- 1Department of Civil and Environmental Engineering, The University of Utah, Salt Lake City, UT, United States
- 2Carl R. Woese Institute for Genomic Biology, The University of Illinois at Urbana-Champaign, Urbana, IL, United States
- 3Departamento de Producción Vegetal y Microbiología, Universidad Miguel Hernández, Alicante, Spain
Viruses play vital biogeochemical and ecological roles by (a) expressing auxiliary metabolic genes during infection, (b) enhancing the lateral transfer of host genes, and (c) inducing host mortality. Even in harsh and extreme environments, viruses are major players in carbon and nutrient recycling from organic matter. However, there is much that we do not yet understand about viruses and the processes mediated by them in the extreme environments such as hypersaline habitats. The Great Salt Lake (GSL) in Utah, United States is a hypersaline ecosystem where the biogeochemical role of viruses is poorly understood. This study elucidates the diversity of viruses and describes virus–host interactions in GSL sediments along a salinity gradient. The GSL sediment virosphere consisted of Haloviruses (32.07 ± 19.33%) and members of families Siphoviridae (39.12 ± 19.8%), Myoviridae (13.7 ± 6.6%), and Podoviridae (5.43 ± 0.64%). Our results demonstrate that salinity alongside the concentration of organic carbon and inorganic nutrients (nitrogen and phosphorus) governs the viral, bacteria, and archaeal diversity in this habitat. Computational host predictions for the GSL viruses revealed a wide host range with a dominance of viruses that infect Proteobacteria, Actinobacteria, and Firmicutes. Identification of auxiliary metabolic genes for photosynthesis (psbA), carbon fixation (rbcL, cbbL), formaldehyde assimilation (SHMT), and nitric oxide reduction (NorQ) shed light on the roles played by GSL viruses in biogeochemical cycles of global relevance.
Introduction
Viruses are the most abundant biological entities (Bergh et al., 1989; Fuhrman, 1999; Suttle, 2005; Nooij et al., 2018; Graham et al., 2019) which infect all forms of known cellular life. Viruses of bacteria and archaea act as a repository of genetic information that influences the evolution and ecophysiology of their host. Each viral infection event can introduce new genetic information into the host genome (Suttle, 2005). Viral infections are a major source of host mortality altering microbial community composition and thus affecting the ecosystems fluxes of nutrients and energy (Weinbauer and Rassoulzadegan, 2004; Rodriguez-Brito et al., 2010; Cram et al., 2016). Viruses hijack cellular machinery of their hosts for viral replication and propagation, while reprogramming the host’s cellular metabolism (Forterre, 2013; Rosenwasser et al., 2016). Viruses can act as agents for the horizontal transfer of ecologically important genes (Jiang and Paul, 1998). Viruses also encode AMGs, host derived genes that are expressed during infection to re-direct hosts metabolism toward pathways that promote viral productivity (Sullivan et al., 2006; Hurwitz and U’Ren, 2016). These genes, AMG, are involved in various metabolisms, including sulfur, nitrogen, and methane, in DNA replication, repair, recombination, and amino acid biosynthesis, and are also detected in extreme environments (Anantharaman et al., 2014; Anderson et al., 2014; Ahlgren et al., 2019). AMGs interfering with carbon fixation and methylotrophy metabolism have been detected in viruses from the lake Baikal (Coutinho et al., 2020). AMGs encoding photosynthetic reaction centers in cyanophages highlight their potential to boost photosynthetic metabolism (Sullivan et al., 2006; Lindell et al., 2007). Cyanophages from the marine environment have also been observed with genes for CP12 (Thompson et al., 2011). The expression of CP12 in the cyanophages’ host causes the switching of carbon flux from the Calvin cycle to the pentose phosphate pathway (PPP). Such AMGs exemplify how viruses can affect biogeochemical cycles of global relevance in ways which beyond killing of their hosts.
Saline lakes, which globally display similar volume (1.04 × 105 km3) as freshwater lakes (1.25 × 105 km3) (Horne and Goldman, 1994), are traditionally deemed of little significance and understudied as they are not easily monetized (Wurtsbaugh et al., 2017). Nevertheless, these saline habitats are critical, have critical roles in the regulation of climate, and global geochemistry (Hoehler et al., 2001; Daffonchio et al., 2006; Sternai et al., 2017). The GSL in the United States represents such an ecosystem. The GSL is the largest terminal saline lake in the Western Hemisphere (Keck and Hassibe, 1979). Between 1955 and 1959, a railway causeway was constructed on the GSL, dividing the lake into north and south arm (Madison, 1970; Cannon and Cannon, 2002; Baxter et al., 2005). The north arm of GSL receives no to little freshwater and displays salinity levels between 28 and 34%. The south arm is fed by different tributaries and displays salinity between 11 and 15% (Baxter et al., 2005; Almeida-Dalmet et al., 2015). Terminal lakes without any water discharge outlet have a higher degree of susceptibility to climate change with varying cycles of drought and flooding (Wurtsbaugh et al., 2017). In the GSL, the rise of lake water level, as high as four meters, between 1983 and 1987 was observed, followed by drought events resulting in recorded lowest elevation (since 1963) recorded in 2016 (Stephens, 1990). The fluctuations in GSL water levels lead to salinity changes that affect the resident microbial community (Wu et al., 2006; Herlemann et al., 2011; Logares et al., 2013).
