- Institute of Animal Husbandry and Veterinary Science, Zhejiang Academy of Agricultural Sciences, Hangzhou, China
Low protein diets are commonly used in the growing-finishing pig stage of swine production; however, the effects of low dietary protein on the intestinal microbiota and their metabolites, and their association with pig sex, remain unclear. The present study aimed to assess the impact of a low crude protein (CP) diet on the gut microbiome and metabolome, and to reveal any relationship with sex. Barrows and gilts (both n = 24; initial body = 68.33 ± 0.881 kg) were allocated into two treatments according to sex. The four groups comprised two pairs of gilts and barrows fed with a high protein diet (CP 17% at stage I; CP 13% at stage II) and a low protein diet (CP 15% at stage I; CP 11% at stage II), respectively, for 51 d. Eight pigs in each group were slaughtered and their colon contents were collected. Intestinal microbiota and their metabolites were assessed using 16S rRNA sequencing and tandem mass spectrometry, respectively. The low protein diet increased intestinal microbiota species and richness indices (P < 0.05) in both sexes compared with the high protein diet. The sample Shannon index was different (P < 0.01) between barrows and gilts. At the genus level, unidentified Clostridiales (P < 0.05), Neisseria (P < 0.05), unidentified Prevotellaceae (P < 0.01) and Gracilibacteria (P < 0.05) were affected by dietary protein levels. The relative abundance of unidentified Prevotellaceae was different (P < 0.01) between barrows and gilts. The influence of dietary protein levels on Neisseria (P < 0.05), unidentified Prevotellaceae (P < 0.01) and Gracilibacteria (P < 0.05) were associated with sex. Metabolomic profiling indicated that dietary protein levels mainly affected intestinal metabolites in gilts rather than barrows. A total of 434 differentially abundant metabolites were identified in gilts fed the two protein diets. Correlation analysis identified that six differentially abundant microbiota communities were closely associated with twelve metabolites that were enriched for amino acids, inflammation, immune, and disease-related metabolic pathways. These results suggested that decreasing dietary protein contents changed the intestinal microbiota in growing-finishing pigs, which selectively affected the intestinal metabolite profiles in gilts.
Introduction
In swine production, normal dietary crude protein (CP) concentration is about 15–22%. However, reducing dietary protein levels has marked benefits, including increasing nitrogen (N) utilization, lessening seasonally feed costs, especially reducing the excretion of N into the environment. Although the recommendation for the dietary CP content was replaced with total N in the new edition of the NRC guidelines [National Research Council (NRC), 2012]. CP% could be calculated by multiplying the common CP coefficient of 6.25, thus dietary CP content recommended by NRC (2012) is reckoned decreasing by 2–4% than the previous edition NRC (1998) [National Research Council (NRC), 1998]. Furthermore, with the development of industrial synthetic amino acids (AA) technology, low protein diet strategy has become increasingly popular, especially in growing-finishing pigs, because pigs in this stage have very large populations, gain the most weight, consume large amounts of dietary protein, and excrete the maximum amount of nitrogen as slurry. Studies in piglets showed that dietary protein levels altered the intestinal microbiota compositions and microbially-derived metabolites (Rist et al., 2013). In growing-finishing pigs, because of their relatively stable microbiota structure, studies focused on environmental influences of N extraction (Zhao et al., 2019; Kim et al., 2020; Wang Y. et al., 2020). In fact, increasing studies indicate that the compositions and structure of the intestinal microbiota can change dynamically in response to many factors, including feed (Isaacson and Kim, 2012; Ananthakrishnan, 2015). Even in older life, the gut microbiota has the propensity of accelerating compositional change (Gi Langille et al., 2014). More significantly, sex-specific differences of intestinal microbiota due to sex hormones in human have been reported (de la Cuesta-Zuluaga et al., 2019). Therefore, we are interested in whether the impacts of dietary nutrients on intestine microbiota and metabolites are associated with pig sex. This is very important for precision nutrition of sex-specific feeding measures.
The mammalian gastrointestinal tract is colonized by thousands of microbial strains that form highly dense, dynamically changing, and extremely complicated communities exceeding 100 trillion microbial cells (Collins et al., 2012). These intestinal microorganisms, which metabolize dietary substances to obtain nutrients and energy while producing other metabolites, play vital roles in host physiology and nutrient metabolism (Lee and Hase, 2014). Therefore, dietary nutrients containing proteins, lipids, and carbohydrates decide the available substrates for the host intestinal microbiota, and affect microbial populations and their metabolic activities. Alternatively, the intestinal microbiota and their metabolic substances that are changed by dietary nutrients could further affect host health and physiological function (Shibata et al., 2017). In particular, intestinal microbial-derived metabolites have been reported recently to control gut inflammatory responses (Arpaia and Rudensky, 2014), thus directly influencing animal body health (Flint, 2016; Glowacki and Martens, 2020), and regulating host immunity (Kim et al., 2016). Therefore, revealing the relationship among dietary nutrients and intestinal microbiota and metabolites is important to improve the health and growth of mammals by regulating dietary compositions.
Protein is an essential nutritional component in human and animal diets throughout life processes. However, undigested excess dietary protein and amino acid will remain in the large intestine, and be fermented by abundant microbes, and generate some metabolites such as ammonia, hydrogen sulfide, and phenolic compounds which will have toxic influences on the host (Libao-Mercado et al., 2009; Kårlund et al., 2019). The microbial fermentation-derived metabolites from proteins are increasing recognized to induce the gut inflammatory response, increase intestinal permeability, and colitis, and are involved in the development of certain metabolic diseases and colorectal cancer (Diether Natalie and Willing Benjamin, 2019). Although both the contents and sources of protein have been demonstrated to change the gut microbiota and their metabolites (Lang et al., 2018), the protein contents, rather than sources, have a much greater impact (Rist et al., 2013). Long-term excessive protein intake has been shown to shift the microbiota composition, and change microbial taxa and fermentation pathways (Korpela, 2018). A low protein diet or dietary protein consumption with high digestibility could reduce the amount of protein reaching the colon, thus limiting the available protein for fermenting bacteria (Mayneris-Perxachs et al., 2016; Ma et al., 2017). However, there have been inconsistent conclusions about the influence of dietary protein levels on the intestinal microbiota. Some studies have reported that moderate dietary protein restriction could optimize the gut microbiota composition to some extent (Chen X. et al., 2018; Liu et al., 2021), while others have suggested that low protein diet decreased the gut microbiota diversity (Singh et al., 2017; Masuoka et al., 2020).
In view of the foregoing, we hypothesized that low protein diets could change the profile of intestinal microbiome and metabolome in the growing-finishing pigs. And these influences may be different in sexes. In the present study, the feeding experiment on barrows and gilts separately fed high protein diet and low protein diet was performed to test this hypothesis.
Materials and Methods
Animals and Experimental Treatments
A total of 24 barrows and 24 gilts (Duroc × Landrace × Yorkshire, 120 ± 2 days old) in the growing-finishing stage with an average initial body weight of 68.33 ± 0.881 kg were randomly assigned to one of two dietary treatments by sex (n = 12). Therefore, the following four groups in this experiment were treated: (1) BHP, barrows fed a high protein diet containing 17% CP in stage I and 15% CP in stage II; (2) GHP: gilts fed a high protein diet containing 17% CP at stage I and 15% CP at stage II; (3) BLP, barrows fed a low protein diet containing 13% CP at stage I and 11% CP at stage II; and (4) GLP, gilts fed a low protein diet containing 13% CP at stage I and 11% CP at stage II. Feed intake and body weight of every experimental animal were recorded using OSBORNE FIRE® performance testing systems Osborne Industries, Inc., Osborne, KS, United States) which include feed intake and performance testing equipment, and automated growth management systems which could perform automatic weighing by recognizing the electronic ear mark during the feed eating time. Therefore, each treatment had one pen with 12 pigs. The experimental diets were formulated based on corn-soybean meal and met the nutritional needs of growing-finishing pigs according to the National Research Council [National Research Council (NRC), 2012] (Supplementary Table 1). All pigs had ad libitum access to food and clean drinking water. The experiment lasted 51 days, and the stage I was 32 days and stage II was 19 days.
Sample Collection and Preparation
The feeding trial ended up while the average final body weight of all experimental pigs was 105.8 ± 1.228 kg. Eight pigs that were the closest to the average body weight of those in their treatment group were selected and electronically stunned and exsanguinated. The gastrointestinal tract was removed after the pigs’ abdominal cavities were opened. A colonic content sample of each pig, which has the most abundant microbiota populations in all intestinal sections and where protein fermentation is thought to mostly occur in the region (Williams et al., 2001; Wang Y. et al., 2020), was collected in 5 mL sterile frozen tubes, immediately flash-frozen in liquid nitrogen, and stored at -80°C for further analysis.
16S rRNA Amplicon Sequencing
Microbial genomic DNA from colonic content samples was extracted using the cetyltrimethylammonium bromide (CATB)/sodium dodecyl sulfate (SDS) method. The DNA concentration and purity of all DNA samples was estimated using a NanoDrop1000 spectrophotometer (Nanodrop Technologies, Wilmington, DE, United States) and 1% agarose gel electrophoresis. According to the concentration, DNA was diluted to 1 ng/μL using sterile water.
Amplicons were generated by PCR of the hypervariable region V3-V4 (341F-806R) of the bacterial 16S rRNA gene using Phusion® High-Fidelity PCR Master Mix with GC Buffer (NEB, Ipswich, MA, United States). The obtained PCR products were purified using GeneJET kit (Thermo Scientific, Waltham, MA, United States). The purified products were used to construct a DNA library using Ion Plus Fragment Library Kit 48 rxns (Thermo Scientific). Sequencing was performed on a Thermo Fisher Ion S5TMXL platform (Thermo Scientific).
Untargeted Metabolomics Analysis
Colon content samples were homogenized in prechilled methanol and 0.1% formic acid by vortexing. The homogenates were incubated on ice for 5 min and then centrifuged at 5,000 × g, at 4°C for 5 min. The supernatant samples were diluted to final concentration 60% aqueous methanol. Subsequently, the samples were transferred to Eppendorf tubes with 0.22 μm filters and were centrifuged at 5,000 × g, at 4°C for 10 min. Finally, the filtrates were injected into the liquid chromatography with tandem mass spectrometry (LC-MS-MS) system for analysis.
LC-MS/MS analyses were performed using an ultra-high performance liquid chromatography (UHPLC) system (Thermo Scientific) coupled with an Orbitrap Q Exactive HF-X mass spectrometer (Thermo Scientific). Samples were injected onto a Hyperil Gold column (100 × 2.1 mm, 1.9 μm) using a 16 min linear gradient at a flow rate of 0.2 mL/min. The eluents for the positive polarity mode were eluent A (0.1% formic acid in Water) and eluent B (methanol). The eluents for the negative polarity mode were eluent A (5 mM ammonium acetate, pH 9.0) and eluent B (methanol). The solvent gradient was set as follows: 2% B, 1.5 min; 2–100% B, 12.0 min; 100% B, 14.0 min; 100–2% B, 14.1 min; 2% B, 16 min. A Q Exactive HF-X mass spectrometer was operated in positive/negative polarity mode with spray voltage of 3.2 kV, a capillary temperature of 320 °C, a sheath gas flow rate of 35 arb, and an aux gas flow rate of 10 arb.
Data Processing
Microbiome. Raw data were processed according to the Cuadapt (Martin, 2011) quality control process. Then the reads were compared with the reference database (Quast et al., 2012) using the UCHIME algorithm (Knight, 2011) to detect chimeric sequences, which were removed (Haas et al., 2011). Finally, clean data were obtained and used for subsequent analysis. Sequence analysis was performed by Uparse software version 7.0.1001 according to a previously published method (Edgar, 2013). Sequences with ≥ 97% similarity were assigned to the same operational taxonomic units (OTUs). OTU abundance information was normalized using a standard of the sequence number corresponding to the sample with the least sequences. Analyses of alpha diversity (α-diversity) and unweighted pair-group method with arithmetic mean (UPGMA) cluster analysis were performed based on the normalized data. Five indices including, observed species, Simpson index, Shannon index, Chao1 and ACE were calculated using QIIME Version1.7.0 (Caporaso et al., 2010). The top 10 most abundant communities at the phylum and genus levels were defined as predominant bacteria and compared among different treatments.
