- Guangdong Provincial Key Laboratory of Insect Developmental Biology and Applied Technology, School of Life Sciences, Institute of Insect Science and Technology, South China Normal University, Guangzhou, China
Insect gut microbiota plays important roles in acquiring nutrition, preventing pathogens infection, modulating immune responses, and communicating with environment. Gut microbiota can be affected by external factors such as foods and antibiotics. Spodoptera frugiperda (Lepidoptera: Noctuidae) is an important destructive pest of grain crops worldwide. The function of gut microbiota in S. frugiperda remains to be investigated. In this study, we fed S. frugiperda larvae with artificial diet with antibiotic mixture (penicillin, gentamicin, rifampicin, and streptomycin) to perturb gut microbiota, and then examined the effect of gut microbiota dysbiosis on S. frugiperda gene expression by RNA sequencing. Firmicutes, Proteobacteria, Bacteroidetes, and Actinobacteria were the most dominant phyla in S. frugiperda. We found that the composition and diversity of gut bacterial community were changed in S. frugiperda after antibiotics treatment. Firmicutes was decreased, and abundance of Enterococcus and Weissella genera was dramatically reduced. Transcriptome analysis showed that 1,394 differentially expressed transcripts (DETs) were found between the control and antibiotics-treated group. The Gene Ontology (GO) and Kyoto Encyclopedia of Genes and Genomes (KEGG) results showed that antibiotics-induced dysbiosis affected many biological processes, such as energy production, metabolism, and the autophagy–lysosome signal pathway. Our results indicated that dysbiosis of gut microbiota by antibiotics exposure affects energy and metabolic homeostasis in S. frugiperda, which help better understand the role of gut microbiota in insects.
Introduction
Gut microbes play important roles in insect growth, development, and many physiological processes of the individual to maintain host health through regulating gene expression (Raymann et al., 2017; Schoeler and Caesar, 2019; Zhou et al., 2021). It has been shown that gut microbes are important in absorbing nutrition, protection from parasites and pathogens, modulation of immune responses, and communications with environment (Engel and Moran, 2013; Jang and Kikuchi, 2020). The dysbiosis of intestinal microbes can affect the metabolic process. In Drosophila, we have demonstrated the impact of microbiome and microbiota-derived sodium butyrate on host transcriptome and metabolome with multi-omics data (Zhou et al., 2021). Our studies revealed that the changes in composition and diversity of insect’s gut microbial community can influence important physiological functions in the host through regulating gene expression.
Gut microbes can regulate herbivore-induced defenses and enhance the insect adaptation to plants (Acevedo et al., 2017; Ugwu et al., 2020), which is important for insect survival and may provide key information for pest control. Gut microbial community is often influenced by weather, temperature, diet, and other environmental factors such as antibiotics (Schoeler and Caesar, 2019; Zhang et al., 2020). In social insects like the honeybee (Apis mellifera), the dysbiosis of gut microbial community caused by antibiotic exposure affects bee health and elevates mortality, in part due to increased susceptibility to pathogens (Powell et al., 2014; Raymann et al., 2017), whereas in the Chinese bumblebees (genus Bombus comprising eight species in Zhang’s paper), antibiotics can cause changes of the sugar metabolism and distributions of antibiotic-resistant genes in main bacterial symbiont Gilliamella (Zhang et al., 2021). In the gypsy moth Lymantria dispar (Lepidoptera: Lymantriidae), gut microbiota in the larval stage eliminated by antibiotics exposure abolished Bacillus thuringiensis insecticidal activity and influenced the immune responses (Broderick et al., 2006). In the taro caterpillar (Spodoptera litura, Lepidoptera: Noctuidae), it was found that artificial diet supplemented with antibiotic streptomycin sulfate affected gut microbial diversity; the activity of digestive enzymes was significantly increased while the activity of detoxifying enzymes was significantly decreased (Thakur et al., 2016). In the diamondback moth Plutella xylostella (Lepidoptera: Plutellidae), antibiotics exposure was related to reduced larval growth, high mortality, malformation of the prepupae, hindered pupation, and adult emergence (Lin et al., 2015).
Gut microbiota is also related to the metabolism, immune responses, and host defense against pathogens (Engel et al., 2012; Rozadilla et al., 2020). It was reported that the mortality caused by B. thuringiensis treatment was reduced when compared with the control (Broderick et al., 2006). In B. thuringiensis-infected Helicoverpa armigera, the mortality of larvae was only 10% when the gut microbiota was eliminated by the 250 and 500 μg/ml antibiotics cocktail (gentamicin, penicillin, rifampicin, and streptomycin), while the mortality of larvae fed on diets devoid of antibiotics was 83.33%. These results implied that the gut microbial community is related to larval death caused by B. thuringiensis treatment (Paramasiva et al., 2014).
Spodoptera frugiperda (Lepidoptera: Noctuidae), which is native in North and South America, has rapidly invaded African and China in recent years, and is an important destructive pest of grain crops worldwide (Goergen et al., 2016; Wu et al., 2019; Li X. et al., 2020). Due to the limited studies on the function of gut microbiota in S. frugiperda, it is urgent to investigate the role of gut microbiota in the growth and development of S. frugiperda, which can provide fundamental information for improving the efficiency of insecticides influenced by the insect gut microbiota. In this study, we characterized S. frugiperda larvae (3-day-old sixth instar larvae, L6D3) microbiota before and after exposure to antibiotic cocktail (gentamicin, penicillin, rifampicin, and streptomycin) by 16S ribosomal RNA (rRNA) amplicon high-throughput sequencing, and then further analyzed the influences of the microbial flora on the expression of host genes in the gut by RNA sequencing (RNA-seq). Our results showed that the diversity of gut microbiota was significantly reduced by antibiotics treatment, and the genes related to metabolism process were obviously affected.
Materials and Methods
Insects
The Spodoptera frugiperda larvae were purchased from Keyun Biocontrol Engineering Co., Ltd. (Jiyuan city) in China. The larvae were fed on artificial diet consisting of soybean powder, wheat germ, and yeast as described previously (Jia et al., 2009). Insects were reared at our laboratory for three generations at 27 ± 1°C with 70 ± 5% relative humidity, and the light:dark = 16 h:8 h photoperiod.
Antibiotics Exposure
The fourth generation of S. frugiperda larvae reared in our laboratory was selected for studying the effects of antibiotics on the gut microbiota of S. frugiperda. The newly hatched larvae were fed on artificial diet containing four antibiotics mixtures (consisting of 500 μg/ml each of penicillin, gentamicin, rifampicin, and streptomycin by dissolving 500 mg each antibiotic in 1 L artificial diet) for 14 days (from newly hatched larvae to 3-day-old sixth instar); the control larvae were fed on artificial diet without antibiotics.
Midgut Tissue Collection
Three-day-old sixth instar larvae (L6D3) surface was sterilized with 75% ethyl alcohol for 1 min and rinsed three times with sterile water. The midguts were dissected in sterile phosphate buffer saline (PBS, pH 7.4, 140 mmol/L NaCl, 2.7 mmol/L KCl, 10 mmol/L Na2HPO4, and 1.8 mmol/L KH2PO4) (Thakur et al., 2016). Each sample contained midguts from five individual larvae, and a total of three biological replicates were performed for each treatment (control and antibiotics treatment).
DNA Extraction and Polymerase Chain Reactions (PCR)
Bacterial genomic DNA was extracted from the midgut contents by using the classical Phenol/Chloroform/Proteinase K method (Bargues et al., 2007). The profiles of gut microbiota were detected using universal primers (forward: 5′-AGAGTTTGATCCTGGCTCAG-3′, and reverse: 5′-AAGGAGGTGATCCAGCCGCA-3′) to amplify the V3–V4 region. The PCR reactions were carried out with 10 μl of 2 × Taq PCR mixture (Biodragon, Beijing, China), 0.4 μl each of specific forward and reverse primers (10 μM), and 10 ng of genomic DNA from control or antibiotics-treated samples. The cycling parameters were 94°C for 2 min, 94°C for 30 s, and followed by 40 cycles of 60°C for 30 s and 72°C for 2 min. The PCR products were analyzed by electrophoresis on 1% agarose.
Quantitative Real-Time PCR
Quantitative real-time PCR (qRT-PCR) reactions were performed in a total reaction volume of 25 μl, including 12.5 μl of 2 × Hieff® qPCR SYBR Green Master Mix (Low Rox Plus) (YEASEN, Shanghai, China) and 0.5 μl each of specific forward and reverse primers (10 μM). The cycling parameters were 95°C for 5 min, followed by 40 cycles of 95°C for 10 s and 60°C for 30 s. The GAPDH (glyceraldehyde-3-phosphate dehydrogenase, GenBank ID: 118271716) gene was used as a reference gene. The fold changes of genes were analyzed according to the method of 2–ΔΔCt (Livak and Schmittgen, 2001). All experiments were repeated at least three times and one representing result was shown; the primers were listed in additional information (Supplementary Table 1).