The microbial community in the northern arm of the GSL has been shown to be less impacted by fluctuations in temperature and salinity (Almeida-Dalmet et al., 2015). In contrast, the lesser saline southern arm has been shown to harbor a diverse and dynamic microbial community as it receives fresh streamflow from the Bear, Weber, and Jordan rivers (Meuser et al., 2013; Boyd et al., 2014; Lindsay et al., 2017; Baxter and Butler, 2020). Although characterization of microbial community and their metabolism in the GSL has advanced, diversity of viruses and their ecological significance in the southern arm of the GSL has still not been explored in detail. Viral studies in the GSL are limited to identifying Haloviruses and their role in infecting halophilic bacteria and archaea (Post, 1981; Baxter et al., 2011; Shen et al., 2012). Recently, Motlagh et al. (2017) provided an insight into GSL sediment virosphere, which was shown to encode genes for nitrogen, carbon, and sulfur cycling (Motlagh et al., 2017). However, the impact of salinity on the viral community and their interaction with the host community of bacteria and archaea remains underexplored. Furthermore, little is known about the diversity of AMGs in hypersaline ecosystems and its potential impacts in biogeochemical cycles of global relevance.
Here, we delineate the bacterial, archaeal, and viral diversity of GSL sediments. To our knowledge, this is the first study of the viral community along the north-south salinity gradients in the GSL. We hypothesize that across the GSL, the diversity of bacteria, archaea, and viruses reduces with an increase in salinity. The objectives of our study were to (a) delineate the viral diversity of GSL, an extreme environment, and (b) elucidate the role of hypersaline viruses in nutrient cycling. Viral, bacterial, and archaeal metagenomes were obtained from three GSL sediments sites along the lake’s north-south transect. From these samples we recovered and characterized viral, bacterial, and archaeal draft genomes for the genome-based ecology study. Viruses were assigned to their putative bacterial and archaeal hosts through computational approaches. In addition, AMGs were identified among the viruses from GSL and their potential ecological roles were characterized.
Materials and Methods
Sample Collection and Measurement of Sediment and Water Quality Parameters
Three sampling sites were chosen in Gilbert Bay and Carrington Bay (southern arm) of GSL on the north to south transect covering a salinity gradient (Figure 1). The three sites on the GSL were (i) CB2, 12.3 miles North-West of Carrington Island (41°10′32.3″ N, 112°39′11.9″ W); (ii) GSL 3510, 6 miles west of Antelope Island (40°53′56″ N, 112°20′56″ W); and (iii) GB-14, 6.8 miles north of Great Salt Lake Marina (40°49′06.2″ N, 112°14′13.6″ W). The samples were collected on 10th August 2017. The flow of high-density brine from the northern arm to the southern arm through the causeway fill material and culverts causes denser brine to settle in the deepest section, thus creating a deep brine layer in the GSL. The deep brine water sample was collected ∼0.5 m from the bottom of the GSL using a peristaltic pump following the United States Geological Survey’s (USGS) and GSL monitoring program water sampling standard operating procedure (SOP) in a 1L HDPE sampling bottle (Wilde, 2011). The tube was cleaned with dilute hydrochloric acid and deionized water three times to remove any previous sampling carryover. Sediment samples were collected from the surface of the GSL sediments with a stainless-steel box corer (Wildco, FL, United States) as performed in Motlagh et al. (2017). The collected samples were immediately placed inside a storage box with ice and shipped to the laboratory within 4 h of sample collection.