Metabolomics. The raw data files generated by UHPLC-MS/MS were processed using the Compound Discoverer 3.0 (CD 3.0, Thermo Scientific) to perform peak alignment, peak picking, and quantitation for each metabolite. The main parameters were set as follows: retention time tolerance, 0.2 min; actual mass tolerance, 5 ppm; signal intensity tolerance, 30%; signal/noise ratio, 3; and minimum intensity, 100000. Next, the peak intensities were normalized to the total spectral intensity. The normalized data were used to predict the molecular formula based on additive ions, molecular ion peaks, and fragment ions. The peaks were then matched with the mzCloud1 and ChemSpider2 databases to obtain the accurate qualitative and relative quantitative results for principal components analysis (PCA) and partial least squares discriminant analysis (PLS-DA). Based on the differential metabolites identified by comparing GHP and GLP, Kyoto Encyclopedia of Genes and Genomes (KEGG) pathway3 analysis was conducted to investigate the metabolomics pathways affected by protein level.
Statistical Analysis
Statistical analysis was performed using the General Linear Model (GLM) procedures in SPSS V.19.0 (IBM Corp., Armonk, NY, United States) for a 2 × 2 factorial arrangement of treatments. The statistical model consisted of the fixed effects of dietary protein levels and pig sexes and their interactions. Single comparison between GHP and GLP while different abundant metabolites were analyzed was performed using Student’s t test in SPSS. The correlation between significantly changed bacteria (at the genus level) and metabolites in GHP and GLP were analyzed by Spearman’s rank correlation test using GraphPad Prim V.8.0 (GraphPad Software, San Diego, CA, United States). The results were expressed as mean and SEM. The P < 0.05 was considered significant. All the indices were analyzed with pig as the experimental unit (n = 8).
Results
DNA Sequence Coverage in Colonic Content
As shown in Supplementary Table 2, 2,668,457 V3-V4 16S rRNA valid sequences reads were obtained from 32 samples, including 78,848 (GHP), 88,485 (GLP), 85,353 (BHP), and 80,871 (BLP) raw reads. After removing chimeric sequences, 74,565, 82,306, 80,145, and 75,997 clean reads remained in the GHP, GLP, BHP, and BLP groups, respectively. The effective proportion of clean reads was 90.14–97.79%. The GC% among the clean reads was 70.16–82.57%. The results showed that there were no dramatic differences in the number of clean reads among the groups.
The Venn diagram analysis of OTUs is shown in Figure 1. There were 342 common OTUs among all groups, and 20, 40, 73, and 129 unique OTUs were identified in the GHP, GLP, BHP, and BLP groups, respectively. From the perspective of dietary crude protein levels, there were 396 common OTUs in the gilt groups, 81 unique OTUs in the GHP group, and 772 in the GLP group. Similarly, there were 1,015 common OTUs in barrow groups, and 164 unique OTUs in the BHP group, and 244 in the BLP group. These results suggested that there were fewer common OTUs in gilts than in barrows. With respect to pig sex, there were 425 common OTUs in the HP groups, with 804 unique OTUs in the BHP group, and 90 in the GHP group. Similarly, there were 1,060 common OTUs in the LP groups, with 264 unique OTUs in the BLP group, and 158 in the GLP group. The results suggested that there were fewer common OTUs in the HP groups than in the LP groups.
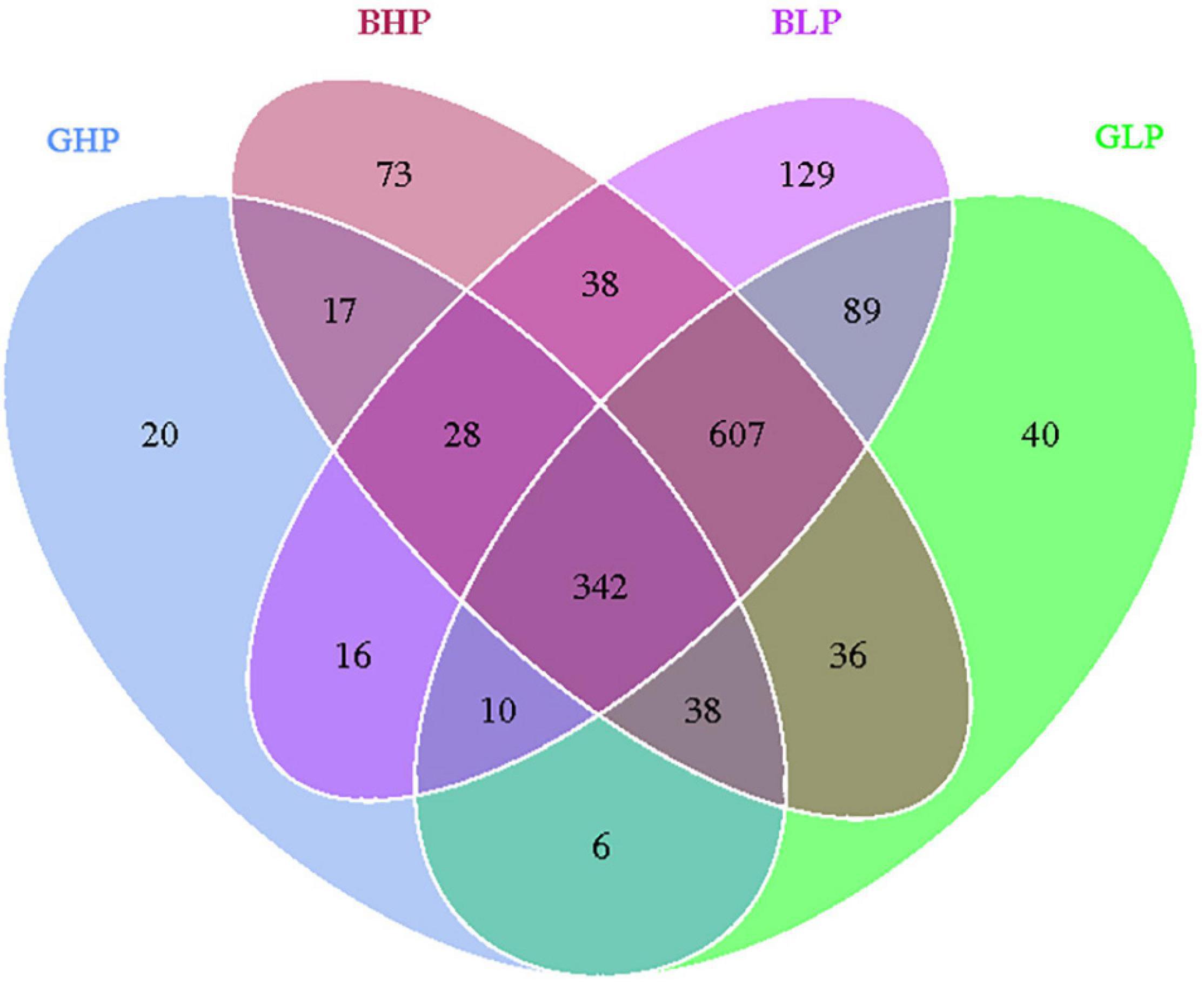
Figure 1. Venn diagram showing the unique and shared OTUs in the different groups. GHP, gilts fed high protein diet; BHP, barrows fed high protein diet; GLP, gilts fed low protein diet; BLP, barrows fed low protein diet.
Observed Species, Microbial α-Diversity, and Cluster Analysis
As shown in Table 1, the low protein diet increased the number of observed species significantly compared with high protein diet (P < 0.05). In contrast, pig sex had no significant effects (P > 0.05). However, pig sex, rather than dietary protein levels, affected the Shannon index significantly (P < 0.01) and was higher in barrows than in gilts. Neither the dietary protein levels nor sex affected the Simpson index. Sample richness indices (ACE and Chao1) were higher (P < 0.05) in the pigs fed the low protein diet. Both indices showed no remarkable differences (P > 0.05) between gilts and barrows. In terms of all the α-diversity indices, no significant interactions (P > 0.05) were found between dietary protein levels and pig sex.
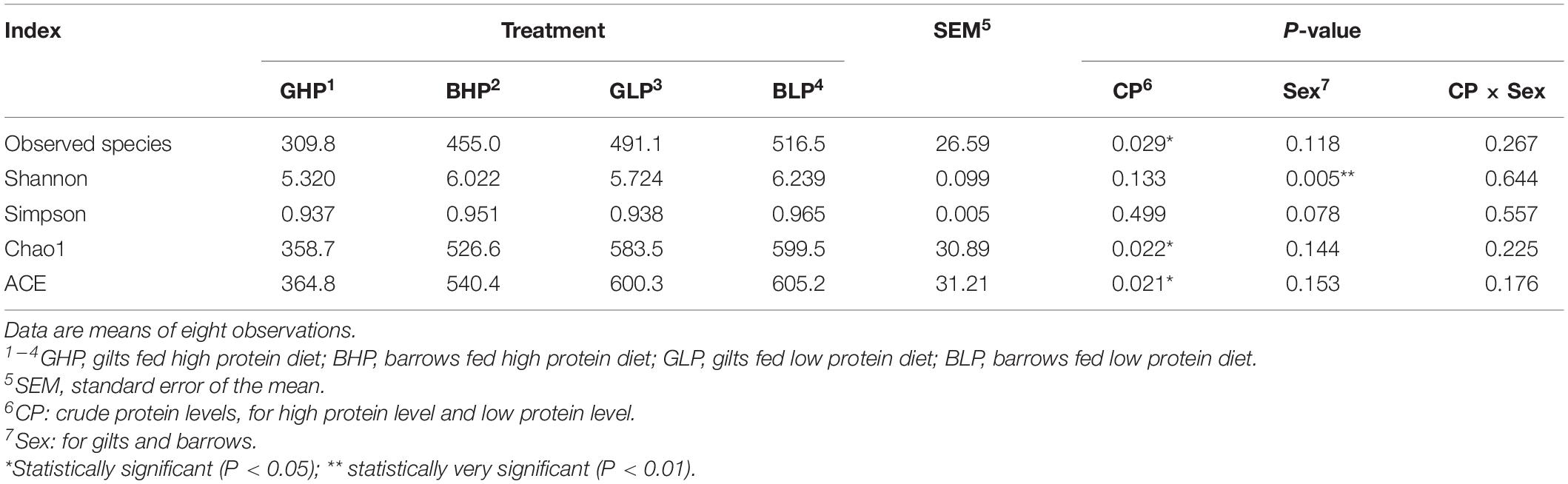
Table 1. Number of observed species, richness and Alpha diversity indices in the colon content samples from each group.
As shown in Figure 2, the UPGMA cluster of community structures at the phylum level were analyzed among the four treatments. The results showed that the GLP and BLP groups were the closest, and then they clustered together with BHP, followed by GHP, indicating that dietary protein levels had greater effects on microbial community structures than pig sex.
