- Faculty of Life Science and Technology, Kunming University of Science and Technology, Kunming, China
Rhodosporidium kratochvilovae strain YM25235 is a cold-adapted oleaginous yeast strain that can grow at 15°C. It is capable of producing polyunsaturated fatty acids. Here, we used the Nanopore Platform to first assemble the R. kratochvilovae strain YM25235 genome into a 23.71 Mb size containing 46 scaffolds and 8,472 predicted genes. To explore the molecular mechanism behind the low temperature response of R. kratochvilovae strain YM25235, we analyzed the RNA transcriptomic data from low temperature (15°C) and normal temperature (30°C) groups using the next-generation deep sequencing technology (RNA-seq). We identified 1,300 differentially expressed genes (DEGs) by comparing the cultures grown at low temperature (15°C) and normal temperature (30°C) transcriptome libraries, including 553 significantly upregulated and 747 significantly downregulated DEGs. Gene ontology and pathway enrichment analysis revealed that DEGs were primarily related to metabolic processes, cellular processes, cellular organelles, and catalytic activity, whereas the overrepresented pathways included the MAPK signaling pathway, metabolic pathways, and amino sugar and nucleotide sugar metabolism. We validated the RNA-seq results by detecting the expression of 15 DEGs using qPCR. This study provides valuable information on the low temperature response of R. kratochvilovae strain YM25235 for further research and broadens our understanding for the response of R. kratochvilovae strain YM25235 to low temperature.
Introduction
One of the crucial ecological factors associated with abiotic stress is low temperatures, which can disrupt microbial homeostasis and affect the biological functions of cells (García-Ríos et al., 2017; Wang et al., 2020). In addition, low temperatures can severely inhibit fungal growth and may also kill it (Wang et al., 2014; Long et al., 2015). Although 10–18°C is considered as a low temperature range for fungus, these are permissive temperature (García-Ríos et al., 2017). Currently, the understanding of the molecular mechanisms of response in fungus to low temperature stress has been explored. For example, several fungal genera adapt to low temperature by changing the expression of different genes as part of the process known as the cold-shock response that has been investigated in Saccharomyces cerevisiae (Aguilera et al., 2007). During adaptation, several cold response-related genes, including those involved in energy preservation, detoxification, osmolyte production, protein folding support, and maintenance of membrane fluidity, are activated (Murata et al., 2006). Growth capacity diminishes as the temperature decreases below the optimum temperatures. Studies in Neurospora crassa have demonstrated a high accumulation of mRNAs of carotenoid genes to reduce oxidative stress under low temperature (Castrillo et al., 2018). These results suggest that differentially expressed genes (DEGs) play a key role under low temperature stress in fungi. Therefore, the identification of genes associated with low temperature response and intensive exploration for molecular mechanisms in response to low temperature at the level of gene expression in fungi are necessary.
Rhodosporidium species have been reported to be capable of synthesizing some value-added compounds with a wide industrial usage, such as lipid (Uprety et al., 2017), carotenoids (Buzzini et al., 2007), enzymes (MacDonald et al., 2016), polyunsaturated fatty acids (PUFAs) (Ageitos et al., 2011; Cui et al., 2016; Wang et al., 2017; He et al., 2019) and sugar alcohols including D-arabitol and galactitol (Jagtap and Rao, 2018; Jagtap et al., 2019). As a species of the genus Rhodosporidium, Rhodosporidium kratochvilovae strain YM25235 (isolated from Chenghai Lake, Yunnan, China) is a cold-adapted oleaginous fungal yeast strain that can grow at 15°C (He et al., 2019), and it is capable of producing PUFAs (Cui et al., 2016; Wang et al., 2017; He et al., 2019). PUFAs are involved in the maintenance of optimal physical and biological properties of cell membranes, which is an essential adaptation strategy in microorganisms against cold stress (Clarke, 2001; Sampath and Ntambi, 2004). Certain fungi produce high levels of PUFAs in response to low temperature (Calvo et al., 2001; Wang et al., 2017). We had previously reported that the strain YM25235 at 15°C produced high amounts of linoleic acid (LA, C18:2Δ9,12) and α-linolenic acid (ALA, C18:3Δ9,12,15), and the inhibition of PUFA biosynthesis negatively influenced the cold adaptation of YM25235 (Cui et al., 2016; Wang et al., 2017). In addition, cold-adapted fungal yeasts have attracted the wide attention of several scientists worldwide because of their significant potential for application in diverse industries (Margesin and Miteva, 2011; Carrasco et al., 2017). These fungal species have evolved physiological strategies to survive in cold climates, including modulation of enzyme kinetics and membrane fluidity (Firdaus-Raih et al., 2018).
For R. kratochvilovae, Miccoli et al. (2018) have completed whole genome sequencing of the strain LS11, and several other Rhodosporidium strains have also been sequenced (Hu and Ji, 2016; Zhang et al., 2016; Tran et al., 2019). In addition, multi-omics analyses of the oleaginous fungal yeast Rhodosporidium toruloides were conducted to shield lights on Pi-limitation-induced lipid accumulation (Wang et al., 2018). By comparing microbial growth and gene expression patterns, several studies demonstrated that expression levels of genes that encode carotenoid biosynthesis were altered in response to light change (Pham et al., 2020). Dinh et al. (2019) reconstructed a genome-scale model of R. toruloides IFO0880’s metabolic network. However, changes in the gene expression profile of these fungal yeasts, especially R. kratochvilovae, under temperature stress have not been investigated.
We performed this study to gain a comprehensive understanding of the molecular adaptation mechanisms to low temperatures in R. kratochvilovae strain YM25235 from the perspective of gene expression. The whole genome of R. kratochvilovae strain YM25235 was sequenced. Subsequently, based on RNA-seq data and the above-sequenced genome of strain YM25235, DEGs were identified between R. kratochvilovae at low temperature (15°C) and normal temperature (30°C) groups. Furthermore, we performed annotation of R. kratochvilovae strain YM25235 genes and functional enrichment analysis [Gene Ontology (GO) and Kyoto Encyclopedia of Genes and Genomes (KEGG) pathway] of DEGs. The DEGs were summarized, and several key pathways involved in low temperature stress tolerance were obtained from the list of KEGG terms enriched by the DEGs. This study will provide insights into the molecular adaptation mechanisms of R. kratochvilovae strain YM25235 under low temperature stress.
Materials and Methods
Culture Conditions and Cell Sample Preparation
For whole genome sequencing, R. kratochvilovae strain YM25235 was grown in yeast extract peptone dextrose (YPD: 1% yeast extract, 2% peptone, and 2% glucose) broth at 30°C to logarithmic growth phase (OD600 = 1.60). For RNA-seq, R. kratochvilovae strain YM25235 was pre-incubated in YPD broth at 30°C for 24 h and then was exposed to 15 and 30°C for another 8 h, with the final OD600 values of ∼2.1 and ∼2.3, respectively, with three technical replicates collected for each sample. The culture broth was centrifuged at 5,000 × g for 10 min at 4°C. For cell disruption, the culture pellets were ground in liquid nitrogen.
DNA Extraction, Genome Sequencing, and Assembly
Total DNA of R. kratochvilovae strain YM25235 was extracted using Wizard Genomic DNA Purification Kit (Promega, Madison, WI, United States). The next experimental procedures were performed according to the standard protocols provided by Oxford Nanopore Technologies (ONT). To optimize the sequencing experiments and improve the throughput, a library was constructed using the SQK-LSK109 Ligation Sequencing Kit. Then, the library was sequenced on the Nanopore PromethION platform. After sequencing, the downstream sequencing data were analyzed by basecalling programs using the Albacore software from the MinKNOW package to convert the raw sequencing data from FAST5 format to FASTQ format (Wick et al., 2019). Further filtering for the adaptor, low quality, and short reads (<2,000 bp in length) resulted in total dataset clean reads. Canu v1.5 software was used to correct the filtered subreads (Koren et al., 2017). Next, we assembled the subreads after error correction using the wtdbg software to obtain the final genome with high accuracy (Ruan and Li, 2019). The BUSCO v2.0 software was used to assess the completeness of the R. kratochvilovae strain YM25235 genome assembly (Simão et al., 2015).
Functional Annotation of the Genome
Because of the relatively low conservation of repeat sequences among different species, predictions of repeat sequences for a particular species require the construction of specific repeat sequence databases. Therefore, we used four software, including LTR_FINDER v1.05, MITE-Hunter, RepeatScout v1.05, and PILER-DF v2.4, to construct a repeat sequence database for the genome of R. kratochvilovae strain YM25235 based on the methods of structural and de novo predictions (Edgar and Myers, 2005; Price et al., 2005; Xu and Wang, 2007; Han and Wessler, 2010). Classification of the repeat sequences was performed using the PASTE Classifier software (Wicker et al., 2007). The type of the classified repeat sequences was subsequently annotated using the Repbase database (Jurka et al., 2005). Repeat sequences in the genome of R. kratochvilovae strain YM25235 were searched in the RepeatMasker v4.0.6 database (Chen, 2004).
The gene structure was predicted using de novo predictions and homologous protein search. Transcript evidence from sequencing transcriptome was used to validate the coding regions of genes. Finally, the prediction results from the three methods were intersected as the final sets of protein-coding genes. In particular, de novo prediction was performed using GenScan, Augustus v2.4, GlimmerHMM v3.0.4, GeneID v1.4, SNAP (version 2006–07–28) (Burge and Karlin, 1997; Stanke and Waack, 2003; Korf, 2004; Majoros et al., 2004; Alioto et al., 2018). Homologous protein-based predictions were implemented using GeMoMa v1.3.1 (Keilwagen et al., 2016); and transcript-based predictions were conducted using the PASA v2.0.2 software (Campbell et al., 2006). The intersection of the results from three methods was obtained by using EVM v1.1.1 (Haas et al., 2008).
To obtain functional annotation information of R. kratochvilovae strain YM25235 genes, the predicted gene sequences were annotated by searching them in the following gene collections using the BLAST tool: Cluster of orthologous groups for eukaryotic complete genomes (KOG) (Tatusov et al., 2000), KEGG (Kanehisa et al., 2004), Swiss-Prot (Boeckmann et al., 2003), and non-redundant protein sequence database (Nr) (Deng et al., 2006). Based on the searched results from the Nr database, the GO annotation information of genes was extracted using the in-house Perl script (Ashburner et al., 2000; Conesa et al., 2005). In addition, domains of the protein sequence of each gene were searched in the Pfam database using the Hmmer software (Eddy, 1998; Finn et al., 2016).
