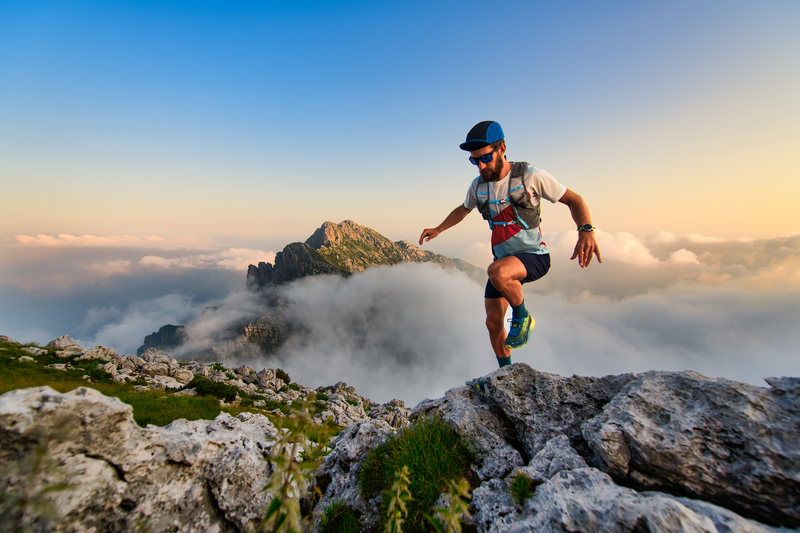
94% of researchers rate our articles as excellent or good
Learn more about the work of our research integrity team to safeguard the quality of each article we publish.
Find out more
ORIGINAL RESEARCH article
Front. Microbiol. , 03 December 2021
Sec. Systems Microbiology
Volume 12 - 2021 | https://doi.org/10.3389/fmicb.2021.711905
This article is part of the Research Topic Omics-based Analysis on the Interaction Between Microbe and Agricultural Animals View all 17 articles
Laying and reproductive performance, egg quality, and disease resistance of hens decrease during the late laying period. Exogenous enzymes promote nutrient digestibility and utilization and improve the intestinal environment. However, the specific regulation of the gut microbiome and metabolome by exogenous enzymes remains unelucidated. This study was conducted to evaluate effects of dietary multi-enzyme supplementation on egg and reproductive performance, egg quality, ileum microbiome, and metabolome of breeders. Here, 224 Hy-Line Brown breeding hens (55 weeks old) were randomly allocated to two groups: dietary controls fed basal diet (DC), and test hens fed 0.2 g/kg corn enzyme diet (CE). Serum levels of total protein, globulin, immunoglobulin Y, and antibodies against the Newcastle disease virus and avian influenza H9 strain were significantly increased (p < 0.05). Egg albumen height, Haugh unit, and fertilization and hatching rates were also significantly increased (p < 0.05) in the CE-fed group. 16S rRNA sequence analysis showed that CE strongly affected both α- and β-diversity of the ileal microbiota. LEfSe analysis revealed that the potentially beneficial genera Lactobacillus, Enterococcus, Faecalicoccus, and Streptococcus were enriched as biomarkers in the CE-fed group. Microbial functional analysis revealed that the functional genes associated with harmful-substance biodegradation was significantly increased in the CE-fed group. Additionally, Spearman correlation analysis indicated that changes in microbial genera were correlated with differential metabolites. In summary, dietary multi-enzyme addition can improve egg quality, humoral immunity, and reproductive performance and regulate the intestinal microbiome and metabolome in breeders. Therefore, multi-enzymes could be used as feed additive to extend breeder service life.
With increasing age, physiological function and digestive enzyme activity decrease and are always accompanied by gut microbiota disorder after the peak laying period in breeding hens, causing significant economic loss (Liu et al., 2013; Jing et al., 2014; Gu et al., 2021). Exogenous addition of enzymes was considered to improve the degradation of harmful macromolecules and activity of endogenous enzymes to assist in the degradation of starch and protein (Gu et al., 2021).
Starch is a complex polysaccharide composed of amylose and amylopectin (AP). AP accounts for 70–80% of most starch sources and requires pullulanase for hydrolysis (Scott et al., 2013; Yin et al., 2018). Pullulanase is an important debranching enzyme that originates from bacteria, plants, and less commonly, fungi. Specifically, it could often attack α-1,6 linkages, thereby efficiently converting branched polysaccharides into small molecular sugars (Hii et al., 2012; Tomasik and Horton, 2012). In contrast to pullulanase, α-amylases split the α-1,4 glycosidic linkages in amylose to yield maltose and glucose (Sarian et al., 2017). Studies have demonstrated that the addition of α-amylase to a corn-soybean diet can release more feed energy and significantly improve apparent nutrient digestibility, digestive enzyme activity, and production performance of poultry (Aderibigbe et al., 2020). Glucoamylase (also known as amyloglucosidase or AMG) is an important digestive enzyme that mainly saccharifies partially processed starch/dextrin to glucose, which helps poultry absorb nutrients (da Costa Luchiari et al., 2021). Previous research indicated that supplementation with glucoamylase or protease combined with amylase could improve starch digestibility and gut microbiota diversity and promote the growth of broilers (Yin et al., 2018). Proteases can enhance protein and amino acid digestibility and reduce the adverse effects of heat-stabilized trypsin inhibitors or lectins, thus improving forage quality (Cowieson et al., 2017; Walk et al., 2018). A significant increase in the ileal digestibility of protein and amino acids occurs with proteases in poultry diets (Romero et al., 2014). Overall, exogenous enzymes can communicate with the host by utilizing indigestible dietary components and providing nutrients to regulate digestive, immune, and antioxidant functions to facilitate production performance and benefit the host (Pan and Yu, 2014; Cowieson and Kluenter, 2019; Monier, 2020; Giacobbo et al., 2021).
The use of enzymes in poultry feed is not uncommon. However, the role of enzymes in feed digestibility, productivity, and health of chickens is influenced by several factors, including the source, type, characteristics, dosage, and composition of complex enzymes as well as the diet structure, composition, and physiological status of chickens. In this study, we first evaluated the effects of new multi-enzymes (proteases, pullulanase, α-amylase, and glucoamylases) on laying performance, egg quality, reproductive performance, and immunity of older breeding hens and investigated the underlying mechanism through in-depth microbiome and metabolome analyses. Our objective was to develop a new nutritional strategy to improve health and extend the service life of breeding hens in their later laying stage.
The Animal Welfare Policy has approved the bird management and handling procedures. All animal procedures were performed according to the principles of the Animal Care and Use Committee of the China Agricultural University. A total of 224 Hy-Line Brown breeding hens (55-week-old) with similar production performances and weights were randomly divided into two treatment groups with seven replicates of 16 hens each (4 hens per cage, 40 cm wide, 62 cm long, and 45 cm high). One is the dietary control fed with basal diet (DC), and the other with 0.2 g/kg corn enzyme diet (CE). The CE diet contained 11,000 u/g proteases, 20 u/g pullulanase, 1,000 u/g α-amylase, and 1,000 u/g glucoamylases, and was provided by the Wuhan SunHY Biological Co., Ltd. All hens were handled following the Hy-Line Brown Laying Hens Management Guide, and the hens were housed at the HuaYu Poultry Breeding Co., Ltd. (Handan, Hebei). All experimental hens were vaccinated with inactivated Newcastle virus (NDV) plus avian influenza virus (H9 subtype) strain vaccine by intermuscular injection at 55 weeks of age. The essential diet is shown in Table 1 and meets the Chinese standards of agricultural trade standardization (NY/T33-2004).
Eggs were collected daily during the experiment. The number of eggs laid, abnormal eggs, broken eggs, and egg weights were recorded on a replicate basis. The feed intake for each repetition was counted every 2 weeks. The average egg production rate, average egg weight, broken egg rate, abnormal egg rate, and feed egg ratio were calculated for 1–4, 5–8, and 1–8 weeks. Mortality was recorded daily as it appeared.