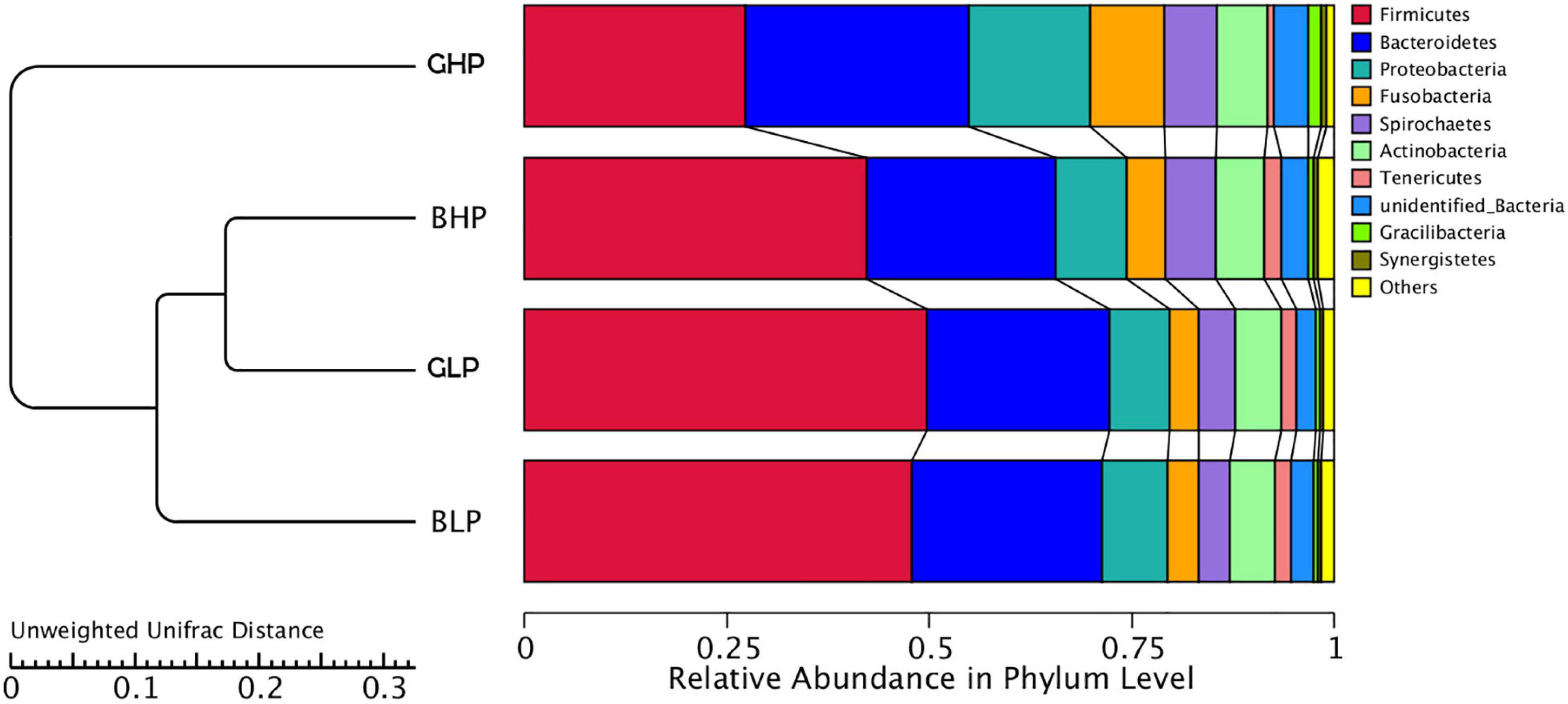
Figure 2. UPGMA cluster tree based on weighted unifrac distances of the OTU community. GHP, gilts fed high protein diet; BHP, barrows fed high protein diet; GLP, gilts fed low protein diet; BLP, barrows fed low protein diet.
Relative Abundance of the Predominant Microbial Community Induced by Dietary Protein Levels in the Colonic Content of Barrows and Gilts
The results for the relative abundance of top 10 members of the microbial community structure in the colonic contents at different levels (phylum and genus) were analyzed. At the phylum level (Supplementary Figure 1 and Table 2), reducing dietary protein level significantly increased the abundance of Actinobacteria (P < 0.05) and decreased the abundance of unidentified bacteria (P < 0.01). No remarkable differences (P > 0.05) in the abundance of any microbial community were found between gilts and barrows. For Proteobacteria, Gracilibacteria, and Synergistetes, there were significant interactions (P < 0.05) between dietary protein levels and pig sex. At the genus level (Figure 3 and Table 3), the top three microbial community, including unidentified Clostridiales (P < 0.05), Neisseria (P < 0.05), and unidentified Prevotellaceae (P = 0.00) were significantly affected by dietary protein levels. The abundance of unidentified Prevotellaceae was also different (P < 0.01) between gilts and barrows. Dietary protein levels and pig sex showed significant interactions in Neisseria (P < 0.05) and unidentified Prevotellaceae (P = 0.00). In addition, the abundances of Gracilibacteria (P < 0.05) and unidentified bacteria (P = 0.00) were significantly affected by dietary protein levels, and showed significant interactions (P < 0.05) between dietary protein levels and pig sex.
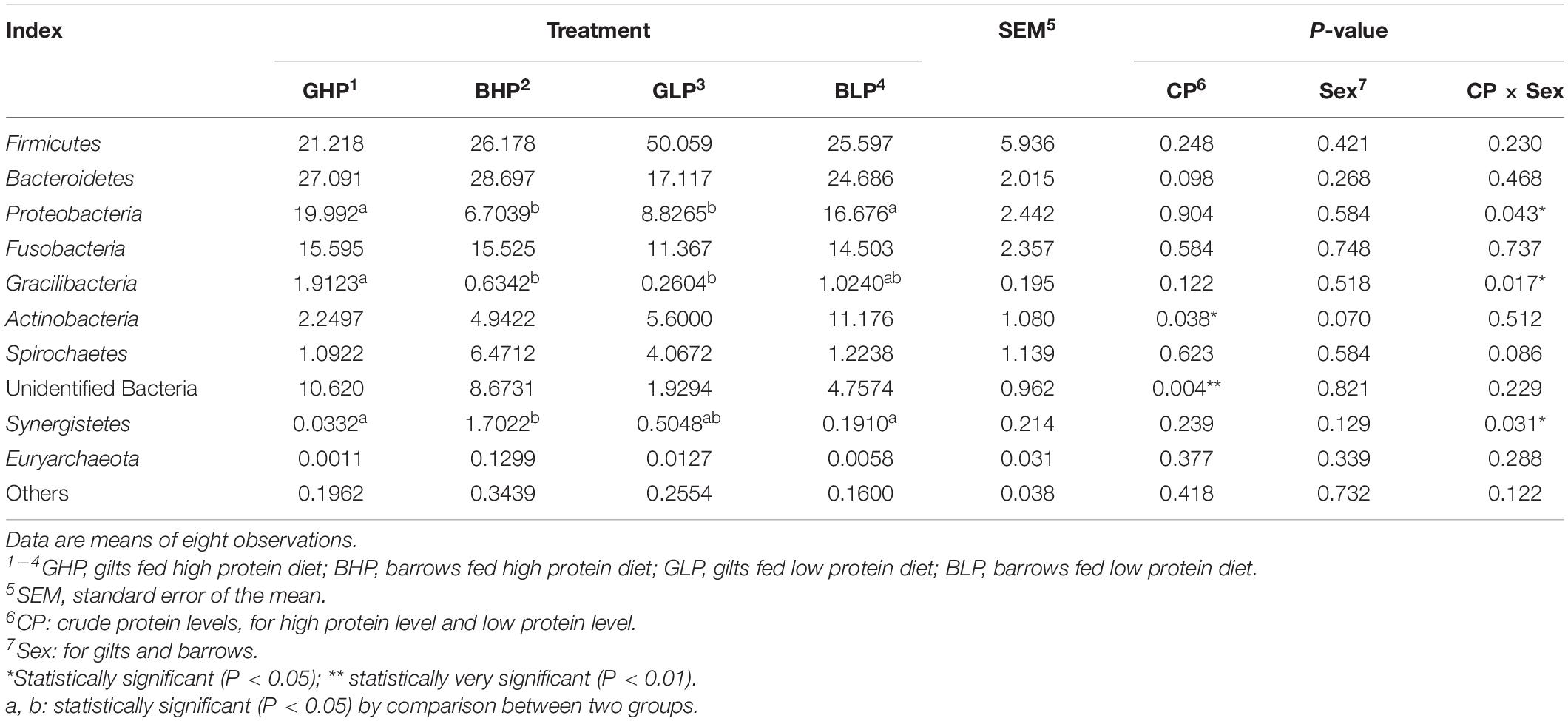
Table 2. Relative abundance of microbial community (Top 10, %) structure in colonic content at phylum level from each group.
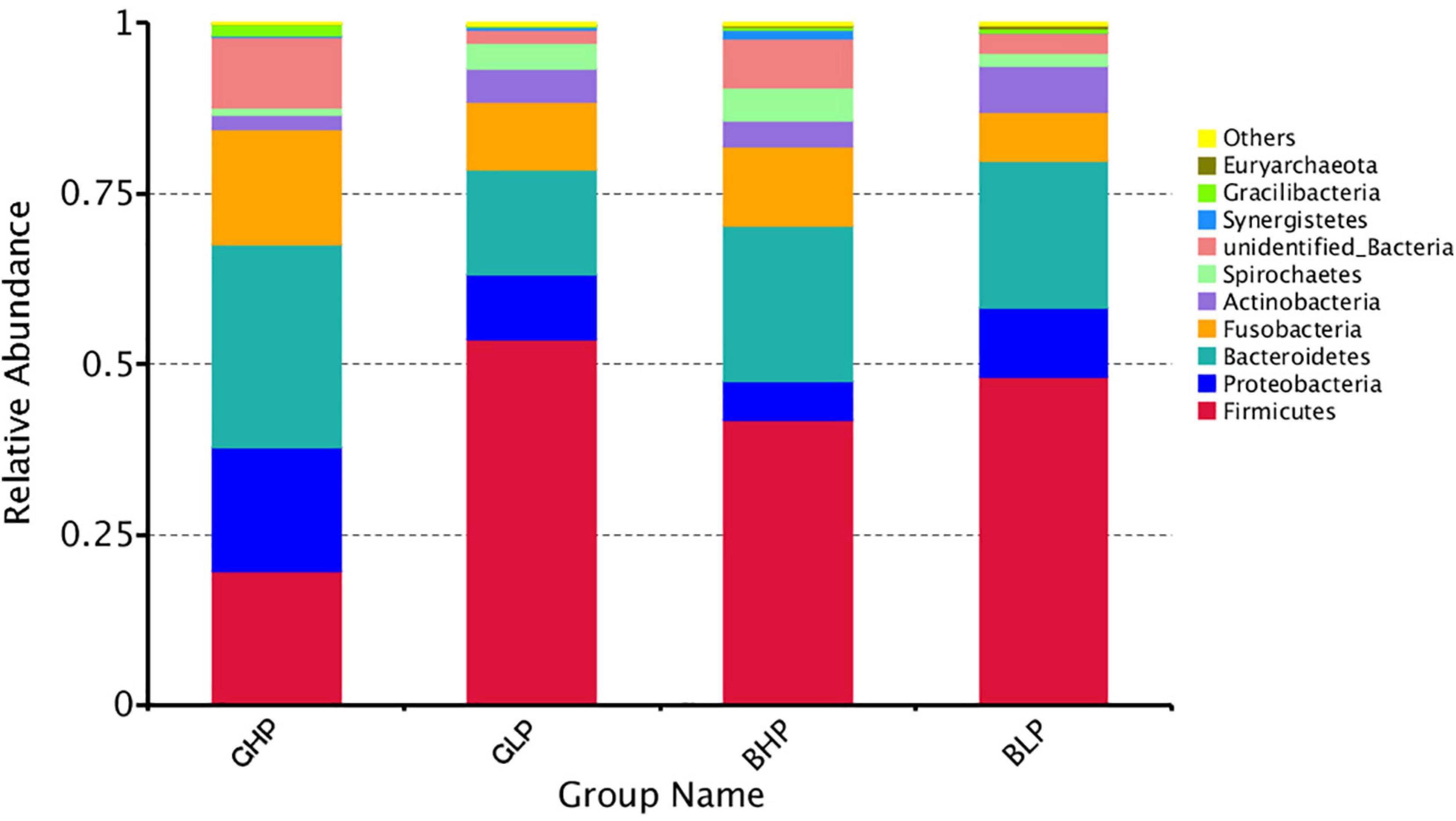
Figure 3. Relative abundance of microbial community (Top 10) structure in the colonic content at Genus level. GHP, gilts fed high protein diet; BHP, barrows fed high protein diet; GLP, gilts fed low protein diet; BLP, barrows fed low protein diet.