Extraction of RNA, Library Construction, and Sequencing
Total RNA of R. kratochvilovae strain YM25235 was purified using RNeasy Mini Kit (Qiagen, Valencia, CA, United States). After extraction of total RNA from the samples, the quality of total RNA [i.e., RNA integrity number (RIN) values, 28S/18S and concentration] was determined using the Agilent 2100 Bioanalyzer (Agilent RNA 6000 Nano Kit). The purity of the samples was assessed using a UV spectrophotometer NanoDropTM, ND-1000 (Thermo Scientific, United States). Next, the cDNA library was constructed. In brief, R. kratochvilovae strain YM25235 mRNA was enriched using magnetic beads with oligo (dT). The resulting mRNA was fragmented by adding an appropriate amount of the shearing reagent under high temperature conditions so that the interrupted mRNA was used as a template for the first-strand cDNA synthesis. Subsequently, the second strand cDNA was synthesized, purified using commercial kits, and sticky ends were repaired. The base “a” was added to the 3′ end of the cDNA and adaptors were ligated, followed by fragment size selection and PCR amplification. The quality of the constructed libraries was checked using the Agilent 2100 Bioanalyzer (Agilent Technologies, United States) and ABI StepOnePlus Real-Time PCR System.
Raw reads generated by RNA sequencing (RNA-seq) were filtered to obtain clean data by removing low-quality reads, adapter contamination, and reads with excessive unknown bases (Cock et al., 2010). Clean reads were mapped to the reference genome of R. kratochvilovae strain YM25235 to avoid contaminants caused by non-R. kratochvilovae strain YM25235 reads using Hierarchical Indexing for Spliced Alignment of Transcripts (HISAT) (Kim et al., 2015).
Quantification of Gene Expression and Identification of Differentially Expressed Genes
Clean reads were mapped to the reference gene sequences using Bowtie 2 (Langmead and Salzberg, 2012). Next, the expression of genes was assessed based on Fragments Per Kilobase per Million (FPKM) values calculated by RSEM (RNA-Seq by Expectation Maximization) methods (Li and Dewey, 2011). DEGs were identified using the DESeq2 software, an R program suitable for the identification of DEGs from high-throughput sequencing data between the control and treatment groups (Love et al., 2014). The DEGs with fold change (FC) ≥ 2 (| log2 ratio| ≥ 1) and p-values (Wald test in Deseq2) corrected by a false discovery rate (corrected p-values) < 0.05 were identified as DEGs.
Functional Enrichment Analysis of Differentially Expressed Genes
To further elucidate the biased biological function of DEGs and signaling pathways, the functional analyses of DEGs were performed using the Blast2GO pipeline (Conesa et al., 2005). The annotated functional terms of DEGs were clustered through the Functional Annotation Clustering tool in the KOBAS software (Xie et al., 2011). The KEGG pathways from each clustered set with an FDR-value < 0.01 were considered to be significantly enriched. Furthermore, the redundant GO terms were systematically discarded using GO trimming (Jantzen et al., 2011).
Validation of RNA-Seq Data and Gene Expression Analysis by qPCR
To validate the results of RNA-seq, 15 DEGs were randomly selected for downstream quantitative real-time PCR (qPCR) analysis, with three biological replicates (Table 1). Parallelly, we obtained the corresponding control sample in the same manner. Purification and evaluation of total RNA were performed using the above-mentioned methods (“Extraction of RNA, Library Construction, and Sequencing”). This was performed thrice independently to generate three biological replicates.
Specific primers used in the qPCR analysis were designed automatically using the Beacon Designer 7 (Supplementary Table 1). For the preparation of cDNA libraries, the first-strand cDNA was synthesized from total RNA using the PrimeScript RT reagent kit with gDNA eraser (TaKaRa, Tokyo, Japan). Next, we used RNase-free water to dilute the preliminary cDNA solution (final concentration, 100 ng/μL). Next, qPCR was performed on the Light Cycler 480 Real-Time PCR System (Roche, Basel, Switzerland) using the Bestar SYBR Green qPCR Master mix (DBI Bioscience, Shanghai, China). The qPCR reaction of each gene in each sample was independently repeated thrice, and experiments were performed in three biological replicates. The qPCR amplification steps were a holding stage at 95°C for 5 min, followed by the cycling stage with 40 cycles of 94°C for 10 s and 60°C for 40 s. The subsequent melt curve stage consisted of 95°C for 15 s, 60°C for 1 min, and 95°C for 15 s. Small subunit rRNA (SSU rRNA) was used as an internal control. The relative expression of target genes was calculated by comparison with SSU rRNA using the 2–ΔΔCt method. The final results are shown as means ± standard deviations (SD) (Livak and Schmittgen, 2001).
Results
Summary and Assembly Information of Genome Sequencing Data
A total of 4,795,537,118 bp raw data of R. kratochvilovae strain YM25235 were obtained from the Nanopore sequencer. Furthermore, 4,578,684,148 bp clean data were obtained. Sequencing depth was 193.04× and the total length of the assembled complete genome was 23.71 Mb, which is higher than that of R. kratochvilovae strain LS11 (22.11 Mb), R. toruloides strain NP11 (20.22 Mb), and R. paludigena (20.66 Mb). The N50 length of the assembly was 1,067,950 bp, which is higher than that of R. kratochvilovae strain LS11 (704,466 bp), R. toruloides strain NP11 (163,970 bp), and R. paludigena (371,695 bp). The average GC content of R. kratochvilovae strain YM25235 genome (67.30%) is higher than that of R. kratochvilovae strain LS11 (66.60%), R. toruloides strain NP11 (62.00%), and R. paludigena (64.3%). A total of 8,472 genes were identified in the genome of R. kratochvilovae strain YM25235, with an average length of 2,151 bp. The number of coding sequence (CDS) was 57,762, and the average length of CDS was 229.57 bp; the average number of CDS per gene was 6.82 bp. The number of introns was 50,188 bp and the average length of the intron was 82.44 bp. Each gene average contained 5.92 introns (Table 2). The results of BUSCO showed that 84.83% of the genes were completed (Table 3), indicating the high quality and completeness of the current genome (Jiang et al., 2019).
Gene Prediction and Annotation
Among the 7,907 predicted genes, 7,859 (99.39%) genes could be annotated by BLASTN (E-value < 1e–5) using NCBI Nr databases based on sequence homology. It should be noted that among these genes assigned to Nr database, the top three species of matched genes number are R. toruloides (6,180, 78.69%), followed by R. glutinis (1,087, 13.84%) and Microbotryum violaceum (226, 2.88%). In addition, 4,602 (58.20%), 4,963 (62.77%), and 7,862 (99.43%) genes could be annotated according to the KOG (Figure 1), SwissProt, and TrEMBL databases, respectively. Furthermore, 2,288 genes could be classified into three GO categories: cellular component, biological process, and molecular function (Figure 2A). To gain further insights into the gene function of R. kratochvilovae strain YM25235, we analyzed 3,143 genes and plotted the KEGG annotation classification statistics (Figure 2B). Summary for number of genes annotated in each database is shown in Supplementary Table 2. In addition, a repeat sequence of 557,107 bp was obtained, accounting for 2.35% of all sequences (Table 4).
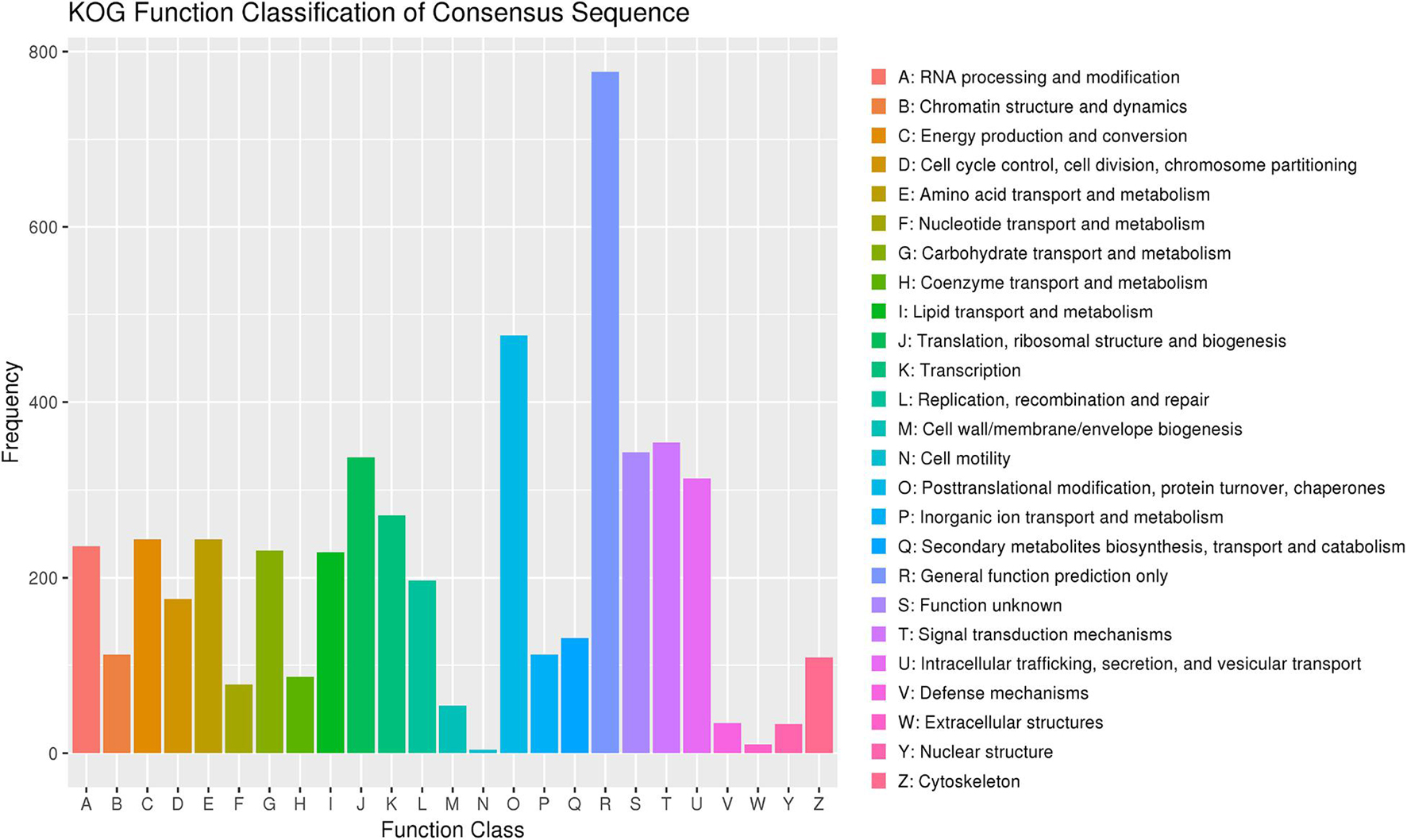
Figure 1. The Eukaryotic Orthologous Groups (KOG) classification of proteins in Rhodosporidium kratochvilovae strain YM25235. The distribution of predicted proteins from R. kratochvilovae strain YM25235 genome according to the functional class by KOG database.