Ten eggs were randomly collected from each replicate (70 eggs/group) for internal and external quality analyses during the last 2 days of the experiment. An egg-shaped index tester was used to measure the egg length and shortest diameter. An eggshell color tester was used to measure the eggshell color value (Konicaminolta CM-2600d). A quasi-static compression device (Robotmation, Japan) was used to measure the eggshell breaking strength. After removing the inner shell membrane, the eggshell thickness was measured using a micrometer screw gauge at three different locations (lower, middle, and upper ends). Egg weight, albumen height, Haugh units, and yolk color were measured using an automatic egg quality analysis device (EMT-5200, Japan).
Blood samples were collected for analyzing blood biochemistry and detecting serum antibody titers for 1 day before the end of the experiment. After fasting for 8 h, one hen per replicate was randomly selected (a total of 7 hens/group), and whole blood was collected from the wing vein using sterile blood collection tubes. The blood was centrifuged at 3,000 rpm for 10 min. The serum was extracted into a sterile 2 mL centrifuge tube and stored at −20°C until detection. Serum was used to detect aspartate aminotransferase (AST), total protein (TP), albumin (ALB), globulin (GLB), albumin/globulin, high-density lipoprotein cholesterol (HDL-C), immunoglobulin Y (IgY), and total antioxidant capacity (T-AOC). All indexes were tested using kits purchased from the Nanjing Jiancheng Bioengineering Institute (Nanjing, China). Other serum samples were used to detect antibody titers of NDV and avian influenza H9 strains by hemagglutination and hemagglutination inhibition assays. The virus, antigen, and positive control sera were purchased from Qingdao Yebio Biological Engineering Co., Ltd.
All hens were inseminated on days 49 and 50 for 2 consecutive days of the formal phase. The semen was mixed and came from the same 12 cocks to ensure consistent semen quality. Eggs were collected on the 53rd–54th days. The total number of eggs produced and eligible hatching eggs were recorded and placed into pre-fumigated incubators. On the 18th day of incubation, the number of fertilized eggs was recorded by candling, and the eggs of identical replicates were placed in one string bag. On the 21st day of incubation, the number of newborn chicks in each replicate was recorded. Lastly, the rates of fertilized eggs and hatch of fertile (HoF) were calculated.
One hen per replicate was randomly selected (a total of 6 hens/group, one sample less than the number of replicates was due to unqualified DNA amplification), and euthanasia was performed using carbon dioxide on the last day of this trial (56 days). The ileum contents from each bird were collected and immediately frozen in liquid nitrogen until DNA extraction. Microbial genomic DNA extraction was conducted according to the manufacturer’s instructions using the QIAamp 96 Powerfecal Qiacube HT Kit (5) (CatNo. 51531). DNA purity and concentration were detected using a NanoDrop 2000 spectrophotometer (Thermo Fisher Scientific, Waltham, MA, United States) and agarose gel electrophoresis. The purified DNA targeted the V3–V4 region of the 16S rDNA gene according to PCR bar-coded primers (343F: 5′-TACGGRAGGCAGCAG-3′ and 798R: 5′-AGGGTATCTAATCCT-3′). PCR was conducted using the KAPA HiFi Hot Start Ready Mix (KAPA Biosystems, Wilmington, MA, United States). Both reverse primers included a barcode and an Illumina sequencing adapter. The PCR products were visualized using 1% agarose gel electrophoresis, purified, and quantified using Agencourt AMPure XP beads (Beckman Coulter Co., United States) and Qubit dsDNA HS assay kit (Thermo Fisher Scientific), respectively. Sequencing was performed using an Illumina MiSeq platform with two paired-end read cycles of 300 bases each (Illumina Inc., San Diego, CA; OE Biotech Company, Shanghai, China).
Microbiota data were subjected to bioinformatics analysis using QIIME software (version 1.8.0) (Caporaso et al., 2010). Data quality filtering, ambiguous bases, low-quality sequence removal, paired-end read assembly, and detachment of chimeric sequences were conducted using QIIME (Caporaso et al., 2010), Trimmomatic (Bolger et al., 2014), FLASH (Reyon et al., 2012), and UCHIME algorithms (Edgar et al., 2011), respectively. Reads with a similarity threshold of ≥ 97% were assigned to the same operational taxonomic unit (OTU) using the Vsearch pipeline (Rognes et al., 2016). Taxonomy was assigned to the OTUs using the SILVA database (v.123) with the RDP classifier at a 70% confidence threshold (Quast et al., 2012). Alpha diversity (Chao1, Observed, Shannon, Simpson’s diversity) and beta diversity (principal coordinate analysis; PCoA) were calculated using QIIME 1.8 scripts.
Linear discriminant analysis (LDA) effect size (LEfSe) (Segata et al., 2011)1 was used to identify representative species. LDA was performed from the phylum to genus level, and LDA scores ≥ 4.0 and p-values < 0.05 were considered signature taxa and selected for plotting and further analysis. The predicted metagenomic functional content was determined using PICRUSt2 software by combining 16s rRNA data against the Greengenes database and the normalized data were analyzed to predict metagenomes using the Kyoto Encyclopedia of Genes and Genomes (KEGG) Orthology database.3 Pairwise statistical comparative analysis (Welch’s t-test, storey FDR correction) of microbial function was performed using STAMP (V2.1.3) (Parks et al., 2014). The microbial co-occurrence network analysis was performed using the CCLasso, sparCC, and NAMAP with Spearman correlation inference algorithm to elucidate gut microbiota interactions by MetagenoNets with default parameters (Nagpal et al., 2020). Only significant correlations (p < 0.05) based on the bootstrapping of 100 iterations were plotted.
The ileal chyme (30 mg) was precisely weighed and transferred to 1.5 mL microcentrifuge tubes (Eppendorf), to which two 3 mm stainless steel beads were added. Then, 20 μL of L-2-chlorophenylalanine (0.3 mg/mL) and 17:0 Lyso PC (1-heptadecanoyl-sn-glycero-3-phosphocholine, 0.01 mg/mL) were used as the internal standard. Both were configured with methanol. An internal standard mixed with 400 μL of methanol aqueous solution (CH3OH: H2O, V: V = 4:1) was added to each sample and pre-cooled at −20°C for 2 min. The sample was then ground in a fully automatic sample fast grinding machine (60 Hz, 2 min; Shanghai Jingxin Industrial Development Co., Ltd., Shanghai, China) and placed in an ultrasonic bath with ice water for 10 min. The sample was placed in a −20°C refrigerator for 20 min before centrifugation at 13,000 rpm at 4°C for 10 min. The supernatant was removed with a syringe and filtered by passing through a 0.22 μm-membrane filter to an LC-MS vial and stored at −80°C for subsequent analysis by LC-MS. Water, acetonitrile, formic acid, and methanol were purchased from CNW Technologies GmbH (Düsseldorf, Germany). L-2-chlorophenylalanine was purchased from Shanghai Hengchuang Bio-Technology Co., Ltd. (Shanghai, China). LysoPC17:0 was purchased from Avanti (Avanti Polar Lipids Inc., United States). All solvents and chemicals were of analytical or high-performance LC grade.
Metabolomics analysis was conducted using the Dionex U3000 UHPLC system (Waltham, MA, United States) coupled to a high-resolution QE plus mass spectrometer (Thermo Fisher Scientific) to analyze the metabolic profiles of the positive and negative ion modes. The LC system was fitted with an ACQUITY UPLC BEH C18 (100 × 2.1 mm, 1.7 μm) and a binary gradient elution system consisting of A) water (containing 0.1% formic acid) and B) acetonitrile (containing 0.1% formic acid) by the following separation gradient: 0 min 5% B, 1 min 5% B, 11 min 100% B, 13 min 100% B, 13.1 min 5% B, and 15 min 5% B. The column temperature was 50°C, and the flow rate was 0.35 mL/min. The injection volume was 5 μL, and the samples were randomized to avoid systematic errors. The mass spectrometer conditions and parameters were as follows: spray voltage, 3,800 V in positive mode, and 3,000 V in negative mode; capillary temperature, 320°C; aux gas heater temperature, 350°C; sheath gas flow rate, 35 arbitrary units; Aux gas flow rate, 8 arbitrary units; mass range: 70–1,000 m/z; full ms resolution, 70,000; MS/MS resolution, 17,500; and NCE, 20 and 40.