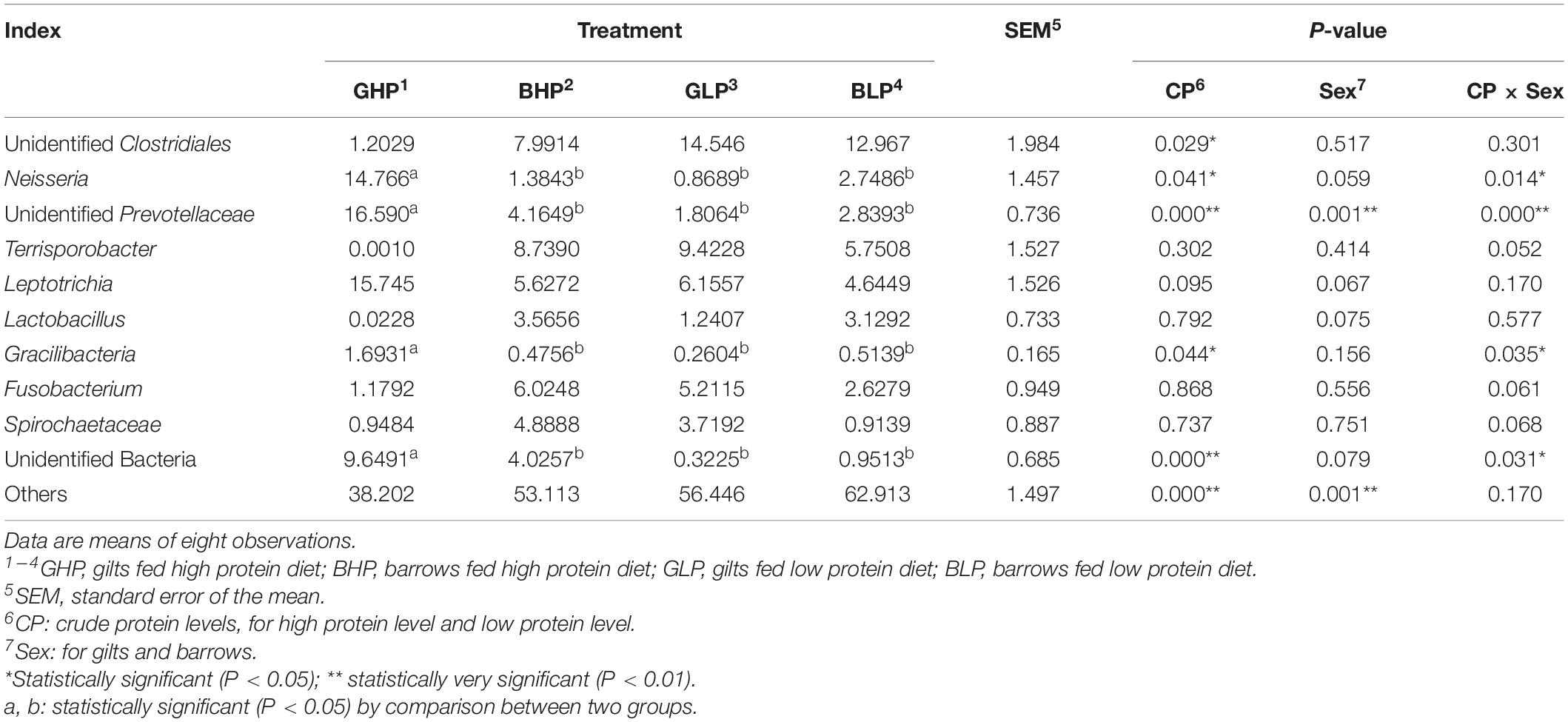
Table 3. Relative abundance of microbial community (Top 10, %) structure in colonic content at genus level from each group.
Metabolome Profiles and PCA of the Main Metabolites in the Colonic Content of Barrows and Gilts Induced by Dietary Protein Levels
To reveal the effects of dietary protein levels and pig sex on intestinal metabolic profiles, LC-MS was used to analyze the metabolome of the colonic content. As shown in Supplementary Table 3, the score plot of LC-MS [electrospray ionization negative (ESI-)] data with 2,037 metabolite signals and LC-MS (ESI+) data with 3,844 metabolite signals were detected.
From the perspective of dietary protein levels [Supplementary Figure 2A(a–d)], the PCA results showed that dietary protein levels had a robust influence on main metabolites of pigs, especially between GHP and GLP groups, in which the metabolic communities were clustered. From the perspective of pig sex [Supplementary Figure 2B(a–d)], the PCA results showed that the main metabolites between the two pairs of BHP and GHP, and BLP and GLP groups were mixed together. Especially for barrows, there was a marked variation among samples even in the same group. In contrast, samples of gilts were more gathered. The PLS-DA score plots (Figures 4A,B) also showed that the GHP and GLP groups were well-separated, suggesting that dietary protein levels caused more significant biochemical changes in gilts compared with that in barrows. These results suggested that main metabolites between gilts and barrows and within barrows fed different dietary protein levels had no significant differences. Therefore, in this study subsequent analysis on microbial different metabolites-related results mainly focused on the experimental gilts fed the HP and LP diets.
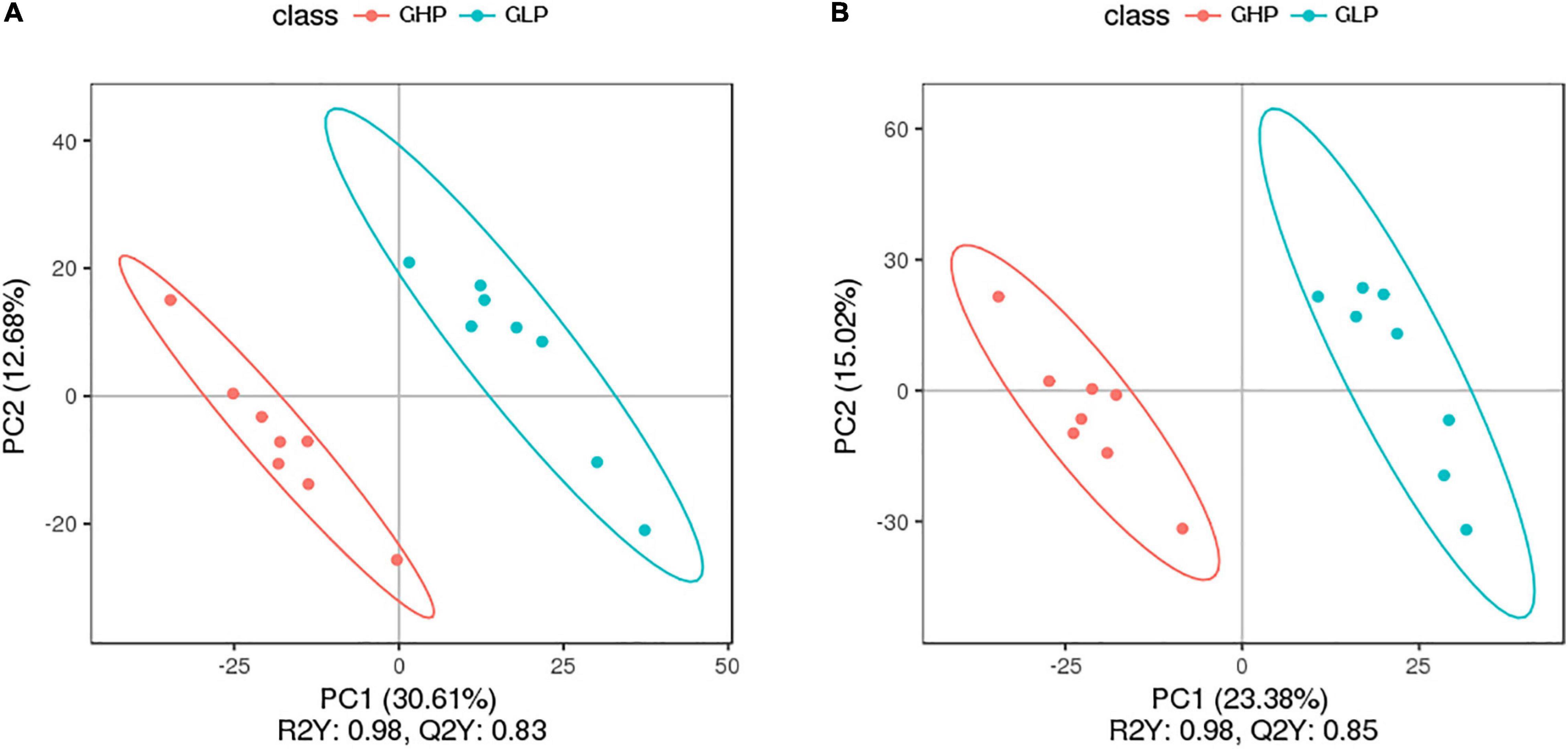
Figure 4. PLS-DA score plot of colonic metabolomic data from gilts fed low and high protein diets. (A) LC-MS (ESI-); (B) LC-MS (ESI+). GHP, gilts fed high protein diet;GLP, gilts fed low protein diet.
Identification and KEGG Analysis of Differently Abundant Metabolites in Colonic Content of Gilts Fed the High Protein and Low Protein Diets
Furthermore, the parameters of variable importance of projection (VIP) > 1.0 and adjusted q < 0.05 were used to detect differentially abundant metabolites in response to different dietary protein levels in gilts. As shown in Figures 5A,B and Supplementary Table 4, compared with those in the GHP group, a total of 156 differentially abundant metabolites in LC-MS (ESI-) were identified in the GLP group, including 32 increased and 124 decreased abundant metabolites. Similarly, 278 metabolites in LC-MS (ESI+) were identified, including 126 increased and 152 decreased abundant metabolites. These results suggested that the low protein diet induced more numbers of metabolites’ abundances decrease and less numbers of metabolites’ abundances increase in all differentially abundant metabolites detected.