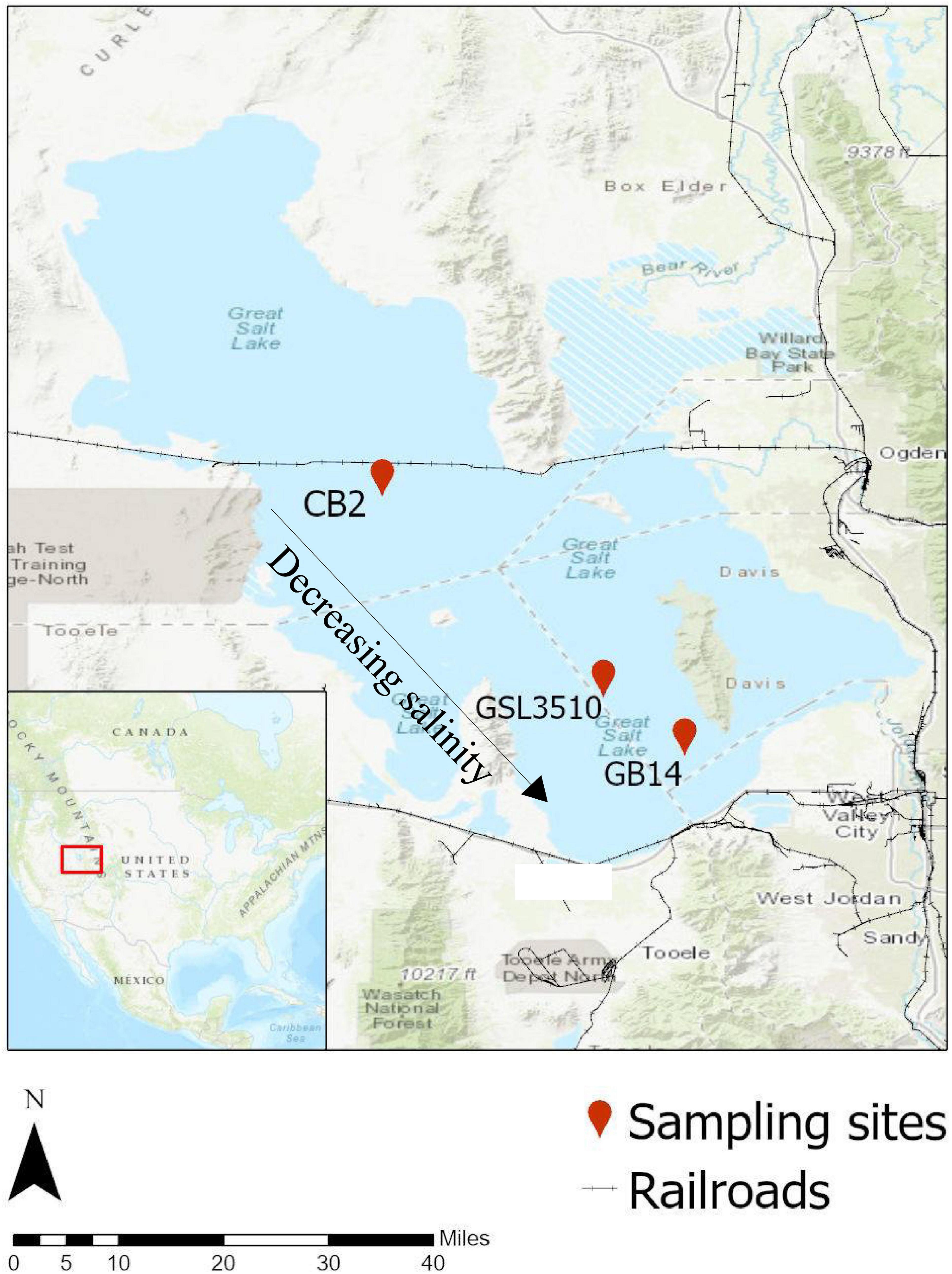
Figure 1. Location of study areas in the Great Salt Lake (GSL). The three sites are (i) CB-2, 12.3 miles North-West of Carrington Island (41°10′32.3″ N, 112°39′11.9″ W); (ii) GSL 3510, 6 miles West of Antelope Island (40°53′56.0″ N, 112°20′56.0″ W); and (iii) GB-14, 6.8 miles North of Great Salt Lake Marina (40°49′06.2″ N, 112°14′13.6″ W). Source: Background Map (Topographic Base Map, ESRI 2020).
Temperature, pH, specific conductivity, ORP, depth, and DO were measured using an onsite sounder equipped with various sensors (YSI 600 XL). The sediment samples were centrifuged at 2,000 × g for 10 min to extract the interstitial water as pore water. The surface water and the extracted pore water were filtered through 0.45 μm mixed cellulose hydrophilic filter paper before further analyses (Millipore, MA, United States). The concentration of ammonium (NH4-N), nitrite (NO2-N), nitrate (NO3-N), orthophosphate (PO4-P), and TOC were measured for both the surface and pore water. Ammonium (NH4-N) and TOC were measured using the HACH methods TNT830 and HACH 10128, respectively, according to the manufacturer’s instructions (HACH, CO, United States). Nitrite (NO2-N), nitrate (NO3-N), and orthophosphate (PO4-P) were analyzed using Ion Chromatography (IC) (Metrohm 883 Basic IC plus) (Pfaff, 1993).