LC-MS raw data were collected by UNIFI (version 1.8.1) and then processed using Progenesis QI (version 2.3) with the following threshold parameters: precursor tolerance of 5 ppm, product tolerance of 10 ppm, and production threshold of 5%. Metabolites were identified by retention time, exact mass, and tandem MS data against the Human Metabolome Project,4 Lipidmaps (v2.3)5 and METLIN6 databases. All metabolites with a percentage of missing values>50% and quality scores<30 were discarded by qualitative screening.
Metabolome data were subjected to bioinformatics analysis using the SIMCA software (version 14.0, Umetrics, Umeå, Sweden). Principal component analysis (PCA) and orthogonal partial least squares discriminant analysis (OPLS-DA) models and plots were constructed using SIMCA. Volcano plots were plotted using the R package ggplot2. The differential metabolites were converted from names to KEGG compound IDs using MetaboAnalyst software (version 5.0),7 CTS (Wohlgemuth et al., 2010), and MBRole software (version 2.0).8 These IDs were used as input files for metabolite set enrichment analysis using MetaboAnalyst 5.0 software [annotations: KEGG pathway; Organism: Homo sapiens (human)] and MBRole 2.0 software [annotations: KEGG pathway; Organism: Gallus gallus (chicken)]. We also applied the pathway topology analysis [annotations: KEGG pathway; Organism: G. gallus (chicken)] to verify our findings using MetaboAnalyst with the default setting. Considering the relative lack of lipid information in the KEGG database, the differential metabolites that were annotated in the LipidMaps database were enriched by LIPEA9 [annotations: KEGG pathway; Organism: G. gallus (chicken)]. Spearman’s correlation between the differential microbial biomarkers and metabolites and the three identified metabolites and six microbial biomarkers were analyzed using R software. Only correlation coefficients with an absolute value of | r| > 0.6 (Adj P-value < 0.05) were considered a significant relationship. Network visualizations were performed using Gephi software (version 0.9.2, The Gephi Consortium, Paris, France) (Dalcin and Jackson, 2018).
All graphs and data calculations were generated using R software (version 4.0.2), Prism8 (GraphPad, United States) software, and SPSS 24.0 (SPSS Inc., Chicago, IL, United States) software. Measurement data are expressed as the mean and standard error. A normal distribution and homogeneity of variance were performed. Comparisons between the two groups were performed using Student’s t-test when it conformed to normal distribution and homogeneity of variance; otherwise, the non-parametric Wilcoxon rank-sum test was performed. P < 0.05 were considered as significant and 0.05 < p < 0.1 was considered a trend.
The laying performance of breeding hens fed the CE diet is presented in Table 2. Egg production, egg weight ratio, damaged egg ratio, abnormal egg ratio, FCR, mortality, and feed intake were not affected by CE administration at 55–59, 59–63, and 55–63 weeks (p > 0.05). The egg quality results are presented in Table 3. CE administration significantly increased the egg albumen height and Haugh unit (p < 0.05) but weakened the yolk color (p < 0.05) compared with those in the DC-fed group at week 63.
Table 3. Effect of supplemental multi-enzyme on the egg quality of aged breeding hens (n = 70/group).
Serum biochemical and antibody levels are physiological indices commonly used to evaluate animal health and immunity. CE administration significantly increased serum TP, GLB, IgY, HDL-C, and T-AOC levels. Serum AST levels were also markedly reduced, and a non-significant trend of decreased A/G (p = 0.055) was observed after supplementation with CE (Figure 1A). Furthermore, CE administration could enhance humoral immunity in hens by increasing serum-specific antibody titers against NDV and avian influenza H9 strains (Figure 1B).
Figure 1. CE can increase the level of serum immunity, antioxidant, and liver-related indicators and significantly improve breeding hens’ reproduction performance (n = 7 hens/group). (A) The levels of biochemical parameters of breeding hens. (B) Serum antibody titers of NDV and avian influenza H9 strain of breeding hens. (C) The reproduction performance efficiency of breeding hens. Left: representative fertilized eggs rate; Right: representative the hatching of fertile (HoF) rate. ALB, Albumin; AST, Aspartate Aminotransferase; GLB, Globulin; HDL-C, High-density lipoprotein cholesterol; IgY, Immunoglobulin Y; ND, Newcastle disease. T-AOC, Total Antioxidant Capacity; TP, Total protein. *P < 0.05 compared to two groups.
Reproductive performance is a vital indicator in breeding poultry, which affects the economic effectiveness of breeder companies. Descriptive data on the reproductive performance of aged breeder hens are shown in Figure 1C. The rate of fertilization and hatching of fertile (HoF) value was significantly improved upon CE supplementation (p < 0.05).
After size filtering, quality control, and chimera removal, an average of 29,200 clean tags and 27,376 valid tags were harvested from each sample for subsequent analysis through 16S amplicon sequencing. The species accumulation curve (Figure 2A) and alpha diversity rarefaction curve (Figure 2B) reached a stable plateau under the sample size and sequencing depth. The alpha diversity index reflects the richness and uniformity of the species composition. The Chao1 and Observed species indices are estimators of phylotype richness, and Shannon and Simpson’s diversity indices reflect both richness and community uniformity. In this study, Shannon and Simpson’s diversity indices were significantly enhanced (p < 0.05), while Chao1 and Observed species had a minimal effect on the addition of CE (Figure 2C). The Venn diagram showed that 635 distinct OTUs were clustered based on 97% sequence similarity, among which 258 were shared by both groups (Figure 2D). PCoA based on weighted UniFrac similarity showed a separation of each group (Figure 2E), with 61.33, 19.76, and 10.34% variation explained by principal components: PC1, PC2, and PC3, respectively (Adonis, p = 0.009, R2 = 0.49).
Figure 2. The microbial community structure in the ileum was significantly altered by adding CE (n = 6 hens/group). (A) Species accumulation curve is used to estimate the rationality of sequencing sample quantity. (B) Alpha diversity Rarefaction curve based on Good’s Coverage value, which reflects the rationale of sequencing depth. (C) Alpha-diversity evaluation of ileum microbial richness and evenness by measuring chao1, observe-species, Shannon, and Simpson’s diversity indexes. (D) Venn diagram is used to represent the amount of shared and unique OTUs numbers. (E) Principal coordinate analysis (PCoA) is used to determine the similarities of microbial communities between different groups. *P < 0.05 compared to two groups.
Firmicutes, Proteobacteria, and Bacteroidetes were the dominant phyla in the aged breeder hens (relative abundance>1%), accounting for more than 98% of the total bacterial community (Figure 3A). The relative abundance of Proteobacteria increased from 5.94 to 21.05%, and the proportion of Firmicutes decreased from 90.79 to 75.87% with CE supplementation.
Figure 3. The stacked graph of microbial community structure at (A) phylum level, (B) family level and (C) genus level (n = 6 hens/group). The bar chart on the right represents the relative abundance distribution of TOP 10 bacteria at different taxonomic levels, respectively.
At the family level, the phyla of Firmicutes mainly contained Lactobacillaceae, Peptostreptococcaceae, Enterococcaceae, Erysipelotrichaceae, and Lachnospiraceae. Proteobacteria consisted of Helicobacteraceae, Pasteurellaceae, and mitochondria, while Bacteroidetes specifically included the Bacteroidales_S24_7_group (Figure 3B) (relative abundance>1%). Lactobacillaceae and Peptostreptococcaceae were the dominant bacteria in the two groups, and their relative abundances in CE and DC were 44.42 vs. 14.38% and 25.07 vs. 70.57%, respectively.
At the genus level, Romboutsia, Lactobacillus, Turicibacter, Enterococcus, Gallibacterium, and Helicobacter were the predominant genera in the two groups (Figure 3C) (relative abundance>1%). With the addition of CE, the relative abundance of Lactobacillus and Enterococcus increased, while the relative abundance of Romboutsia decreased.
LDA and effective size comparisons (LEfSe) were conducted to identify the core taxa most likely to explain the differences between the groups. The CE-treated samples appeared to be dominated by Lactobacillus, Enterococcus, Faecalicoccus, and Streptococcus, whereas DC samples showed Romboutsia, Faecalibacterium, and Burkholderia as the dominant genera (Figure 4A).
Figure 4. DC and CE have differential bacteria composition and functional preferences (n = 6 hens/group). (A) LEfSe analysis was performed to identify the bacteria that are differentially represented among the two groups. (B) Microbial functional analysis was conducted by PICRUSt software under different experimental conditions.