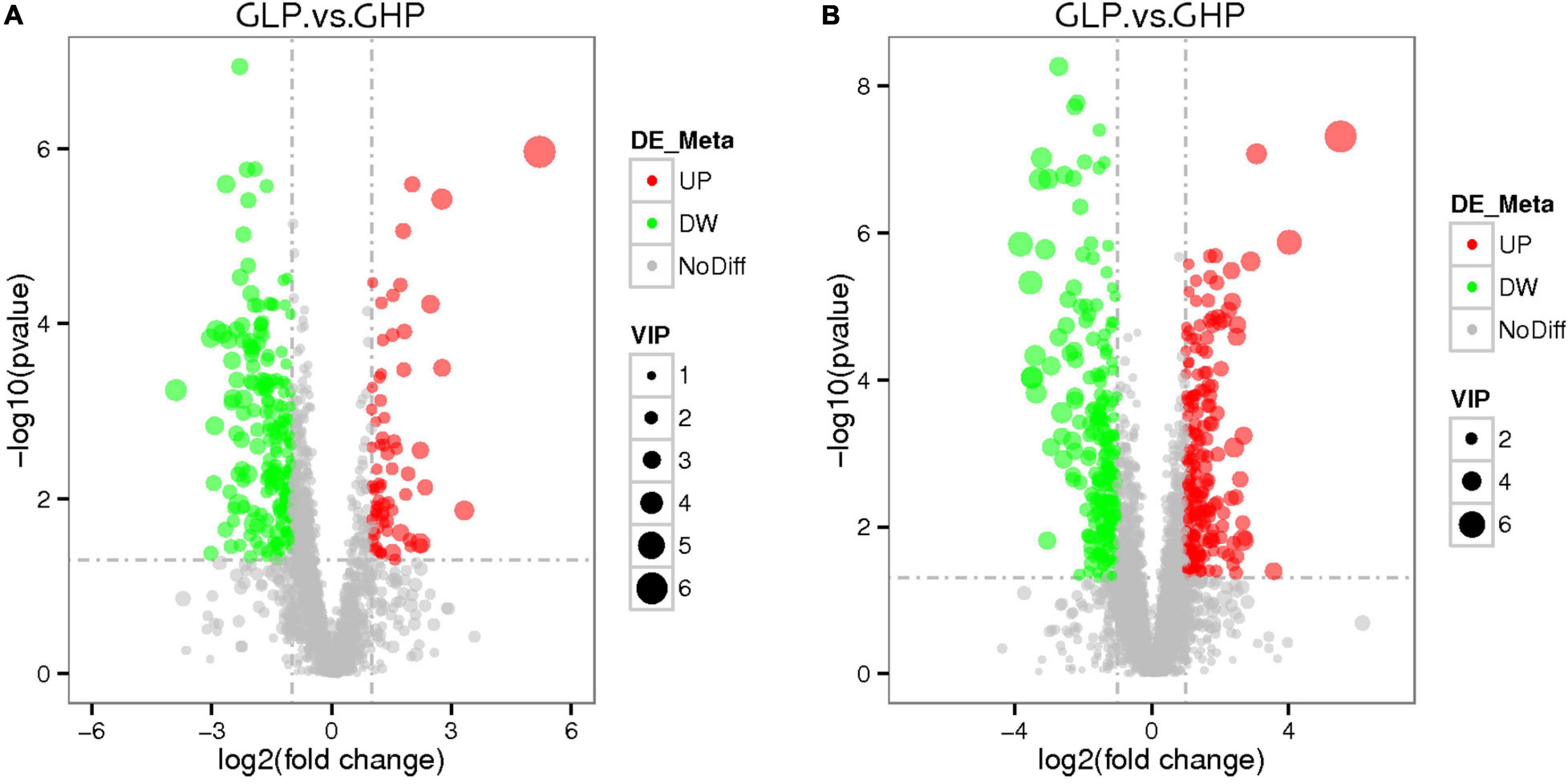
Figure 5. Score volcano plots of colonic metabolomic data from gilts fed low and high protein diets. (A) LC-MS (ESI-); (B) LC-MS (ESI+). GHP, gilts fed high protein diet; GLP, gilts fed low protein diet.
The KEGG was used to analyze the pathways of the differentially abundant metabolites between the two gilt groups. As shown in Figures 6A,B and Supplementary Table 5, the metabolic pathways of the Phosphotransferase system (PTS), Ascorbate and aldarate metabolism, the HIF-1 signaling pathway, and Asthma and Glutathione metabolism were associated with four metabolites in LC-MS (ESI-) and were significantly affected by dietary protein levels. Inflammatory mediator regulation of TRP channels, the Fc epsilon RI signaling pathway, Linoleic acid metabolism, Degradation of aromatic compounds, and Biosynthesis of alkaloids derived from histidine and purine were associated with nine metabolites in LC-MS (ESI+) and were significantly affected by dietary protein levels. Interestingly, the metabolite vitamin C (also named ascorbic acid), was enriched and regulated the pathways of PTS, Ascorbate and aldarate metabolism, the HIF-1 signaling, and Glutathione metabolism.
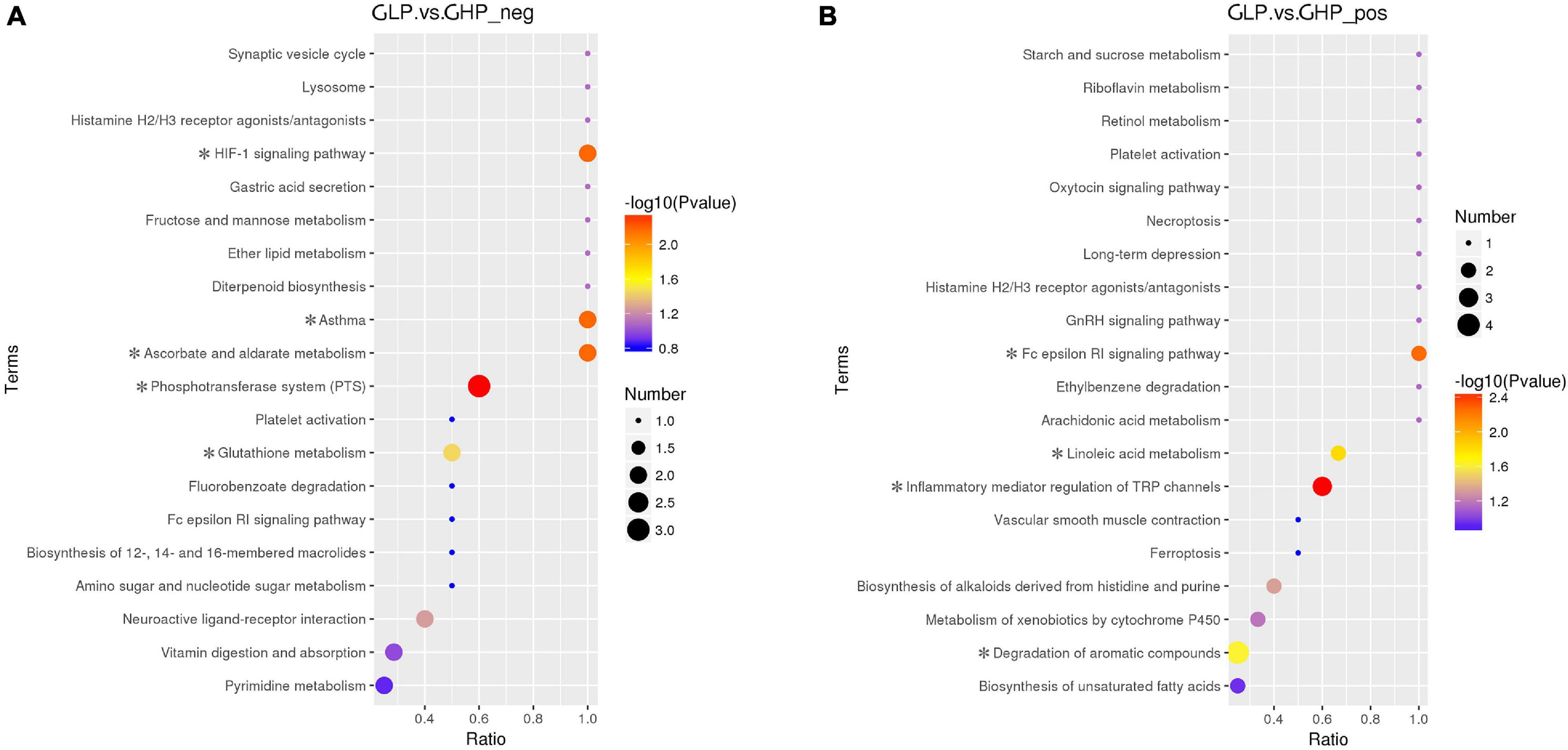
Figure 6. KEGG pathway enrichment of the differentially abundant metabolites between the two gilt groups. ∗P < 0.05, (A) LC-MS (ESI-); (B) LC-MS (ESI+). GHP, gilts fed high protein diet; GLP, gilts fed low protein diet.
Correlation Between the Predominant Microbial Community and Differentially Abundant Metabolites in the Colonic Content of Gilts Induced by Dietary Protein Levels
There were six genus-level microbial communities whose proportions showed significant differences in response to dietary protein levels (Supplementary Table 6). Compared with the GHP group, the proportions of unidentified Clostridiales (P < 0.05) and Terrisporobacter (P < 0.01) were significantly increased in GLP and the proportions of the remaining communities, Neisseria (P < 0.05), unidentified Prevotellaceae (P = 0.00), Gracilibacteria (P < 0.05), and unidentified bacteria (P < 0.01), were decreased.
To further reveal the crosstalk between the microbiota and the host, the six communities were selected and used to analyze the correlation with 12 changed metabolites that were enriched in above KEGG analysis. As shown in Figure 7, the proportion of unidentified Clostridiales was associated positively with the levels of the Platelet-activating factor (P < 0.05), Cinnamaldehyde (P < 0.01), Carbazole (P < 0.01), and Arachidonic acid (P < 0.01). Neisseria was associated positively with Vitamin C (P < 0.01), Histamine (P < 0.01), Naphthalene (P < 0.01), and Acetophenone (P < 0.01), and negatively with Cinnamaldehyde (P < 0.05) and 3-Phenylpropanoic acid (P < 0.05). Unidentified Prevotellaceae was associated positively with Vitamin C (P < 0.05), D-Mannose 6-phosphate (P < 0.05), Dihomo-gamma-linolenic acid (P < 0.05), Naphthalene (P < 0.05) and Dolichotheline (P < 0.05), but negatively with Platelet-activating factor (P < 0.01), Cinnamaldehyde (P < 0.01), 3-Phenylpropanoic acid (P < 0.01), Carbazole (P < 0.01), and Arachidonic acid (P < 0.01). The proportion of Terrisporobacter was associated positively with Platelet-activating factor (P < 0.01), Cinnamaldehyde (P < 0.01), Carbazole (P < 0.01), and Arachidonic acid (P < 0.01), but negatively with Vitamin C (P < 0.05) and Histamine (P < 0.01). Gracilibacteria were associated positively with Vitamin C (P < 0.01), Histamine (P < 0.01), Dihomo-gamma-linolenic acid (P < 0.01), Naphthalene (P < 0.01), and Acetophenone (P < 0.01), but negatively with Cinnamaldehyde (P < 0.05) and 3-Phenylpropanoic acid (P < 0.01). Unidentified bacteria were associated positively with Histamine (P < 0.01) and Naphthalene (P < 0.05) but negatively with Platelet-activating factor (P < 0.05), Cinnamaldehyde (P < 0.01), 3-Phenylpropanoic acid (P < 0.01), Carbazole (P < 0.01), Arachidonic acid (P < 0.01), and Dihomo-gamma-linolenic acid (P < 0.01).