Preparation of Viral Concentrates and Viral Nucleic Acid Extraction
The viral concentrates were prepared according to the protocol used by Motlagh et al. (2017) for hypersaline sediments. For each site, 300 g of sediments were mixed with three times the volume of autoclaved and filtered 1% (w/v) potassium citrate buffer (10 g/l potassium citrate, 1.44 g/l of disodium phosphate, 0.24 g/l of monopotassium phosphate, pH 7). The mixture was placed on a shaker at 160 rpm on ice overnight to detach the free viruses from the sediment mass and bring them into the solution. Following the overnight shaking, the sample mixture was centrifuged at 7800 × g at 4°C on an Avanti J-E centrifuge (Beckman Coulter, CA, United States) in J-LITE®JLA-10.500 Fixed-Angle Aluminum Rotor for 45 min to pellet down the bacterial debris as well as the sediments. The retained supernatant was then sequentially filtered through various pore-sized membrane filter units (mixed cellulose ester filters; 1.2 μm, 0.8 μm, 0.45 μm, 0.22 μm) to avoid any contamination from microbial cells. The filtrate was centrifuged overnight at 12,300 × g at 4°C to pellet the virus particles. The pellet was re-suspended in SMG buffer (5.8 g/l sodium chloride, 2 g/l magnesium sulfate, 5 ml/l of 5% (w/v) gelatin, 50 ml/l of 1M Tris-Cl, pH 7.5) and filtered through 0.22 μm pore size filter paper (Millipore Co., MA, United States) to remove any residual bacterial cell and sediment debris.
The viral particles in the SMG buffer were purified using cesium chloride (CsCl) density gradient at 1.4–1.6 g/cc density by isopycnic centrifugation at 35,000 rpm, 20°C for 3 h (Angly et al., 2006). The cesium chloride purification was carried out twice to ensure the highest removal of any residual bacterial cell debris and other particles, thereby guaranteeing extracted viral particles’ purity.
The purified virus samples were dialyzed overnight through dialysis tubing with a nominal MWCO of 3500 at 4°C against SMG buffer. 10 μL of the CsCl purified virus samples were loaded on Formvar/Carbon 400 mesh grids and allowed to settle for a minute. The sample was blotted out using bibulous paper, and the grids were negatively stained with 1% (w/v) uranyl acetate for 1 min. Excess staining liquid was removed using a bibulous paper. Before examining under JEOL JEM-1400 TEM (Tokyo, Japan), the grid was air-dried at an accelerating voltage of 120 kV.
DNA was extracted from purified viral particles based on a spin column purification method using a phage DNA isolation kit (Norgen Biotek Corp., Canada). The viral sample was subjected to DNase treatment with RNase-free DNase I (Thermo Scientific, CA, United States) at 37°C for 30 min, followed by the DNase I inactivation at 75°C for 5 min before the lysis process to remove any residual microbial DNA and digest any free DNase in the sample. Since it is difficult to obtain a high yield of viral DNA from environmental samples, 4 μl of Proteinase K (20 mg/mL) (Promega, Madison, WI, United States) was added to the sample incubated at 55°C for 30 min to increase viral DNA yield. The extracted DNA’s quantity and quality were checked on a NanoDrop ND 2,000 spectrophotometer (Thermo Scientific, United States) at 260 and 280 nm. PCR amplification of the hypervariable V4-V9 region was performed using 515/1492R universal 16S rRNA gene primer set as a test to confirm the absence of microbial DNA contamination in the viral DNA extract (Diemer and Stedman, 2012). The PCR products were electrophoresed in a 1% agarose gel stained with ethidium bromide (10 μg/ml) and visualized under UV illumination (Molecular Imager Gel Doc XR+, BIO-RAD).
Genomic DNA Extraction From Microbes
The genomic DNA was extracted from 0.5 g of sediment samples according to the manufacturer’s instructions using DNeasy PowerSoil Kit (QIAGEN). For each site. the genomic DNA was extracted in triplicates and later pooled together. Similar to viral nucleic acid, microbial genomic DNA concentration was measured using NanoDrop ND 2,000 spectrophotometer (Thermo Scientific, United States).