Significant differences in the gut microbiota were observed between the two groups; however, their functions remain unknown. Hence, we performed a PICRUSt analysis to predict the potential functions of the gut microbiota. All functional genes were divided at level 3. When filtered for non-bacterial functional pathway, the predicted metabolic functional categories in the CE-fed group were related to pathways of biodegradation and metabolism of several xenobiotics, such as “polycyclic aromatic hydrocarbon degradation,” “aminobenzoate degradation,” and “ethylbenzene degradation.” The CE group was also enriched for pathways such as “glycosyltransferases,” “carbohydrate digestion and absorption,” and “D-Arginine, and D-ornithine metabolism.” Pathways such as “sporulation,” “cyanoamino acid metabolism,” “biosynthesis of ansamycins,” “thiamin metabolism,” and “methane metabolism” were enriched in the DC group (Figure 4B).
The ileal metabolome was analyzed in both groups to investigate the effect of multi-enzyme supplementation on the ileal chyme. LC-MS detected 23,595 untargeted peaks, and 4,884 metabolites were annotated. To reduce dimensionality, we applied PCA and OPLS-DA to leverage both unsupervised and supervised dimensionality reduction techniques to achieve this goal. Both PCA and OPLS-DA showed separation and discrimination (Figures 5A,B). The quality parameter values of the OPLS-DA model were predicted to be [R2X (cum) = 0.733, R2Y (cum) = 0.947] and fitness [Q2 (cum) = 0.698], which indicated that the model had good reliability and predictability (Figure 5C). The volcano plot indicated up-and downregulated differential metabolites based on statistical values (p < 0.05, | log2FC| > 1), and p < 0.001, | log2FC| > 2 was considered to have higher significance (Figure 5D).
Figure 5. The gut metabolites were remarkably perturbed by adding CE (n = 6 hens/group). (A) Principal component analyses (PCA) of ileum metabolome. (B) Orthogonal projections to latent structures-discriminate analysis (OPLS-DA) score plot was performed on ileum in DC and CE groups. In the permutation validation plot (C) (200 cycles) the Y-axis intercepts of R2 and Q2 are 0.855 and −0.517, respectively, indicating that the model is valid. (D) Volcano plots of ileum profiles showing log2(fold-change) and −log10(p-value) in metabolites levels induced by adding CE (up-regulated in red and down-regulated in green). The labeled metabolites were of particular interest (| fold change| > 4, p < 0.001).
In total, 180 differential metabolites were assigned based on VIP values (VIP > 1) and p-values (p < 0.05). The results of MBRole and MetaboAnalyst (Figure 6A) showed that the differential metabolites were enriched in the “aminoacyl-tRNA biosynthesis,” “ABC transporters,” “D-glutamine and D-glutamate metabolism,” and “arginine biosynthesis pathway.” Moreover, the “arginine biosynthesis pathway” was the most prominent position in the topological analysis (Figure 6B). The LIPEA results (Figure 6A) indicated that the following pathways were significantly enriched by inputting differential lipid metabolites: “glycerophospholipid metabolism,” “glycosylphosphatidylinositol (GPI)-anchor biosynthesis,” “autophagy—other,” “autophagy—animal,” and “ferroptosis” pathways.
Figure 6. Differential metabolites were significantly enriched in multiple pathways, suggesting that the addition of CE remarkably perturbed gut metabolic activities (n = 6 hens/group). (A) Functional KEGG pathway enrichment analysis of the differential expressed metabolites was carried out through three different platforms (LIPEA, MBRole, and MetaboAnalyst). (B) Analysis of metabolic pathways of DC and CE groups of differentially expressed metabolites was shown by bubble plot, each dot represents a metabolic pathway. X-axis and Y-axis stand for pathway impact and −log10(P-value), respectively.
To further explore the complex microbial community structures in the DC and CE groups, we performed co-occurrence network analysis by calculating CCLasso (Fang et al., 2015), sparCC (Friedman and Alm, 2012), and NAMAP with Spearman correlation inference algorithm via MetagenoNets between microbial taxa at the genus level based on 16S sequencing (Nagpal et al., 2020; Figure 7A). The results showed that the addition of CE significantly increased the interrelationship between bacteria under all three algorithms [edges: 2,541 vs. 954; 815 vs. 425; 137 vs. 33, CE vs. DC (CCLasson, SparCC, Spearman, respectively)], while the number of correlated nodes did not change significantly [nodes: 91 vs. 90; 88 vs. 89; 47 vs. 39, CE vs. DC (CCLasson, SparCC, Spearman, respectively)]. CCLasso obtained the highest number of interrelationships, followed by the SparCC and Spearman algorithms. All three algorithms indicated that CE activated the interactions between bacteria. Different algorithms have unique advantages and shortcomings. SparCC (Friedman and Alm, 2012) is a microbial network algorithm developed based on the log-ratio transformation of compositional data, which solves the problem of poor performance of the Spearman algorithm under the sparsity condition of bacterial communities; however, it did not consider the influence of errors in the compositional data (Fang et al., 2015). CCLasso made improvements based on such issues and had the characteristic of better edge recovery.
Figure 7. CE enhanced co-expression correlation among the microbial community and the differential metabolites were tightly associated with the signature microbiota. (A) Microbial community co-expression network analysis explores the relationship between CE and DC groups through three standard algorithms. Different nodes represent different bacterial genera, colors represent phyla, and the dots size represent node degree. (B) A network diagram of differential microbiota-metabolites demonstrates that the up-regulated microbial were closely related with the up-regulated metabolites while down-regulated microbial were closely related with the down-regulated metabolites.
Constructing a network between differential microbiota and metabolites is important for understanding their interaction relationships. Spearman correlation analysis of six microbiota (by LEfSe, LDA > 4, p < 0.05) and 180 metabolites (by p < 0.05, VIP > 1) was conducted (Figure 7B). The results showed that the bacteria enriched in CE were remarkably correlated with the upregulated metabolites. In contrast, the bacteria enriched in DCs were remarkably correlated with downregulated metabolites, reflecting a clear differential interaction pattern. This result further demonstrated a significant change in microbe-mediated metabolic patterns after the addition of CE.
To further identify more specific and sensitive markers of metabolites, we performed a more stringent threshold criteria (p < 0.001, | FC| > 4, VIP > 1) (Figure 8A). The top focus metabolites were used to perform correlation analysis with the signature microbiota (LDA > 4, p < 0.05) (Figure 8B). The results showed that Lactobacillus was significantly positively correlated with 6-hydroxy-5-methoxyindole glucuronide and negatively correlated with doxycycline and cinchonidine, while Romboutsia and Burkholderia had the opposite regulation pattern to Lactobacillus. In addition, both Enterococcus and Faecalicoccus were negatively correlated with cinchonidine, and Enterococcus was also significantly positively correlated with 6-hydroxy-5-methoxyindole glucuronide.
Figure 8. Correlation analysis was conducted between the top focus metabolites and the signature microbiota to explore the key factors influencing organismal fitness by adding CE. (A) Heat map of differential metabolites with a bar graph of VIP values from previous OPLS-DA model. Only if the metabolites were a satisfied condition of VIP > 1 were selected for the following association analysis. (B) The correlation of top focus metabolites and the signature microbiota.
Enzyme supplementation of poultry feed is of great significance in nutrition, economics, and the environment. Enzymes can improve the utilization of carbohydrates, proteins, lipids, and phytate phosphorus in feed to reduce the waste of fodder values and pollutant emissions (Douglas et al., 2000; Dosković et al., 2013). Our study showed that supplementation with multiple enzymes had no significant effect on laying performance, which contrasted with the results of previous studies. Studies by Khan et al. (2011) showed that adding 2.0 g/kg multi-enzyme preparation can increase egg production, egg weight, and egg mass; and improve the feed conversion ratio and bodyweight of layers without changing feed intake. Although enzymes have a significant impact on the performance of poultry, their application is greatly limited by the wide variety of enzymes and harsh application conditions. A previous study showed that the addition of complex enzymes (phytase, xylanase, cellulase, α-amylase, and acid-protease) had little effect on the production performance of aged hens (60–68 weeks old) but increased intestinal enzyme activity and nutrient retention (Wen et al., 2012). Interestingly, adding enzymes to low-protein and low-AME diets significantly improves hen and broiler performance and digestive enzyme activities (Zhou et al., 2009; Zhu et al., 2014; Rehman et al., 2018). Therefore, we supposed that adding multi-enzyme preparations has little impact on production performance, partly due to the balanced nutrition diet and the health status of breeding hens.