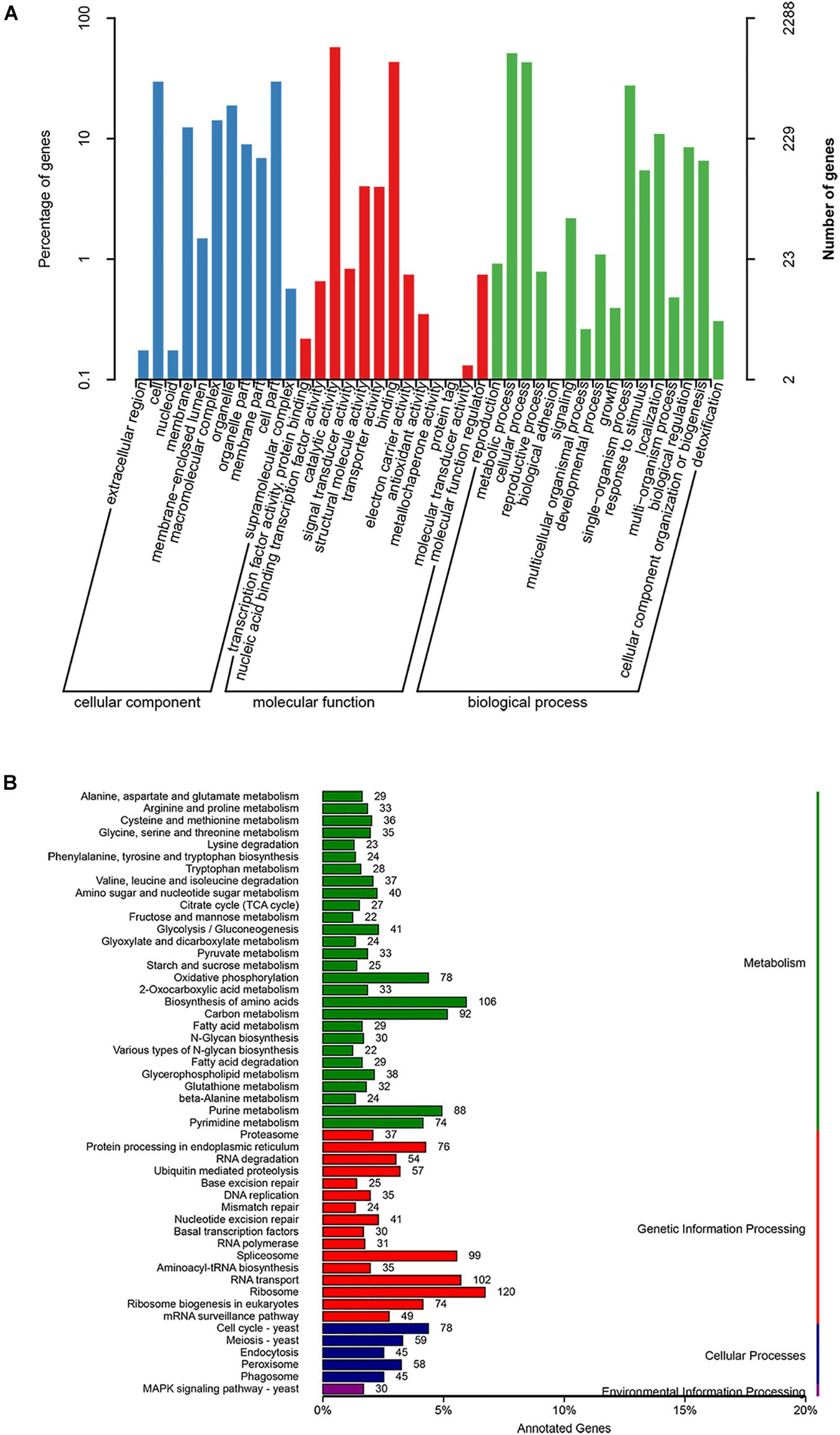
Figure 2. Gene ontology (GO) and Kyoto Encyclopedia of Genes and Genomes (KEGG) classifications of proteins in R. kratochvilovae strain YM25235. Distribution of predicted proteins from R. kratochvilovae strain YM25235 genome by (A) GO and (B) KEGG databases.
Summary of Transcriptome Sequencing Data
After filtering the raw data, a total of 32.34 and 31.12 million clean reads were obtained for the treatment and control groups, respectively. The percentage of reads mapping to the R. kratochvilovae strain YM25235 genome was 81.1% in the treatment group and 75.9% in the control group. Uniform alignment rates across samples indicate the comparability of data between the samples (Table 5). The number of predicted novel genes was 40, and a total of 8,048 expressed genes were detected, including 8,008 known genes and 40 predicted novel genes. A total of 5,227 novel transcripts were detected, of which 4,840 belonged to the novel alternatively spliced isoforms of known protein-coding genes, 40 transcripts of novel protein-coding genes, and the remaining 347 to long non-coding RNAs. For the functional annotation, the reference gene set was aligned with sequences from major databases, including Swiss-Prot, Nr, GO, and KEGG. We performed principal component analysis (PCA) among all the samples (Supplementary Figure 1).
Identification of Differential Gene Expression
Overall, 1,300 genes were significantly determined to be DEGs, which comprised 553 upregulated and 747 downregulated DEGs (Figure 3). They occupied 16.19% of all genes that were identified in this study. The top 10 DEGs with the most dramatic expression changes were listed in Table 6. The top three most upregulated DEGs were histone-binding protein RBBP4, molecular chaperone GrpE, protein of pyridoxal phosphate phosphatase-related family (PLPP). The three most downregulated DEGs were NADH oxidase (Nox), glycosyltransferase family 31 protein (GT31), and esterase/lipase (LIP). In addition, a minority of DEGs were annotated to hypothetical proteins, indicating their unknown functions. The qPCR analysis for all 15 DEGs represents a close correlation (Pearson’s correlation coefficients = 0.815, p < 0.01) in fold changes for DEGs between RNA-seq and qPCR (Figure 4), suggesting the accuracy of RNA-seq and DEG analyses.
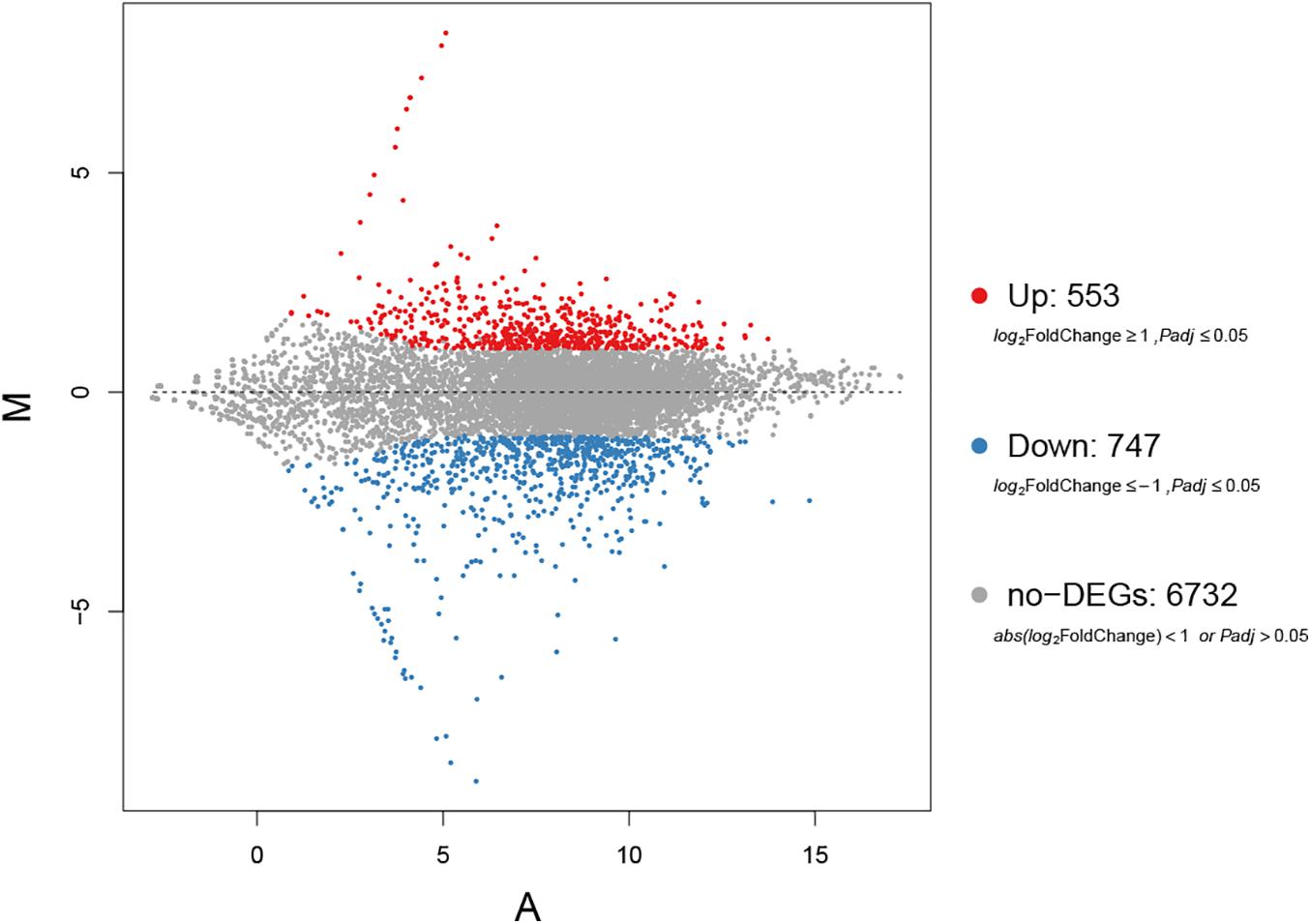
Figure 3. A volcano plot of differentially expressed genes (DEGs). The red spots indicate significant upregulation, whereas blue spots indicate significant downregulation. The black spots signify no significantly changed genes.