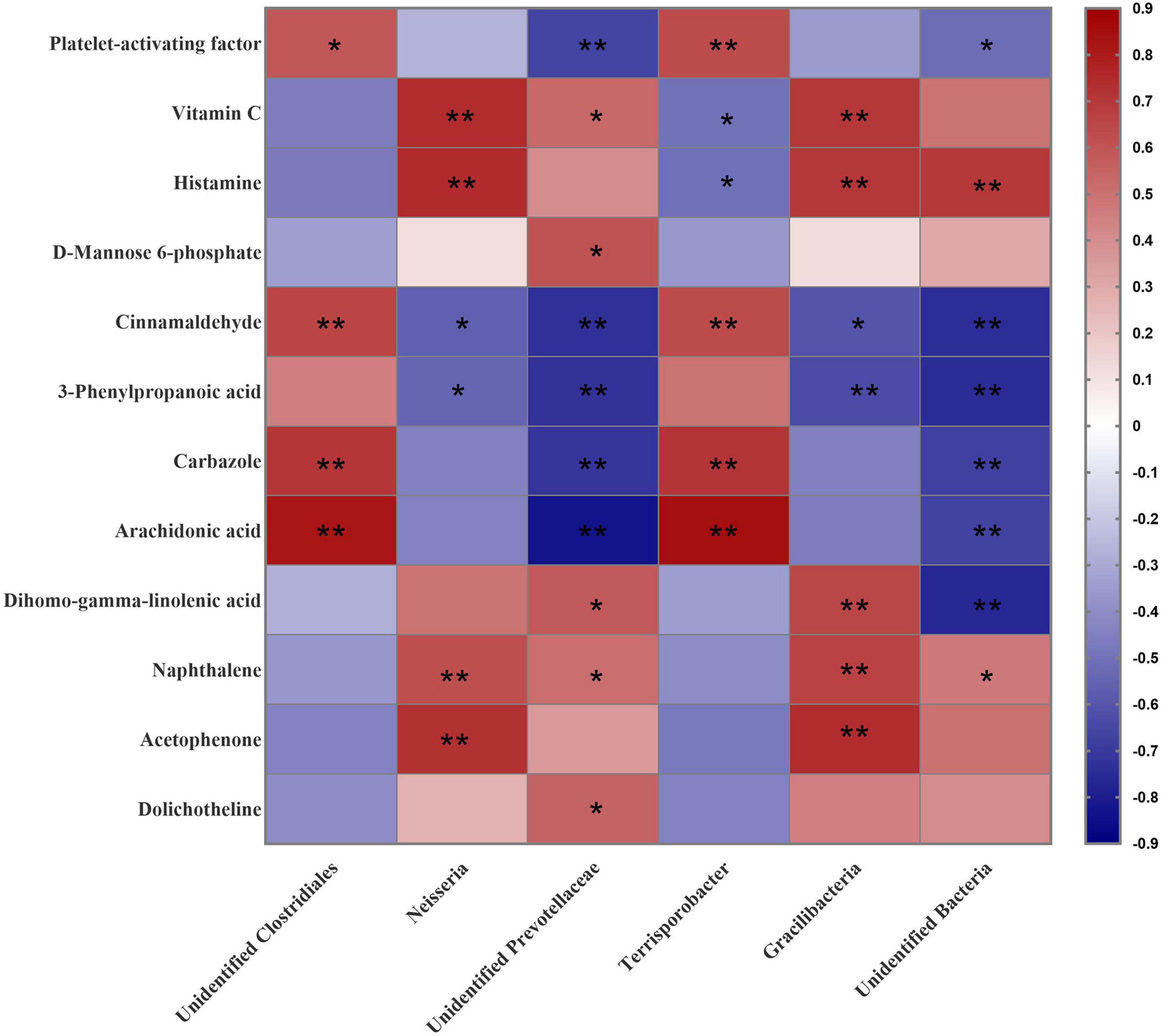
Figure 7. Correlation analysis between the predominant microbial community at genus level (relative abundance) and differentially abundant metabolites in the colonic content of gilts induced by different dietary protein levels. *P < 0.05; **P < 0.01.
Discussion
Dietary protein intake is essential for the health and growth of humans and animals. However, inappropriate amino acid composition and protein content consumption, including both excess and deficiency, has adverse effects on the body, especially the intestinal microbiota, which is very sensible to subtle environmental changes. Previous studies reported that dietary protein levels had no significant effects on microbiota diversity; they did alter microbial composition in the hindgut of growing-finishing pigs (Zhou et al., 2016; Fan et al., 2017; Chen J. et al., 2018; González-Prendes et al., 2019; Zhao et al., 2020). However, our results revealed that the low protein diet not only changed the microbiota composition, but also increased microbial species and improved microbial diversity and richness in growing-finishing pigs. These inconsistent results might be related to the dietary protein content used and the reduction levels. The gut microbiota was also demonstrated to be affected by sampling different intestinal segments. Dietary protein levels changed the ileal microbiota diversity rather than that in feces in weaned piglets (Pollock et al., 2019). Moderate lowering of the dietary protein concentration also improved the α-diversity of that intestinal microbial community in growing pigs (Peng et al., 2017; Chen X. et al., 2018). However, an excessive protein decrease, i.e., a very low protein intake, was detrimental to the microbiota structure (Shi et al., 2019; Spring et al., 2020). Therefore, changes in microbiota upon dietary protein in pigs are dose- and site-dependent (Zhang et al., 2020). Our results demonstrated that the dietary protein content could affect the intestinal microbiota diversity in pigs at the growing-finishing stage, and suggested that the low protein diet formulated in the present study was appropriate and helpful to increase intestinal microbial diversity and species in growing-finishing pigs.
In terms of intestinal microbiota composition, our results demonstrated the four main phyla Firmicutes, Bacteroidetes, Proteobacteria, and Fusobacteria in all groups accounted for over 77% of all bacteria phyla. The former three phyla were also demonstrated to be the most abundant bacterial communities in the feces of piglets (Shili et al., 2020). We found the proportion of Actinobacteria increased when the dietary protein level was lower. Although the role of Actinobacteria in the intestine is unclear, which are considered probiotics and could produce biological active substances with anti-inflammatory and antibacterial properties (Demain and Sanchez, 2009). Our results revealed that the low protein diet mainly increased the proportion of Clostridiales, and decreased Prevotellaceae, Neisseria and Gracilibacteria. Similarly, the abundances of Clostridiales were decreased by feeding high protein diets in the large intestinal content of rats and in the feces of dogs (Hang et al., 2012; Liu et al., 2015). However, this was contrary to the previous results (Pieper et al., 2012; Luo et al., 2015) which showed that a low protein diet decreased the relative abundance of Clostridium in weaned piglets. The difference was attributed to the Clostridiales, which were detected in growing-finishing pigs and piglets belong to different genera, suggesting they probably played different roles. Interestingly, a previous study found that the proportion of Prevotella in fecal samples was lower in piglets fed with a low protein diet than in those fed a high protein diet, but was higher in the ileal digesta (Kaewtapee et al., 2017). The inconsistence on the microbiota from different intestinal sampling sections was also demonstrated by Rist et al. (2014). This variation might reflect the specific functions of bacteria in different intestinal segments. Therefore, the roles of the differentially abundant bacteria in the gut should be investigated in further studies.
Although dietary protein levels had greater influence than pig sex, our results revealed there were also the differences of microbiota diversity and communities between barrows and gilts. More interestingly, the two factors showed obvious interactions in microbial community structure. The interactions resulted in the influences of dietary protein levels or pig sex being compromised or more robust, or even offset or reversed. Specifically, at the phylum level the relative abundances of both Proteobacteria and Gracilibacteria were lower in the GLP group than in the GHP group, whereas Proteobacteria was higher in the BLP group than in the BHP group. Gracilibacteria showed no significant difference between barrow groups. By contrast, Synergistetes showed no significant difference between gilt groups, but was lower in the BLP group than in the BHP group. At the genus level, the relative abundances of Neisseria, Unidentified Prevotellaceae, Gracilibateria, and unidentified bacteria were higher in the GLP group than in the GHP group and higher in the GHP group than in the BHP group, but all four communities showed no significant differences between the BLP and BHP groups, or between the GLP and BLP groups. These interactions also suggested that the low protein diet had a greater influence on intestinal microbial communities in gilts than in barrows. Thus, the present study revealed the effects of combing two factors of pig sex and dietary protein content on the intestinal microbiota, which indicated new feeding strategies could be developed, such as designing different dietary protein concentrations according to pig sex to ensuring intestinal microbiota health. In addition, our results suggested that it is necessary to use single sex animals for studying intestinal microbiota to remove sex-related inherent differences.
Dietary interventions had major influences on the metabolic composition and abundance of the intestinal microbiome (Mitchell et al., 2020). Previous studies demonstrated the roles of dietary starch, fat/fiber (Heinritz et al., 2016; Yu M. et al., 2019) and fermented feed (Lu et al., 2019) in growing-finishing pigs. Low protein diet was speculated to increase intestinal metabolic activity by the functional prediction on gut microbial flora of pigs fed different protein content diets because genes in intestinal microorganisms at different protein levels are mainly enriched in the “metabolism pathway” (Wang D. et al., 2020). Differences of gut microbiota and its metabolites in piglets induced by protein restriction were demonstrated, and low protein diet was confirmed to decrease mainly the concentrations of biogenic amines, phenolic and indole compounds, and acetate and total SCFA (Yu et al., 2017; Hou et al., 2021). Similarly, we also found an abundant decrease of histamine, phenolic and acetate compounds, etc., in growing-finishing pigs fed low protein diet. More significantly, in the present study PCA and PLS-DA analysis demonstrated that colonic metabolites were influenced by both dietary protein levels and pig sex, in which the GHP and GLP groups had a clear separation compared with the other pairs of groups. These results indicated a low protein diet specifically shifted the metabolic profile in gilts. Interestingly, an increase in protein consumption for 10 weeks had no influences on the fecal microbiota and volatile metabolites in healthy older men (Mitchell et al., 2020). Notably, in the above study, all the participants were men rather than women. In contrast, another study on female pigs rather than male also proved that sanitary conditions affected the colonic microbiome and metabolome (te Pas et al., 2020). The differences of metabolic profile between pig sexes may largely contribute to the sex-related microbial compositions by sex hormones which had been confirmed to modulate gut microbiota (Razavi et al., 2019). Sex steroid levels have been demonstrated to be correlated with intestinal microbiota composition and diversity (Kaliannan et al., 2018; Shin et al., 2019). The possible mechanisms of sex steroids on gut micorbiota could induce the permeability of intestinal mucosal integrity (van der Giessen et al., 2019), bind to sex hormone receptors in gut (Menon et al., 2013), and regulate bile acid levels (Org et al., 2016) and immune response (Fransen et al., 2017). The present study was the first time to reveal the female-specific influences on intestinal microbial metabolome in swine.
In recent years, two types of protein metabolites produced by intestinal microbes, biogenic amines and short chain fatty acids (SCFAs), which are generated by deamination and decarboxylation, respectively, have been studied (Fan et al., 2015). However, the present study found the SCFA levels were not different between the GHP and GLP groups. In fact, previous studies demonstrated that the main SCFAs, such as acetate, propionate, and butyrate, are generated in the hindgut from carbohydrates fermentation rather than proteins. Branched chain fatty acids also belong to the SCFAs, which account for about 5–10% of total SCFAs, are produced by the microbiota exclusively from branched chain amino acids (BCAAs) (Blachier et al., 2007; Ma et al., 2010). However, our results showed the abundances of BCAAs had no significant differences between dietary protein content groups. This may be due to metabolites detected using untargeted analysis and a slight difference of BCAAs’ content between two diets.
The growth performance of the pigs in this study has been reported (Shang et al., 2020). Generally, dietary protein levels had no significant influence (P > 0.05) on the average daily gain (ADG) and average daily feed intake (ADFI), but low protein diet improved (P < 0.05) the ratio of feed and gain (F/G). In contrast, pig sex had a more obvious difference in the growth performance. Barrows showed a greater ADG (P = 0.00) and ADFI (P = 0.00), but no difference (P > 0.05) in F/G. These results indicated a moderate decrease of dietary protein concentration could benefit for improving feed efficiency. And this may due to the different response of the intestinal microbiota and metabolites of pigs fed different protein levels. Therefore, in this study we firstly investigated the relationship of intestinal microbiota and metabolites in barrows and gilts. Pearson’s correlation analysis showed that the relative abundances of bacteria at the genus level were closely associated with the concentrations of 12 specific metabolites. Among these metabolites, histamine showed the largest decrease. Dietary histidine was considered to involve in protein-restricted response in low protein diet-fed pigs and affected gut microbiota (Kang et al., 2020). The polyamine histamine is a well-known pro-inflammatory factor (Smuda and Bryce, 2011). Consistently, KEGG analysis also found that several inflammation-related pathways were enriched. These results suggested that the intestinal Neisseria and Gracilibacteria, which are histamine-producing bacteria, might induce inflammation by the increase of histamine concentration in female pigs fed with the higher protein diet. More evidence directly related to inflammation is needed to support the speculation in future study. In addition, an abundant decrease of other biogenic amines including tryptamine, putrescine, and cadaverine was confirmed in colonic content of adult pigs fed a low protein diet (Yu D. et al., 2019). The present study also found that the dietary protein content regulated the proportions of certain special bacteria and their metabolites, such as fatty acids, vitamin C, and platelet-activating factor, which might have subsequent influences on immune, diseases and lipid related metabolism processes. Further research examining the potential roles of these different metabolites may be more significant to our understanding the function of microbiota induced by dietary protein change and its interaction with host health.