Blood biochemical indices reflect the health status of hens. CE administration increased the levels of TP and GLB in the serum. This was probably due to the adequate degradation of proteins promoted by the enzymes, which improve the absorption and utilization of amino acids in the small intestine (Al-Homidan et al., 2020). IgY is the primary serum antibody mainly distributed in poultry serum and egg yolk to protect the hens and their offspring from pathogens (Thirumalai et al., 2019). The improvement of in the titers of IgY and serum NDV and avian influenza H9 strain antibodies was associated with enhanced disease resistance. Enhanced humoral immunity was possibly related to the immune-regulatory effects of some oligosaccharides and beneficial microbiota in the gut after feeding with multiple enzymes. Additionally, CE administration significantly increased the serum HDL-C and T-AOC content and decreased the AST content. The T-AOC reflects the cumulative effect of all antioxidants in the blood and body fluids (Suresh et al., 2009; Liu et al., 2021). Breeding hens frequently face oxidative stress and ovarian aging problems in the later laying stage, which considerably affect their performance and physiology (Liu et al., 2018). AST is a sensitive marker for detecting liver injury, and high levels of AST indicate liver damage (Yousefi et al., 2005). HDL-C is considered “good cholesterol” and is associated with cardiovascular health. It can accelerate lipid migration from peripheral tissues to the liver, where cholesterol can be metabolized into bile acids (Li et al., 2018; Duan et al., 2019). Therefore, adding multiple enzymes to the diet enhances host systemic immunity, improves antioxidant capacity, and has no adverse effects on liver function.
Egg quality and reproductive performance, two important economic traits for breeding hens, tend to decrease rapidly because of the lower efficiency of absorption and immunity with age (Liu et al., 2001; Bain et al., 2016). We found that adding multiple enzymes can significantly increase albumen height and Haugh unit, indicating that the addition of multiple enzymes improves egg freshness. We then analyzed reproductive performance and found that CE administration also significantly enhanced the rate of fertilization and HoF value. The improvement of these two reproduction indexes may be related to the high-quality protein of the eggs and increased deposition of IgY in the yolk, thereby improving the reproductive performance of aged breeding hens (Thirumalai et al., 2019). In summary, CE supplementation can effectively enhance egg quality and reproductive performance of breeding hens.
To further discern the underlying mechanism of the enzyme on the productive performance and immune function of hens. The gut microbiota and metabolome after enzyme treatment were analyzed. Our results showed that adding CE had minimal effect on Chao1 and Observed species, but significantly increased Shannon and Simpson’s diversity indices. Meanwhile, the PCoA showed a clear separation between the CE and DC groups, which indicated that multiple enzymes could dramatically alter the gut microbiota with increasing microbial evenness without decreasing microbial richness (Zhang et al., 2017). Consistent with the results of previous studies, Firmicutes, Proteobacteria, and Bacteroidetes were the dominant phyla in the ileum of hens, accounting for more than 98% of the total bacteria (Pan and Yu, 2014; Liu et al., 2021). The genera Lactobacillus, Enterococcus, Streptococcus, and Faecalicoccus were the signature taxa of the CE group determined using LEfSe (LDA > 4, p < 0.05). Lactobacillus spp. contribute to intestinal health, immunity enhancement, nutrient absorption, and bile acid hydrolysis (Staley et al., 2017; Xiao et al., 2017). Enterococcus spp. are lactic acid bacteria that produce bacteriocins against pathogenic bacteria and regulate nutrient metabolism (Hanchi et al., 2018). Streptococcus spp. such as S. thermophiles and S. salivarius are often considered to have probiotic effects, which help establish intestinal immune homeostasis and regulate the inflammatory response of the host (Akpinar et al., 2011; Kaci et al., 2014). Analysis of microbial co-occurrence network patterns suggested that the addition of multi-enzymes remarkably increased the interactions between gut microbiota without affecting the number of interacting bacteria, illustrating that adding multiple enzymes enhanced the communication between bacteria. Correlation analysis of differential microbiota and metabolites demonstrated that the gut microbiota signature genera were strongly correlated with altered metabolites. Therefore, the addition of multi-enzyme modulated immune function and metabolism may be related to altering the intestinal microbiota, increasing the relative abundance of potentially beneficial bacteria, and enhancing the interaction between bacteria.
The gene function analysis of the predicted metagenomes from the DC group suggested that the microbial pathways were significantly enriched in the sporulation and biosynthesis of ansamycins. Spores can store the microbiota’s hereditary material in a harmful or unsuitable environment so that their metabolism in this state is 10 million times slower than in normally growing bacteria (Huang and Hull, 2017; Bressuire-Isoard et al., 2018). Ansamycins are antibiotics produced by several Actinomycetes strains and have an inhibitory effect on the growth of many bacteria (Vardanyan and Hruby, 2016). Bacteria inhibit the growth of their surrounding bacteria by synthesizing antibiotics to compete for limited resources, leading to a vicious cycle in the gut environment. Metabolic pathway enrichment analysis showed significant enrichment of several pathways, including glycerophospholipid metabolism, autophagy, and ferroptosis. This could be because the bacteria in the DC group lacked genes related to the degradation of harmful substances and the higher concentration of antibiotics surrounding them. Hence, bacteria may degrade their components or excess proteins through autophagy to provide nutrition for survival or directly induce ferroptosis-like death in the DC group (Deretic and Levine, 2009; Xu et al., 2019; Shen et al., 2020). Spearman correlation analysis revealed that two top-focused metabolites, doxycycline, and cinchonidine, enriched in the DC group, were positively correlated with the DC signature bacteria Romboutsia spp. and Burkholderia spp. Doxycycline, a tetracycline, has a bacteriostatic effect by inhibiting the synthesis of bacterial proteins by destroying transfer RNA and messenger RNA at ribosomal sites (Raval et al., 2018). Because doxycycline is significant for maintaining animal health and controlling vertically transmitted diseases, it has been widely used in the breeding industry (Yan et al., 2018). Studies have shown that doxycycline mainly affects the relative abundance of Firmicutes and Proteobacteria and reduces the richness and evenness of the flora (Boynton et al., 2017; Stavroulaki et al., 2021). Cinchonidine is an alkaloid found in several foods such as fruits, herbs, spices, and olives (Olea europaea) (Eyal, 2018). However, the biosynthetic pathway of cinchonidine remains unclear (Maldonado et al., 2017). Overall, the bacteria in the DC group enriched genes related to sporulation and biosynthesis of ansamycins pathways and lacked communication. The intestinal environment had a higher doxycycline content than the CE group, which would affect the microbial community structure and reduce the evenness (Stavroulaki et al., 2021).
The gene function analysis of the predicted metagenomes from the CE group suggested that the microbial pathways were significantly enriched in the biodegradation and metabolism of multiple harmful substances. Polycyclic aromatic hydrocarbons (PAHs) are widely distributed organic pollutants with genetic toxicity and carcinogenicity that can significantly interfere with gut microbiota and are associated with harmful effects on host health (Ghosal et al., 2016; Redfern et al., 2021). Ethylbenzene is a toxic aromatic organic compound metabolized by the organism, and the accumulation of xenobiotics in an organism may cause tissue damage and harm the host (Pan et al., 2020). The addition of multiple enzymes significantly enriched microbial functional genes related to the degradation of the aforementioned harmful substances, which suggested that the microbes of the CE group may have a better ability to degrade toxic organic compounds and maintain homeostasis of the gut environment to create a better intestinal environment. Meanwhile, the ileum microbiota in the CE group also enriched “glycosyltransferases” pathways, which may promote bacterial surface antigen formation, thus stimulating the host immune system and improving humoral immunity (Hong et al., 2019). Spearman correlation analysis revealed that one top-focused metabolite, 6-hydroxy-5-methoxyindole glucuronide, enriched in the CE group, was positively correlated with the CE signature bacteria Lactobacillus spp., and Enterococcus spp. 6-Hydroxy-5-methoxyindole glucuronide, a member of the glucuronide family. It is a natural metabolite of 6-hydroxy-5-methoxyindole generated in the liver by UDP glucuronyltransferase, which assists with the excretion of toxic substances, drugs, or other substances that cannot be used as an energy source (Zhao et al., 2012; Liu et al., 2019). Collectively, the addition of multiple enzymes can improve the ability of microbes to degrade harmful substances, and the potentially beneficial bacteria enriched in the CE group are closely related to the metabolite 6-Hydroxy-5-methoxyindole glucuronide that facilitates the excretion of toxic substances. Thus, CE addition can benefit hen health, possibly by affecting the metabolic function of intestinal bacteria.
