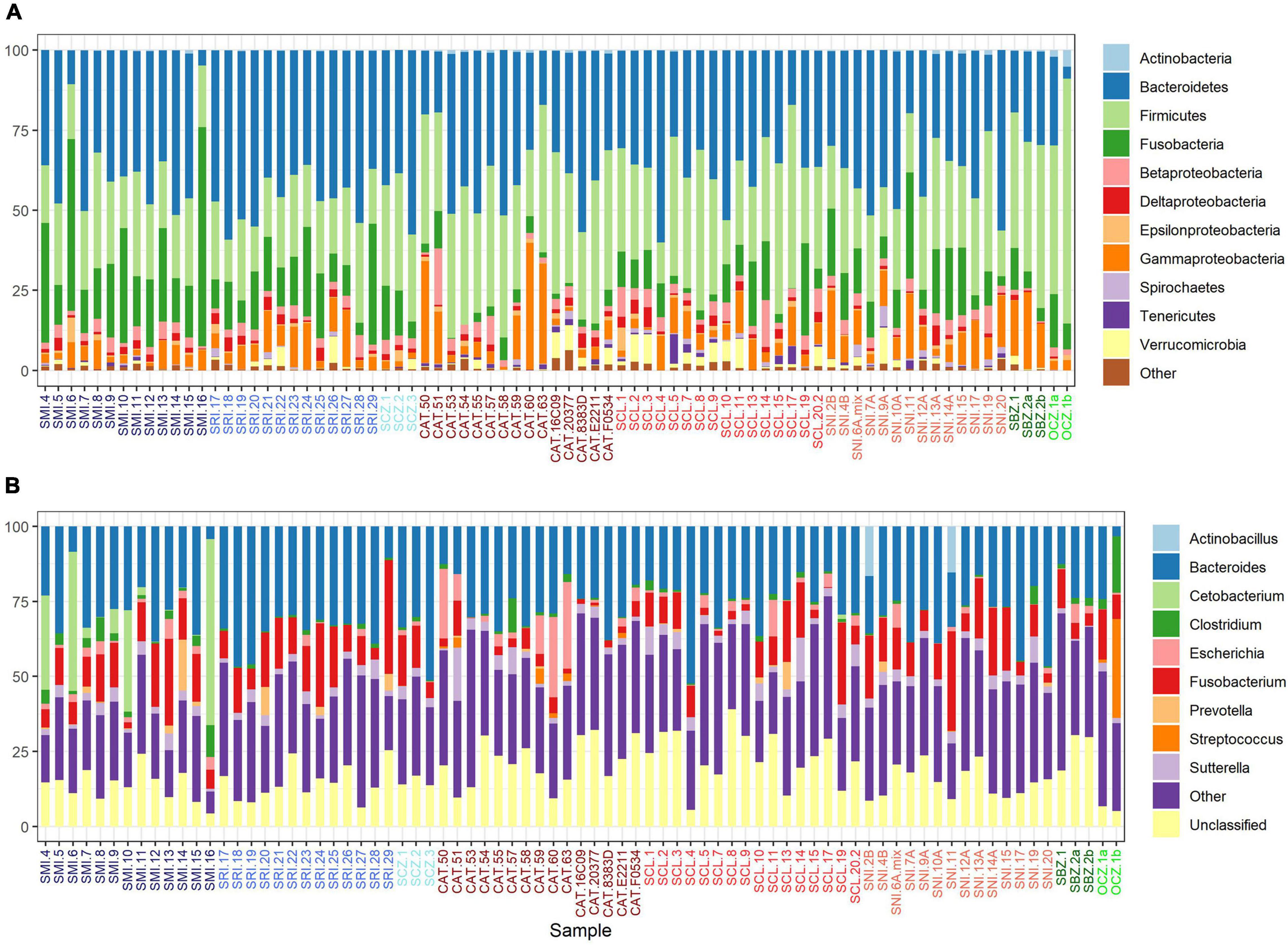
Figure 2. Percent relative abundance of the major taxa (A) and genera (B) recovered from U. littoralis scat per sample. Sample names are colored by source (island or zoo).
Between-Group Diversity Visualization
The bacterial communities largely clustered into two main groups based on geography (Figures 3A,B). We identified two main clusters based on the dendrogram (Figure 3A). One cluster is entirely composed of samples from the southern islands (SNI, SCL, and CAT), while the other contained all of the northern and zoo samples, as well as a subset of southern samples including about half of the SCL samples and two from SNI. In the PCoA (Figure 3B), axis 1 (16%) largely described the distance between the northern/zoo samples group and the southern islands group, including the aforementioned exceptions, and axis 2 (11.4%) predominantly described the variation within those groups.
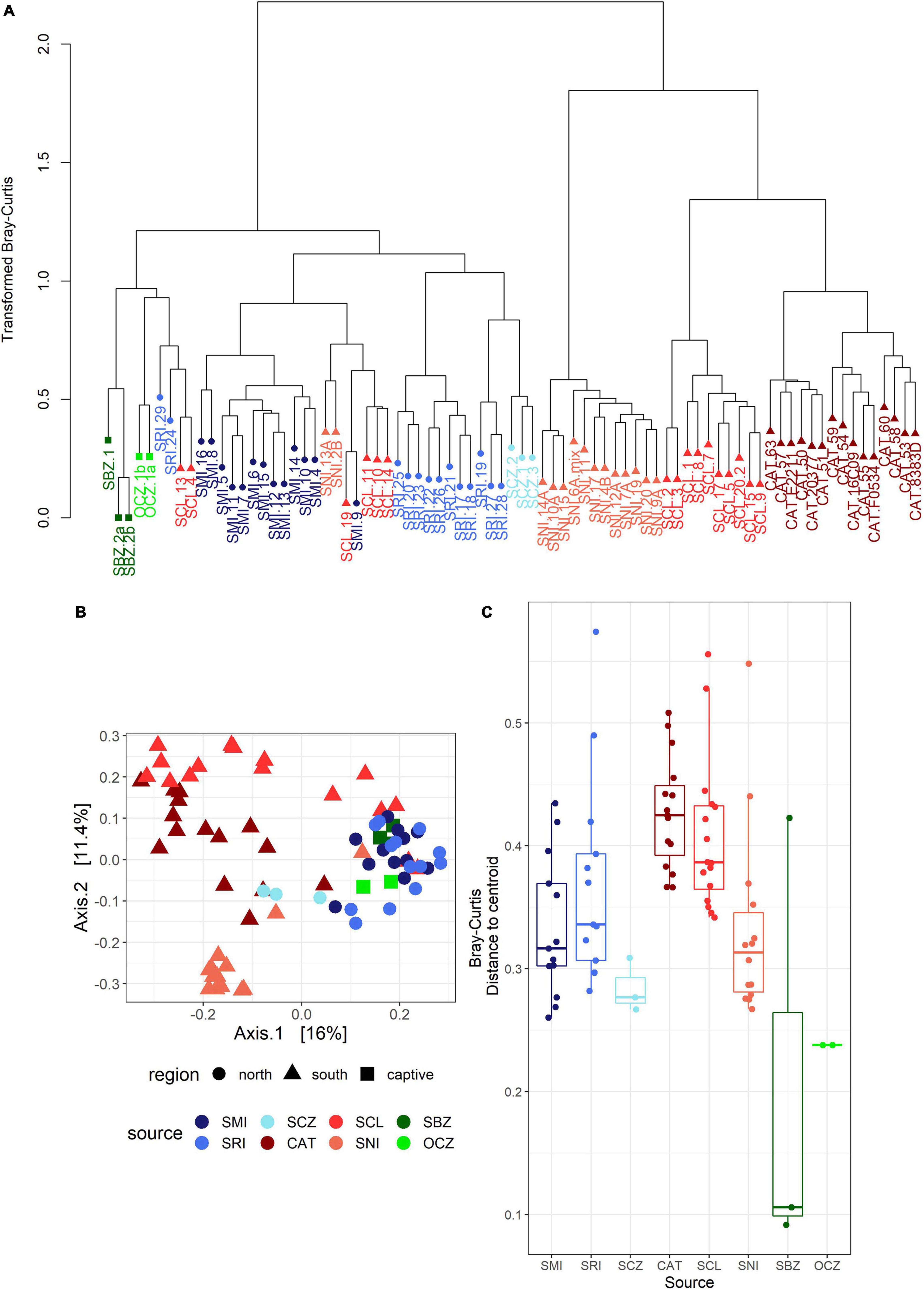
Figure 3. (A) Dendrogram of transformed data based on Bray–Curtis dissimilarity. Sample names are colored by island or zoo (see Figure 1) and shapes correspond to region: circle = northern islands, triangle = southern islands, and square = captive samples. (B) Principal coordinates analysis (PCoA) based on Bray–Curtis dissimilarity. The points are samples colored by source (island or zoo) and the shapes represent northern or southern Channel Islands and captive samples. (C) Boxplot of multivariate dispersion as measured by the distance to the centroid per sample and displayed by source.