Gut Microbiota Analysis
The 16S rRNA PCR products (V3–V4 region) obtained previously from gut microbiota were sequenced by the Biomarker Technologies company (Beijing, China) using the Illumina nova-seq 6000 platform. The gut microbiota sequencing data were summarized in Supplementary Table 2. The clean reads were clustered to obtain operational taxonomic units (OTU) by the USEARCH software based on 97.0% similarity (Edgar, 2013). Based on the Silva database (Quast et al., 2013), the feature sequences were annotated by using the Naive Bayes classifier in taxonomy, and the community composition at different levels (from phylum to species) was counted in each sample. The Quantitative Insights Into Microbial Ecology (QIIME) software was used to generate the species abundance tables at different taxonomic levels (Caporaso et al., 2010), and the R language tool was used to draw the community structure map for each sample at the taxonomic level (Charlop-Powers and Brady, 2015).
RNA Sequencing Analysis
Each sample contained midguts from five S. frugiperda individuals at L6D3; each group contained three biological replicates. Total RNAs were extracted using RNAiso plus reagent (TaKaRa, Dalian, China). About 5 μg of total RNA from each sample was prepared for library construction. RNA concentration and purity were measured using NanoDrop 2000 (Thermo Fisher Scientific, Wilmington, DE, United States). RNA integrity was assessed using the RNA Nano 6000 Assay Kit of the Agilent Bioanalyzer 2100 system (Agilent Technologies, CA, United States). The VAHTS Universal V6 RNA-seq Library Prep Kit for Illumina® (NR604-02, Vazyme, Nanjing, China) was used for library construction. The mRNA was enriched using the VAHTS mRNA Capture Beads (N401, Vazyme, Nanjing, China) and high-throughput sequencing was performed using Illumina nova-seq 6000 platform. The clean reads of each sample were aligned to the reference S. frugiperda genome (NCBI: ZJU_Sfru_1.0) by HISAT2 software (Kim et al., 2015). The aligned reads were assembled by StringTie software (Pertea et al., 2015).
The raw reads were saved in FASTQ format, and the raw sequencing data have been deposited in the NCBI Gene Expression Omnibus database under accession number: GSE1754761. The quality assessment was listed in the Supplementary Tables 3, 4. The Fragments Per Kilobase of transcript per Million fragments mapped (FPKM) values represent the expression levels of transcripts (Chen et al., 2020). Analysis of differentially expressed transcripts (DETs) was performed by using FPKM value of the log2(fold changes) >1 and the standard of false discovery rate (FDR) <0.001. To study the function of DETs, DETs were further classified according to Gene Ontology (GO) annotation by GOseq R packages based on Wallenius non-central hyper-geometric distribution under the standard FDR < 0.05 (Young et al., 2010). Functions of DETs pathways were determined though the Kyoto Encyclopedia of Genes and Genomes (KEGG) database, which is under the hypergeometric test and the p-value < 0.05 as the standard (Kanehisa et al., 2008). Gene function was also annotated based on the following databases: Nr (NCBI non-redundant protein sequences), Nt (NCBI non-redundant nucleotide sequences), Pfam (Protein family), KOG/COG (Clusters of Orthologous Groups of proteins), Swiss-Prot (A manually annotated and reviewed protein sequence database).
Statistical Analysis
The gene expression data were analyzed by using the GraphPad Prism 5 software (GraphPad Software Inc., San Diego, CA, United States), and the significant difference was determined by the control one-way ANOVA analysis of variances and Tukey’s Multiple Comparison Test. The values are mean ± SEM (standard error of mean) (n = 3), and p-value < 0.05 indicates significant difference. The 16S rRNA sequencing and S. frugiperda RNA sequencing analysis have been described in the corresponding subheadings.
Results
Effects of Antibiotics Exposure on the S. frugiperda Gut Bacterial Community
To investigate the effect of antibiotics exposure on the gut microbial community, we reared newly hatched larvae on the artificial diet containing antibiotics mixtures (the concentration of each antibiotic was 500 μg/ml). The midguts of S. frugiperda at L6D3 fed on artificial diet with or without antibiotics at L6D3 were collected and analyzed (Figure 1A). A total of 390,639 high-quality reads were obtained through performing 16S rRNA pyrosequencing based on the V3–V4 region by Illumina nova-seq 6000 platform; the microbiota data were summarized in different groups (Figure 1 and Supplementary Table 2).
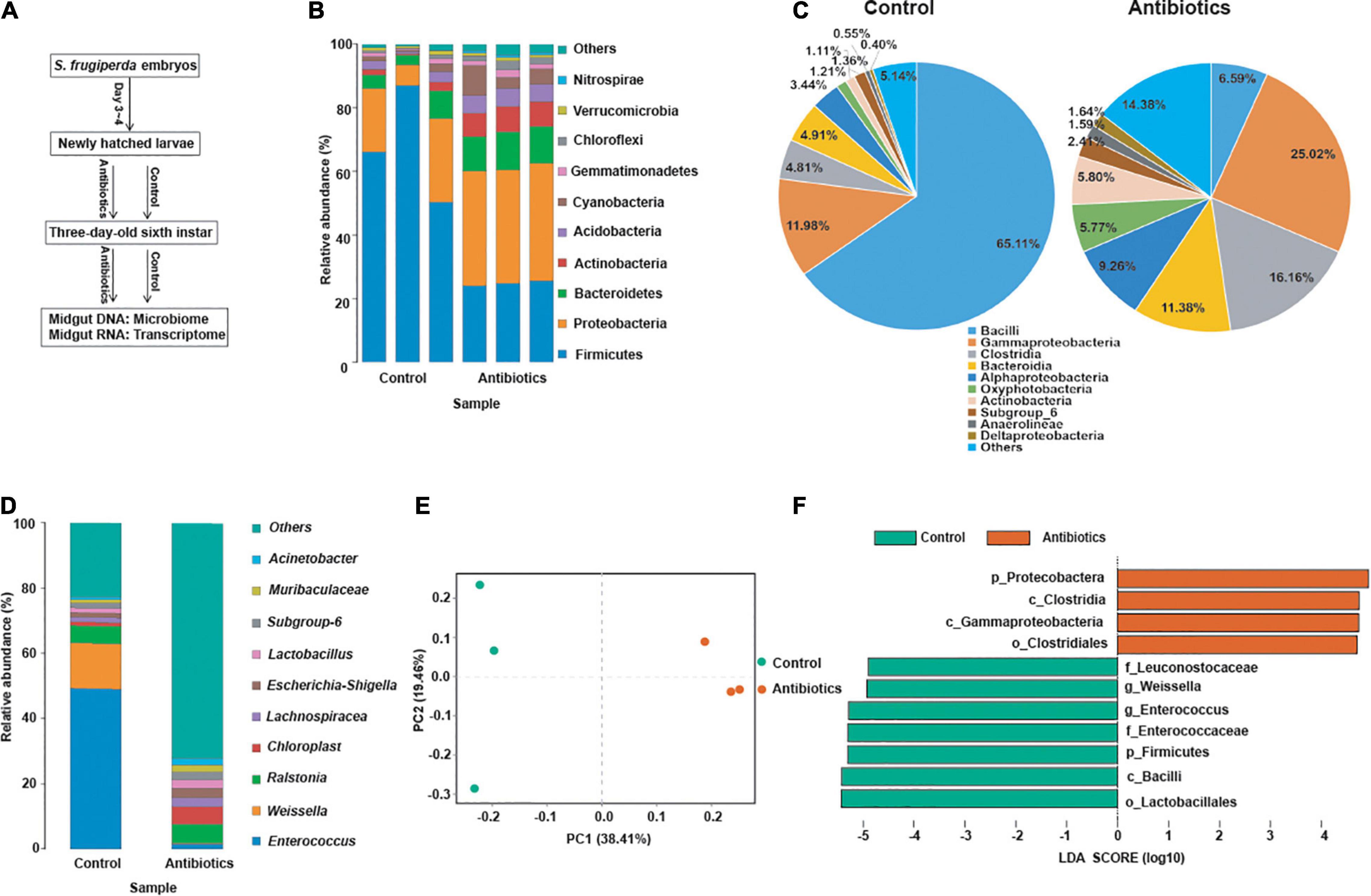
Figure 1. Effects of antibiotics exposure on gut microbiota structure and diversity in the S. frugiperda larvae. (A) A schematic diagram of the assay. (B–D) The relative abundance of gut microbiota at the phylum (B), class (C), and genus (D) levels, respectively, from the control and the antibiotics groups. (E) Beta-diversity was displayed by principal coordinates analysis (PCoA) based on the Bray–Curtis method in control and antibiotics groups. (F) Differentially abundant bacteria between the control and antibiotics groups were analyzed by LEFSe analysis with different haze levels. Linear discriminant analysis (LDA) scores indicate the degree of consistent difference in relative abundance between the control and antibiotics groups, log LDA score of >4.5 was used for the analysis.