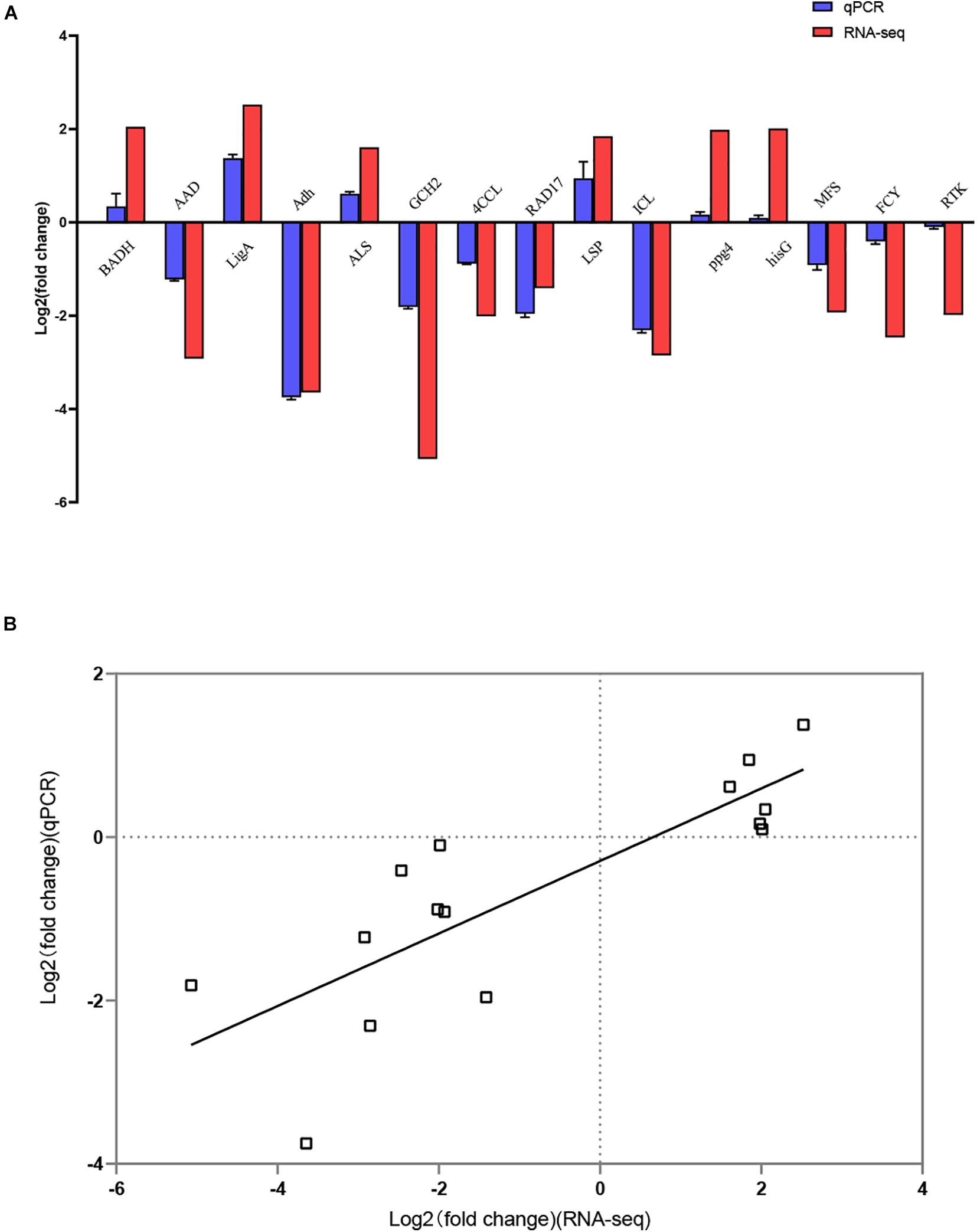
Figure 4. Confirmation of RNA-seq results by quantitative real-time PCR (qPCR). (A) Comparison of the fold change in expression of 15 selected DEGs as detected using RNA-seq and qPCR. (B) Correlation of the fold change in the expression of 15 DEGs analyzed by RNA-seq and qPCR.
GO and KEGG Enrichment Analyses of DEGs
All DEGs were divided into three GO categories: biological process (19 terms), molecular function (18 terms), and cellular component (6 terms) (Figure 5). Among the BP terms, the three GO terms containing the highest number of DEGs were metabolic process, cellular process, and cellular component organization or biogenesis. Moreover, some BP terms involving response to low temperature were detected, such as biological process, negative regulation of biological process and growth. In the MF category, catalytic activity, binding, and transporter activity were the top three terms, and for the CC category, cell, cell part, and membrane were the top three terms. In addition, the membrane part ranked fourth.
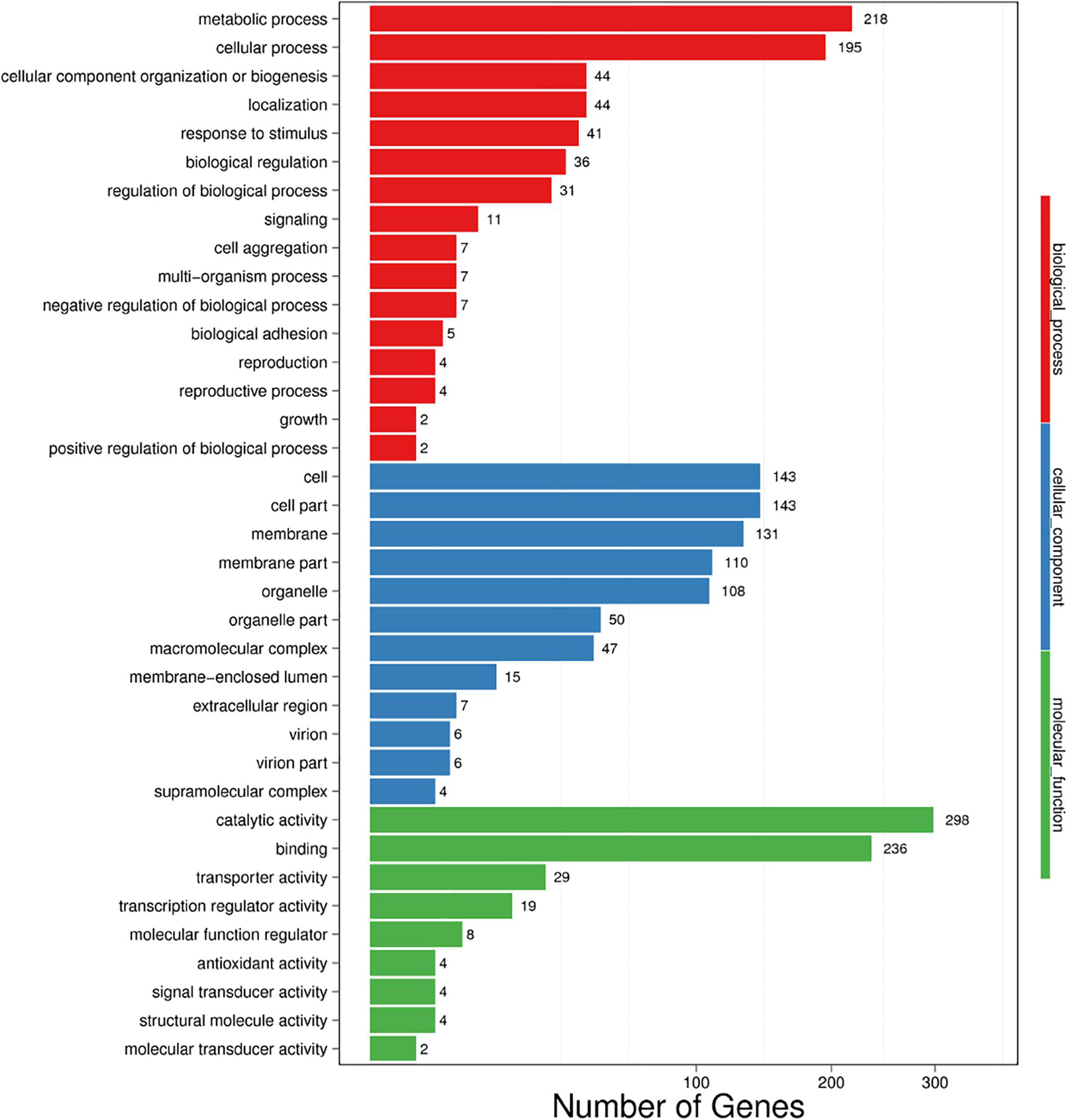
Figure 5. Vertical histogram presentation of DEG-enriched GO terms. BP (red): biological process, CC (blue): cellular component, and MF (green): molecular function.