Drivers of the Bacterial Communities
The strongest driver of differences among bacterial communities was source (Table 1 and Figure 3C). Across all samples, weight and month of sample collection also significantly influenced the bacterial communities (Table 1). Although, it is important to note that there is a known significant difference in body size among the islands (Wayne et al., 1991), which was also found in our weight data (ANOVAsource, P = 5.0 × 10–9). The highest mean weight was found on CAT, and SNI had the lowest mean weight. When broken out by island, month was the driving factor on SRI, sex the largest factor on CAT, and on SCL weight and year drove the difference in bacterial communities (Table 1 and Supplementary Figure 3). To look further into the influence of collection timing on SRI and SCL, we evaluated precipitation over the period assessed for each island (Supplementary Figure 4). Sex was also found to be the driving factor on SNI; however, the pattern was driven by one male, and when removed there were no significant factors (Table 1 and Supplementary Figure 3). We investigated the difference between the southern samples that grouped with the northern samples and those that did not and found the best model included weight, month collected, and the group in which the sample was extracted as significant drivers of bacterial community difference. For results from our model testing see Supplementary Tables 4B–H. It should be noted that not all variables were homogenously distributed, but the heterogeneity in the source term is likely biologically relevant (Supplementary Table 6 and Figure 3C). Further, SCZ did not have enough samples (N = 3) to conduct the permutational ANOVA, and there were no significant factors identified for SMI likely due to small sample size (N = 6) after removing samples with missing data.
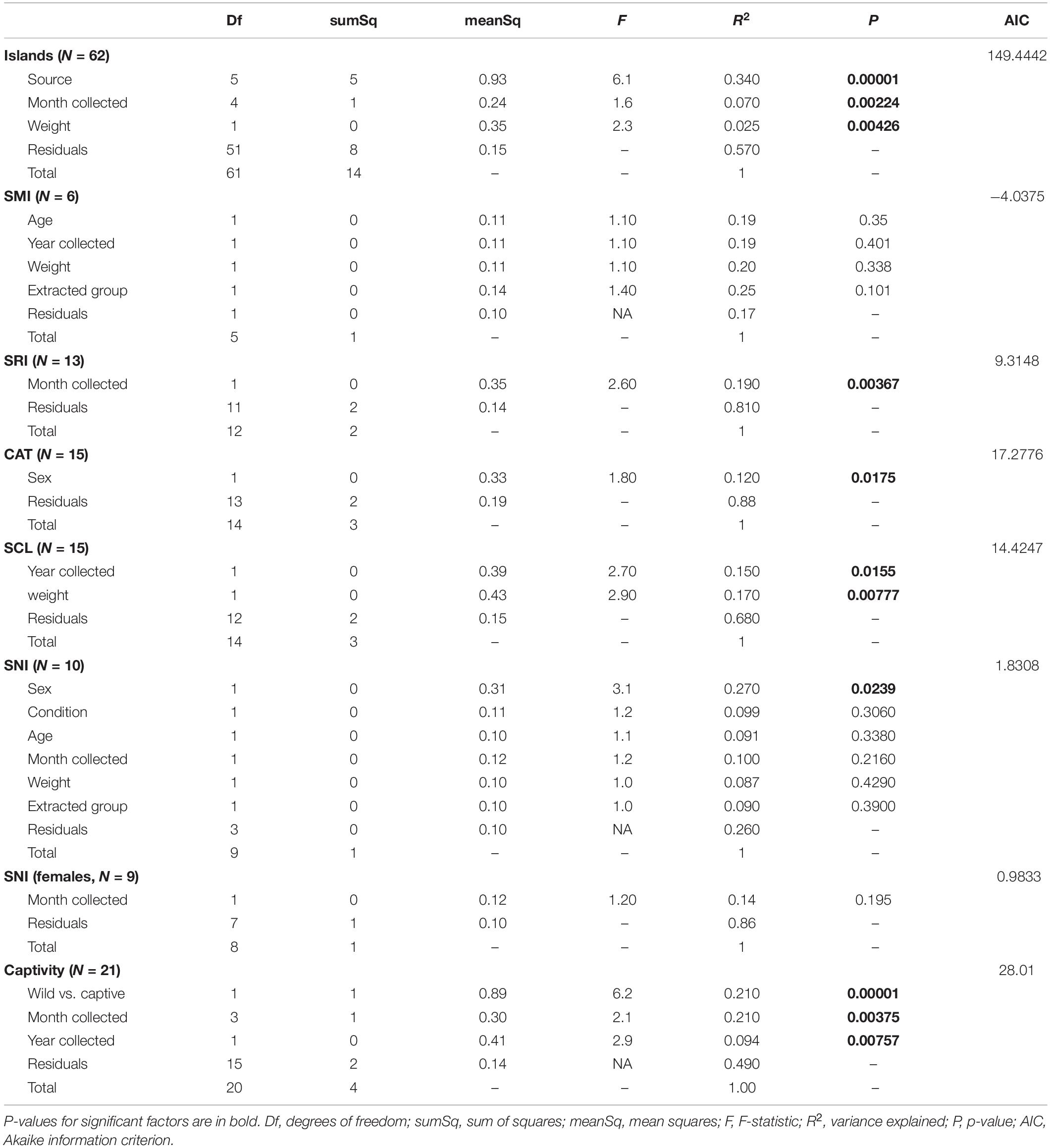
Table 1. Results from the permutational ANOVAs (Bray–Curtis dissimilarity, permutations = 99999) for the best models.
We found 173 differentially abundant ASVs significant at the α = 0.01 level in our significant factors across islands. On SRI we found 72% of the significantly differentially abundant ASVs were elevated in samples collected in November, while 28% of the ASVs were elevated in December (Table 2). On CAT we found 38% of the significantly differentially abundant ASVs were elevated in female samples, opposed to 62% of the ASVs being elevated in males (Table 2). Additionally, on SCL we found the majority of the significantly differentially abundant ASVs were elevated in samples collected from 2014 (87%) and heavier foxes (88%). Since weight was evaluated as a continuous variable, this result can be interpreted as an increase in ASV abundance with increasing weight. Both on SRI and SCL the genus Megamonas was significantly differentially abundant in samples collected in December and January (for additional differences at the genus level see Supplementary Table 7).
To explicitly test for the effect of captivity on the scat bacterial microbiome, we probed differences between the captive and SCL samples because all zoo individuals were either descendants of SCL foxes or born on the island and then taken into captivity (Figure 4). We found that the best model for these data included the captivity factor along with the month and year that the sample was collected, and all three terms were significant (Table 1). Body condition and weight could not be considered due to missing data. We found that the majority of the significantly differentially abundant ASVs were elevated in wild samples (81%) compared to captive (Table 2). There was a nearly equal number of significantly differentially abundant ASVs between sampled years, but more differentiated ASVs from samples collected in months later in the year. In fact, Megamonas was again seen as a significant player in samples from later months, which could be driven by the same result found in SCL samples alone (Supplementary Table 7).