As shown in Figure 1, at the phylum level, Firmicutes, Proteobacteria, and Bacteroidetes were the most abundant phyla in both the control and antibiotics-treated groups, but the abundance of Firmicutes was significantly decreased in the antibiotics group compared with the control group (Figure 1B). At the class level, there was a significant decrease in the abundance of Bacilli, which was 65.11% in the control group and reduced to 6.59% in the antibiotics-treated group (Figure 1C). Moreover, at the genus level, the abundance of Enterococcus was dramatically reduced from 49.35% in the control group to 1.66% in the antibiotics-treated group, and the abundance of Weissella was also reduced from 13.85 to 0.11% after antibiotics exposure (Figure 1D). According to principal coordinates analysis (PCoA) based on the Bray–Curtis method, the result suggested that the gut microbiota of the antibiotics group deviated from that of the control group (Figure 1E). In addition, the linear discriminant analysis effect size (LEFSe) analysis was performed, and the results showed that Firmicutes (phylum), Bacilli (class), Lactobacillales (order), Enterococcaceae and Leuconostocaceae (family), and Weissella and Enterococcus (genera) were rich in the control group (Figure 1E). While Firmicutes (phylum) was not detected in the antibiotics group, Proteobacteria (phylum), Clostridia and Gammaproteobacteria (class), and Clostridiales (order) were rich after antibiotics exposure (Figure 1F). These results indicated that gut bacterial community was different after antibiotics exposure compared to the control group.
RNA-Sequencing and Statistics of Gene Expression
To determine the effect of microbiota on gene expression in S. frugiperda gut, RNA samples of the midguts from control and antibiotics groups (n = 3) were collected and sequenced. In total, more than 4.4 million raw reads were obtained from every library with GC content and Q30 at 45 and 94%, respectively (Supplementary Table 3). Among these clean reads, 74.37–78.31% from each transcriptome were mapped to the S. frugiperda genome (Supplementary Table 4).
Based on the FPKM, principal components analysis (PCA) of transcriptome data between the control and antibiotics groups were performed, and the results indicated that PC1 principal component contributes 69.5% to the two-dimensional principal component axis; the control and antibiotics groups were significantly divided into two groups on the main component axis of PC1 (Figure 2A). To analyze the DETs between the control and antibiotics groups, the Venn figure suggested that 1,394 transcripts were differentially expressed between the two groups, 420 DETs were expressed only in the antibiotics group, 973 DETs were expressed only in the control group, and 7,266 transcripts were co-expressed in both groups (Figure 2B). Among these DETs, 432 transcripts were upregulated and 962 transcripts were downregulated in the antibiotics group vs. control group (Figure 2C). To analyze the expression pattern of DETs between the control and antibiotics groups, we drew the heatmap according to the FPKM of gene expression (Figure 2D), and the results showed that the expression levels of transcripts were significantly different in the antibiotics group compared with the control group.
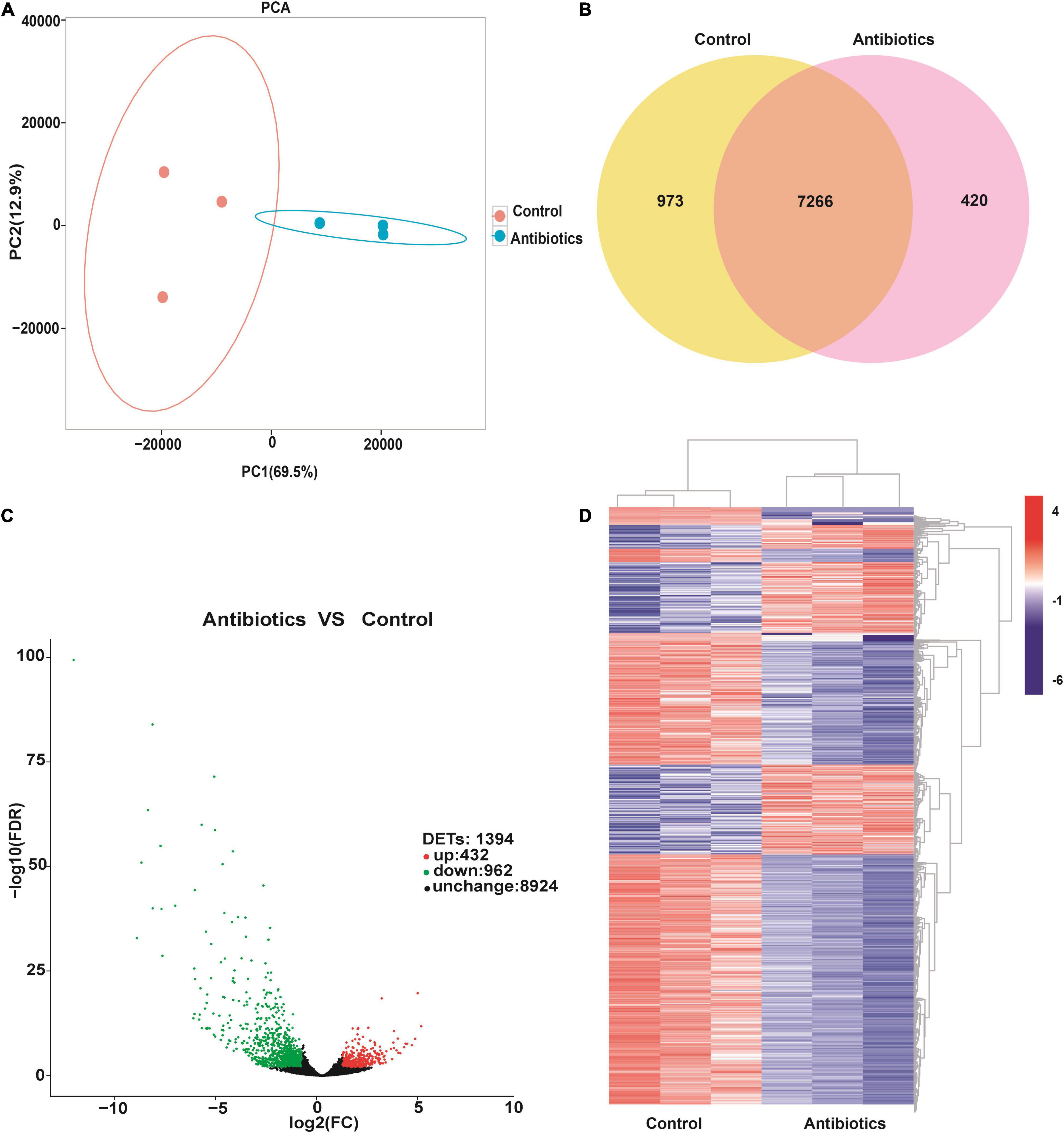
Figure 2. Differentially expressed transcripts in the gut of S. frugiperda at L6D3 between the control and antibiotics groups. (A) Principal component analysis (PCA) of differentially expressed transcripts based on the FPKM values of transcripts between the control and antibiotics exposure groups. (B) Venn diagram indicating the numbers of transcripts in the gut of S. frugiperda between the control and antibiotics exposure groups. (C) Volcano plot of differentially expressed transcripts in the gut of S. frugiperda between the control and antibiotics exposure groups. The red dots show the upregulated DETs, the green dots show the downregulated DETs, and the black dots show the unchanged DETs. (D) Hierarchical cluster heatmap showing the expression patterns of differentially expressed transcripts between the control and antibiotics group among every samples.
Gene Ontology Analysis of Differentially Expressed Transcripts Between the Control and Antibiotics Groups
To further analyze the function of DETs between the control and antibiotics groups, GO analysis was performed by the GOseq R packages based on Wallenius non-central hyper-geometric distribution (Young et al., 2010). Between the antibiotics-treated and the control groups, a total of 1,394 DETs were divided into three classes: biological processes, cellular component, and molecular function (Figure 3), revealing that a large number of DETs were enriched in the GO terms including signaling, cellular component organization or biogenesis, response to stimulus, biological regulation, single-organism process, metabolic process, cellular process of biological process, membrane, membrane part, cell, cell part, organelle of cellular component, binding, catalytic activity, transporter activity, molecular transducer activity, signal transducer activity, and nucleic acid binding transcription factor activity of molecular function, and the downregulated genes were more than upregulated DETs (Figure 3). A small number of upregulated DETs were enriched in the GO term including the detoxification, supramolecular complex, extracellular region, electron carrier activity, metallochaperone activity, structural molecule activity, and antioxidant activity. Therefore, GO classification analysis suggested that bio-regulatory process and metabolic levels may be active in the midgut of S. frugiperda after antibiotics exposure.