In the pathway enrichment analysis, 107 KEGG pathways were enriched by DEGs (Supplementary Table 3). Furthermore, the top 20 KEGG pathways were enriched by DEGs and are presented in Figure 6. MAPK signaling pathway-yeast (ko04011) and metabolic pathways (ko01100) contained the most DEGs. The remaining KEGG terms were primarily associated with amino acid metabolism [e.g., alanine, aspartate, and glutamate metabolism (ko00250), valine, leucine, and isoleucine degradation (ko00310), and lysine degradation (ko00310)], carbohydrate metabolism [e.g., amino sugar and nucleotide sugar metabolism (ko00520) and propanoate metabolism (ko00640)], lipid metabolism [i.e., steroid biosynthesis (ko00100)], cell growth and death (i.e., cell cycle-yeast [ko04111] and meiosis yeast (ko04113), and translation [i.e., RNA transport (ko03013) and mRNA surveillance pathway (ko03015)]. In addition, all 1,300 DEGs included in different pathways were listed in Supplementary File 2 List of DEGs.
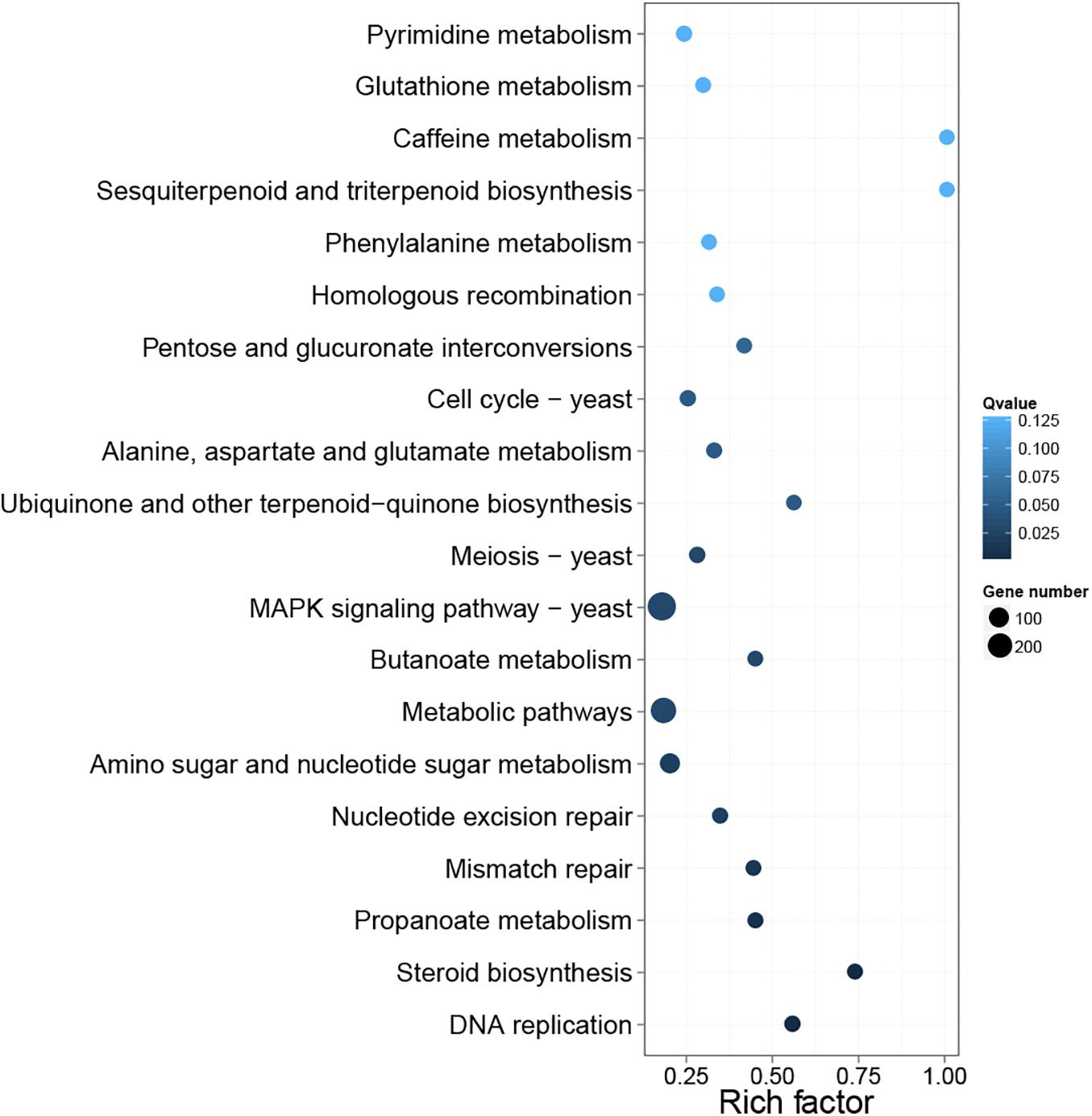
Figure 6. Top 20 differential gene pathway enrichment results. The X-axis represents the enrichment factor value, and the Y-axis represents the pathway name. Color represents the Q value, with smaller values representing more significant enrichment results. The size of the dots represents the number of DEGs. Rich factor refers to the enrichment factor value, and a larger value indicates a more pronounced enrichment result.
Discussion
Compared with several Rhodosporidium strains that have been sequenced, R. kratochvilovae strain YM25235 was isolated from Chenghai Lake in Yunnan, China. This plateau lake locates at the border of Tibet Plateau and Yunnan plateau, where the water temperature ranges from 2 to 31°C, with an average temperature of about 15°C (Wan et al., 2005). The high-quality genomic and transcriptomic data of R. kratochvilovae strain YM25235 were obtained and the molecular mechanism regarding low temperature response of R. kratochvilovae strain YM25235 was explored in this study. The comparative transcriptomic analysis of R. kratochvilovae strain YM25235 treated by low temperature and control temperature identified several DEGs primarily involved in BP functions (e.g., metabolism, regulation processes, and stress response). It was established that low temperature induced changes in the biological process of R. kratochvilovae strain YM25235. In addition, the terms associated with metabolism were overrepresented (77, 71.96% of all terms) among the enriched KEGG pathways and adjustment of metabolism function due to gene expression changes primarily contributed to the low temperature response of R. kratochvilovae strain YM25235.
In particular, steroids can serve as important components of cell membranes, which can alter the fluidity of cell membranes (Fernández-Cabezón et al., 2018). Moreover, triterpenoid serves as a precursor for steroid biosynthesis (Davis and Croteau, 2000). In this study, several KEGG pathways involved in sesquiterpenoid and triterpenoid biosynthesis were significantly enriched. This evidence suggested that steroids could act as a crucial factor for changes in the protective ability of R. kratochvilovae strain YM25235 against low temperature by altering the fluidity of cell membranes. Therefore, genes involved in steroid products are useful targets in genetic manipulation to improve the cold resistance of R. kratochvilovae strain YM25235. Another analysis revealed that the DEGs of the biosynthesis of steroid pathway were overwhelmingly upregulated (Supplementary Figure 2), further supporting that R. kratochvilovae strain YM25235 adapts to the low temperature environment by adjusting the biosynthesis of steroids. Ubiquinone and other terpenoid quinone are involved in MVA (Dallner and Sindelar, 2000). The MVA pathway, in turn, is upstream of not only steroid biosynthesis but also carotenoid biosynthesis (Katsuki and Bloch, 1967; Groeneveld, 1999). However, carotenoids could modulate membrane fluidity because carotenoids can stabilize the membrane to respond to low temperature (Chattopadhyay et al., 1997; Jagannadham et al., 2000). Low temperature stress also leads to increased intracellular reactive oxygen species (ROS) (Gasch, 2003), more carotenoids biosynthesis can mitigate cellular damage caused by ROS (Breierová et al., 2018). In this study, ubiquinone and other terpenoid quinone biosynthesis were significantly enriched. Certain amino acids, including alanine, aspartate, glutamate, and phenylalanine metabolic pathways were enriched, suggesting an altered utilization of amino acids by R. kratochvilovae strain YM25235 at low temperature, similar to the findings reported by Beltran et al. (2007). In addition, glutathione metabolism was significantly enriched, glutathione (GSH) protects the cells by neutralizing (i.e., reducing) ROS (Wu et al., 2004), Thus, GSH might protect R. kratochvilovae strain YM25235 from increased ROS at low temperature. In addition, the DNA replication, mismatch repair, and nucleotide excision repair pathways were enriched in the current study. Previous studies have reported genes whose expression is affected by low temperature associated with DNA replication (Driessen et al., 2014). Thus, it can be inferred that the changes occurring in the levels of DNA replication might be involved in the important biological processes in R. kratochvilovae strain YM25235 acting at low temperature. Moreover, most of the downregulated DEGs were related to propanoate metabolism, valine, leucine, and isoleucine degradation, pentose, and glucuronate interconversion. We speculate that low temperature limits the progression of these biological responses (Supplementary Figure 2).
MAPKs are serine/threonine kinases that, when phosphorylated, enter the nucleus and phosphorylate several transcription factors, enzymes, and other proteins that modulate the cellular activity (Cowan and Storey, 2003). The MAPK gene family is well known for its function in transmitting stress signals from the environment to the cell nucleus (Cowan and Storey, 2003). Significant enrichment of the MAPK pathway in this study indicated the implication of MAPKs in transmitting stress signals from the environment to the cell nucleus in R. kratochvilovae strain YM25235 at low temperature. Traditionally, the HOG pathway, a conserved MAPK cascade, has been considered a specific signaling pathway, responding to changes in external solute concentrations (Hohmann, 2002; Westfall et al., 2004). However, the HOG pathway was demonstrated to function as a transducer of cold stimuli (Panadero et al., 2006). Hog1, as a key gene of the HOG pathway was implicated in response to low temperature stress in our lab study (unpublished). However, Hog1 was not identified as a DEG in this study. We speculate that this process is achieved at the protein level or by certain other mechanisms and is not manifested by differences in the transcript levels.