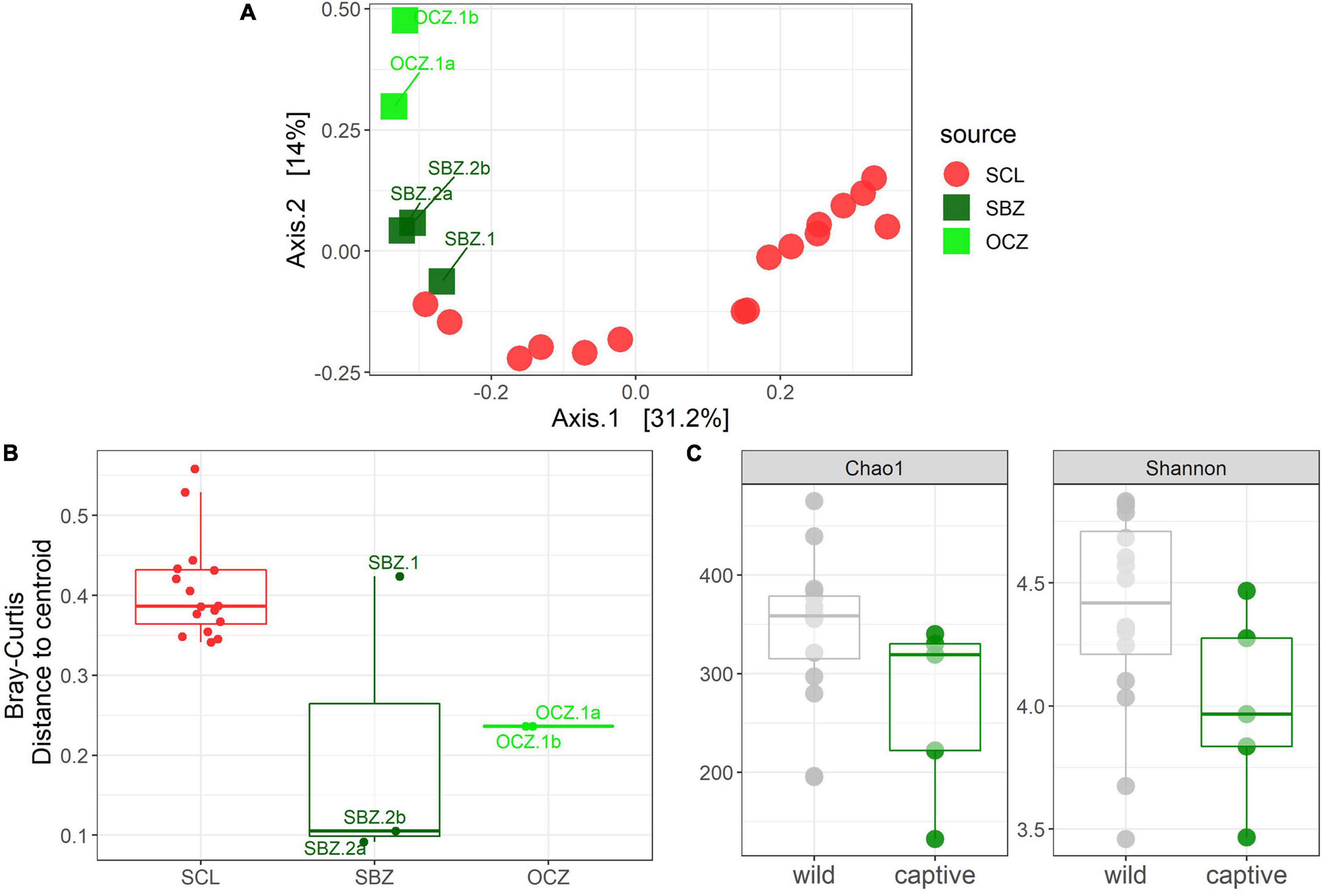
Figure 4. (A) Principal coordinates analysis (PCoA) based on Bray–Curtis dissimilarity of samples from SCL and captivity (SBZ, OCZ). (B) Boxplot of multivariate dispersion as measured by the distance to the centroid per sample and displayed by source. (C) Chao 1 and Shannon diversity metrics between wild and captive samples.
Patterns of Alpha Diversity
Statistical analyses on alpha diversity can be misleading (Willis, 2019), therefore we explore qualitative alpha diversity patterns to gain insight into the statistically significant factors we identified through the permutational multivariate analysis of variance (PERMANOVAs). When comparing across sources, scat bacterial communities from the three southern islands, especially CAT, generally had higher ASV richness and evenness compared to the northern Channel Islands (Supplementary Figure 5A). Across all samples analyzed, ASV richness and evenness peaked in October, while richness, but not evenness, trended toward increasing with weight (Supplementary Figures 5B,C). In bacterial communities from SRI, scat collected in November had higher richness and evenness compared to those collected in December (Supplementary Figure 5D). Males had higher evenness, but not richness, compared to females on CAT (Supplementary Figure 5E). Meanwhile, the evenness of bacterial communities on SCL declined with increasing weight while richness was constant (Supplementary Figure 5F). Both richness and evenness were higher in samples collected in 2014 compared to those collected in 2015 on SCL (Supplementary Figure 5G). Samples collected from captive foxes had lower richness and evenness than those collected from wild foxes (Figure 4C).
Discussion
Recent advances and cost efficiency in high-throughput sequencing has made culture-independent methods of surveying microbial communities tractable. Combined with the insight that host-associated microbiota may aid in rapid adaptation to environmental change (Alberdi et al., 2016), conservation biologists are increasingly using sequencing to assess microbiome diversity. We sought to characterize a baseline and identify determining factors in each isolated subspecies within the threatened U. littoralis by using high-throughput amplicon sequencing. Few studies address microbiome differences among geographically isolated intraspecific populations, yet such studies provide insight into the mechanisms driving the composition of commensal microbes. We report distinct bacterial communities on each island as well as between wild and captive foxes.
Geography Drives the Island Fox Microbiome
Geographic source is a large contributor to bacterial community composition in U. littoralis scat (Figure 3). This result is expected, given that many biotic and abiotic factors change with biogeography which have been previously shown to affect the bacterial microbiome (Linnenbrink et al., 2013). Overall, the scat bacterial communities of U. littoralis were made up of the usual mammalian gut taxa; Bacteroidetes, Firmicutes, Fusobacteria, and Proteobacteria are consistent, abundant players in the canid scat bacterial communities (Ley et al., 2008; Zhang and Chen, 2010). In other studies, bacterial community differences among populations have been shown to be driven by differences in diet (Barelli et al., 2015), which could also contribute to the differences we see across islands in our dataset. For example, the lower relative abundance of Firmicutes classes Bacilli and Clostridia on SMI and SRI, and the higher abundance of Fusobacteriales, compared to the southern islands could reflect a more protein-rich diet (Deitch et al., 1987; Hang et al., 2012; Finlayson-Trick et al., 2017). This hypothesis is consistent with data showing that foxes on SRI consume the lowest amount of fruit among the islands and that on SMI the only fruit consumed by foxes is that of a non-native plant and instead they rely heavily on insects (Cypher et al., 2014). The month the sample was collected likely reflects differences in diet as well, as discussed later in this section. Interestingly, we found a unidirectional pattern in which a subset of samples from southern islands grouped with northern samples, but none of our predictor variables were able to explain this pattern (data not shown). More work is therefore needed to understand this finding and other deviations from a previous phylogenetic tree (Robinson et al., 2016). In future work we will therefore conduct a formal test of phylosymbiosis to better understand the impact of host genetics on the microbiome.
While geography had the greatest impact on the scat bacterial communities, weight, timing of the collection, and sex also contributed to the geographic patterns. Weight was a significant factor that influenced the bacterial community within and among islands, although as previously noted body size (including weight) is significantly different among islands (Wayne et al., 1991). Additionally, weight was a significant factor within an island, specifically on SCL, where the majority of the significantly differentially abundant ASVs in heavier samples were associated with Clostridia. This result is concordant with previous work that showed Clostridiales to be more abundant in obese pet dogs (Handl et al., 2013). Obesity has also been associated with increased levels of Firmicutes (Ley et al., 2005, 2006; Turnbaugh et al., 2009; Riva et al., 2017), and while foxes are rarely overweight especially in the wild, we found a similar pattern of increased amounts of Firmicutes in heavier foxes. Weight is a well-studied factor, especially in humans and mice, that affects the microbiome (Bäckhed et al., 2004; Ley et al., 2005, 2006; Riva et al., 2017), and thus also an expected result of our study.