DNA Library Preparation and Sequencing
For both the microbial and viral DNA samples, library construction was performed using the Swift Biosciences Accel-NGS 1S Plus DNA Library Kit. Briefly, around 50 ng of genomic DNA was heat-denatured and hybridized with oligonucleotides consisting of random hexamers linked to Illumina P5 adapter sequences. Strand replication was accomplished using EpiGnome polymerase. Double-stranded DNA was heat-denatured to enable ligation of the EpiGnome Terminal Tagging Oligo, which adds Illumina P7 adapter sequence to the 3′ end of the replicated strand. Adapter-ligated DNA molecules were enriched by ten cycles of PCR, and the amplified library was subsequently purified using Agencourt AMPure XP beads (Beckman Coulter Genomics, CA, United States). The library’s concentration was measured using the Qubit dsDNA HS Assay (Invitrogen, CA, United States). An aliquot of the library was resolved on an Agilent 2200 Tape Station using a D1000 assay to define the sequencing library’s size distribution. Libraries were adjusted to a concentration of approximately 10 nM, and quantitative PCR was performed using the Kapa Library Quant Kit (Kapa Biosystems, MA, United States) to calculate the molarity of adapter-ligated DNA molecules. The concentration was further adjusted following qPCR to prepare the library for Illumina sequence analysis. The samples were sequenced on an Illumina MiSeq Benchtop DNA sequencer (Illumina, CA, United States) with 300-cycles paired-end at Core Facility, HCI, University of Utah. This study attempts to avoid applying any amplification process to minimize biases for the virus metagenomics and its analysis.
Metagenome Sequence Processing and Analysis
Quality control, assembly, and gene prediction for both metagenome and metavirome datasets were performed simultaneously using the same pipeline. The raw reads generated by the Illumina MiSeq sequencer were first checked for quality using FastQC v0.11.8 (Andrews, 2010) and then trimmed using Trimmomatic v0.38 (Bolger et al., 2014). The read-through adapters (ILLUMINACLIP: Swift-PE.fa:2:30:10), low-quality base calls at the start and end of reads (LEADING:3, TRAILING:3), reads with an average Phred score lower than 20 in a sliding window of 4 bp (SLIDINGWINDOW:4:20) were trimmed from the raw reads. Also, sequencing reads less than 100 bp were discarded from further analyses (MINLEN:100).
The quality-controlled reads were de novo assembled using metaSPAdes v3.13.0 (Bankevich et al., 2012) with a range of kmer values (21, 33, 55, 77, 99, 127), and the best-assembled scaffolds, as reported by SPAdes, were chosen. Only the contigs longer than 1 kbp were retained and referred to as ‘RMC’ and ‘RVC’ for the microbial and viral dataset, respectively (Supplementary Figure S2).
Taxonomic Classification and Open Reading Frame Prediction of Viral, Bacterial, and Archaeal Sequences
Viral contigs were identified in the RMC and RVC using VirSorter (Roux et al., 2015). “Viromes” database- a combination of RefSeqABVir sequences and virome sequences sampled from freshwater, seawater, and human gut- was used as a reference database allowing for the detection of new viruses (Roux et al., 2015). For each of the three sites, viral contigs identified within the RMC and RVC were grouped and named as ‘CVC’ from here onward. Similarly, ‘CMC’ consisted of RMC with viral contigs removed. Supplementary Figure S2 shows the schematic workflow of the abovementioned procedure. The relative percentage abundance of CVCs was obtained by mapping the reads from both metagenome and metavirome datasets for individual sites. NCBI RefSeq Viral database and Integrated Microbial Genome/Virus (IMG/VR) database (Paez-Espino et al., 2019) filtered for viral genomes identified in freshwater, marine, saline, and hypersaline environments were used for taxonomic annotation of viral genomes. The RefSeq Viral database and the filtered IMG/VR database are referred to as RVG and EVG databases. The viral contigs were annotated for taxonomic classification against the RVG and EVG using tblastx v.2.9.0 (e-value 10–5) and the best blast hit was selected using enveomics (Rodriguez-R and Konstantinidis, 2016). The lifestyle of the viruses based on the conserved protein domains were predicted using BACPHLIP (Hockenberry and Wilke, 2021). The bacterial and archaeal communities were profiled using Metaphlan version 2.7.7 (Truong et al., 2015) using default parameters. ORFs were identified in the CMC and CVC using Prodigal v2.6.3 (Hyatt et al., 2010). ORFs were annotated using SUPER-FOCUS (e-value 10–5) (Silva et al., 2016). The functional profile was constructed by mapping the trimmed reads to the predicted ORFs using BBMap (Bushnell, 2014).