Conclusion
Combined intestinal microbiome and metabolome analysis demonstrated that the dietary protein content selectively altered the gut microbial diversity, composition, and metabolic profiles in the colon of growing-finishing pigs. Decreasing dietary protein level with the supplement of essential amino acids improved feed efficiency of experimental pigs, increased the intestinal microbial species and diversity. The influences of dietary protein on the microbiota composition were significantly different between barrows and gilts. In particular, the concentrations of intestinal metabolites in gilts, including histamine, fatty acids, vitamin C, and platelet-activating factor, which involve amino acids, immune, and inflammation related metabolism, were altered by changing the dietary protein levels. These results suggested that decreasing dietary protein contents changed the intestinal microbiota in growing-finishing pigs, which selectively affected the intestinal metabolite profiles in gilts.
Data Availability Statement
The datasets presented in this study can be found in online repositories. The names of the repository/repositories and accession number(s) can be found below: https://www.ncbi.nlm.nih.gov/, PRJNA738339.
Author Contributions
ZX was the Principal Investigator of the relevant project (2021C02007). XT designed the study, in charge of this study and was the Principal Investigator of the relevant project (2018C02035), and wrote the manuscript. BD designed this study together and joined in part of this study. QY performed animal feeding experiment. XM and JW joined in part of this study. All authors were variously involved in completion of this manuscript.
Funding
This study was supported by the Science Technology Department of Zhejiang Province (2021C02007 and 2018C02035). The funders had no role in study collection, data collection and analysis, decision to publish, or preparation of the manuscript.
Conflict of Interest
The authors declare that the research was conducted in the absence of any commercial or financial relationships that could be construed as a potential conflict of interest.
Publisher’s Note
All claims expressed in this article are solely those of the authors and do not necessarily represent those of their affiliated organizations, or those of the publisher, the editors and the reviewers. Any product that may be evaluated in this article, or claim that may be made by its manufacturer, is not guaranteed or endorsed by the publisher.
Acknowledgments
We would like to thank H. J. Jiang, J. H. LV, and H. B. Guo for their help. We would also like to thank the native English speaking scientists of Elixigen Company (Huntington Beach, California) for editing our manuscript.
Supplementary Material
The Supplementary Material for this article can be found online at: https://www.frontiersin.org/articles/10.3389/fmicb.2021.717727/full#supplementary-material
Supplementary Figure 1 | Relative abundance of microbial community (Top 10) structure in the colonic content at Phylum level.
Supplementary Figure 2 | PCA score plot of colonic metabolomic data for barrows and gilts fed low and high protein diets. (A) Effects of dietary protein levels (a), BHP-BLP (ESI-); (b), BHP-BLP (ESI-); (c), GHP-GLP (ESI-); (d), GHP-GLP (ESI-); (B) Effects of pig sex (a) BHP-GHP (ESI-); (b) BHP-GHP (ESI-); (c) BLP-GLP (ESI-); (d) BLP-GLP (ESI-).
Supplementary Table 1 | The compositions and nutrition levels of the experimental diets (Dry matter basis, %).
Supplementary Table 2 | Raw reads and selected effective sequences in each sample.
Supplementary Table 3 | All metabolites detected for barrows and gilts fed low and high protein diets.
Supplementary Table 4 | Differentially abundant metabolites between GHP and GLP.
Supplementary Table 5 | All KEGG pathways enriched between GHP and GLP.
Supplementary Table 6 | Comparison of the relative abundance (Top 10, %) of microbial community structure in colonic content at genus level between GHP and GLP.
Footnotes
References
Ananthakrishnan, A. N. (2015). Epidemiology and risk factors for IBD. Nat. Rev. Gastroenterol. Hepatol. 12, 205–217.
Arpaia, N., and Rudensky, A. Y. (2014). Microbial metabolites control gut inflammatory responses. Proc Natl Acad Sci. 111, 2058.
Blachier, F., Mariotti, F., Huneau, J. F., and Tome, D. (2007). Effects of amino acid-derived luminal metabolites on the colonic epithelium and physiopathological consequences. Amino Acids. 33, 547–562. doi: 10.1007/s00726-006-0477-9
Caporaso, J., Kuczynski, J., Stombaugh, J., Bittinger, K., Bushman, F., and Costello, E. (2010). QIIME allows analysis of high-throughput community sequencing data. Nat. Methods 7, 335–336.
Chen, J., Kang, B., Jiang, Q., Han, M., Zhao, Y., Long, L., et al. (2018). Alpha-ketoglutarate in low-protein diets for growing pigs: effects on cecal microbial communities and parameters of microbial metabolism. Front. Microbiol. 9:1057. doi: 10.3389/fmicb.2018.01057
Chen, X., Song, P., Fan, P., He, T., Jacobs, D., Levesque, C. L., et al. (2018). Moderate dietary protein restriction optimized gut microbiota and mucosal barrier in growing pig model. Front. Cell Infect. Microbiol. 8:246. doi: 10.3389/fcimb.2018.00246
Collins, S. M., Surette, M., and Bercik, P. (2012). The interplay between the intestinal microbiota and the brain. Nat. Rev. Microbiol. 10, 735–742.
de la Cuesta-Zuluaga, J., Kelley, S. T., Chen, Y., Escobar, J. S., Mueller, N. T., Ley, R. E., et al. (2019). Age- and sex-dependent patterns of gut microbial diversity in human adults. mSystems 4:e00261-19. doi: 10.1128/mSystems.00261-19
Demain, A. L., and Sanchez, S. (2009). Microbial drug discovery: 80 years of progress. J. Antibiot. 62, 5–16.
Diether Natalie, E., and Willing Benjamin, P. (2019). Microbial fermentation of dietary protein: an important factor in diet–microbe–host interaction. Microorganisms 7:19. doi: 10.3390/microorganisms7010019
Edgar, R. C. (2013). UPARSE: highly accurate OTU sequences from microbial amplicon reads. Nat. Methods 10, 996–998. doi: 10.1038/nmeth.2604
Fan, P., Li, L., Rezaei, A., Eslamfam, S., and Ma, X. (2015). Metabolites of dietary protein and peptides by intestinal microbes and their impacts on gut. Curr. Protein. Pept. Sci. 16, 646–654.
Fan, P., Liu, P., Song, P., Chen, X., and Ma, X. (2017). Moderate dietary protein restriction alters the composition of gut microbiota and improves ileal barrier function in adult pig model. Sci. Rep. 7:434. doi: 10.1038/srep43412
Fransen, F., van Beek, A. A., Borghuis, T., Meijer, B., Hugenholtz, F., van der Gaast-de Jongh, C., et al. (2017). The impact of gut microbiota on gender-specific differences in immunity. Front. Immunol. 8:754. doi: 10.3389/fimmu.2017.00754
Gi Langille, M., Meehan, C. J., Koenig, J. E., Dhanani, A. S., Rose, R. A., Howlett, S. E., et al. (2014). Microbial shifts in the aging mouse gut. Microbiome 2:50.
Glowacki, R. W. P., and Martens, E. C. (2020). In sickness and health: Effects of gut microbial metabolites on human physiology. PLoS Pathog. 16:e1008370. doi: 10.1371/journal.ppat.1008370
González-Prendes, R., Pena, R. N., Solé, E., Seradj, A. R., and Ramayo-Caldas, Y. (2019). Modulatory effect of protein and carotene dietary levels on pig gut microbiota. Sci. Rep. 9:14582. doi: 10.1038/s41598-019-51136-6
Haas, B. J., Gevers, D., Earl, A. M., Feldgarden, M., Ward, D. V., Giannoukos, G., et al. (2011). Chimeric 16S rRNA sequence formation and detection in sanger and 454-pyrosequenced PCR amplicons. Genome Res. 21, 494–504. doi: 10.1101/gr.112730.110
Hang, I., Rinttila, T., Zentek, J., Kettunen, A., Alaja, S., Apajalahti, J., et al. (2012). Effect of high contents of dietary animal-derived protein or carbohydrates on canine faecal microbiota. BMC Vet. Res. 8:90.
Heinritz, S. N., Weiss, E., Eklund, M., Aumiller, T., Louis, S., Rings, A., et al. (2016). Intestinal microbiota and microbial metabolites are changed in a pig model fed a high-fat/low-fiber or a low-fat/high-fiber diet. PLoS One. 11:e0154329.
Hou, L., Wang, L., Qiu, Y., Xiong, Y., Xiao, H., Yi, H., et al. (2021). Effects of protein restriction and subsequent realimentation on body composition, gut microbiota and metabolite profiles in weaned piglets. Animals 11:6. doi: 10.3390/ani11030686
Isaacson, R., and Kim, H. B. (2012). The intestinal microbiome of the pig. Anim. Health Res. Rev. 13, 100–109.
Kaewtapee, C., Burbach, K., Tomforde, G., Hartinger, T., Camarinha-Silva, A., Heinritz, S., et al. (2017). Effect of Bacillus subtilis and Bacillus licheniformis supplementation in diets with low- and high-protein content on ileal crude protein and amino acid digestibility and intestinal microbiota composition of growing pigs. J. Anim. Sci. Biotechnol. 8:37. doi: 10.1186/s40104-017-0168-2
Kaliannan, K., Robertson, R. C., Murphy, K., Stanton, C., Kang, C., Wang, B., et al. (2018). Estrogen-mediated gut microbiome alterations influence sexual dimorphism in metabolic syndrome in mice. Microbiome 6:205. doi: 10.1186/s40168-018-0587-0
Kang, M., Yin, J., Ma, J., Wu, X., Huang, K., Li, T., et al. (2020). Effects of dietary histidine on growth performance, serum amino acids, and intestinal morphology and microbiota communities in low protein diet-fed piglets. Med. Inflamm. 2020:1240152. doi: 10.1155/2020/1240152
Kårlund, A., Gómez-Gallego, C., Turpeinen, A. M., Palo-oja, O. M., El-Nezami, H., and Kolehmainen, M. (2019). Protein supplements and their relation with nutrition, microbiota composition and health: is more protein always better for sports people? Nutritents 11:8. doi: 10.3390/nu11040829
Kim, M., Qie, Y., Park, J., and Kim, C. (2016). Gut microbial metabolites fuel host antibody responses. Cell Host. Microbe. 20, 202–214.
Kim, Y. J., Kim, T. H., Song, M. H., An, J. S., Yun, W., Lee, J. H., et al. (2020). Effects of different levels of crude protein and protease on nitrogen utilization, nutrient digestibility, and growth performance in growing pigs. J. Anim. Sci. Technol. 52, 659–667. doi: 10.5187/jast.2020.62.5.659
Knight, R. (2011). UCHIME improves sensitivity and speed of chimera detection. Bioinformatics 27, 2194–2200. doi: 10.1093/bioinformatics/btr381
Korpela, K. (2018). Diet, microbiota, and metabolic health: trade-off between accharolytic and proteolytic fermentation. Annu. Rev. Food Sci. Technol. 9, 65–84.
Lang, J. M., Pan, C., Cantor, R. M., Tang, W. H. W., Garcia-Garcia, J. C., Kurtz, I., et al. (2018). Impact of individual traits, saturated fat, and protein source on the gut microbiome. MBio 9, e1604–e1618. doi: 10.1128/mBio.01604-18
Lee, W., and Hase, K. (2014). Gut microbiota-generated metabolites in animal health and disease. Nat. Chem. Biol. 10:416.