The top 10 identified DEGs might play a key role for R. kratochvilovae strain YM25235 when grown at low temperature. The RBBP4 gene has been reported to regulate signaling through the MAPK pathway (Li et al., 2019). The activity of GrpE, a nucleotide exchange factor for the heat shock protein DnaK, is downregulated in response to increasing temperature (Bracher and Verghese, 2015). In this study, the expression of GrpE significantly increased at low temperature, indicating that GrpE is a key molecule participating in the response of R. kratochvilovae strain YM25235 to low temperature. The nucleus export protein Brr6 is involved in mRNA and protein export from the nucleus and contribute to mitosis (Lo Presti et al., 2007). The translational activity of mRNA-based protein biosynthesis greatly decreased at low temperatures (Jones and Inouye, 1996). Vitamin B6 as oxygen reactive species scavengers and factors was able to increase resistance to biotic and abiotic stress (Ehrenshaft et al., 1999; Bilski et al., 2000). This vitamin comprises six interconvertible pyridine compounds (vitamers) such as pyridoxal 5’-phosphate (PLP) and five others (ShuoHao et al., 2019). Gene that codes a protein of pyridoxal phosphate phosphatase-related family (PLPP) were significantly upregulated in this study, indicating that PLPP plays a key role in responding to low temperature. In this study, the transcript levels of certain genes involved in regulating the transcription were significantly changed at low temperature. HTH and GATA were detected with significant changes at the transcript level. Most of these proteins were transcription activators and are known to negatively regulate their expression (Baumeister et al., 1992). Thus, the results revealed potential key roles of these genes for R. kratochvilovae strain YM25235 to grow at low temperature. Notably, the functions of several top DEGs remain largely unexplored, and they cannot be linked well to the response of R. kratochvilovae strain YM25235 to low temperature. However, this study is the first to reveal their acute response of R. kratochvilovae strain YM25235 to low temperature.
Membrane rigidity increases when cells are incubated at low temperatures in vitro (Hayashi and Maeda, 2006). Microorganisms overcome the membrane stiffness at low temperatures by adapting to the environment to keep the membrane fluidity constant to a degree (Morgan-Kiss et al., 2006). To accomplish this, cold stress triggers a coordinated response that induces fatty acid desaturases and dehydrogenases, which in turn increase the ratio of polyunsaturated to saturated fatty acids and/or actually decrease the length of fatty acid chains in the membrane (Dickens and Thompson, 1981; Avery et al., 1995; Carty et al., 1999). The PUFAs association with low temperature response in R. kratochvilovae strain YM25235 has been confirmed in our previous study (Cui et al., 2016). However, no pathway and no DEGs related to PUFA biosynthesis were significantly enriched in this study. This is because the response to low temperature stress is a complex process. We speculate that this process may be achieved at the protein level or by certain other mechanisms and is not manifested by differences in the transcript levels.
Conclusion
In this study, the genome-wide transcriptional changes of R. kratochvilovae strain YM25235 at low temperature was obtained by whole genome sequencing of R. kratochvilovae strain YM25235 and transcriptome sequencing from low temperature (15°C) and normal temperature (30°C) groups. Likewise, this study also showed that there exist genes whose functions are still not known and certain mechanisms of response to low temperature cannot be elucidated. Further experimental verifications are needed. Notably, despite the DEGs obtained in this study are related to low temperature response, further verification for DEGs directly involved in the response is required in the future.
Data Availability Statement
The datasets presented in this study can be found in online repositories. The names of the repository/repositories and accession number(s) can be found below: https://www.ncbi.nlm.nih.gov/, PRJNA739038 (genome sequencing and assembly) and PRJNA739250 (Transcriptome).
Author Contributions
RG, QZ, and Q-LZ contributed to the conception and design of the study. RG and XZ did the laboratory work and acquired all raw data. RG and MH did the bioinformatic analyses. YW and XJ provided the research materials. RG wrote the first draft of the manuscript. QZ and Q-LZ wrote sections of the manuscript. All authors contributed to manuscript revision, read, and approved the submitted version.
Funding
This work was supported by the National Natural Science Foundation of China (31960466 and 31660454).
Conflict of Interest
The authors declare that the research was conducted in the absence of any commercial or financial relationships that could be construed as a potential conflict of interest.
Publisher’s Note
All claims expressed in this article are solely those of the authors and do not necessarily represent those of their affiliated organizations, or those of the publisher, the editors and the reviewers. Any product that may be evaluated in this article, or claim that may be made by its manufacturer, is not guaranteed or endorsed by the publisher.
Supplementary Material
The Supplementary Material for this article can be found online at: https://www.frontiersin.org/articles/10.3389/fmicb.2021.727105/full#supplementary-material
References
Ageitos, J. M., Vallejo, J. A., Veiga-Crespo, P., and Villa, T. G. (2011). Oily yeasts as oleaginous cell factories. Appl. Microbiol. Biotechnol. 90, 1219–1227. doi: 10.1007/s00253-011-3200-z
Aguilera, J., Randez-Gil, F., and Prieto, J. A. (2007). Cold response in Saccharomyces cerevisiae: new functions for old mechanisms. FEMS Microbiol. Rev. 31, 327–341. doi: 10.1111/j.1574-6976.2007.00066.x
Alioto, T., Blanco, E., Parra, G., and Guigó, R. (2018). Using geneid to Identify Genes. Curr. Protoc. Bioinform. 64:e56. doi: 10.1002/cpbi.56
Ashburner, M., Ball, C. A., Blake, J. A., Botstein, D., Butler, H., Cherry, J. M., et al. (2000). Gene ontology: tool for the unification of biology. Nat. Genet. 25, 25–29. doi: 10.1038/75556
Avery, S. V., Lloyd, D., and Harwood, J. L. (1995). Temperature-dependent changes in plasma-membrane lipid order and the phagocytotic activity of the amoeba Acanthamoeba castellanii are closely correlated. Biochem. J. 312, 811–816. doi: 10.1042/bj3120811
Baumeister, R., Müller, G., Hecht, B., and Hillen, W. (1992). Functional roles of amino acid residues involved in forming the alpha-helix-turn-alpha-helix operator DNA binding motif of Tet repressor from Tn10. Proteins 14, 168–177. doi: 10.1002/prot.340140204
Beltran, G., Rozès, N., Mas, A., and Guillamón, J. M. (2007). Effect of low-temperature fermentation on yeast nitrogen metabolism. World J. Microbiol. Biotechnol. 23, 809–815. doi: 10.1007/s11274-006-9302-6
Bilski, P., Li, M., Ehrenshaft, M., Daub, M., and Chignell, C. (2000). Vitamin B6 (pyridoxine) and its derivatives are efficient singlet oxygen quenchers and potential fungal antioxidants. Photochem. Photobiol. 71, 129–134. doi: 10.1562/0031-865520000710129SIPVBP2.0.CO2
Boeckmann, B., Bairoch, A., Apweiler, R., Blatter, M.-C., Estreicher, A., Gasteiger, E., et al. (2003). The SWISS-PROT protein knowledgebase and its supplement TrEMBL in 2003. Nucleic Acids Res. 31, 365–370. doi: 10.1093/nar/gkg095
Bracher, A., and Verghese, J. (2015). The nucleotide exchange factors of Hsp70 molecular chaperones. Front. Mol. Biosci. 2:10. doi: 10.3389/fmolb.2015.00010
Breierová, E., Čertík, M., Márová, I., and Vadkertiová, R. (2018). The effect of Zn(II) ions and reactive oxygen on the uptake of zinc and production of carotenoids by selected red yeasts. Chem. Biodiv. 15:e1800069. doi: 10.1002/cbdv.201800069
Burge, C., and Karlin, S. (1997). Prediction of complete gene structures in human genomic DNA. J. Mol. Biol. 268, 78–94. doi: 10.1006/jmbi.1997.0951
Buzzini, P., Innocenti, M., Turchetti, B., Libkind, D., van Broock, M., and Mulinacci, N. (2007). Carotenoid profiles of yeasts belonging to the genera Rhodotorula, Rhodosporidium, Sporobolomyces, and Sporidiobolus. Can. J. Microbiol. 53, 1024–1031. doi: 10.1139/W07-068
Calvo, A. M., Gardner, H. W., and Keller, N. P. (2001). Genetic connection between fatty acid metabolism and sporulation in Aspergillus nidulans. J. Biol. Chem. 276, 25766–25774. doi: 10.1074/jbc.M100732200
Campbell, M. A., Haas, B. J., Hamilton, J. P., Mount, S. M., and Buell, C. R. (2006). Comprehensive analysis of alternative splicing in rice and comparative analyses with Arabidopsis. BMC Genom. 7:327. doi: 10.1186/1471-2164-7-327
Carrasco, M., Alcaíno, J., Cifuentes, V., and Baeza, M. (2017). Purification and characterization of a novel cold adapted fungal glucoamylase. Microbial. Cell Factories 16:75. doi: 10.1186/s12934-017-0693-x
Carty, S. M., Sreekumar, K. R., and Raetz, C. R. H. (1999). Effect of cold shock on lipid a biosynthesis in Escherichia coli. J. Biol. Chem. 274, 9677–9685. doi: 10.1074/jbc.274.14.9677
Castrillo, M., Luque, E. M., Pardo-Medina, J., Limón, M. C., Corrochano, L. M., and Avalos, J. (2018). Transcriptional basis of enhanced photoinduction of carotenoid biosynthesis at low temperature in the fungus Neurospora crassa. Res. Microbiol. 169, 78–89. doi: 10.1016/j.resmic.2017.11.003
Chattopadhyay, M., Jagannadham, M., Vairamani, M., and Shivaji, S. (1997). Carotenoid pigments of an Antarctic psychrotrophic bacterium Micrococcus roseus: temperature dependent biosynthesis, structure, and interaction with synthetic membranes. Biochem. Biophys. Res. Commun. 239, 85–90. doi: 10.1006/bbrc.1997.7433
Chen, N. (2004). Using repeat masker to identify repetitive elements in genomic sequences. Curr. Protoc. Bioinform. Chapter 4:Unit4.10. doi: 10.1002/0471250953.bi0410s05
Clarke, S. D. (2001). Polyunsaturated fatty acid regulation of gene transcription: a molecular mechanism to improve the metabolic syndrome. J. Nut. 131, 1129–1132. doi: 10.1093/jn/131.4.1129
Cock, P. J., Fields, C. J., Goto, N., Heuer, M. L., and Rice, P. M. (2010). The sanger FASTQ file format for sequences with quality scores, and the Solexa/Illumina FASTQ variants. Nucleic Acids Res. 38, 1767–1771. doi: 10.1093/nar/gkp1137
Conesa, A., Gotz, S., Garcia-Gomez, J. M., Terol, J., Talon, M., and Robles, M. (2005). Blast2GO: a universal tool for annotation, visualization and analysis in functional genomics research. Bioinformatics 21, 3674–3676. doi: 10.1093/bioinformatics/bti610
Cowan, K. J., and Storey, K. B. (2003). Mitogen-activated protein kinases: new signaling pathways functioning in cellular responses to environmental stress. J. Exp. Biol. 206, 1107–1115. doi: 10.1242/jeb.00220
Cui, J., He, S., Ji, X., Lin, L., Wei, Y., and Zhang, Q. (2016). Identification and characterization of a novel bifunctional Δ12/Δ15-fatty acid desaturase gene from Rhodosporidium kratochvilovae. Biotechnol. Lett. 38, 1155–1164. doi: 10.1007/s10529-016-2090-7
Dallner, G., and Sindelar, P. J. (2000). Regulation of ubiquinone metabolism. Free Radical Biol. Med. 29, 285–294. doi: 10.1016/s0891-5849(00)00307-5
Davis, E. M., and Croteau, R. (2000). “Cyclization Enzymes in the Biosynthesis of Monoterpenes, Sesquiterpenes, and Diterpenes,” in Biosynthesis: Aromatic Polyketides, Isoprenoids, Alkaloids, eds F. J. Leeper and J. C. Vederas (Berlin: Springer Berlin Heidelberg), 53–95. doi: 10.1007/3-540-48146-X_2
Deng, Y. Y., Li, J. Q., Wu, S. F., Zhu, Y., Chen, Y. W., and He, F. C. (2006). Integrated nr database in protein annotation system and its localization. Computer Eng. 32, 71–72.