Binning of Bacterial and Archaeal Contigs and Phylogenetic Analysis
The RMCs were binned into draft bacterial and archaeal genomes. First, the mean coverage of contigs was calculated by mapping the quality-controlled reads to the RMCs using Bowtie2 (Langmead and Salzberg, 2012). Then, using default parameters in MetaBAT2 (Kang et al., 2019), microbial and archaeal contigs were binned into draft metagenome-assembled genomes (MAGs). The recovered MAGs were profiled and checked for quality (genome completeness and contamination) using CheckM v1.0.7 (Parks et al., 2015). MAGs with contamination greater than 5% were manually refined in Anvi’o using differential coverage, tetranucleotide frequency, and marker gene content (Eren et al., 2015). Post refinement of the MAGs, the phylogenetic markers, and relative abundance of the MAGs was assessed using ‘tree_qa’ and ‘profile’ commands, respectively, in CheckM (Parks et al., 2015). The taxonomy of the MAGs was also determined using the Genome Taxonomy Database Toolkit (GTDB-Tk) (Chaumeil et al., 2019). The MAGs with contamination less than 5% were placed in the reference tree inferred from the concatenation of 43 conserved marker genes using CheckM v1.0.7 (Parks et al., 2015). The reference tree was annotated to distinguish different ‘Class’ levels and identify the MAGs from different sites in the GSL using CLC Genomics Workbench v.12 (CLC Bio, Denmark). The branches of the reference tree represent ‘Class’ taxonomy. Each ‘Class’ was denoted with individual color. The branches with the GSL MAGs were expanded to show their taxonomic position in the phylogenetic tree. The MAGs from individual sites in the GSL are distinguished by different symbols. The MAGs were annotated using Prokka v1.14.6 (e-value 10–5) (Seemann, 2014). The annotated proteins for selected MAGs were parsed through MicrobeAnnotator (Ruiz-Perez et al., 2020) to check for the genome’s metabolic potential based on KEGG modules.
Computational Host Prediction of Viral Sequences
From the CMCs, clustered regularly interspaced short palindromic repeats (CRISPR) arrays were predicted with CASC, the CRISPR detection and validation tool (Nasko et al., 2019). The number of unique and shared spacers was determined by clustering the ‘bonafide’ spacer sequences at 97% nucleotide similarity using CD-HIT-EST (Li and Godzik, 2006). The correct spacers were used as query sequences to search against the CVCs, RVG, and EVG using Blastn (word size 7). The blastn hits were filtered using specific parameters (minimum alignment length: 15 bp; minimum percentage identity: 90%; minimum query coverage: 95%; evalue ≤ 0.1; maximum one nucleotide mismatch) to be considered a significant hit. Bacterial and archaeal contigs were annotated against the NCBI non-redundant nucleotide database using tblastx v.2.9.0.
Viruses were also assigned to their putative hosts by comparing the shared genetic content between viruses and hosts described in Coutinho et al. (2020). Bacterial and Archaeal genomes from the NCBI RefSeq database were used as reference genomes. GTDB was used to assign taxonomy to the reference genomes. CRISPR spacers (three points), homology matches (two points), and shared tRNAs (one point) were analyzed as virus–host association signals as described in Coutinho et al. (2020).
Data Analysis
The data analysis and visualizations in this study were conducted using Microsoft Excel, Python 3, and Origin 2020b. The phylogenetic tree was produced using CLC Genomics Workbench (CLC Bio, Denmark).
Results
Water Quality and Sediment Characteristics
Water chemistry parameters (temperature, pH, specific conductivity, ORP, DO) were measured onsite in three sampling locations at the Great Salt Lake (Table 1 and Supplementary Figure S1). The surface and sediment pore-water’s water quality parameters (inorganic nitrogen, phosphorus content, and organic carbon) were measured and summarized in Table 1. Salinity at sites CB2, GSL3510, and GB14 (Figure 1) was measured to be 17.59% (175.9 ppt), 12.14% (121.4 ppt), and 11.66% (116.6 ppt), respectively, demonstrating salinity differences across the sampled sites. Further, variability in DO in the deep layer of the overlying water column was observed with the oxygen-rich zone in GB14 (DO: 6.84 mg/L), oxygen deficiency in GSL 3510 (DO: 0.44 mg/L), and anoxia in CB2 (DO ∼ 0 mg/L) (Table 1). In terms of carbon and nutrients availability, sediments from site CB2 consisted of a higher concentration of TOC, nitrogen (N), and phosphorus (P) than GSL3510 and GB14 sites (Table 1). The extracted sediment pore water had higher TOC, ammonical-nitrogen (NH4-N), and phosphate-phosphorous (PO4-P) concentration than the overlying water column at all GSL sites (Table 1).