The timing of sample collection also significantly influenced the scat bacterial communities in U. littoralis both among and within islands, specifically on SCL and SRI. The top NCBI Blast hit for the ASV with the largest effect (largest log2 fold change) in foxes in 2014 on SCL was Parasutterella secunda, which is proposed to be a core taxon that has a role in bile acid maintenance and cholesterol metabolism (Ju et al., 2019). The top NCBI Blast hit for the ASV with the largest effect in foxes in 2015 on SCL was Bacteroides coprocola, which is also associated with bile and the breakdown of plant polysaccharides (Kitahara et al., 2005). At the genus level Megamonas, shown to ferment glucose to short chain fatty acids (Chevrot et al., 2008; Sakon et al., 2008), consistently came up associated with collection timing. Temporal changes in the gut microbiome can be associated with seasonal changes in diet (Ren et al., 2017). The winter is typically the rainy season in southern California, which drastically changes the flora and fauna for the subsequent months. Exemplary of this process, the total rainfall on SRI and SCL in December of 2014 was 9–25 times greater than the surrounding months [Supplementary Figure 4; (Western Regional Climate Center, 2019)] and likely changed food source availability for the foxes. The top NCBI Blast hit for the ASV with the largest effect in foxes in November on SRI was Diplorickettsia massiliensis, which is an obligate bacterium of arthropods (Mediannikov et al., 2010). The top NCBI Blast hit for the ASV with the largest effect in foxes in December on SRI was Rosenbergiella australiborealis, which was isolated from plant nectar (Lenaerts et al., 2014). The taxa that correlate with collection timing, especially on SRI, appear to be more associated with environmental drivers of diet than with host metabolism.
Sex contributed to the differences in bacterial communities, which is consistent with several mammalian gut studies (McKenna et al., 2008; Phillips et al., 2012; Nelson et al., 2013; Menke et al., 2017). While this result was significant on two islands, the pattern was likely driven by one male sample on SNI so we focused on the differences found between sexes in CAT individuals. The top differentiated bacteria between sexes appear to be associated with diet. The top NCBI Blast hit for the ASV with the largest effect in females was Fusobacterium mortiferum, which metabolizes sucrose (Pikis et al., 2002). The top NCBI Blast hit for the ASV with the largest effect in males was Faecalibacterium prausnitzii, which is a well-studied gut commensal that produces butyrate and is indicated as a sentinel in gut health (Sokol et al., 2008; Khan et al., 2012). While sex-biased differences in diet can explain differences in gut microbiota (Bolnick et al., 2014), the previous study of the island fox’s diet did not look at differences between sexes (Cypher et al., 2014) so more work is needed here to tease apart that relationship. Alternatively, sex hormones have been proposed as a mechanism for sex-specific microbiome differences (Org et al., 2016).
Captivity Alters the Fox Microbiome
Captivity significantly altered the scat microbiome of U. littoralis as evidenced by shifts in specific taxa (i.e., Firmicutes) and lower overall bacterial diversity. Despite the small sample sizes including biological replicates, this strong pattern emerged and is consistent with previous studies (Amato et al., 2013; Kohl et al., 2014; McKenzie et al., 2017; Guo et al., 2019; Schmidt et al., 2019). The top NCBI Blast hit for the ASV with the largest effect in wild foxes was Ruficoccus amylovorans, which is a diazotroph that reduces nitrate and degrades starch (Lin et al., 2017). In contrast, the top NCBI Blast hit for the ASV with the largest effect in captive foxes was Streptococcus alactolyticus, a potentially pathogenic species associated with diseases (ruminal acidosis, infective endocarditis, and colorectal cancer) in humans and animals (Jans et al., 2015; Jans and Boleij, 2018). Furthermore, several subspecies in the Streptococcus bovis/Streptococcus equinus Complex, to which S. alactolyticus belongs, have antimicrobial resistance (Jans and Boleij, 2018; Pompilio et al., 2019). While the mechanism of the bacterial shifts seen in this study remains unknown, previous studies have suggested changes in diet between captive individuals and their wild counterparts drive community differences in microbiota (Clayton et al., 2016; McKenzie et al., 2017; Metcalf et al., 2017; Greene et al., 2018; Oliveira et al., 2020). In the wild, island foxes are opportunistically omnivorous feeding on small mammals, insects, and native fruits (Cypher et al., 2014), while individuals in captivity are fed food items not found on the Channel Islands [e.g., dog food, hard-boiled eggs, fruits, and vegetables; (Coonan et al., 2010)]. Our results point to the influence that captivity can have on the fox immune system and subsequently the scat bacterial community. Reduced gut microbiome diversity can affect nutrient absorption and immune system function, ultimately affecting the overall health of the organism (Wienemann et al., 2011; Amato, 2013; Bragg et al., 2020). While there is some evidence that captive animals can gain back lost microbes upon release into the wild (Chong et al., 2019), carnivores have been shown to be more susceptible to losing microbial diversity in captivity (Metcalf et al., 2017) and there is evidence that it is more difficult to gain back wild microbes with an increasing number of generations in captivity (Webster et al., 2011; Becker et al., 2014). Going forward it will be imperative to manage host-associated microbiomes for captive foxes, especially if they will be released into the wild. To encourage a more diverse gut microbiome in captivity, managers could increase microbial reservoirs in enclosures (i.e., Loudon et al., 2014), shift diets to be more diverse and mimic natural food items, and decrease the use of antibiotics, as presented in Chong et al. (2019). More intensive approaches, including using prebiotics and probiotics that reflect the wild microbiome and/or fecal transplants could also be included to maintain diverse gut microbes.
For the captive samples, the PCoA (Figure 4A) and multivariate dispersion (Figure 4B) both show that SBZ2 and OCZ1 (both born in captivity) are similar to each other, while SCZ1 (born on SCL) is more similar to SCL. This pattern, while not confirmed statistically, suggests that inoculation from the natal environment may be more important than current environmental effects, which warrants further study. This legacy effect has been well-characterized in humans (Bokulich et al., 2016) and identified in other taxa (Jacob et al., 2015; Martínez-García et al., 2016; Gillingham et al., 2019). Some organisms have been able to maintain 60–70% of their wild microbes in captivity (Kohl and Dearing, 2014; Kohl et al., 2017), which could suggest that animals born in the wild but housed in captivity may have better health outcomes than those born in captivity.
Conservation Implications
In this first assessment of the scat microbiome across all islands of the Channel Island fox, we found patterns largely driven by geography, with food availability emerging as a strong contributing factor. These results add to the mounting evidence of distinctions among the six subspecies. Discussions of a genetic rescue to augment island populations of low genetic diversity by introducing animals from islands of higher genetic diversity will have to consider the microbiome differences as they represent possible divergences in natural history (diet) and immune systems that could be incompatible with other island-specific pathogens (Kohl et al., 2018). Secondly, even though there is extremely low genome-wide diversity in U. littoralis, a recent study found no signs of inbreeding depression in skeletal morphological traits (Robinson et al., 2018). The prevailing thought is that, on some of the islands, foxes have been able to persist despite the negative consequences of small population sizes and low genetic diversity. Perhaps other factors, such as the microbiome, contribute to their longevity and health, and the plasticity of the microbiome contributes to acclimatizing and eventually adapting to demographic and environmental changes (Alberdi et al., 2016). The insights provided in this study attest to the potential that microbiome research has for informing conservation science.
Data Availability Statement
The dataset generated for this study can be found in the NCBI SRA (BioProject PRJNA560412). The R code and metadata for the analyses are available on GitHub https://github.com/NicoleAdams-sci/islandFox_microbiome.
Ethics Statement
Ethical review and approval was not required for the animal study because we did not conduct the field work. Instead, trained personnel from other institutions with appropriate permits collected the scat samples and later made them available for this study (see section “Acknowledgments”).
Author Contributions
SE and NA conceived and designed the study. NA and MB performed the laboratory work and analyzed the data. NA and SE wrote the manuscript. All authors contributed to the article and approved the submitted version.
Funding
This work was supported by a grant from The Offield Family Foundation and the University of Southern California Dornsife College of Letters, Arts and Sciences.
Conflict of Interest
The authors declare that the research was conducted in the absence of any commercial or financial relationships that could be construed as a potential conflict of interest.
Publisher’s Note
All claims expressed in this article are solely those of the authors and do not necessarily represent those of their affiliated organizations, or those of the publisher, the editors and the reviewers. Any product that may be evaluated in this article, or claim that may be made by its manufacturer, is not guaranteed or endorsed by the publisher.
Acknowledgments
This project was made possible by generous funding from the Offield Family Foundation. We are profoundly grateful to Xiaoming Wang for the original inspiration for our fox research, and for securing funding. A special thanks to Bob Wayne for counsel on developing this specific project. We thank the following people and institutions for their sample contributions: Tim Coonan and Angela Guglielmino formerly at the National Parks Service, Julie King formerly at the Catalina Island Conservancy, Francesca Ferrara and Melissa Booker with the United States Navy, and Daniel Biteman at the Institute for Wildlife Studies, Erin Boydston of United States Geological Survey, Estelle Sandhaus at the Santa Barbara Zoo, David Place from the Orange County Zoo, and Donald Richardson at the California Living Museum. Thanks to Lauren Stoneburner for assistance with DNA extractions. More thanks to David Needham for the mock community and to Michael Lee for his guiding materials, and to both for comments on an earlier draft and general counsel.