Dickens, B. F., and Thompson, G. A. (1981). Rapid membrane response during low-temperature acclimation Correlation of early changes in the physical properties and lipid composition of Tetrahymena microsomal membranes. Biochim. et Biophys. Acta (BBA) Biomembranes 644, 211–218. doi: 10.1016/0005-2736(81)90377-1
Dinh, H. V., Suthers, P. F., Chan, S. H. J., Shen, Y., Xiao, T., Deewan, A., et al. (2019). A comprehensive genome-scale model for Rhodosporidium toruloides IFO0880 accounting for functional genomics and phenotypic data. Metabolic Eng. Commun. 9:e00101. doi: 10.1016/j.mec.2019.e00101
Driessen, R. P. C., Sitters, G., Laurens, N., Moolenaar, G. F., Wuite, G. J. L., Goosen, N., et al. (2014). Effect of temperature on the intrinsic flexibility of DNA and its interaction with architectural proteins. Biochemistry 53, 6430–6438. doi: 10.1021/bi500344j
Eddy, S. R. (1998). Profile hidden markov models. Bioinformatics 14, 755–763. doi: 10.1093/bioinformatics/14.9.755
Edgar, R. C., and Myers, E. W. (2005). PILER: identification and classification of genomic repeats. Bioinformatics 21(Suppl. 1), i152–i158. doi: 10.1093/bioinformatics/bti1003
Ehrenshaft, M., Bilski, P., Li, M. Y., Chignell, C. F., and Daub, M. E. (1999). A highly conserved sequence is a novel gene involved in de novo vitamin B6 biosynthesis. Proc. Natl. Acad. Sci. U.S.A. 96, 9374–9378. doi: 10.1073/pnas.96.16.9374
Fernández-Cabezón, L., Galán, B., and García, J. L. (2018). New insights on steroid biotechnology. Front. Microbiol. 9:958. doi: 10.3389/fmicb.2018.00958
Finn, R. D., Coggill, P., Eberhardt, R. Y., Eddy, S. R., Mistry, J., Mitchell, A. L., et al. (2016). The Pfam protein families database: towards a more sustainable future. Nucleic Acids Res. 44, D279–D285. doi: 10.1093/nar/gkv1344
Firdaus-Raih, M., Hashim, N. H. F., Bharudin, I., Abu Bakar, M. F., Huang, K. K., Alias, H., et al. (2018). The Glaciozyma antarctica genome reveals an array of systems that provide sustained responses towards temperature variations in a persistently cold habitat. PLoS One 13:e0189947. doi: 10.1371/journal.pone.0189947
García-Ríos, E., Morard, M., Parts, L., Liti, G., and Guillamón, J. M. (2017). The genetic architecture of low-temperature adaptation in the wine yeast Saccharomyces cerevisiae. BMC Genom. 18:159. doi: 10.1186/s12864-017-3572-2
Gasch, A. P. (2003). “The environmental stress response: a common yeast response to diverse environmental stresses,” in Yeast Stress Responses, eds S. Hohmann and W. H. Mager (Berlin: Springer Berlin Heidelberg), 11–70. doi: 10.1007/3-540-45611-2_2
Groeneveld, H. W. (1999). Tracing steroid synthesis in plants. Crit. Rev. Biochem. Mol. Biol. 34, 59–69. doi: 10.1080/10409239991209192
Haas, B. J., Salzberg, S. L., Zhu, W., Pertea, M., Allen, J. E., Orvis, J., et al. (2008). Automated eukaryotic gene structure annotation using evidencemodeler and the program to assemble spliced alignments. Genome Biol. 9:R7. doi: 10.1186/gb-2008-9-1-r7
Han, Y., and Wessler, S. R. (2010). MITE-hunter: a program for discovering miniature inverted-repeat transposable elements from genomic sequences. Nucleic Acids Res. 38:e199. doi: 10.1093/nar/gkq862
Hayashi, M., and Maeda, T. (2006). Activation of the HOG pathway upon cold stress in Saccharomyces cerevisiae. J. Biochem. 139, 797–803. doi: 10.1093/jb/mvj089
He, J., Cui, Z., Ji, X., Luo, Y., Wei, Y., and Zhang, Q. (2019). Novel histidine kinase gene HisK2301from Rhodosporidium kratochvilovae contributes to cold adaption by promoting biosynthesis of polyunsaturated fatty acids and glycerol. J. Agric. Food Chem. 67, 653–660. doi: 10.1021/acs.jafc.8b04859
Hohmann, S. (2002). Osmotic stress signaling and osmoadaptation in yeasts. Microbiol. Mol. Biol. Rev. MMBR 66, 300–372. doi: 10.1128/MMBR.66.2.300-372.2002
Hu, J., and Ji, L. (2016). Draft genome sequences of Rhodosporidium toruloides strains ATCC 10788 and ATCC 10657 with compatible mating types. Genome Announ. 4:e00098-16. doi: 10.1128/genomeA.00098-16
Jagannadham, M. V., Chattopadhyay, M. K., Subbalakshmi, C., Vairamani, M., Narayanan, K., Mohan Rao, C., et al. (2000). Carotenoids of an Antarctic psychrotolerant bacterium, Sphingobacterium antarcticus, and a mesophilic bacterium, Sphingobacterium multivorum. Arch. Microbiol. 173, 418–424. doi: 10.1007/s002030000163
Jagtap, S. S., Bedekar, A. A., Liu, J.-J., Jin, Y.-S., and Rao, C. V. (2019). Production of galactitol from galactose by the oleaginous yeast Rhodosporidium toruloides IFO0880. Biotechnol. Biofuels 12:250. doi: 10.1186/s13068-019-1586-5
Jagtap, S. S., and Rao, C. V. (2018). Production of d-arabitol from d-xylose by the oleaginous yeast Rhodosporidium toruloides IFO0880. Appl. Microbiol. Biotechnol. 102, 143–151. doi: 10.1007/s00253-017-8581-1
Jantzen, S. G., Sutherland, B. J., Minkley, D. R., and Koop, B. F. (2011). GO trimming: systematically reducing redundancy in large gene ontology datasets. BMC Res. Notes 4:267. doi: 10.1186/1756-0500-4-267
Jiang, W., Lv, Y., Cheng, L., Yang, K., Bian, C., Wang, X., et al. (2019). Whole-genome sequencing of the giant devil catfish, Bagarius yarrelli. Genome Biol. Evol. 11, 2071–2077. doi: 10.1093/gbe/evz143
Jones, P. G., and Inouye, M. (1996). RbfA, a 30S ribosomal binding factor, is a cold-shock protein whose absence triggers the cold-shock response. Mol. Microbiol. 21, 1207–1218. doi: 10.1111/j.1365-2958.1996.tb02582.x
Jurka, J., Kapitonov, V. V., Pavlicek, A., Klonowski, P., Kohany, O., and Walichiewicz, J. (2005). Repbase update, a database of eukaryotic repetitive elements. Cytogenet Genome Res. 110, 462–467. doi: 10.1159/000084979
Kanehisa, M., Goto, S., Kawashima, S., Okuno, Y., and Hattori, M. (2004). The KEGG resource for deciphering the genome. Nucleic Acids Res. 32, D277–D280. doi: 10.1093/nar/gkh063
Katsuki, H., and Bloch, K. (1967). Studies on the biosynthesis of ergosterol in yeast: formation of methylated intermediates. J. Biol. Chem. 242, 222–227. doi: 10.1016/S0021-9258(19)81452-7
Keilwagen, J., Wenk, M., Erickson, J. L., Schattat, M. H., Grau, J., and Hartung, F. (2016). Using intron position conservation for homology-based gene prediction. Nucleic Acids Res. 44:e89. doi: 10.1093/nar/gkw092
Kim, D., Langmead, B., and Salzberg, S. L. (2015). HISAT: a fast spliced aligner with low memory requirements. Nat. Methods 12, 357–360. doi: 10.1038/nmeth.3317
Koren, S., Walenz, B. P., Berlin, K., Miller, J. R., Bergman, N. H., and Phillippy, A. M. (2017). Canu: scalable and accurate long-read assembly via adaptive k-mer weighting and repeat separation. Genome Res. 27, 722–736. doi: 10.1101/gr.215087.116
Langmead, B., and Salzberg, S. L. (2012). Fast gapped-read alignment with bowtie 2. Nat. Methods 9, 357–359. doi: 10.1038/nmeth.1923
Li, B., and Dewey, C. N. (2011). RSEM: accurate transcript quantification from RNA-Seq data with or without a reference genome. BMC Bioinform. 12:323. doi: 10.1186/1471-2105-12-323
Li, Y. D., Lv, Z., Xie, H. Y., and Zheng, S. S. (2019). Retinoblastoma binding protein 4 up-regulation is correlated with hepatic metastasis and poor prognosis in colon cancer patients. Hepatobil. Pancreat. Dis. Int. 18, 446–451. doi: 10.1016/j.hbpd.2019.08.006
Livak, K. J., and Schmittgen, T. D. (2001). Analysis of relative gene expression data using real-time quantitative PCR and the 2−ΔΔCT method. Methods 25, 402–408. doi: 10.1006/meth.2001.1262
Lo Presti, L., Cockell, M., Cerutti, L., Simanis, V., and Hauser, P. M. (2007). Functional characterization of Pneumocystis carinii brl1 by transspecies complementation analysis. Eukaryot Cell 6, 2448–2452. doi: 10.1128/EC.00321-07
Long, Y., Yan, J., Song, G., Li, X., Li, X., Li, Q., et al. (2015). Transcriptional events co-regulated by hypoxia and cold stresses in Zebrafish larvae. BMC Genomics 16:385. doi: 10.1186/s12864-015-1560-y