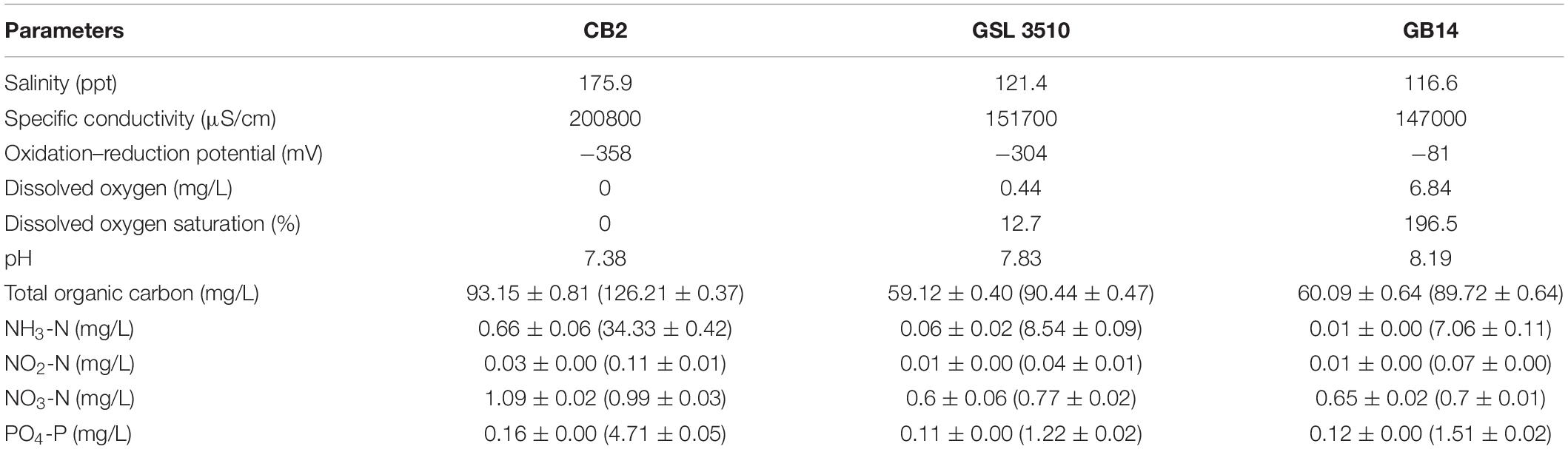
Table 1. Environmental properties determined for sediments, pore water (included within parentheses), and lake water sampled on 10th August 2017 from three locations in the Great Salt Lake.
Morphological Diversity of Viruses in Great Salt Lake Sediments
The purified viral particles observed under a TEM show tailed and non-tailed viral morphologies of the GSL sediments (Figure 2A). The tailed morphologies belong to the order Caudovirales, tailed viruses that infect bacteria and archaea, which are prevalent in hypersaline environments (Sencilo and Roine, 2014). Spherical, head-tail, and lemon-shaped viral morphologies were also observed. The morphological characterization, however, cannot establish the taxonomic identity of the viruses. Therefore, genomic analysis was incorporated to elucidate the viral diversity of hypersaline GSL.
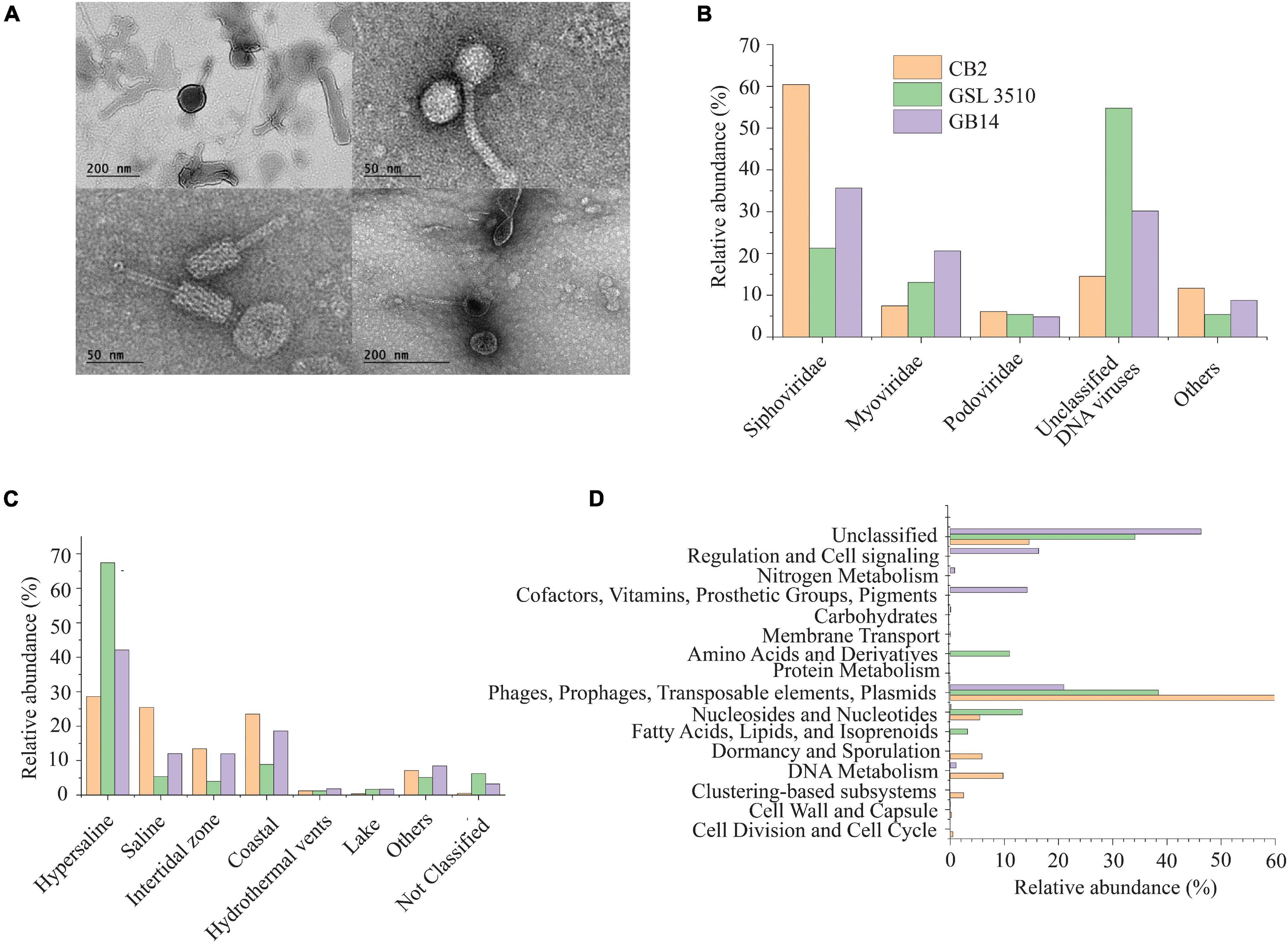
Figure 2. (A) Morphological diversity of the viruses in the Great Salt Lake sediments observed via the transmission electron microscope (TEM). (B) Family level viral community composition from the Great Salt Lake sediments. (C) Ecosystem-based community composition of viruses from the Great Salt Lake sediments based on the similarity with viruses identified in different aquatic ecosystems (IMG/VR database). (D) Functional classification (SEED subsystem Level I) of the viral open reading frames (ORFs).
Bioinformatic Analysis of Bacterial, Archaeal, and Viral Metagenomes
Following the quality trimming, an average of 95.92% metagenomic reads and 96.87% metavirome reads were retained (Supplementary Table S1). The assembly statistics and the number of ORFs detected from each site is shown in Supplementary Table S2.