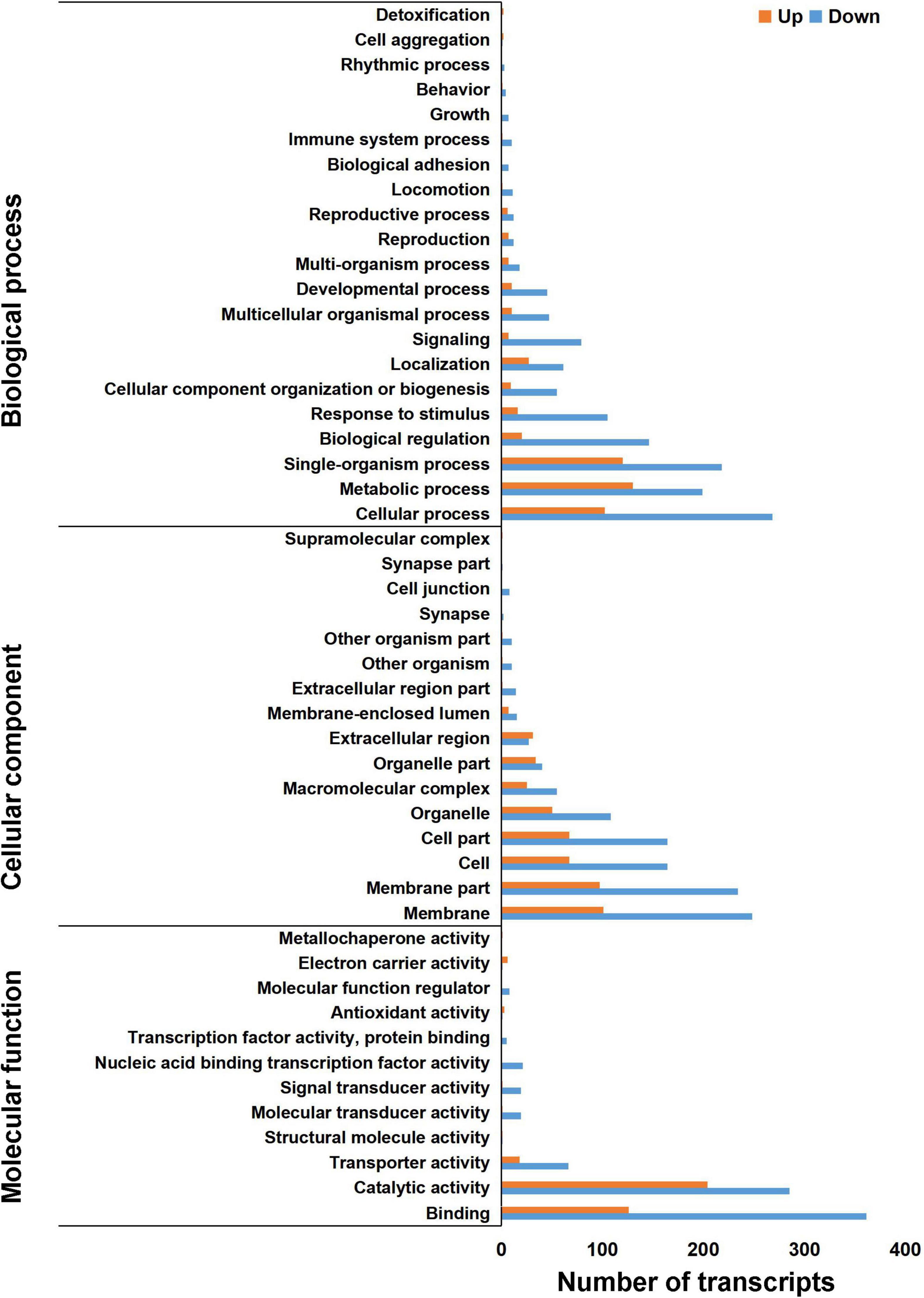
Figure 3. Gene Ontology annotation of differentially expressed transcripts in the gut of S. frugiperda at L6D3 between the antibiotics-treated and control groups at biological process, cellular component, and molecular function levels.
Kyoto Encyclopedia of Genes and Genomes Pathway Analysis of Differentially Expressed Transcripts Between the Control and Antibiotics Groups
To study the signal pathway enrichment of DETs after antibiotics exposure, KEGG analysis was performed with KOBAS software according to the signal pathway data online2 (Mao et al., 2005; Kanehisa et al., 2008). The results revealed that differentially upregulated transcripts were enriched in oxidative phosphorylation, metabolism of xenobiotics by cytochrome P450, glycerolipid metabolism, drug metabolism-cytochrome P450, protein processing in endoplasmic reticulum, valine, leucine and isoleucine degradation and glutathione metabolism pathways (Figure 4A), implying that the energy metabolism was active after antibiotics exposure in S. frugiperda midgut. The differentially downregulated transcripts were mainly enriched in the autophagy, ubiquitin-mediated proteolysis, glycosaminoglycan degradation, and lysosome pathways (Figure 4B).
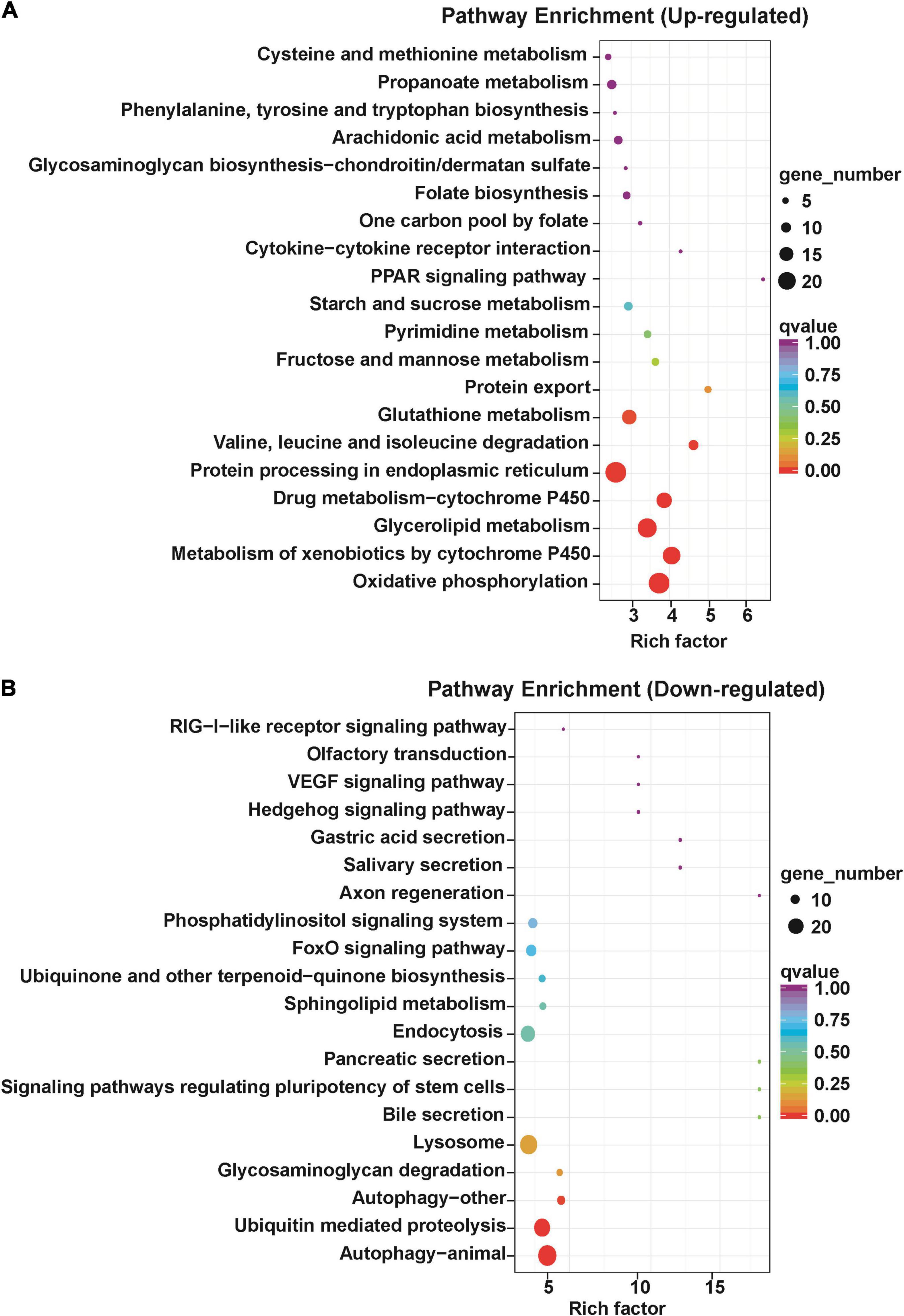
Figure 4. Kyoto Encyclopedia of Genes and Genomes annotation of differentially expressed transcripts in the gut of S. frugiperda at L6D3 after feeding antibiotics mixtures. (A) Top 20 of upregulated pathway enrichment. (B) Top 20 of downregulated pathway enrichment.
In particular, a large number of DETs related to autophagy were downregulated in the antibiotics group (Figure 5 and Supplementary Table 5). As is known, autophagy is a very important and conserved self-digestion pathway in maintaining cell homeostasis and function, and can eventually induce programmed cell death (He et al., 2017; Thorburn, 2018). In this study, autophagy-related genes were downregulated in the antibiotics-treated group; this implies that decreased autophagy may contribute to the disorder of gut microbiota to maintain intestinal balance.