Love, M. I., Huber, W., and Anders, S. (2014). Moderated estimation of fold change and dispersion for RNA-seq data with DESeq2. Genome Biol. 15:550. doi: 10.1186/s13059-014-0550-8
MacDonald, M. C., Arivalagan, P., Barre, D. E., MacInnis, J. A., and D’Cunha, G. B. (2016). Rhodotorula glutinis Phenylalanine/tyrosine ammonia lyase enzyme catalyzed synthesis of the methyl ester of para-hydroxycinnamic acid and its potential antibacterial activity. Front. Microbiol. 7:281. doi: 10.3389/fmicb.2016.00281
Majoros, W. H., Pertea, M., and Salzberg, S. L. (2004). Tigr scan and glimmer HMM: two open source ab initio eukaryotic gene-finders. Bioinformatics 20, 2878–2879. doi: 10.1093/bioinformatics/bth315
Margesin, R., and Miteva, V. (2011). Diversity and ecology of psychrophilic microorganisms. Res. Microbiol. 162, 346–361. doi: 10.1016/j.resmic.2010.12.004
Miccoli, C., Palmieri, D., De Curtis, F., Lima, G., Ianiri, G., and Castoria, R. (2018). Complete genome sequence of the biocontrol agent yeast Rhodotorula kratochvilovae strain LS11. Genome Announ. 6:e00120-18. doi: 10.1128/genomeA.00120-18
Morgan-Kiss, R. M., Priscu, J. C., Pocock, T., Gudynaite-Savitch, L., and Huner, N. P. A. (2006). Adaptation and acclimation of photosynthetic microorganisms to permanently cold environments. Microbiol. Mol. Biol. Rev. MMBR 70, 222–252. doi: 10.1128/MMBR.70.1.222-252.2006
Murata, Y., Homma, T., Kitagawa, E., Momose, Y., Sato, M. S., Odani, M., et al. (2006). Genome-wide expression analysis of yeast response during exposure to 4 C. Extremophiles 10, 117–128. doi: 10.1007/s00792-005-0480-1
Panadero, J., Pallotti, C., Rodríguez-Vargas, S., Randez-Gil, F., and Prieto, J. A. (2006). A downshift in temperature activates the high osmolarity glycerol (HOG) pathway, which determines freeze tolerance in Saccharomyces cerevisiae∗. J. Biol. Chem. 281, 4638–4645. doi: 10.1074/jbc.M512736200
Pham, K. D., Shida, Y., Miyata, A., Takamizawa, T., Suzuki, Y., Ara, S., et al. (2020). Effect of light on carotenoid and lipid production in the oleaginous yeast Rhodosporidium toruloides. Biosci. Biotechnol. Biochem. 84, 1501–1512. doi: 10.1080/09168451.2020.1740581
Price, A. L., Jones, N. C., and Pevzner, P. A. (2005). De novo identification of repeat families in large genomes. Bioinformatics 21(Suppl. 1), i351–i358. doi: 10.1093/bioinformatics/bti1018
Ruan, J., and Li, H. (2019). Fast and accurate long-read assembly with wtdbg2. Nat. Methods 17, 155–158. doi: 10.1101/530972
Sampath, H., and Ntambi, J. M. (2004). Polyunsaturated fatty acid regulation of gene expression. Nutrit. Rev. 62, 333–339. doi: 10.1111/j.1753-4887.2004.tb00058.x
ShuoHao, H., Jing, L., Jie, Z., JianYun, Z., and LongQuan, H. (2019). Identification and characterization of a pyridoxal 5’-phosphate phosphatase in tobacco plants. Plant Sci. 278, 88–95. doi: 10.1016/j.plantsci.2018.10.014
Simão, F., Waterhouse, R. M., Panagiotis, I., Kriventseva, E. V., and Zdobnov, E. M. (2015). BUSCO: assessing genome assembly and annotation completeness with single-copy orthologs. Bioinformatics 31, 3210–3212. doi: 10.1093/bioinformatics/btv351
Stanke, M., and Waack, S. (2003). Gene prediction with a hidden Markov model and a new intron submodel. Bioinformatics 19(Suppl. 2), ii215–ii225. doi: 10.1093/bioinformatics/btg1080
Tatusov, R. L., Galperin, M. Y., Natale, D. A., and Koonin, E. V. (2000). The COG database: a tool for genome-scale analysis of protein functions and evolution. Nucleic Acids Res. 28, 33–36. doi: 10.1093/nar/28.1.33
Tran, T. N., Ngo, D.-H., Nguyen, N. T., and Ngo, D.-N. (2019). Draft genome sequence data of Rhodosporidium toruloides VN1, a strain capable of producing natural astaxanthin. Data Brief 26:104443. doi: 10.1016/j.dib.2019.104443
Uprety, B. K., Dalli, S. S., and Rakshit, S. K. (2017). Bioconversion of crude glycerol to microbial lipid using a robust oleaginous yeast Rhodosporidium toruloides ATCC 10788 capable of growing in the presence of impurities. Energy Conv. Manag. 135, 117–128. doi: 10.1016/j.enconman.2016.12.071
Wan, G. J., Chen, J. A., Wu, F. C., Xu, S. Q., Bai, Z. G., Wan, E. Y., et al. (2005). Coupling between 210Pbex and organic matter in sediments of a nutrient-enriched lake: an example from Lake Chenghai, China. Chem. Geol. 224, 223–236. doi: 10.1016/j.chemgeo.2005.07.025
Wang, C., Chen, Y., Zhou, H., Li, X., and Tan, Z. (2020). Adaptation mechanisms of Rhodococcus sp. CNS16 under different temperature gradients: Physiological and transcriptome. Chemosphere 238:124571. doi: 10.1016/j.chemosphere.2019.124571
Wang, J., Chen, W., Nian, H., Ji, X., Lin, L., Wei, Y., et al. (2017). Inhibition of polyunsaturated fatty acids synthesis decreases growth rate and membrane fluidity of Rhodosporidium kratochvilovae at low temperature. Lipids 52, 729–735. doi: 10.1007/s11745-017-4273-y
Wang, Q., Tan, X., Jiao, S., You, F., and Zhang, P. J. (2014). Analyzing cold tolerance mechanism in transgenic zebrafish (Danio rerio). PLoS One 9:e102492. doi: 10.1371/journal.pone.0102492
Wang, Y., Zhang, S., Zhu, Z., Shen, H., Lin, X., Jin, X., et al. (2018). Systems analysis of phosphate-limitation-induced lipid accumulation by the oleaginous yeast Rhodosporidium toruloides. Biotechnol. Biofuels 11:148. doi: 10.1186/s13068-018-1134-8
Westfall, P. J., Ballon, D. R., and Thorner, J. (2004). When the stress of your environment makes you go hog wild. Science 306:1511. doi: 10.1126/science.1104879
Wick, R. R., Judd, L. M., and Holt, K. E. (2019). Performance of neural network basecalling tools for Oxford Nanopore sequencing. Genome Biol. 20:129. doi: 10.1186/s13059-019-1727-y
Wicker, T., Sabot, F., Hua-Van, A., Bennetzen, J. L., Capy, P., Chalhoub, B., et al. (2007). A unified classification system for eukaryotic transposable elements. Nat. Rev. Genet. 8, 973–982. doi: 10.1038/nrg2165
Wu, G., Fang, Y.-Z., Yang, S., Lupton, J. R., and Turner, N. D. (2004). Glutathione metabolism and its implications for health. J. Nut. 134, 489–492. doi: 10.1093/jn/134.3.489
Xie, C., Mao, X., Huang, J., Ding, Y., Wu, J., Dong, S., et al. (2011). KOBAS 2.0: a web server for annotation and identification of enriched pathways and diseases. Nucleic Acids Res. 39(Suppl. 2), W316–W322. doi: 10.1093/nar/gkr483
Xu, Z., and Wang, H. (2007). LTR_FINDER: an efficient tool for the prediction of full-length LTR retrotransposons. Nucleic Acids Res. 35, W265–W268. doi: 10.1093/nar/gkm286
Keywords: Rhodosporidium kratochvilovae, low temperature, genome sequencing, differential expressed genes, RNA-Seq, qPCR
Citation: Guo R, He M, Zhang X, Ji X, Wei Y, Zhang Q-L and Zhang Q (2021) Genome-Wide Transcriptional Changes of Rhodosporidium kratochvilovae at Low Temperature. Front. Microbiol. 12:727105. doi: 10.3389/fmicb.2021.727105
Received: 18 June 2021; Accepted: 26 August 2021;
Published: 16 September 2021.
Edited by:
Gloria Paz Levicán, University of Santiago, ChileReviewed by:
Fang-Fang Wang, Institute of Microbiology, Chinese Academy of Sciences (CAS), ChinaSujit Jagtap, University of Illinois at Urbana-Champaign, United States
Copyright © 2021 Guo, He, Zhang, Ji, Wei, Zhang and Zhang. This is an open-access article distributed under the terms of the Creative Commons Attribution License (CC BY). The use, distribution or reproduction in other forums is permitted, provided the original author(s) and the copyright owner(s) are credited and that the original publication in this journal is cited, in accordance with accepted academic practice. No use, distribution or reproduction is permitted which does not comply with these terms.
*Correspondence: Qi-Lin Zhang, emhhbmdxbEBrdXN0LmVkdS5jbg==; Qi Zhang, cXpoYW5nMzdAa3VzdC5lZHUuY24=