Supplementary Material
The Supplementary Material for this article can be found online at: https://www.frontiersin.org/articles/10.3389/fmicb.2021.748323/full#supplementary-material
Abbreviations
ANOVA, analysis of variance; ASV, amplicon sequencing variant; CAT, Santa Catalina Island; PERMANOVA, permutational multivariate analysis of variance; SCL, San Clemente Island; SCZ, Santa Cruz Island; SMI, San Miguel Island; SNI, San Nicolas Island; SRI, Santa Rosa Island.
Footnotes
References
Alberdi, A., Aizpurua, O., Bohmann, K., Zepeda-mendoza, M. L., and Gilbert, M. T. P. (2016). Do vertebrate gut metagenomes confer rapid ecological adaptation? Trends Ecol. Evol. 31, 689–699. doi: 10.1016/j.tree.2016.06.008
Amato, K. R. (2013). Co-evolution in context: The importance of studying gut microbiomes in wild animals. Microbiome Sci. Med. 1, 10–29. doi: 10.2478/micsm-2013-0002
Amato, K. R., Yeoman, C. J., Kent, A., Righini, N., Carbonero, F., Estrada, A., et al. (2013). Habitat degradation impacts black howler monkey (Alouatta pigra) gastrointestinal microbiomes. ISME J. 7, 1344–1353. doi: 10.1038/ismej.2013.16
Anders, S., and Huber, W. (2010). Differential expression analysis for sequence count data. Genome Biol. 11:R106. doi: 10.1038/npre.2010.4282.1
Anderson, M. J. (2006). Distance-based tests for homogeneity of multivariate dispersions. Biometrics 62, 245–253. doi: 10.1111/j.1541-0420.2005.00440.x
Anderson, M. J. (2017). Permutational multivariate analysis of variance (PERMANOVA). Wiley StatsRef Stat. Ref. Online, doi: 10.1002/9781118445112.stat07841
Bäckhed, F., Ding, H., Wang, T., Hooper, L. V., Gou, Y. K., Nagy, A., et al. (2004). The gut microbiota as an environmental factor that regulates fat storage. Proc. Natl. Acad. Sci. U. S. A. 101, 15718–15723. doi: 10.1073/pnas.0407076101
Bakker, V. J., Doak, D. F., and Ferrara, F. J. (2021). Understanding extinction risk and resilience in an extremely small population facing climate and ecosystem change. Ecosphere 12:e03724. doi: 10.1002/ECS2.3724
Barelli, C., Albanese, D., Donati, C., Pindo, M., Dallago, C., Rovero, F., et al. (2015). Habitat fragmentation is associated to gut microbiota diversity of an endangered primate: implications for conservation. Sci. Rep. 5:14862. doi: 10.1038/srep14862
Bartram, A. K., Lynch, M. J., Stearns, J. C., Moreno-hagelsieb, G., and Neufeld, J. D. (2011). Generation of multi-million 16S rRNA gene libraries from complex microbial communities by assembling paired-end Illumina reads. Appl. Environ. Microbiol. 77, 3846–3852. doi: 10.1128/AEM.02772-10
Becker, M. H., Richards-Zawacki, C. L., Gratwicke, B., and Belden, L. K. (2014). The effect of captivity on the cutaneous bacterial community of the critically endangered Panamanian golden frog (Atelopus zeteki). Biol. Conserv. 176, 199–206. doi: 10.1016/j.biocon.2014.05.029
Bisanz, J. E. (2018). qiime2R: Importing QIIME2 Artifacts and Associated Data Into R Sessions. Available Online at: https://github.com/jbisanz/qiime2R (accessed October 8, 2018).
Bokulich, N. A., Chung, J., Battaglia, T., Henderson, N., Jay, M., Li, H., et al. (2016). Antibiotics, birth mode, and diet shape microbiome maturation during early life. Microbiome 8:343ra82. doi: 10.1126/scitranslmed.aad7121
Bolger, A. M., Lohse, M., and Usadel, B. (2014). Trimmomatic: a flexible trimmer for Illumina sequence data. Bioinformatics 30, 2114–2120. doi: 10.1093/bioinformatics/btu170
Bolnick, D. I., Snowberg, L. K., Hirsch, P. E., Lauber, C. L., Org, E., Parks, B., et al. (2014). Individual diet has sex-dependent effects on vertebrate gut microbiota. Nat. Commun. 5:4500. doi: 10.1038/ncomms5500
Bragg, M., Freeman, E. W., Lim, H. C., Songsasen, N., and Muletz-Wolz, C. R. (2020). Gut microbiomes differ among dietary types and stool consistency in the captive red wolf (Canis rufus). Front. Microbiol. 11:2777. doi: 10.3389/fmicb.2020.590212
Callahan, B. J., McMurdie, P. J., and Holmes, S. P. (2017). Exact sequence variants should replace operational taxonomic units in marker-gene data analysis. ISME J. 11, 2639–2643. doi: 10.1038/ismej.2017.119
Callahan, B. J., McMurdie, P. J., Rosen, M. J., Han, A. W., Johnson, A. J. A., and Holmes, S. P. (2016). DADA2: High-resolution sample inference from Illumina amplicon data. Nat. Methods 13, 581–583. doi: 10.1038/nmeth.3869
Caporaso, J. G., Kuczynski, J., Stombaugh, J., Bittinger, K., Bushman, F. D., Costello, E. K., et al. (2010). QIIME allows analysis of high-throughput community sequencing data. Nat. Methods 7, 335–336. doi: 10.1038/nmeth.f.303
Caporaso, J. G., Lauber, C. L., Walters, W. A., Berg-Lyons, D., Lozupone, C. A., Turnbaugh, P. J., et al. (2011). Global patterns of 16S rRNA diversity at a depth of millions of sequences per sample. Proc. Natl. Acad. Sci. U. S. A. 108, 4516–4522. doi: 10.1073/pnas.1000080107
Cheng, Y., Fox, S., Pemberton, D., Hogg, C., Papenfuss, A. T., and Belov, K. (2015). The Tasmanian devil microbiome-implications for conservation and management. Microbiome 3:76. doi: 10.1186/s40168-015-0143-0
Chevrot, R., Carlotti, A., Sopena, V., Marchand, P., and Rosenfeld, E. (2008). Megamonas rupellensis sp. nov., an anaerobe isolated from the caecum of a duck. Int. J. Syst. Evol. Microbiol. 58, 2921–2924. doi: 10.1099/IJS.0.2008/001297-0
Chong, R., Grueber, C. E., Fox, S., Wise, P., Barrs, V. R., Hogg, C. J., et al. (2019). Looking like the locals - gut microbiome changes post-release in an endangered species. Anim. Microbiome 1:8. doi: 10.1186/s42523-019-0012-4
Clayton, J. B., Vangay, P., Huang, H., Ward, T., Hillmann, B. M., Al-Ghalith, G. A., et al. (2016). Captivity humanizes the primate microbiome. Proc. Natl. Acad. Sci. 113, 10376–10381. doi: 10.1073/pnas.1521835113
Clifford, D. L., Mazet, J. A. K., Dubovi, E. J., Garcelon, D. K., Coonan, T. J., Conrad, P. A., et al. (2006). Pathogen exposure in endangered island fox (Urocyon littoralis) populations: Implications for conservation management. Biol. Conserv. 131, 230–243. doi: 10.1016/j.biocon.2006.04.029
Colborn, A. S., Kuntze, C. C., Gadsden, G. I., and Harris, N. C. (2020). Spatial variation in diet–microbe associations across populations of a generalist North American carnivore. J. Anim. Ecol. 89, 1952–1960. doi: 10.1111/1365-2656.13266
Coonan, T. J., Guglielmino, A., and Shea, R. (2014). Island Fox Recovery Program: Channel Islands National Park 2013 Annual Report. Natural Resource Report NPS/MEDN/NRR 2014/845. Fort Collins, CO: National Park Service.
Coonan, T. J., Schwemm, C. A., and Garcelon, D. K. (2010). Decline and Recovery of the Island Fox. New York City: Cambridge University Press.
Cypher, B. L., Madrid, A. Y., Van Horn Job, C. L., Kelly, E., Harrison, S. W. R., and Westall, T. L. (2011). Resource exploitation by island foxes: Implications for conservation. Nongame Wildl. Progr. Rep. 2011–04.