Taken together, the results showed that CE supplementation may provide a nutrient-rich environment for bacteria by improving the digestion and absorption of starch and protein, elevating the excretion of toxins and harmful substances, and reshaping the structure of the ileal microbial community such that Lactobacillus spp. are the dominant bacteria and the relative abundance of common potentially beneficial bacteria, such as Enterococcus and Streptococcus, is increased. Follow-up studies are needed to ascertain the changes in the gut microbiome and metabolome induced by complex enzymes on intestinal cell function.
Overall, administration of 0.2 g/kg of dietary multi-enzyme could enhance humoral immunity and improve egg quality and reproductive efficiency together with intestinal microbial community structure and metabolite composition of aged breeding hens. Multi-enzymes could be used to enhance the immunity and reproductive performance of old breeding hens and extend their service life.
The microbial raw sequencing data and the metabolome data were deposited into the NCBI Sequence Read Archive database (SRA accession: PRJNA728385).
The animal study was reviewed and approved by Animal care and Use Committee of China Agricultural University.
ZW and ZN conceptualized the project. YL performed experiments, collected data, analyzed data, and wrote the manuscript. LQ designed studies and made insightful edits. DZ performed experiments. All authors reviewed the manuscript and approved the final submission.
This work was supported by the China Agriculture Research System (CARS-40). Funders had no participation in the study design, analysis, or writing of this article.
DZ was employed by company Huayu Agricultural Science and Technology Co., Ltd.
The remaining authors declare that the research was conducted in the absence of any commercial or financial relationships that could be construed as a potential conflict of interest.
All claims expressed in this article are solely those of the authors and do not necessarily represent those of their affiliated organizations, or those of the publisher, the editors and the reviewers. Any product that may be evaluated in this article, or claim that may be made by its manufacturer, is not guaranteed or endorsed by the publisher.
We would be deeply grateful to all of the participants involved in the study and all of the research staff and students working on the project. We would like to thank Editage (www.editage.cn) for English language editing.
Aderibigbe, A., Cowieson, A. J., Sorbara, J. O., and Adeola, O. (2020). Growth phase and dietary α-amylase supplementation effects on nutrient digestibility and feedback enzyme secretion in broiler chickens. Poult. Sci. 99, 6867–6876. doi: 10.1016/j.psj.2020.09.007
Akpinar, A., Yerlikaya, O., and Kiliccedil, S. (2011). Antimicrobial activity and antibiotic resistance of Lactobacillus delbrueckii ssp. bulgaricus and Streptococcus thermophilus strains isolated from Turkish homemade yoghurts. Afr. J. Microbiol. Res. 5, 675–682. doi: 10.5897/AJMR10.835
Al-Homidan, I., Ebeid, T., Al-Muzaini, A., Abou-Emera, O., Mostafa, M., and Fathi, M. (2020). Impact of dietary fenugreek, mung bean, and garden cress on growth performance, carcass traits, blood measurements, and immune response in broiler chickens. Livest. Sci. 242:104318. doi: 10.1016/j.livsci.2020.104318
Bain, M. M., Nys, Y., and Dunn, I. C. (2016). Increasing persistency in lay and stabilising egg quality in longer laying cycles. What are the challenges? Brit. Poult. Sci. 57, 330–338. doi: 10.1080/00071668.2016.1161727
Bolger, A. M., Lohse, M., and Usadel, B. (2014). Trimmomatic: a flexible trimmer for Illumina sequence data. Bioinformatics 30, 2114–2120. doi: 10.1093/bioinformatics/btu170
Boynton, F. D. D., Ericsson, A. C., Uchihashi, M., Dunbar, M. L., and Wilkinson, J. E. (2017). Doxycycline induces dysbiosis in female C57BL/6NCrl mice. BMC Res. Notes 10:644. doi: 10.1186/s13104-017-2960-7
Bressuire-Isoard, C., Broussolle, V., and Carlin, F. (2018). Sporulation environment influences spore properties in Bacillus: evidence and insights on underlying molecular and physiological mechanisms. FEMS Microbiol. Rev. 42, 614–626. doi: 10.1093/femsre/fuy021
Caporaso, J. G., Kuczynski, J., Stombaugh, J., Bittinger, K., Bushman, F. D., Costello, E. K., et al. (2010). QIIME allows analysis of high-throughput community sequencing data. Nat. Methods 7, 335–336. doi: 10.1038/nmeth.f.303
Cowieson, A., Lu, H., Ajuwon, K., Knap, I., and Adeola, O. (2017). Interactive effects of dietary protein source and exogenous protease on growth performance, immune competence and jejunal health of broiler chickens. Anim. Prod. Sci. 57, 252–261. doi: 10.1071/AN15523
Cowieson, A. J., and Kluenter, A. M. (2019). Contribution of exogenous enzymes to potentiate the removal of antibiotic growth promoters in poultry production. Anim. Feed Sci. Technol. 250, 81–92. doi: 10.1016/j.anifeedsci.2018.04.026
da Costa Luchiari, I., Cedeno, F. R. P., de Macêdo Farias, T. A., Picheli, F. P., de Paula, A. V., Monti, R., et al. (2021). Glucoamylase immobilization in corncob powder: assessment of enzymatic hydrolysis of starch in the production of glucose. Waste Biomass Valor. 12, 5491–5504. doi: 10.1007/s12649-021-01379-0
Dalcin, E., and Jackson, P. W. (2018). A network-wide visualization of the implementation of the global strategy for plant conservation in Brazil. Rodriguesia 69, 1613–1639. doi: 10.1590/2175-7860201869411
Deretic, V., and Levine, B. (2009). Autophagy, immunity, and microbial adaptations. Cell Host Microbe 5, 527–549. doi: 10.1016/j.chom.2009.05.016
Dosković, V., Bogosavljević-Bosković, S., Pavlovski, Z., Milošević, B., Škrbić, Z., Rakonjac, S., et al. (2013). Enzymes in broiler diets with special reference to protease. Worlds Poult. Sci. J. 69, 343–360. doi: 10.1017/S0043933913000342
Douglas, M. W., Parsons, C. M., and Bedford, M. R. (2000). Effect of various soybean meal sources and Avizyme on chick growth performance and ileal digestible energy. J. Appl. Poult. Res. 9, 74–80. doi: 10.1093/japr/9.1.74
Duan, M., Sun, X., Ma, N., Liu, Y., Luo, T., Song, S., et al. (2019). Polysaccharides from Laminaria japonica alleviated metabolic syndrome in BALB/c mice by normalizing the gut microbiota. Int. J. Biol. Macromol. 121, 996–1004. doi: 10.1016/j.ijbiomac.2018.10.087