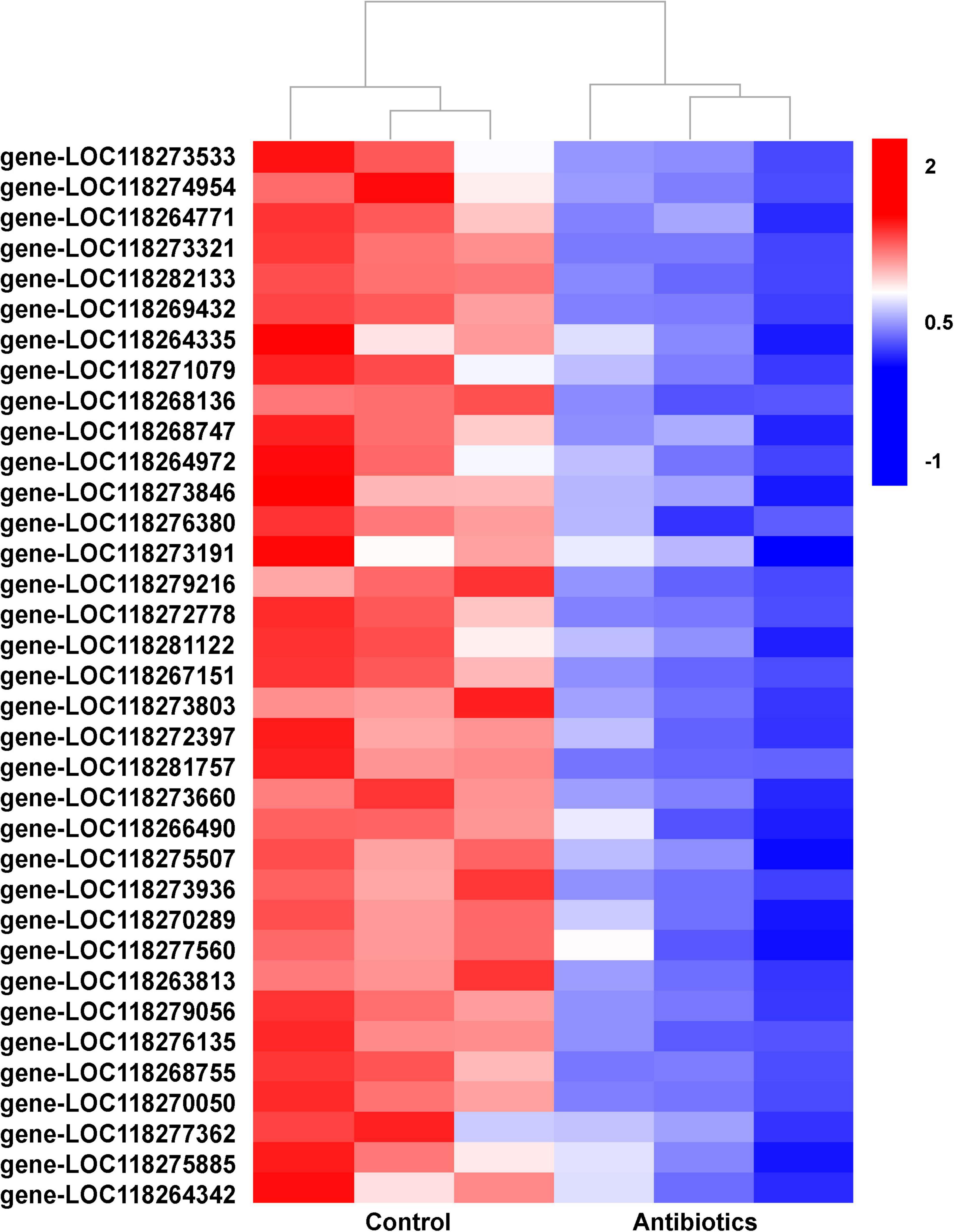
Figure 5. Hierarchical cluster heatmap showing the expression patterns of differentially expressed transcripts related to autophagy between the control and antibiotics groups among every samples. The values of log2 (fold change) were for the antibiotics group vs. control group and the description of differentially expressed transcripts were listed (Supplementary Table 5).
Expression of Selected Differentially Expressed Transcripts by Quantitative Real-Time PCR
To validate the results of RNA-seq data, we selected 12 DETs for qRT-PCR. Of these selected DETs, six were upregulated genes, and the other six were downregulated genes in the midguts after antibiotics treatment (Table 1). These DETs were related to metabolism, oxidative phosphorylation and detoxication, and immunity reactions, which can be activated by the stimulus of the environment in insects. Cytochrome c oxidase subunit 6A (gene-LOC118269158) is involved in energy production and conversion; glutathione S-transferase 2 (gene-LOC118271637), lipase member H, pancreatic lipase-related protein (gene-LOC118273886), and alcohol dehydrogenase (gene-LOC118272357) are the main enzymes involved in amino acid and lipid metabolism; and UDP-glucuronosyltransferase 2C1 (gene-LOC118277905) is involved in carbohydrate transport and metabolism. All the above genes were significantly upregulated in the midgut of antibiotics group compared to the control group (Table 1 and Figure 6).
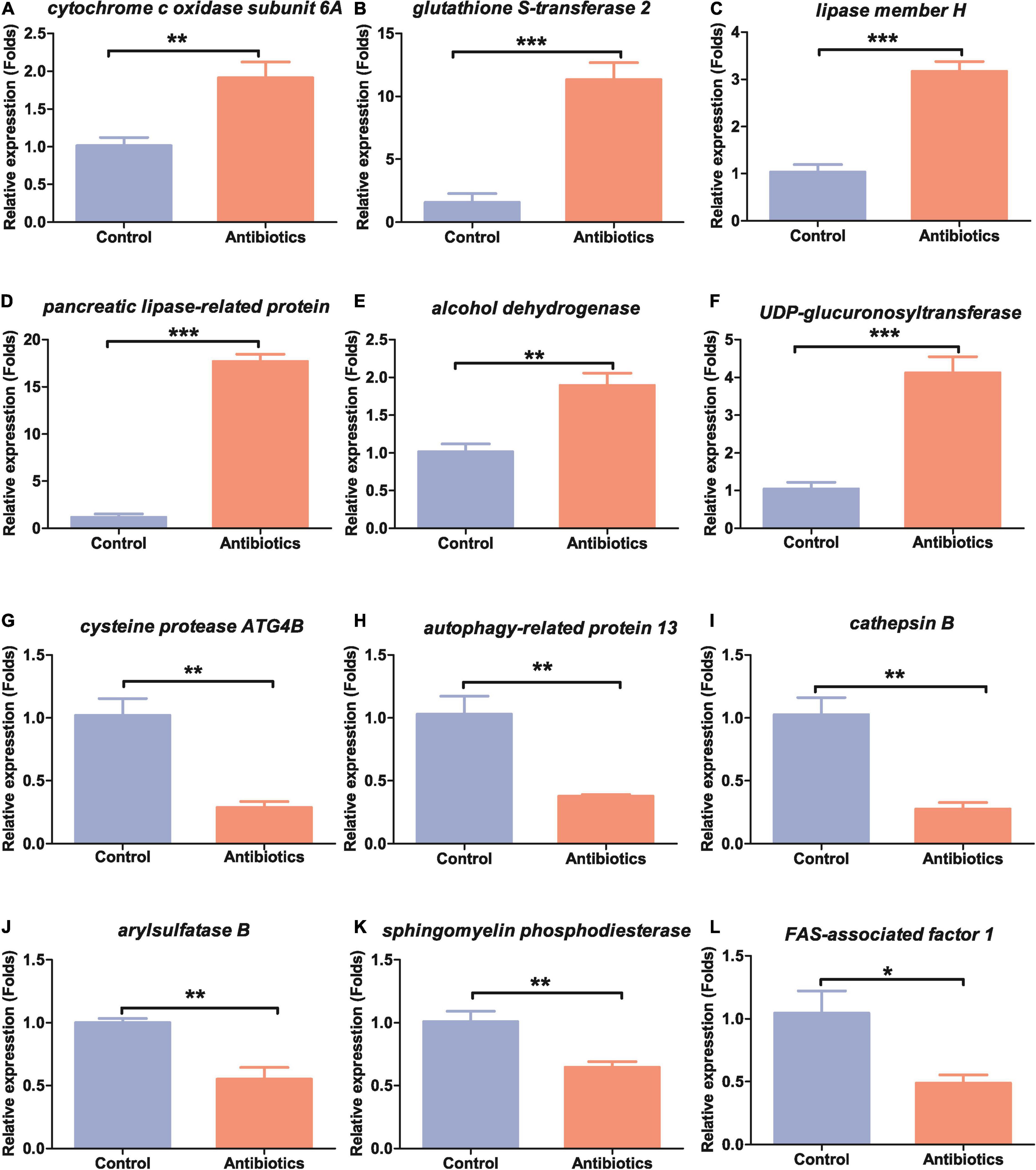
Figure 6. Expression patterns of the selected 12 differentially expressed genes in the guts of S. frugiperda between the control and antibiotics exposure groups. (A) cytochrome c oxidase subunit 6A, gene-LOC118269158; (B) glutathione S-transferase 2, gene-LOC118271637; (C) lipase member H, gene-LOC118273368; (D) pancreatic lipase-related protein, gene-LOC118273886; (E) alcohol dehydrogenase, gene-LOC118272357; (F) UDP-glucuronosyltransferase 2C1, gene-LOC118277905; (G) cysteine protease ATG4B, gene-LOC11827332; (H) autophagy-related protein 13, gene-LOC118279216; (J) arylsulfatase B, gene-LOC118268623; (I) cathepsin B, gene-LOC118264342; (K) sphingomyelin phosphodiesterase, gene-LOC118270137; (L) FAS-associated factor 1, gene-LOC118265774. *p < 0.05, **p < 0.01, and ***p < 0.001.