Cypher, B. L., Madrid, A. Y., Van Horn Job, C. L., Kelly, E. C., Harrison, S. W. R., and Westall, T. L. (2014). Multi-population comparison of resource exploitation by island foxes: Implications for conservation. Glob. Ecol. Conserv. 2, 255–266. doi: 10.1016/j.gecco.2014.10.001
Davis, N. M., Proctor, D. M., Holmes, S. P., Relman, D. A., and Callahan, B. J. (2018). Simple statistical identification and removal of contaminant sequences in marker-gene and metagenomics data. Microbiome 6:226. doi: 10.1186/s40168-018-0605-2
DeCandia, A. L., Brenner, L. J., King, J. L., and VonHoldt, B. M. (2020). Ear mite infection is associated with altered microbial communities in genetically depauperate Santa Catalina Island foxes (Urocyon littoralis catalinae). Mol. Ecol. 29, 1463–1475. doi: 10.1111/mec.15325
DeCandia, A. L., Leverett, K. N., and VonHoldt, B. M. (2019). Of microbes and mange: consistent changes in the skin microbiome of three canid species infected with Sarcoptes scabiei mites. Parasit. Vectors 12:488. doi: 10.1186/s13071-019-3724-0
Deitch, E. A., Winterton, J., and Berg, R. (1987). Effect of starvation, malnutrition, and trauma on the gastrointestinal tract flora and bacterial translocation. Arch. Surg. 122, 1019–1024. doi: 10.1001/archsurg.1987.01400210057008
DeSantis, T. Z., Hugenholtz, P., Larsen, N., Rojas, M., Brodie, E. L., Keller, K., et al. (2006). Greengenes, a chimera-checked 16S rRNA gene database and workbench compatible with ARB. Appl. Environ. Microbiol. 72, 5069–5072. doi: 10.1128/AEM.03006-05
Doak, D. F., Bakker, V. J., and Vickers, W. (2013). Using population viability criteria to assess strategies to minimize disease threats for an endangered carnivore. Conserv. Biol. 27, 303–314. doi: 10.1111/cobi.12020
Finlayson-Trick, E. C. L., Getz, L. J., Slaine, P. D., Thornbury, M., Lamoureux, E., Cook, J., et al. (2017). Taxonomic differences of gut microbiomes drive cellulolytic enzymatic potential within hind-gut fermenting mammals. PLoS One 12:e0189404. doi: 10.1371/journal.pone.0189404
Fontaine, S. S., Novarro, A. J., and Kohl, K. D. (2018). Environmental temperature alters the digestive performance and gut microbiota of a terrestrial amphibian. J. Exp. Biol. 221:jeb187559. doi: 10.1242/jeb.187559
Funk, W. C., Lovich, R. E., Hohenlohe, P. A., Hofman, C. A., Morrison, S. A., Sillett, T. S., et al. (2016). Adaptive divergence despite strong genetic drift: genomic analysis of the evolutionary mechanisms causing genetic differentiation in the island fox (Urocyon littoralis). Mol. Ecol. 25, 2176–2194. doi: 10.1111/mec.13605
Gaffney, P. M., Witte, C., Clifford, D. L., Imai, D. M., O’Brien, T. D., Trejo, M., et al. (2015). Systemic amyloid A amyloidosis in island foxes (Urocyon littoralis): Severity and risk factors. Vet. Pathol. 53, 637–647. doi: 10.1177/0300985815604725
Gilbert, J. A., Jansson, J. K., and Knight, R. (2014). The Earth Microbiome Project: successes and aspirations. BMC Biol. 12:69. doi: 10.1186/s12915-014-0069-1
Gillingham, M. A. F., Béchet, A., Cézilly, F., Wilhelm, K., Rendón-Martos, M., Borghesi, F., et al. (2019). Offspring microbiomes differ across breeding sites in a panmictic species. Front. Microbiol. 10:35. doi: 10.3389/fmicb.2019.00035
Gould, N. P., and Andelt, W. F. (2013). Effect of anthropogenically developed areas on spatial distribution of island foxes. J. Mammal. 94, 662–671. doi: 10.1644/12-MAMM-A-027.1
Greene, L. K., McKenney, E. A., O’Connell, T. M., and Drea, C. M. (2018). The critical role of dietary foliage in maintaining the gut microbiome and metabolome of folivorous sifakas. Sci. Rep. 8:14482. doi: 10.1038/s41598-018-32759-7
Grieneisen, L. E., Charpentier, M. J. E., Alberts, S. C., Blekhman, R., Bradburd, G., Tung, J., et al. (2019). Genes, geology and germs: Gut microbiota across a primate hybrid zone are explained by site soil properties, not host species. Proc. R. Soc. B Biol. Sci. 286:20190431. doi: 10.1098/rspb.2019.0431
Guo, W., Mishra, S., Wang, C., Zhang, H., Ning, R., Kong, F., et al. (2019). Comparative study of gut microbiota in wild and captive giant pandas (Ailuropoda melanoleuca). Genes (Basel). 10:827. doi: 10.3390/genes10100827
Guo, W., Ren, K., Ning, R., Li, C., Zhang, H., Li, D., et al. (2020). Fecal microbiota transplantation provides new insight into wildlife conservation. Glob. Ecol. Conserv. 24:e01234. doi: 10.1016/j.gecco.2020.e01234
Gustafson, G. G. (2020). Measuring density and activity of feral cats using camera traps on San clemente Island, CA. UC Agric. Nat. Resour. Proc. Vertebr. Pest Conf. 29:12510. doi: 10.1111/acv.12510
Handl, S., German, A. J., Holden, S. L., Dowd, S. E., Steiner, J. M., Heilmann, R. M., et al. (2013). Faecal microbiota in lean and obese dogs. FEMS Microbiol. Ecol. 84, 332–343. doi: 10.1111/1574-6941.12067
Hang, I., Rinttila, T., Zentek, J., Kettunen, A., Alaja, S., Apajalahti, J., et al. (2012). Effect of high contents of dietary animal-derived protein or carbohydrates on canine faecal microbiota. BMC Vet. Res. 8:90. doi: 10.1186/1746-6148-8-90
Harris, N. C., Coonan, T. J., King, J. L., and Dunn, R. R. (2013). Endemism in host-parasite interactions among island populations of an endangered species. Divers. Distrib. 19, 377–385. doi: 10.1111/ddi.12016
Hofman, C. A., Rick, T. C., Maldonado, J. E., Collins, P. W., Erlandson, J. M., Fleischer, R. C., et al. (2016). Tracking the origins and diet of an endemic island canid (Urocyon littoralis) across 7300 years of human cultural and environmental change. Quat. Sci. Rev. 146, 147–160. doi: 10.1016/j.quascirev.2016.06.010
Hug, L. A., Baker, B. J., Anantharaman, K., Brown, C. T., Probst, A. J., Castelle, C. J., et al. (2016). A new view of the tree of life. Nat. Microbiol. 1, 1–6. doi: 10.1038/nmicrobiol.2016.48
Jacob, S., Parthuisot, N., Vallat, A., Ramon-Portugal, F., Helfenstein, F., and Heeb, P. (2015). Microbiome affects egg carotenoid investment, nestling development and adult oxidative costs of reproduction in Great tits. Funct. Ecol. 29, 1048–1058. doi: 10.1111/1365-2435.12404
Jans, C., and Boleij, A. (2018). The road to infection: Host-microbe interactions defining the pathogenicity of Streptococcus bovis/Streptococcus equinus complex members. Front. Microbiol. 9:603. doi: 10.3389/fmicb.2018.00603
Jans, C., Meile, L., Lacroix, C., and Stevens, M. J. A. (2015). Genomics, evolution, and molecular epidemiology of the Streptococcus bovis/Streptococcus equinus complex (SBSEC). Infect. Genet. Evol. 33, 419–436. doi: 10.1016/j.meegid.2014.09.017
Ju, T., Kong, J. Y., Stothard, P., and Willing, B. P. (2019). Defining the role of Parasutterella, a previously uncharacterized member of the core gut microbiota. ISME J. 13, 1520–1534. doi: 10.1038/s41396-019-0364-5
Kdyson (2020). AICc_Permanova.R. Available online at: https://github.com/kdyson/R_Scripts/blob/master/AICc_PERMANOVA.R (accessed March 2020).