Edgar, R. C., Haas, B. J., Clemente, J. C., Quince, C., and Knight, R. (2011). UCHIME improves sensitivity and speed of chimera detection. Bioinformatics 27, 2194–2200. doi: 10.1093/bioinformatics/btr381
Eyal, S. (2018). The fever tree: from Malaria to neurological diseases. Toxins 10:491. doi: 10.3390/toxins10120491
Fang, H., Huang, C., Zhao, H., and Deng, M. (2015). CCLasso: correlation inference for compositional data through Lasso. Bioinformatics 31, 3172–3180. doi: 10.1093/bioinformatics/btv349
Friedman, J., and Alm, E. J. (2012). Inferring correlation networks from genomic survey data. PLoS Comput. Biol. 8:e1002687. doi: 10.1371/journal.pcbi.1002687
Ghosal, D., Ghosh, S., Dutta, T. K., and Ahn, Y. (2016). Current state of knowledge in microbial degradation of polycyclic aromatic hydrocarbons (PAHs): a review. Front. Microbiol. 7:1369. doi: 10.3389/fmicb.2016.01369
Giacobbo, F. C., Eyng, C., Nunes, R. V., de Souza, C., Teixeira, L. V., Pilla, R., et al. (2021). Different enzymatic associations in diets of broiler chickens formulated with corn dried at various temperatures. Poult. Sci. 100:101013. doi: 10.1016/j.psj.2021.01.035
Gu, Y., Chen, Y., Jin, R., Wang, C., Wen, C., and Zhou, Y. (2021). A comparison of intestinal integrity, digestive function, and egg quality in laying hens with different ages. Poult. Sci. 100:100949. doi: 10.1016/j.psj.2020.12.046
Hanchi, H., Mottawea, W., Sebei, K., and Hammami, R. (2018). The genus Enterococcus: between probiotic potential and safety concerns—an update. Front. Microbiol. 9:1791. doi: 10.3389/fmicb.2018.01791
Hii, S. L., Tan, J. S., Ling, T. C., and Ariff, A. B. (2012). Pullulanase: role in starch hydrolysis and potential industrial applications. Enzyme. Res. 2012:921362. doi: 10.1155/2012/921362
Hong, S., Shi, Y., Wu, N. C., Grande, G., Douthit, L., Wang, H., et al. (2019). Bacterial glycosyltransferase-mediated cell-surface chemoenzymatic glycan modification. Nat. Commun. 10:1799. doi: 10.1038/s41467-019-09608-w
Huang, M., and Hull, C. M. (2017). Sporulation: how to survive on planet Earth (and beyond). Curr. Genet. 63, 831–838. doi: 10.1007/s00294-017-0694-7
Jing, M., Munyaka, P., Tactacan, G., Rodriguez-Lecompte, J., and House, J. (2014). Performance, serum biochemical responses, and gene expression of intestinal folate transporters of young and older laying hens in response to dietary folic acid supplementation and challenge with Escherichia coli lipopolysaccharide. Poult. Sci. 93, 122–131. doi: 10.3382/ps.2013-03384
Kaci, G., Goudercourt, D., Dennin, V., Pot, B., Doré, J., Ehrlich, S. D., et al. (2014). Anti-inflammatory properties of Streptococcus salivarius, a commensal bacterium of the oral cavity and digestive tract. Appl. Environ. Microbiol. 80, 928–934. doi: 10.1128/AEM.03133-13
Khan, S. H., Atif, M., Mukhtar, N., Rehman, A., and Fareed, G. (2011). Effects of supplementation of multi-enzyme and multi-species probiotic on production performance, egg quality, cholesterol level and immune system in laying hens. J. Appl. Anim. Res. 39, 386–398. doi: 10.1080/09712119.2011.621538
Li, Z., Kim, H. J., Park, M. S., and Ji, G. E. (2018). Effects of fermented ginseng root and ginseng berry on obesity and lipid metabolism in mice fed a high-fat diet. J. Ginseng Res. 42, 312–319. doi: 10.1016/j.jgr.2017.04.001
Liu, H.-K., Long, D., and Bacon, W. (2001). Preovulatory luteinizing hormone surge interval in old and young laying turkey hens early in the egg production period. Poult. Sci. 80, 1364–1370. doi: 10.1093/ps/80.9.1364
Liu, X., Lin, X., Mi, Y., Li, J., and Zhang, C. (2018). Grape seed proanthocyanidin extract prevents ovarian aging by inhibiting oxidative stress in the hens. Oxid. Med. Cell. Longev. 2018:9390810. doi: 10.1155/2018/9390810
Liu, X., Wang, X., Sun, H., Guo, Z., Liu, X., Yuan, T., et al. (2019). Urinary metabolic variation analysis during pregnancy and application in gestational diabetes mellitus and spontaneous abortion biomarker discovery. Sci. Rep. 9:2605. doi: 10.1038/s41598-019-39259-2
Liu, Y., Cheng, X., Zhen, W., Zeng, D., Qu, L., Wang, Z., et al. (2021). Yeast culture improves egg quality and reproductive performance of aged breeder layers by regulating gut microbes. Front. Microbiol. 12:439. doi: 10.3389/fmicb.2021.633276
Liu, Y., Li, Y., Liu, H.-N., Suo, Y.-L., Hu, L.-L., Feng, X.-A., et al. (2013). Effect of quercetin on performance and egg quality during the late laying period of hens. Br. Poult. Sci. 54, 510–514. doi: 10.1080/00071668.2013.799758
Maldonado, C., Barnes, C. J., Cornett, C., Holmfred, E., Hansen, S. H., Persson, C., et al. (2017). Phylogeny predicts the quantity of antimalarial alkaloids within the iconic yellow Cinchona Bark (Rubiaceae: Cinchona calisaya). Front. Plant. Sci. 8:391. doi: 10.3389/fpls.2017.00391
Monier, M. N. (2020). Efficacy of dietary exogenous enzyme supplementation on growth performance, antioxidant activity, and digestive enzymes of common carp (Cyprinus carpio) fry. Fish Physiol. Biochem. 46, 713–723. doi: 10.1007/s10695-019-00745-z
Nagpal, S., Singh, R., Yadav, D., and Mande, S. S. (2020). MetagenoNets: comprehensive inference and meta-insights for microbial correlation networks. Nucleic Acids Res. 48, W572–W579. doi: 10.1093/nar/gkaa254
Pan, D., and Yu, Z. (2014). Intestinal microbiome of poultry and its interaction with host and diet. Gut Microbes 5, 108–119. doi: 10.4161/gmic.26945
Pan, F., Zhang, L.-L., Luo, H.-J., Chen, Y., Long, L., Wang, X., et al. (2020). Dietary riboflavin deficiency induces ariboflavinosis and esophageal epithelial atrophy in association with modification of gut microbiota in rats. Eur. J. Nutr. 60, 807–820. doi: 10.1007/s00394-020-02283-4
Parks, D. H., Tyson, G. W., Hugenholtz, P., and Beiko, R. G. (2014). STAMP: statistical analysis of taxonomic and functional profiles. Bioinformatics 30, 3123–3124. doi: 10.1093/bioinformatics/btu494
Quast, C., Pruesse, E., Yilmaz, P., Gerken, J., Schweer, T., Yarza, P., et al. (2012). The SILVA ribosomal RNA gene database project: improved data processing and web-based tools. Nucleic Acids Res. 41, D590–D596. doi: 10.1093/nar/gks1219
Raval, J. P., Chejara, D. R., Ranch, K., Joshi, P. (2018). “Development of injectable in situ gelling systems of doxycycline hyclate for controlled drug delivery system,” in Applications of Nanocomposite Materials in Drug Delivery A volume in Woodhead Publishing Series in Biomaterials, eds Inamuddin, A. M. Asiri, and A. Mohammad (Sawston: Woodhead Publishing), 149–162.