Many genes were also shown to be downregulated in qRT-PCR experiments as in RNA-seq data. Cysteine protease ATG4B (gene-LOC118273321), autophagy-related protein 13 (gene-LOC118279216), and cathepsin B (gene-LOC118264342) were significantly downregulated (Table 1 and Figures 6G–I). Arylsulfatase B (gene-LOC118268623), a gene related to inorganic ion transport and metabolism, and sphingomyelin phosphodiesterase (gene-LOC118270137), a gene related to lipid transport and metabolism, were downregulated (Table 1 and Figures 6J,K). Genes related to immunity such as FAS-associated factor 1 (gene-LOC118265774) were also downregulated (Table 1 and Figure 6L). These results suggested that S. frugiperda gut homeostasis was significantly affected by antibiotics exposure.
Discussion
In this study, we exposed the newly hatched S. frugiperda larvae to antibiotics cocktails consisting of penicillin, gentamicin, rifampicin, and streptomycin. We firstly identified the gut bacterial community of S. frugiperda between the control group and antibiotics group. We found that the abundance of gut microbiota from phylum to genera level was decreased in the antibiotics group compared with the control group (Figure 1). Among the top 10 phyla, Firmicutes, Proteobacteria, Bacteroidetes, Actinobacteria, Acidobacteria, Chloroflexi, and Nitrospirae were significantly different in S. frugiperda midgut after antibiotics exposure, in which the dominant Firmicutes was decreased significantly and Actinobacteria was increased. Of the top genera, Enterococcus and Weissella were significantly decreased in relative abundance (Figure 1D). Therefore, antibiotics exposure affected the composition and distribution of intestinal microorganisms of S. frugiperda. Our results were consistent with previous studies and suggest that antibiotics could alter the microbial community in insects in lepidopteran insects (Broderick et al., 2006; Thakur et al., 2016; Raymann et al., 2017; Chen et al., 2018; Martínez-Solís et al., 2020).
The Enterococcus genus is known to be abundant in the intestinal tracts of various animals, possess the capacity to acquire and disseminate antimicrobial resistant determinants and the ability to produce various virulence genes that enables it to establish infections (Iweriebor et al., 2016). In many lepidopteran species, Enterococcus was one of most abundant genera (Paniagua Voirol et al., 2018). The Enterococcus genus was reduced in midgut of gypsy moth reared on antibiotic mixtures (penicillin, gentamicin, rifampicin, and streptomycin), which was necessary for B. thuringiensis-induced larval mortality (Broderick et al., 2006). In Bombyx mori, inhibition of Enterococcus by fluoride can increase amino acid synthesis, reduce cell carbohydrate transport and energy production (Li G. et al., 2020). Our data suggested that the abundance of Enterococcus was significantly reduced in the antibiotics-treated group, and the transcriptome data suggested that genes related to carbohydrate transport and metabolism and energy production were also upregulated, implying that the Enterococcus genus may affect the metabolism level in the gut of S. frugiperda. Weissella is a genus of lactic acid bacteria and is often found in insects (de Jonge et al., 2020; Tan et al., 2021). In Locusta migratoria, Weissella genus was the most common bacterium in the midguts and hindguts, and it would decrease after infection with the pathogens (Tan et al., 2021). It has been reported that Weissella cibaria strain TM128 can decrease fungal infection levels by 50% (Trias et al., 2008). In our study, the abundance of Weissella was also decreased in the gut of S. frugiperda after antibiotics treatment, implying that the resistance to pathogen infection may be decreased after antibiotics treatment.
It has been demonstrated that the gut microbiota can regulate many biological processes by influencing the related gene expression in insects (Broderick et al., 2014; Zhu et al., 2020). Therefore, we performed transcriptomic analysis by RNA-seq to further identify the genes involved in biological processes influenced by intestinal microbial population after antibiotics treatment. We found that 962 DETs were downregulated and 432 were upregulated between the control and antibiotics group (Figure 2B). GO enrichment analysis and KEGG signal pathway analysis also revealed that many metabolism processes were regulated by the DETs (Figures 4, 5). In particular, we found that cytochrome c oxidase subunit 6A (gene-LOC118269158) and UDP-glucuronosyltransferase 2C1 (gene-LOC118277905) were significantly upregulated. Our results were consistent with the results in S. litura and Chinese bumblebee that gut microbiota affected the energy and carbohydrate metabolism after antibiotics exposure (Thakur et al., 2016; Zhang et al., 2021). Meanwhile, the triacylglycerol lipase gene and glutathione S-transferase (GST) were upregulated (Figure 6). It was speculated that gut microbiota regulated the process of hydrolyzing fats into fatty acids and glycerols by lipase. GSTs are the major enzymes in detoxification of endogenous and/or xenobiotic compounds and may also affect metabolic and signaling pathways (Ketterman et al., 2011). It was reported that S. frugiperda may be active in detoxifying metabolism and xenobiotic metabolism under the stimuli such as insecticides and starvation. Insects could reallocate resources when needed for survival; this makes it very difficult for pest control (Poivet et al., 2021; Shu et al., 2021; Wang et al., 2021). Lipases and GSTs were significantly increased in the gut of S. litura after the antibiotic (streptomycin sulfate) treatment, implying that they might play key roles in improving the efficiency of conversion of ingested food to insect biomass and further resulted in faster development, which is responsible for antibiotics tolerance (Thakur et al., 2016; Lu et al., 2021). Overall, our results were consistent with the previous studies that biological processes can be influenced by intestinal microbial population through transcriptional regulation.
It is noteworthy that genes related to the autophagy pathways were downregulated in the gut of S. frugiperda after antibiotics treatment according to KEGG analysis (Figures 4B, 5). In particular, the expression of cathepsin B (gene-LOC118264342) and autophagy-related protein 13 homolog (gene-LOC118279216) was significantly downregulated in the antibiotics-exposed gut of S. frugiperda (Figure 6). It has been suggested that cathepsin B performs its function in protein turnover as an initiator protease during lysosome-mediated autophagy/apoptosis (Bhoopathi et al., 2010; Yang et al., 2017). Moreover, it has been reported that autophagy is indispensable for intestinal homeostasis maintenance, gut ecology regulation, gut immune response, and anti-microbial protection (Larabi et al., 2020). In the autophagy-related 5 (Atg5)-deficient mice model, the diversity of gut microbiota was significantly decreased (Yang et al., 2018). In our results, the abundance and diversity of dominant gut microbiota were also decreased in the gut of S. frugiperda after antibiotics exposure (Figure 1). Therefore, it could be speculated that intestinal autophagy of S. frugiperda might be suppressed with the decrease of dominant gut microbiota after antibiotics treatment; this process may play important roles in maintaining a balance of intestinal homeostasis and function during S. frugiperda development. The ubiquitin-mediated proteolysis pathway was downregulated in the antibiotics group compared to the control (Figure 4B). Posttranslational modification mediated by ubiquitin conjugation plays an important role in inflammation, which involved many biochemical reactions through ubiquitin systems (Hershko and Ciechanover, 1998). Some studies suggested that ubiquitin E3 ligase was associated with the gut microbiota in the inflammatory bowel diseases (Kathania et al., 2020) and immune response (Ebner et al., 2017). Therefore, ubiquitin-mediated proteolysis pathway may interact with intestinal bacteria in S. frugiperda gut, which could further influence the immune system, as the immune deficiency-like protein was downregulated in the antibiotics group (Figure 6). The glycosaminoglycan-degrading enzyme arylsulfatase B, a gene related to lysosomal storage disorders (Pohl et al., 2018), was also downregulated in our study. Sphingomyelin phosphodiesterase (SMPD) is a hydrolase that performs the function in metabolic reactions related to sphingomyelin (Han et al., 2018), which was downregulated in the antibiotics-exposed gut (Figure 6). These results may imply that it is necessary for S. frugiperda to maintain the intestinal homeostasis and metabolism to adapt to artificial diet with antibiotics mixture.
Conclusion
Our study revealed that antibiotics mixture exposure significantly affected the gut microbiota and induced dysbiosis in S. frugiperda. As per our transcriptomic analysis, we further elucidate the expression of the large number of genes related to metabolism and intestinal homeostasis involved in sugar, lipid, protein metabolism, autophagy, and immunity after antibiotics exposure in the gut of S. frugiperda. Taken together, our findings provide fundamental information for pest control by using antibiotics to affect gut microbiota.
Data Availability Statement
The datasets presented in this study can be found in online repositories. The names of the repository/repositories and accession number(s) can be found below: https://www.ncbi.nlm.nih.gov/, GSE175476.
Author Contributions
YC and XYW designed the experiment and revised the manuscript. YC and HZ carried out the experiment and the bioinformatics analysis. YL and QC reared S. frugiperda larvae, collected and prepared RNA samples for RNA-seq, and performed some RT-PCR. X-QY provided suggestions for our study and revised the manuscript. All authors read and approved the final manuscript.