Libao-Mercado, A. J. O., Zhu, C. L., Cant, J. P., Lapierre, H., Thibault, J., Sève, B., et al. (2009). Dietary and endogenous amino acids are the main contributors to microbial protein in the upper gut of normally nourished pigs. J. Nutr. 139, 1088–1094. doi: 10.3945/jn.108.103267
Liu, R., He, J., Ji, X., Zheng, W., and Yao, W. (2021). A moderate reduction of dietary crude protein provide comparable growth performance and improve metabolism via changing intestinal microbiota in Sushan nursery pigs. Animals 11:1166. doi: 10.3390/ani11041166
Liu, X. X., Blouin, J. M., Santacruz, A., Lan, A., Andriamihaja, M., Wilkanowicz, S., et al. (2015). High-protein diet modifies colonic microbiota and luminal environment but not colonocyte metabolism in the rat model: the increased luminal bulk connection. Am. J. Physiol. Gastrointest Liver Physiol. 307, G459–G470. doi: 10.1152/ajpgi.00400.2013
Lu, J., Zhang, X., Liu, Y., Cao, H., Han, Q., Xie, B., et al. (2019). Effect of fermented corn-soybean meal on serum immunity, the expression of genes related to gut immunity, gut microbiota, and bacterial metabolites in grower-finisher pigs. Front. Microbiol. 10:2620. doi: 10.3389/fmicb.2019.02620
Luo, Z., Li, C., Cheng, Y., Hang, S., and Zhu, W. (2015). Effects of low dietary protein on the metabolites and microbial communities in the caecal digesta of piglets. Arch. Anim. Nutr. 69, 212–226.
Ma, N., Tian, Y., Wu, Y., and Ma, X. (2017). Contributions of the interaction between dietary protein and gut microbiota to intestinal health. Curr. Protein. Pept. Sci. 18, 795–808.
Ma, X., He, P., Sun, P., and Han, P. (2010). Lipoic acid: an immunomodulator that attenuates glycinin-induced anaphylactic reactions in a rat model. J. Agric. Food Chem. 58, 5086–5092. doi: 10.1021/jf904403u
Martin, M. (2011). Cutadapt removes adapter sequences from high-throughput sequencing reads. Embnet. J. 17, 10–12. doi: 10.1089/cmb.2017.0096
Masuoka, H., Suda, W., Tomitsuka, E., Shindo, C., and Hirayama, K. (2020). The influences of low protein diet on the intestinal microbiota of mice. Sci. Rep. 10:17077.
Mayneris-Perxachs, J., Bolick, D. T., Leng, J., Medlock, G. L., Kolling, G. L., Papin, J. A., et al. (2016). Protein- and zinc-deficient diets modulate the murine microbiome and metabolic phenotype. Am. J. Clin. Nutr. 104, 1253–1262. doi: 10.3945/ajcn.116.131797
Menon, R., Watson, S. E., Thomas, L. N., Allred, C. D., Dabney, A., Azcarate-Peril, M. A., et al. (2013). Diet complexity and estrogen receptor β status affect the composition of the murine intestinal microbiota. Appl. Environ. Microbiol. 79, 5763–5773. doi: 10.1128/AEM.01182-13
Mitchell, S., McKenzie, E., Mitchell, C., Milan, A., Zeng, N., D’Souza, R., et al. (2020). A period of 10 weeks of increased protein consumption does not alter faecal microbiota or volatile metabolites in healthy older men: a randomised controlled trial. J. Nutr. Sci. 9:e25.
National Research Council (NRC) (1998). Nutrient Requirements of Swine. Washington DC: National Academy Press.
National Research Council (NRC) (2012). Nutrient Requirements of Swine. Washington DC: National Academy Press.
Org, E., Mehrabian, M., Parks, B. W., Shipkova, P., Liu, X., Drake, T. A., et al. (2016). Sex differences and hormonal effects on gut microbiota composition in mice. Gut. Microbes. 7, 313–322.
Peng, Y., Yu, K., Mu, C., Hang, S., Che, L., and Zhu, W. (2017). Progressive response of large intestinal bacterial community and fermentation to the stepwise decrease of dietary crude protein level in growing pigs. Appl. Microbiol. Biotechnol. 101, 1–12. doi: 10.1007/s00253-017-8285-6
Pieper, R., Kröger, S., Richter, J. F., Wang, J., Martin, L., Bindelle, J., et al. (2012). Fermentable fiber ameliorates fermentable protein-induced changes in microbial ecology, but not the mucosal response, in the colon of piglets. J. Nutr. 142:661. doi: 10.3945/jn.111.156190
Pollock, J., Hutchings, M. R., Hutchings, K. E. K., Gally, D. L., and Houdijk, J. G. M. (2019). Changes in the ileal, but not fecal, microbiome in response to increased dietary protein level and enterotoxigenic Escherichia coli exposure in pigs. Appl. Environ. Microbiol. 85:e01252.
Quast, C., Pruesse, E., Yilmaz, P., Gerken, J., and Glckner, F. O. (2012). The SILVA ribosomal RNA gene database project: improved data processing and web-based tools. Nucl. Acids Res. 41, D590–D596. doi: 10.1093/nar/gks1219
Razavi, A. C., Potts, K. S., Kelly, T. N., and Bazzano, L. A. (2019). Sex, gut microbiome, and cardiovascular disease risk. Biol. Sex Differ. 10:29.
Rist, V. T. S., Weiss, E., Eklund, M., and Mosenthin, R. (2013). Impact of dietary protein on microbiota composition and activity in the gastrointestinal tract of piglets in relation to gut health: a review. Animal 7:1067. doi: 10.1017/S1751731113000062
Rist, V. T. S., Weiss, E., Sauer, N., Mosenthin, R., and Eklund, M. (2014). Effect of dietary protein supply originating from soybean meal or casein on the intestinal microbiota of piglets. Anaerobe 25, 72–79.
Shang, X., Deng, B., Zhu, X., Tao, X., Yuan, Q., and Feng, S. (2020). Effect of dietary protein levels on digestion and metabolism of growing-finishing pigs. J. Nort. Agric. University 51, 54–62.
Shi, Q., Zhu, Y., Wang, J., Yang, H., and Zhu, W. (2019). Protein restriction and succedent realimentation affecting ileal morphology, ileal microbial composition and metabolites in weaned piglets. Animal 13, 1–10. doi: 10.1017/S1751731119000776
Shibata, N., Kunisawa, J., and Kiyono, H. (2017). Dietary and microbial metabolites in the regulation of host immunity. Front. Microbiol. 8:21.
Shili, C. N., Broomhead, J. N., Spring, S. C., Lanahan, M. B., and Pezeshki, A. (2020). A novel corn-expressed phytase improves daily weight gain, protein efficiency ratio and nutrients digestibility and alters fecal microbiota in pigs fed with very low protein diets. Animals 10:1926. doi: 10.3390/ani10101926
Shin, J. H., Park, Y. H., Sim, M., Kim, S. A., Joung, H., and Shin, D. M. (2019). Serum level of sex steroid hormone is associated with diversity and profiles of human gut microbiome. Res. Microbiol. 170, 192–201. doi: 10.1016/j.resmic.2019.03.003
Singh, R. K., Chang, H. W., Yan, D., Lee, K. M., Ucmak, D., Wong, K., et al. (2017). Influence of diet on the gut microbiome and implications for human health. J. Transl. Med. 15, 1–17.
Smuda, C., and Bryce, P. J. (2011). New developments in the use of histamine and histamine receptors. Curr. Allergy Asthma Rep. 11, 94–100.
Spring, S., Premathilake, H., Bradway, C., Shili, C., and Pezeshki, A. (2020). Effect of very low-protein diets supplemented with branched-chain amino acids on energy balance, plasma metabolomics and fecal microbiome of pigs. Sci. Rep. 10:15859. doi: 10.1038/s41598-020-72816-8
te Pas, M. F. W., Jansman, A. J. M., Kruijt, L., van der Meer, Y., Vervoort, J. J. M., and Schokker, D. (2020). Sanitary conditions affect the colonic microbiome and the colonic and systemic metabolome of female pigs. Front. Vet. Sci. 7:585730. doi: 10.3389/fvets.2020.585730
van der Giessen, J., van der Woude, C. J., Peppelenbosch, M. P., and Fuhler, G. M. A. (2019). Direct effect of sex hormones on epithelial barrier function in inflammatory bowel disease models. Cells 8:261. doi: 10.3390/cells8030261
Wang, D., Chen, G., Song, L., Chai, M., Wang, Y., Shui, S., et al. (2020). Effects of dietary protein levels on bamei pig intestinal colony compositional traits. Biomed. Res. Int. 2020:2610431. doi: 10.1155/2020/2610431
Wang, H., Xu, R., Zhang, H., Su, Y., and Zhu, W. (2020). Swine gut microbiota and its interaction with host nutrient metabolism. Anim. Nutr. 6, 410–420.
Wang, Y., Han, S., Zhou, J., Li, P., Wang, G., Yu, H., et al. (2020). Effects of dietary crude protein level and N-carbamylglutamate supplementation on nutrient digestibility and digestive enzyme activity of jejunum in growing pigs. J. Anim. Sci. 98, 1–10. doi: 10.1093/jas/skaa088
Williams, B. A., Verstegen, M., and Tamminga, S. (2001). Fermentation in the large intestine of single-stomached animals and its relationship to animal health. Nut. Res. Rev. 14:207. doi: 10.1079/NRR200127
Yu, D., Zhu, W., and Hang, S. (2019). Effects of long-term dietary protein restriction on intestinal morphology, digestive enzymes, gut hormones, and colonic microbiota in pigs. Animals 9:180. doi: 10.3390/ani9040180
Yu, M., Li, Z., Chen, W., Rong, T., Wang, G., and Ma, X. (2019). Microbiome-metabolomics analysis investigating the impacts of dietary starch types on the composition and metabolism of colonic microbiota in finishing pigs. Front. Microbiol. 10:11. doi: 10.3389/fmicb.2019.01143
Yu, M., Zhang, C., Yang, Y., Mu, C., Su, Y., Yu, K., et al. (2017). Long-term effects of early antibiotic intervention on blood parameters, apparent nutrient digestibility, and fecal microbial fermentation profile in pigs with different dietary protein levels. J. Anim. Sci. Biotechnol. 8:60. doi: 10.1186/s40104-017-0192-2
Zhang, H., Wielen, N. V., Hee, B. V., Wang, J., Hendriks, W., and Gilbert, M. (2020). Impact of fermentable protein, by feeding high protein diets, on microbial composition, microbial catabolic activity, gut health and beyond in pigs. Microorganisms 8:1735. doi: 10.3390/microorganisms8111735
Zhao, Y., Tian, G., Chen, D., Zheng, P., and Yu, B. (2019). Effect of different dietary protein levels and amino acids supplementation patterns on growth performance, carcass characteristics and nitrogen excretion in growing-finishing pigs. J. Anim. Sci. Biotechno. 10:75. doi: 10.1186/s40104-019-0381-2
Zhao, Y., Tian, G., Chen, D., Zheng, P., Yu, J., He, J., et al. (2020). Dietary protein levels and amino acid supplementation patterns alter the composition and functions of colonic microbiota in pigs. Anim. Nutr. 6, 143–151.
Keywords: dietary protein level, growing-finishing pig, microbiota, metabolite, barrow, gilt
Citation: Tao X, Deng B, Yuan Q, Men X, Wu J and Xu Z (2021) Low Crude Protein Diet Affects the Intestinal Microbiome and Metabolome Differently in Barrows and Gilts. Front. Microbiol. 12:717727. doi: 10.3389/fmicb.2021.717727
Received: 31 May 2021; Accepted: 29 July 2021;
Published: 20 August 2021.
Edited by:
Riadh Hammami, University of Ottawa, CanadaReviewed by:
Nicholas Gabler, Iowa State University, United StatesRongrong Ding, South China Agricultural University, China
Sarah C. Pearce, Agricultural Research Service, United States Department of Agriculture, United States
Copyright © 2021 Tao, Deng, Yuan, Men, Wu and Xu. This is an open-access article distributed under the terms of the Creative Commons Attribution License (CC BY). The use, distribution or reproduction in other forums is permitted, provided the original author(s) and the copyright owner(s) are credited and that the original publication in this journal is cited, in accordance with accepted academic practice. No use, distribution or reproduction is permitted which does not comply with these terms.
*Correspondence: Ziwei Xu, zjsxzw@126.com
†These authors share first authorship