Khan, M. T., Duncan, S. H., Stams, A. J. M., Van Dijl, J. M., Flint, H. J., and Harmsen, H. J. M. (2012). The gut anaerobe Faecalibacterium prausnitzii uses an extracellular electron shuttle to grow at oxic-anoxic interphases. ISME J. 6, 1578–1585. doi: 10.1038/ismej.2012.5
Kitahara, M., Sakamoto, M., Ike, M., Sakata, S., and Benno, Y. (2005). Bacteroides plebeius sp. nov. and Bacteroides coprocola sp. nov., isolated from human faeces. Int. J. Syst. Evol. Microbiol. 55, 2143–2147. doi: 10.1099/ijs.0.63788-0
Kohl, K. D., Brun, A., Magallanes, M., Brinkerhoff, J., Laspiur, A., Acosta, J. C., et al. (2017). Gut microbial ecology of lizards: insights into diversity in the wild, effects of captivity, variation across gut regions and transmission. Mol. Ecol. 26, 1175–1189. doi: 10.1111/mec.13921
Kohl, K. D., and Dearing, M. D. (2014). Wild-caught rodents retain a majority of their natural gut microbiota upon entrance into captivity. Environ. Microbiol. Rep. 6, 191–195. doi: 10.1111/1758-2229.12118
Kohl, K. D., Skopec, M. M., and Dearing, M. D. (2014). Captivity results in disparate loss of gut microbial diversity in closely related hosts. Conserv. Physiol. 2:cou009. doi: 10.1093/conphys/cou009
Kohl, K. D., Varner, J., Wilkening, J. L., and Dearing, M. D. (2018). Gut microbial communities of American pikas (Ochotona princeps): Evidence for phylosymbiosis and adaptations to novel diets. J. Anim. Ecol. 87, 323–330. doi: 10.1111/1365-2656.12692
Kreisinger, J., Bastien, G., Hauffe, H. C., Marchesi, J., and Perkins, S. E. (2015). Interactions between multiple helminths and the gut microbiota in wild rodents. Philos. Trans. R. Soc. B Biol. Sci. 370:20140295. doi: 10.1098/rstb.2014.0295
Lenaerts, M., Álvarez-Pérez, S., de Vega, C., Van Assche, A., Johnson, S. D., Willems, K. A., et al. (2014). Rosenbergiella australoborealis sp. nov., Rosenbergiella collisarenosi sp. nov. and Rosenbergiella epipactidis sp. nov., three novel bacterial species isolated from floral. Syst. Appl. Microbiol. 37, 402–411. doi: 10.1016/j.syapm.2014.03.002
Ley, R. E., Bäckhed, F., Turnbaugh, P., Lozupone, C. A., Knight, R. D., and Gordon, J. I. (2005). Obesity alters gut microbial ecology. Proc. Natl. Acad. Sci. U. S. A. 102, 11070–11075. doi: 10.1073/pnas.0504978102
Ley, R. E., Hamady, M., Lozupone, C., Turnbaugh, P., Roy, R., Bircher, J. S., et al. (2008). Evolution of mammals and their gut microbes. Science 320, 1647–1651. doi: 10.1126/science.1155725.Evolution
Ley, R. E., Turnbaugh, P. J., Klein, S., and Gordon, J. I. (2006). Human gut microbes associated with obesity. Nature 444, 1022–1023. doi: 10.1038/nature4441021a
Lin, S. Y., Hameed, A., Liu, Y. C., Hsu, Y. H., Hung, M. H., Lai, W. A., et al. (2017). Ruficoccus amylovorans gen. nov., sp. nov., an amylolytic and nitrate-reducing diazotroph of the family Puniceicoccaceae. Int. J. Syst. Evol. Microbiol. 67, 956–962. doi: 10.1099/ijsem.0.001723
Linnenbrink, M., Wang, J., Hardouin, E. A., Kunzel, S., Metzler, D., and Baines, J. F. (2013). The role of biogeography in shaping diversity of the intestinal microbiota in house mice. Mol. Ecol. 22, 1904–1916. doi: 10.1111/mec.12206
Loudon, A. H., Woodhams, D. C., Parfrey, L. W., Archer, H., Knight, R., McKenzie, V., et al. (2014). Microbial community dynamics and effect of environmental microbial reservoirs on red-backed salamanders (Plethodon cinereus). ISME J. 8, 830–840. doi: 10.1038/ismej.2013.200
Love, M. I., Huber, W., and Anders, S. (2014). Moderated estimation of fold change and dispersion for RNA-seq data with DESeq2. Genome Biol. 15:550. doi: 10.1186/s13059-014-0550-8
Martínez-García, Á, Martín-Vivaldi, M., Ruiz-Rodríguez, M., Martínez-Bueno, M., Arco, L., Rodríguez-Ruano, S. M., et al. (2016). The microbiome of the uropygial secretion in hoopoes is shaped along the nesting phase. Microb. Ecol. 72, 252–261. doi: 10.1007/s00248-016-0765-1
Mcfall-Ngai, M., Hadfield, M. G., Bosch, T. C. G., Carey, H. V., Domazet-Lo, T., Douglas, A. E., et al. (2013). Animals in a bacterial world, a new imperative for the life sciences. PNAS 110, 3229–3236. doi: 10.1073/pnas.1218525110/-/DCSupplemental
McKenna, P., Hoffmann, C., Minkah, N., Aye, P. P., Lackner, A., Liu, Z., et al. (2008). The macaque gut microbiome in health, lentiviral infection, and chronic enterocolitis. PLoS Pathog. 4:e20. doi: 10.1371/journal.ppat.0040020
McKenney, E. A., Koelle, K., Dunn, R. R., and Yoder, A. D. (2018). The ecosystem services of animal microbiomes. Mol. Ecol. 27, 2164–2172. doi: 10.1111/mec.14532
McKenzie, V. J., Song, S. J., Delsuc, F., Prest, T. L., Oliverio, A. M., Korpita, T. M., et al. (2017). The effects of captivity on the mammalian gut microbiome. Integr. Comp. Biol. 57, 690–704. doi: 10.1093/icb/icx090
McMurdie, P. J., and Holmes, S. (2013). Phyloseq: An R package for reproducible interactive analysis and graphics of microbiome census data. PLoS One 8:e61217. doi: 10.1371/journal.pone.0061217
McMurdie, P. J., and Holmes, S. (2014). Waste not, want not: why rarefying microbiome data is inadmissible. PLoS Comput. Biol. 10:e1003531. doi: 10.1371/journal.pcbi.1003531
Mediannikov, O., Sekeyová, Z., Birg, M.-L., and Raoult, D. (2010). A novel obligate intracellular gamma-proteobacterium associated with Ixodid ticks, Diplorickettsia massiliensis, gen. nov., sp. nov. PLoS One 5:e11478. doi: 10.1371/journal.pone.0011478
Menke, S., Meier, M., Mfune, J. K. E., Melzheimer, J., Wachter, B., and Sommer, S. (2017). Effects of host traits and land-use changes on the gut microbiota of the Namibian black-backed jackal (Canis mesomelas). FEMS Microbiol. Ecol. 93, 1–16. doi: 10.1093/femsec/fix123
Metcalf, J. L., Song, S. J., Morton, J. T., Weiss, S., Seguin-Orlando, A., Joly, F., et al. (2017). Evaluating the impact of domestication and captivity on the horse gut microbiome. Sci. Rep. 7, 1–9. doi: 10.1038/s41598-017-15375-9
Moeller, A. H., Suzuki, T. A., Lin, D., Lacey, E. A., Wasser, S. K., and Nachman, M. W. (2017). Dispersal limitation promotes the diversification of the mammalian gut microbiota. Proc. Natl. Acad. Sci. U. S. A. 114, 13768–13773. doi: 10.1073/pnas.1700122114
Nelson, T. M., Rogers, T. L., Carlini, A. R., and Brown, M. V. (2013). Diet and phylogeny shape the gut microbiota of Antarctic seals: A comparison of wild and captive animals. Environ. Microbiol. 15, 1132–1145. doi: 10.1111/1462-2920.12022
Oksanen, J., Blanchet, F. G., Friendly, M., Kindt, R., Legendre, P., McGlinn, D., et al. (2020). vegan: Community Ecology Package. R Package Version 2.5-2. Available online at: https://CRAN.R-project.org/package=vegan
Oliveira, B. C. M., Murray, M., Tseng, F., and Widmer, G. (2020). The fecal microbiota of wild and captive raptors. Anim. Microbiome 2:15. doi: 10.1186/s42523-020-00035-7
Org, E., Mehrabian, M., Parks, B. W., Shipkova, P., Liu, X., Drake, T. A., et al. (2016). Sex differences and hormonal effects on gut microbiota composition in mice. Gut Microbes 7, 313–322. doi: 10.1080/19490976.2016.1203502
Pedregosa, F., Varoquaux, G., Gramfort, A., Michel, V., Thirion, B., Grisel, O., et al. (2011). Scikit-learn: Machine Learning in Python. Available Online at: http://scikit-learn.sourceforge.net. (accessed February 1, 2021).