Funding
This work was supported by the National Natural Science Foundation of China (32070615 and 81902093), Guangdong Provincial Natural Science Foundation (2021A1515010823), Guangdong Provincial Science and Technology Agricultural Program (KTP20200105), Guangzhou Science and Technology Project (202002030100), China Postdoctoral Science Foundation (2021M691094), and Guangdong Province Universities and Colleges Pearl River Scholar Funded Scheme to XYW.
Conflict of Interest
The authors declare that the research was conducted in the absence of any commercial or financial relationships that could be construed as a potential conflict of interest.
Publisher’s Note
All claims expressed in this article are solely those of the authors and do not necessarily represent those of their affiliated organizations, or those of the publisher, the editors and the reviewers. Any product that may be evaluated in this article, or claim that may be made by its manufacturer, is not guaranteed or endorsed by the publisher.
Supplementary Material
The Supplementary Material for this article can be found online at: https://www.frontiersin.org/articles/10.3389/fmicb.2021.727434/full#supplementary-material
Footnotes
References
Acevedo, F. E., Peiffer, M., Tan, C. W., Stanley, B. A., Stanley, A., Wang, J., et al. (2017). Fall armyworm-associated gut bacteria modulate plant defense responses. Mol. Plant Microbe Interact. 30, 127–137. doi: 10.1094/mpmi-11-16-0240-R
Bargues, M. D., Artigas, P., Mera, Y., Sierra, R. L., Pointier, J. P., and Mas-Coma, S. (2007). Characterisation of Lymnaea cubensis, L-viatrix and L-neotropica n. sp., the main vectors of Fasciola hepatica in Latin America, by analysis of their ribosomal and mitochondrial DNA. Ann. Trop. Med. Parasitol. 101, 621–641. doi: 10.1179/136485907x229077
Bhoopathi, P., Chetty, C., Gujrati, M., Dinh, D. H., Rao, J. S., and Lakka, S. (2010). Cathepsin B facilitates autophagy-mediated apoptosis in SPARC overexpressed primitive neuroectodermal tumor cells. Cell Death Differ. 17, 1529–1539. doi: 10.1038/cdd.2010.28
Broderick, N. A., Buchon, N., and Lemaitre, B. (2014). Microbiota-induced changes in Drosophila melanogaster host gene expression and gut morphology. mBio 5, e01117–14. doi: 10.1128/mBio.01117-14
Broderick, N. A., Raffa, K. F., and Handelsman, J. (2006). Midgut bacteria required for Bacillus thuringiensis insecticidal activity. Proc. Natl. Acad. Sci. U.S.A. 103, 15196–15199. doi: 10.1073/pnas.0604865103
Caporaso, J. G., Kuczynski, J., Stombaugh, J., Bittinger, K., Bushman, F. D., Costello, E. K., et al. (2010). QIIME allows analysis of high-throughput community sequencing data. Nat. Methods 7, 335–336. doi: 10.1038/nmeth.f.303
Charlop-Powers, Z., and Brady, S. F. (2015). phylogeo: an R package for geographic analysis and visualization of microbiome data. Bioinformatics 31, 2909–2911. doi: 10.1093/bioinformatics/btv269
Chen, B., Du, K., Sun, C., Vimalanathan, A., Liang, X., Li, Y., et al. (2018). Gut bacterial and fungal communities of the domesticated silkworm (Bombyx mori) and wild mulberry-feeding relatives. ISME J. 12, 2252–2262. doi: 10.1038/s41396-018-0174-1
Chen, Y., Ou, J., Liu, Y., Wu, Q., Wen, L., Zheng, S., et al. (2020). Transcriptomic analysis of the testicular fusion in Spodoptera litura. BMC Genomics 21:171. doi: 10.1186/s12864-020-6494-3
de Jonge, N., Michaelsen, T. Y., Ejbye-Ernst, R., Jensen, A., Nielsen, M. E., Bahrndorff, S., et al. (2020). Housefly (Musca domestica L.) associated microbiota across different life stages. Sci. Rep. 10:7842. doi: 10.1038/s41598-020-64704-y
Ebner, P., Versteeg, G. A., and Ikeda, F. (2017). Ubiquitin enzymes in the regulation of immune responses. Crit. Rev. Biochem. Mol. Biol. 52, 425–460. doi: 10.1080/10409238.2017.1325829
Edgar, R. C. (2013). UPARSE: highly accurate OTU sequences from microbial amplicon reads. Nat. Methods 10, 996–998. doi: 10.1038/nmeth.2604
Engel, P., Martinson, V. G., and Moran, N. A. (2012). Functional diversity within the simple gut microbiota of the honey bee. Proc. Natl. Acad. Sci. U.S.A. 109, 11002–11007. doi: 10.1073/pnas.1202970109
Engel, P., and Moran, N. A. (2013). The gut microbiota of insects-diversity in structure and function. FEMS Microbiol. Rev. 37, 699–735. doi: 10.1111/1574-6976.12025
Goergen, G., Kumar, P. L., Sankung, S. B., Togola, A., and Tamo, M. (2016). First report of outbreaks of the fall armyworm Spodoptera frugiperda (J E Smith) (Lepidoptera: Noctuidae), a new alien invasive pest in west and central Africa. PLoS One 11:e0165632. doi: 10.1371/journal.pone.0165632
Han, L., Ding, G., Liu, Y., Huang, J., and Wu, J. (2018). Characterization of sphingomyelin phosphodiesterase expression in Bumblebee (Bombus lantschouensis). J. Insect Sci. 18:20. doi: 10.1093/jisesa/iey106
He, Z., Guo, L., Shu, Y., Fang, Q., Zhou, H., Liu, Y., et al. (2017). Autophagy protects auditory hair cells against neomycin-induced damage. Autophagy 13, 1884–1904. doi: 10.1080/15548627.2017.1359449
Hershko, A., and Ciechanover, A. (1998). The ubiquitin system. Annu. Rev. Biochem. 67, 425–479. doi: 10.1146/ANNUREV.BIOCHEM.67.1.425
Iweriebor, B. C., Obi, L. C., and Okoh, A. I. (2016). Macrolide, glycopeptide resistance and virulence genes in Enterococcus species isolates from dairy cattle. J. Med. Microbiol. 65, 641–648. doi: 10.1099/jmm.0.000275
Jang, S., and Kikuchi, Y. (2020). Impact of the insect gut microbiota on ecology, evolution, and industry. Curr. Opin. Insect Sci. 41, 33–39. doi: 10.1016/j.cois.2020.06.004
Jia, B., Liu, Y., Zhu, Y. C., Liu, X., Gao, C., and Shen, J. (2009). Inheritance, fitness cost and mechanism of resistance to tebufenozide in Spodoptera exigua (Hubner) (Lepidoptera: Noctuidae). Pest Manage Sci. 65, 996–1002. doi: 10.1002/ps.1785
Kanehisa, M., Araki, M., Goto, S., Hattori, M., Hirakawa, M., Itoh, M., et al. (2008). KEGG for linking genomes to life and the environment. Nucleic Acids Res. 36, D480–D484. doi: 10.1093/nar/gkm882
Kathania, M., Tsakem, E. L., Theiss, A. L., and Venuprasad, K. (2020). Gut microbiota contributes to spontaneous colitis in E3 ligase itch-deficient mice. J. Immunol. 204, 2277–2284. doi: 10.4049/jimmunol.1701478
Ketterman, A. J., Saisawang, C., and Wongsantichon, J. (2011). Insect glutathione transferases. Drug Metab. Rev. 43, 253–265. doi: 10.3109/03602532.2011.552911
Kim, D., Langmead, B., and Salzberg, S. L. (2015). HISAT: a fast spliced aligner with low memory requirements. Nat. Methods 12, 357–360. doi: 10.1038/nmeth.3317
Larabi, A., Barnich, N., and Nguyen, H. T. T. (2020). New insights into the interplay between autophagy, gut microbiota and inflammatory responses in IBD. Autophagy 16, 38–51. doi: 10.1080/15548627.2019.1635384
Li, G., Shi, M., Zhao, S., Li, D., Long, Y., Yang, C., et al. (2020). RNA-Seq comparative analysis reveals the response of Enterococcus faecalis TV4 under fluoride exposure. Gene 726:144197. doi: 10.1016/j.gene.2019.144197
Li, X., Wu, M., Ma, J., Gao, B., Wu, Q., Chen, A., et al. (2020). Prediction of migratory routes of the invasive fall armyworm in eastern China using a trajectory analytical approach. Pest Manage Sci. 76, 454–463. doi: 10.1002/ps.5530
Lin, X., Kang, Z., Pan, Q., and Liu, T. (2015). Evaluation of five antibiotics on larval gut bacterial diversity of Plutella xylostella (Lepidoptera: Plutellidae). Insect Sci. 22, 619–628. doi: 10.1111/1744-7917.12168
Livak, K. J., and Schmittgen, T. D. (2001). Analysis of relative gene expression data using real-time quantitative PCR and the 2(-Delta Delta C(T)) method. Methods 25, 402–408. doi: 10.1006/meth.2001.1262