Redfern, L. K., Jayasundara, N., Singleton, D. R., Di Giulio, R. T., Carlson, J., Sumner, S. J., et al. (2021). The role of gut microbial community and metabolomic shifts in adaptive resistance of Atlantic killifish (Fundulus heteroclitus) to polycyclic aromatic hydrocarbons. Sci. Total. Environ. 776:145955. doi: 10.1016/j.scitotenv.2021.145955
Rehman, Z., Kamran, J., Abd El-Hack, M., Alagawany, M., Bhatti, S., Ahmad, G., et al. (2018). Influence of low-protein and low-amino acid diets with different sources of protease on performance, carcasses and nitrogen retention of broiler chickens. Anim. Prod. Sci. 58, 1625–1631. doi: 10.1071/AN16687
Reyon, D., Tsai, S. Q., Khayter, C., Foden, J. A., Sander, J. D., and Joung, J. K. (2012). FLASH assembly of TALENs for high-throughput genome editing. Nat. Biotechnol. 30:460. doi: 10.1038/nbt.2170
Rognes, T., Flouri, T., Nichols, B., Quince, C., and Mahé, F. (2016). VSEARCH: a versatile open source tool for metagenomics. PeerJ 4:e2584. doi: 10.7717/peerj.2584
Romero, L., Sands, J., Indrakumar, S., Plumstead, P., Dalsgaard, S., and Ravindran, V. (2014). Contribution of protein, starch, and fat to the apparent ileal digestible energy of corn-and wheat-based broiler diets in response to exogenous xylanase and amylase without or with protease. Poult. Sci. 93, 2501–2513. doi: 10.3382/ps.2013-03789
Sarian, F. D., Janeček, Š, Pijning, T., Ihsanawati, Nurachman, Z., Radjasa, O. K., et al. (2017). A new group of glycoside hydrolase family 13 α-amylases with an aberrant catalytic triad. Sci. Rep. 7:44230. doi: 10.1038/srep44230
Scott, K. P., Gratz, S. W., Sheridan, P. O., Flint, H. J., and Duncan, S. H. (2013). The influence of diet on the gut microbiota. Pharmacol. Res. 69, 52–60. doi: 10.1016/j.phrs.2012.10.020
Segata, N., Izard, J., Waldron, L., Gevers, D., Miropolsky, L., Garrett, W. S., et al. (2011). Metagenomic biomarker discovery and explanation. Genome Biol. 12:R60. doi: 10.1186/gb-2011-12-6-r60
Shen, X., Ma, R., Huang, Y., Chen, L., Xu, Z., Li, D., et al. (2020). Nano-decocted ferrous polysulfide coordinates ferroptosis-like death in bacteria for anti-infection therapy. Nano Today 35:100981. doi: 10.1016/j.nantod.2020.100981
Staley, C., Weingarden, A. R., Khoruts, A., and Sadowsky, M. J. (2017). Interaction of gut microbiota with bile acid metabolism and its influence on disease states. Appl. Microbiol. Biotechnol. 101, 47–64. doi: 10.1007/s00253-016-8006-6
Stavroulaki, E., Suchodolski, J. S., Pilla, R., Fosgate, G. T., Sung, C.-H., Lidbury, J. A., et al. (2021). Short-and long-term effects of amoxicillin/clavulanic acid or doxycycline on the gastrointestinal microbiome of growing cats. bioRxiv [Preprint]. doi: 10.1101/2021.05.28.446115
Suresh, D. R., Annam, V., Pratibha, K., and Prasad, B. V. M. (2009). Total antioxidant capacity – a novel early bio-chemical marker of oxidative stress in HIV infected individuals. J. Biomed. Sci. 16:61. doi: 10.1186/1423-0127-16-61
Thirumalai, D., Ambi, S. V., Vieira-Pires, R. S., Xiaoying, Z., Sekaran, S., and Krishnan, U. (2019). Chicken egg yolk antibody (IgY) as diagnostics and therapeutics in parasitic infections–A review. Int. J. Biol. Macromol. 136, 755–763. doi: 10.1016/j.ijbiomac.2019.06.118
Tomasik, P., and Horton, D. (2012). Enzymatic conversions of starch. Adv. Carbohydr. Chem. Biochem. 68, 59–436. doi: 10.1016/b978-0-12-396523-3.00001-4
Vardanyan, R., and Hruby, V. (2016). “Chapter 30 – Antibiotics,” in Synthesis of Best-Seller Drugs, eds R. Vardanyan and V. Hruby (Boston, MA: Academic Press), 573–643.
Walk, C., Pirgozliev, V., Juntunen, K., Paloheimo, M., and Ledoux, D. (2018). Evaluation of novel protease enzymes on growth performance and apparent ileal digestibility of amino acids in poultry: enzyme screening. Poult. Sci. 97, 2123–2138. doi: 10.3382/ps/pey080
Wen, C., Wang, L., Zhou, Y., Jiang, Z., and Wang, T. (2012). Effect of enzyme preparation on egg production, nutrient retention, digestive enzyme activities and pancreatic enzyme messenger RNA expression of late-phase laying hens. Anim. Feed Sci. Technol. 172, 180–186. doi: 10.1016/j.anifeedsci.2011.11.012
Wohlgemuth, G., Haldiya, P. K., Willighagen, E., Kind, T., and Fiehn, O. (2010). The CHEMICAL TRANSLATION SERVICE—a web-based tool to improve standardization of metabolomic reports. Bioinformatics 26, 2647–2648. doi: 10.1093/bioinformatics/btq476
Xiao, Y., Xiang, Y., Zhou, W., Chen, J., Li, K., and Yang, H. (2017). Microbial community mapping in intestinal tract of broiler chicken. Poult. Sci. 96, 1387–1393. doi: 10.3382/ps/pew372
Xu, Y., Zhou, P., Cheng, S., Lu, Q., Nowak, K., Hopp, A.-K., et al. (2019). A bacterial effector reveals the V-ATPase-ATG16L1 axis that initiates xenophagy. Cell 178, 552–566.e20. doi: 10.1016/j.cell.2019.06.007
Yan, Q., Li, X., Ma, B., Zou, Y., Wang, Y., Liao, X., et al. (2018). Different concentrations of doxycycline in swine manure affect the microbiome and degradation of doxycycline residue in soil. Front. Microbiol. 9:3129. doi: 10.3389/fmicb.2018.03129
Yin, D., Yin, X., Wang, X., Lei, Z., Wang, M., Guo, Y., et al. (2018). Supplementation of amylase combined with glucoamylase or protease changes intestinal microbiota diversity and benefits for broilers fed a diet of newly harvested corn. J. Anim. Sci. Biotechnol. 9:24. doi: 10.1186/s40104-018-0238-0
Yousefi, M., Shivazad, M., and Sohrabi-Haghdoost, I. (2005). Effect of dietary factors on induction of fatty liver-hemorrhagic syndrome and its diagnosis methods with use of serum and liver parameters in laying hens. Int. J. Poult. Sci 4, 568–572. doi: 10.3923/ijps.2005.568.572
Zhang, M., Liu, X., Li, Y., Wang, G., Wang, Z., and Wen, J. (2017). Microbial community and metabolic pathway succession driven by changed nutrient inputs in tailings: effects of different nutrients on tailing remediation. Sci. Rep. 7:474. doi: 10.1038/s41598-017-00580-3
Zhao, T., Zhang, H., Zhao, T., Zhang, X., Lu, J., Yin, T., et al. (2012). Intrarenal metabolomics reveals the association of local organic toxins with the progression of diabetic kidney disease. J. Pharm. Biomed. Anal. 60, 32–43. doi: 10.1016/j.jpba.2011.11.010
Zhou, Y., Jiang, Z., Lv, D., and Wang, T. (2009). Improved energy-utilizing efficiency by enzyme preparation supplement in broiler diets with different metabolizable energy levels. Poult. Sci. 88, 316–322. doi: 10.3382/ps.2008-00231
Keywords: multi-enzyme, aged layers, immunity, reproduction performance, microbiome, metabolome
Citation: Liu Y, Zeng D, Qu L, Wang Z and Ning Z (2021) Multi-Enzyme Supplementation Modifies the Gut Microbiome and Metabolome in Breeding Hens. Front. Microbiol. 12:711905. doi: 10.3389/fmicb.2021.711905
Received: 19 May 2021; Accepted: 04 November 2021;
Published: 03 December 2021.
Edited by:
Haidong Yao, Karolinska Institutet (KI), SwedenReviewed by:
Lisa Osborne, University of British Columbia, CanadaCopyright © 2021 Liu, Zeng, Qu, Wang and Ning. This is an open-access article distributed under the terms of the Creative Commons Attribution License (CC BY). The use, distribution or reproduction in other forums is permitted, provided the original author(s) and the copyright owner(s) are credited and that the original publication in this journal is cited, in accordance with accepted academic practice. No use, distribution or reproduction is permitted which does not comply with these terms.
*Correspondence: Zhong Wang, d2FuZ3poQGNhdS5lZHUuY24=; Zhonghua Ning, bmluZ3poaEBjYXUuZWR1LmNu
Disclaimer: All claims expressed in this article are solely those of the authors and do not necessarily represent those of their affiliated organizations, or those of the publisher, the editors and the reviewers. Any product that may be evaluated in this article or claim that may be made by its manufacturer is not guaranteed or endorsed by the publisher.
Research integrity at Frontiers
Learn more about the work of our research integrity team to safeguard the quality of each article we publish.