Phillips, C. D., Phelan, G., Dowd, S. E., McDonough, M. M., Ferguson, A. W., Delton Hanson, J., et al. (2012). Microbiome analysis among bats describes influences of host phylogeny, life history, physiology and geography. Mol. Ecol. 21, 2617–2627. doi: 10.1111/j.1365-294X.2012.05568.x
Pikis, A., Immel, S., Robrish, S. A., and Thompson, J. (2002). Metabolism of sucrose and its five isomers by Fusobacterium mortiferum. Microbiology 148, 843–852. doi: 10.1099/00221287-148-3-843
Pompilio, A., Di Bonaventura, G., and Gherardi, G. (2019). An overview on Streptococcus bovis/Streptococcus equinus complex isolates: Identification to the species/subspecies level and antibiotic resistance. Int. J. Mol. Sci. 20:480. doi: 10.3390/ijms20030480
R Core Team (2016). R: A Language and Environment for Statistical Computing. Vienna: R Foundation for Statistical Computing.
Ren, T., Boutin, S., Humphries, M. M., Dantzer, B., Gorrell, J. C., Coltman, D. W., et al. (2017). Seasonal, spatial, and maternal effects on gut microbiome in wild red squirrels. Microbiome 5, 1–14. doi: 10.1186/s40168-017-0382-3
Riva, A., Borgo, F., Lassandro, C., Verduci, E., Morace, G., Borghi, E., et al. (2017). Pediatric obesity is associated with an altered gut microbiota and discordant shifts in Firmicutes populations. Environ. Microbiol. 19, 95–105. doi: 10.1111/1462-2920.13463
Robinson, J. A., Brown, C., Kim, B. Y., Lohmueller, K. E., and Wayne, R. K. (2018). Purging of strongly deleterious mutations explains long-term persistence and absence of inbreeding depression in island foxes. Curr. Biol. 28, 3487–3494. doi: 10.1016/J.CUB.2018.08.066
Robinson, J. A., Vecchyo, D. O., Fan, Z., Marsden, C. D., Lohmueller, K. E., and Wayne, R. K. (2016). Genomic flatlining in the endangered island fox. Curr. Biol. 26, 1183–1189. doi: 10.1016/j.cub.2016.02.062
Sakon, H., Nagai, F., Morotomi, M., and Tanaka, R. (2008). Sutterella parvirubra sp. nov. and Megamonas funiformis sp. nov., isolated from human faeces. Int. J. Syst. Evol. Microbiol. 58, 970–975. doi: 10.1099/IJS.0.65456-0
Sanchez, J. N., and Hudgens, B. R. (2020). Vaccination and monitoring strategies for epidemic prevention and detection in the Channel Island fox (Urocyon littoralis). PLoS One 15:e0232705. doi: 10.1371/JOURNAL.PONE.0232705
Schmidt, E., Mykytczuk, N., and Schulte-Hostedde, A. I. (2019). Effects of the captive and wild environment on diversity of the gut microbiome of deer mice (Peromyscus maniculatus). ISME J. 13, 1293–1305. doi: 10.1038/s41396-019-0345-8
Schoenherr, A. A., Feldmeth, R., and Emerson, M. J. (2003). Natural History of the Islands of California. Oakland: University of California Press.
Sokol, H., Pigneur, B., Watterlot, L., Lakhdari, O., Bermúdez-Humarán, L. G., Gratadoux, J. J., et al. (2008). Faecalibacterium prausnitzii is an anti-inflammatory commensal bacterium identified by gut microbiota analysis of Crohn disease patients. Proc. Natl. Acad. Sci. U. S. A. 105, 16731–16736. doi: 10.1073/pnas.0804812105
Trevelline, B. K., Fontaine, S. S., Hartup, B. K., and Kohl, K. D. (2019). Conservation biology needs a microbial renaissance: A call for the consideration of host-associated microbiota in wildlife management practices. Proc. Biol. Sci. 286:20182448. doi: 10.1098/rspb.2018.2448
Turnbaugh, P. J., Ridaura, V. K., Faith, J. J., Rey, F. E., Knight, R., and Gordon, J. I. (2009). The effect of diet on the human gut microbiome: A metagenomic analysis in humanized gnotobiotic mice. Sci. Transl. Med. 1:6ra14. doi: 10.1126/scitranslmed.3000322
Vickers, T. W., Clifford, D. L., Garcelon, D. K., King, J. L., Duncan, L., Gaffney, P. M., et al. (2015). Pathology and epidemiology of ceruminous gland tumors among endangered Santa Catalina Island foxes (Urocyon littoralis catalinae) in the Channel Islands, USA. PLoS One 10:e0143211. doi: 10.1371/journal.pone.0143211
Villers, L. M., Jang, S. S., Lent, C. L., Lewin-Koh, S.-C., and Norosoarinaivo, J. A. (2008). Survey and comparison of major intestinal flora in captive and wild ring-tailed lemur (Lemur catta) populations. Am. J. Primatol. 70, 175–184. doi: 10.1002/ajp
Wasimuddin, Menke, S., Melzheimer, J., Thalwitzer, S., Heinrich, S., Wachter, B., et al. (2017). Gut microbiomes of free-ranging and captive Namibian cheetahs: Diversity, putative functions and occurrence of potential pathogens. Mol. Ecol. 26, 5515–5527. doi: 10.1111/mec.14278
Wayne, R. K., George, S. B., Gilbert, D., Collins, P. W., and Steven, D. (1991). A morphologic and genetic study of the island fox, Urocyon littoralis. Evolution (N. Y) 45, 1849–1868. doi: 10.1111/j.1558-5646.1991.tb02692.x
Webster, N. S., Cobb, R. E., Soo, R., Anthony, S. L., Battershill, C. N., Whalan, S., et al. (2011). Bacterial community dynamics in the marine sponge Rhopaloeides odorabile under in situ and ex situ cultivation. Mar. Biotechnol. 13, 296–304. doi: 10.1007/s10126-010-9300-4
Western Regional Climate Center. (2019). Available online at: https://raws.dri.edu/ (accessed January 2019).
Wienemann, T., Schmitt-Wagner, D., Meuser, K., Segelbacher, G., Schink, B., Brune, A., et al. (2011). The bacterial microbiota in the ceca of Capercaillie (Tetrao urogallus) differs between wild and captive birds. Syst. Appl. Microbiol. 34, 542–551. doi: 10.1016/j.syapm.2011.06.003
Willis, A. D. (2019). Rarefaction, alpha diversity, and statistics. Front. Microbiol. 10:2407. doi: 10.3389/fmicb.2019.02407
Keywords: 16S rRNA gene, captivity, Channel Island fox, conservation, microbiota
Citation: Adams NE, Becker MA and Edmands S (2021) Effect of Geography and Captivity on Scat Bacterial Communities in the Imperiled Channel Island Fox. Front. Microbiol. 12:748323. doi: 10.3389/fmicb.2021.748323
Received: 03 August 2021; Accepted: 21 October 2021;
Published: 01 December 2021.
Edited by:
Lifeng Zhu, Nanjing Normal University, ChinaCopyright © 2021 Adams, Becker and Edmands. This is an open-access article distributed under the terms of the Creative Commons Attribution License (CC BY). The use, distribution or reproduction in other forums is permitted, provided the original author(s) and the copyright owner(s) are credited and that the original publication in this journal is cited, in accordance with accepted academic practice. No use, distribution or reproduction is permitted which does not comply with these terms.
*Correspondence: Nicole E. Adams, Tmljb2xlQWRhbXMuU2NpQGdtYWlsLmNvbQ==
†Present address: Nicole Adams, Department of Fisheries and Wildlife, Michigan State University, East Lansing, MI, United States Madeleine Becker, Department of Biosciences, School of Systems Biology, George Mason University, Fairfax, VA, United States