Lu, K., Cheng, Y., Li, Y., Li, W., Zeng, R., and Song, Y. (2021). Phytochemical flavone confers broad-spectrum tolerance to insecticides in Spodoptera litura by activating ROS/CncC-mediated xenobiotic detoxification pathways. J. Agric. Food Chem. 69, 7429–7445. doi: 10.1021/acs.jafc.1c02695
Mao, X. Z., Cai, T., Olyarchuk, J. G., and Wei, L. P. (2005). Automated genome annotation and pathway identification using the KEGG Orthology (KO) as a controlled vocabulary. Bioinformatics 21, 3787–3793. doi: 10.1093/bioinformatics/bti430
Martínez-Solís, M., Collado, M. C., and Herrero, S. (2020). Influence of diet, sex, and viral infections on the gut microbiota composition of Spodoptera exigua caterpillars. Front. Microbiol. 11:753. doi: 10.3389/fmicb.2020.00753
Paramasiva, I., Sharma, H. C., and Krishnayya, P. V. (2014). Antibiotics influence the toxicity of the delta endotoxins of Bacillus thuringiensis towards the cotton bollworm, Helicoverpa armigera. BMC Microbiol. 14:200. doi: 10.1186/1471-2180-14-200
Pertea, M., Pertea, G. M., Antonescu, C. M., Chang, T. C., Mendell, J. T., and Salzberg, S. L. (2015). StringTie enables improved reconstruction of a transcriptome from RNA-seq reads. Nat. Biotechnol. 33, 290–295. doi: 10.1038/nbt.3122
Pohl, S., Angermann, A., Jeschke, A., Hendrickx, G., Yorgan, T. A., Makrypidi-Fraune, G., et al. (2018). The lysosomal protein arylsulfatase B is a key enzyme involved in skeletal turnover. J. Bone Miner. Res. 33, 2186–2201. doi: 10.1002/jbmr.3563
Poivet, E., Gallot, A., Montagné, N., Senin, P., Monsempès, C., Legeai, F., et al. (2021). Transcriptome profiling of starvation in the peripheral chemosensory organs of the crop pest Spodoptera littoralis caterpillars. Insects 12:573. doi: 10.3390/insects12070573
Powell, J. E., Martinson, V. G., Urban-Mead, K., and Moran, N. A. (2014). Routes of acquisition of the gut microbiota of the honey bee Apis mellifera. Appl. Environ. Microbiol. 80, 7378–7387. doi: 10.1128/aem.01861-14
Quast, C., Pruesse, E., Yilmaz, P., Gerken, J., Schweer, T., Yarza, P., et al. (2013). The SILVA ribosomal RNA gene database project: improved data processing and web-based tools. Nucleic Acids Res. 41, D590–D596. doi: 10.1093/nar/gks1219
Raymann, K., Shaffer, Z., and Moran, N. A. (2017). Antibiotic exposure perturbs the gut microbiota and elevates mortality in honeybees. PLoS Biol. 15:e2001861. doi: 10.1371/journal.pbio.2001861
Rozadilla, G., Cabrera, N. A., Virla, E. G., Greco, N. M., and Mccarthy, C. B. (2020). Gut microbiota of Spodoptera frugiperda (J E Smith) larvae as revealed by metatranscriptomic analysis. J. Appl. Entomol. 144, 351–363. doi: 10.1111/jen.12742
Schoeler, M., and Caesar, R. (2019). Dietary lipids, gut microbiota and lipid metabolism. Rev. Endocr. Metab. Disord. 20, 461–472. doi: 10.1007/s11154-019-09512-0
Shu, B., Yu, H., Li, Y., Zhong, H., Li, X., Cao, L., et al. (2021). Identification of azadirachtin responsive genes in Spodoptera frugiperda larvae based on RNA-seq. Pestic. Biochem. Physiol. 172:104745. doi: 10.1016/j.pestbp.2020.104745
Tan, S., Yin, Y., Cao, K., Zhao, X., Wang, X., Zhang, Y. X., et al. (2021). Effects of a combined infection with Paranosema locustae and Beauveria bassiana on Locusta migratoria and its gut microflora. Insect Sci. 28, 347–354. doi: 10.1111/1744-7917.12776
Thakur, A., Dhammi, P., Saini, H. S., and Kaur, S. (2016). Effect of antibiotic on survival and development of Spodoptera litura (Lepidoptera: Noctuidae) and its gut microbial diversity. Bull. Entomol. Res. 106, 387–394. doi: 10.1017/s0007485316000031
Thorburn, A. (2018). Autophagy and disease. J. Biol. Chem. 293, 5425–5430. doi: 10.1074/jbc.R117.810739
Trias, R., Baneras, L., Montesinos, E., and Badosa, E. (2008). Lactic acid bacteria from fresh fruit and vegetables as biocontrol agents of phytopathogenic bacteria and fungi. Int. Microbiol. 11, 231–236. doi: 10.2436/20.1501.01.66
Ugwu, J. A., Liu, M., Sun, H., and Asiegbu, F. O. (2020). Microbiome of the larvae of Spodoptera frugiperda (J E Smith) (Lepidoptera: Noctuidae) from maize plants. J. Appl. Entomol. 144, 764–776. doi: 10.1111/jen.12821
Paniagua Voirol, L. R., Frago, E., Kaltenpoth, M., Hilker, M., and Fatouros, N. E. (2018). Bacterial symbionts in lepidoptera: their diversity, transmission, and impact on the host. Front. Microbiol. 9:556. doi: 10.3389/fmicb.2018.00556
Wang, L., Yang, Q., Tang, R., Liu, X., Fan, Z., Li, J., et al. (2021). Gene expression differences between developmental stages of the fall armyworm (Spodoptera frugiperda). DNA Cell Biol. 40, 580–588. doi: 10.1089/dna.2020.6191
Wu, Q., He, L., Shen, X., Jiang, Y., Liu, J., Hu, G., et al. (2019). Estimation of the potential infestation area of newly-invaded fall armyworm Spodoptera frugiperda in the Yangtze river valley of China. Insects 10:298. doi: 10.3390/insects10090298
Yang, K., Bae, E., Ahn, S. G., Pang, K., Park, Y., Park, J., et al. (2017). Co-chaperone BAG2 determines the pro-oncogenic role of cathepsin B in triple-negative breast cancer cells. Cell Rep. 21, 2952–2964. doi: 10.1016/j.celrep.2017.11.026
Yang, L., Liu, C., Zhao, W., He, C., Ding, J., Dai, R., et al. (2018). Impaired autophagy in intestinal epithelial cells alters gut microbiota and host immune responses. Appl. Environ. Microbiol. 84:e00880. doi: 10.1128/aem.00880-18
Young, M. D., Wakefield, M. J., Smyth, G. K., and Oshlack, A. (2010). Gene ontology analysis for RNA-seq: accounting for selection bias. Genome Biol. 11:R14. doi: 10.1186/gb-2010-11-2-r14
Zhang, X., Li, T., Zhou, C., Zhao, D., Zhu, Y., Bing, X., et al. (2020). Antibiotic exposure perturbs the bacterial community in the small brown planthopper Laodelphax striatellus. Insect Sci. 27, 895–907. doi: 10.1111/1744-7917.12675
Zhang, Z., Huang, M., Qiu, L., Song, R., Zhang, Z., Ding, Y., et al. (2021). Diversity and functional analysis of Chinese bumblebee gut microbiota reveal the metabolic niche and antibiotic resistance variation of Gilliamella. Insect Sci. 28, 302–314. doi: 10.1111/1744-7917.12770
Zhou, F., Liu, B., Liu, X., Li, Y., Wang, L., Huang, J., et al. (2021). The impact of microbiome and microbiota-derived sodium butyrate on Drosophila transcriptome and metabolome revealed by multi-omics analysis. Metabolites 11:298. doi: 10.3390/metabo11050298
Keywords: gut microbiota, antibiotics, metabolic homeostasis, energy, autophagy, Spodoptera frugiperda
Citation: Chen Y, Zhou H, Lai Y, Chen Q, Yu X-Q and Wang X (2021) Gut Microbiota Dysbiosis Influences Metabolic Homeostasis in Spodoptera frugiperda. Front. Microbiol. 12:727434. doi: 10.3389/fmicb.2021.727434
Received: 22 June 2021; Accepted: 31 August 2021;
Published: 30 September 2021.
Edited by:
Amparo Latorre, University of Valencia, SpainReviewed by:
Beatriz Sabater-Munoz, Polytechnic University of Valencia, SpainFathiya Mbarak Khamis, International Centre of Insect Physiology and Ecology (ICIPE), Kenya
Copyright © 2021 Chen, Zhou, Lai, Chen, Yu and Wang. This is an open-access article distributed under the terms of the Creative Commons Attribution License (CC BY). The use, distribution or reproduction in other forums is permitted, provided the original author(s) and the copyright owner(s) are credited and that the original publication in this journal is cited, in accordance with accepted academic practice. No use, distribution or reproduction is permitted which does not comply with these terms.
*Correspondence: Xiaoyun Wang, wangxy@scnu.edu.cn