From both metagenomes and metaviromes assembly, VirSorter (Roux et al., 2015) identified a total of 3,295 contigs (∼1 to 74.42 kbp) as viral and 21 contigs as prophages (∼9.68 to 1784.23 kbp). The number of VirSorter (Roux et al., 2015) identified viruses and prophages from both the metagenomes and metaviromes dataset in each site is described in Supplementary Table S3. The contigs identified as viral contigs by VirSorter were referred to as viral genomes. A total of 578, 81, and 41 viral contigs from CB2, GSL3510, and GB14 had sizes greater than 5 kbp.
Taxonomic and Functional Diversity of Bacterial and Archaeal Community Along the Salinity Gradient in the Great Salt Lake Sediments
The taxonomic profiling with MetaPhlAn v2.7.7 (Truong et al., 2015) revealed that 19.60, 89.74, and 34.56% of the quality filtered reads were respectively assigned to archaea in samples CB2, GSL3510, and GB14 while remaining ones were assigned bacteria. More than half of the quality-filtered reads were taxonomically classified to be Euryarchaeota, followed by Proteobacteria in sediment samples across three sites in GSL (Figure 3A). Bacterial and archaeal sequences belonging to genus Thioalkalivibrio, Halorubrum, Methanohalophilus, Halobacterium, and family Halanaerobiaceae were identified at the three GSL sites (Supplementary Figures S4D,E). Archaea belonging to the Haloarcula genus was found to be in sediments of CB2 and GSL 3510. Genus Halanaerobium, Marinobacter, Halomonas, Haloquadratum, and Nodularia, which were not found in GSL 3510 and GB14, were identified in CB2. Similarly, the genus Gillisiae was only identified at site GSL 3510. The Shannon diversity index of the bacterial and archaeal community across the GSL was calculated at the species level. The Shannon diversity index (H) of site CB2 was the highest (H = 0.89), followed by GSL 3510 (H = 0.74) and GB14 (H = 0.42). In the GSL, bacterial and archaeal diversity represented by Shannon index (H) is in congruence with decreasing salinity. This is contrasting as hypersaline environments are constrained in bacterial and archaeal diversity due to extreme environmental conditions; an increase in salinity leads to decreased bacterial and archaeal diversity (Ventosa et al., 2014; Ji et al., 2019).
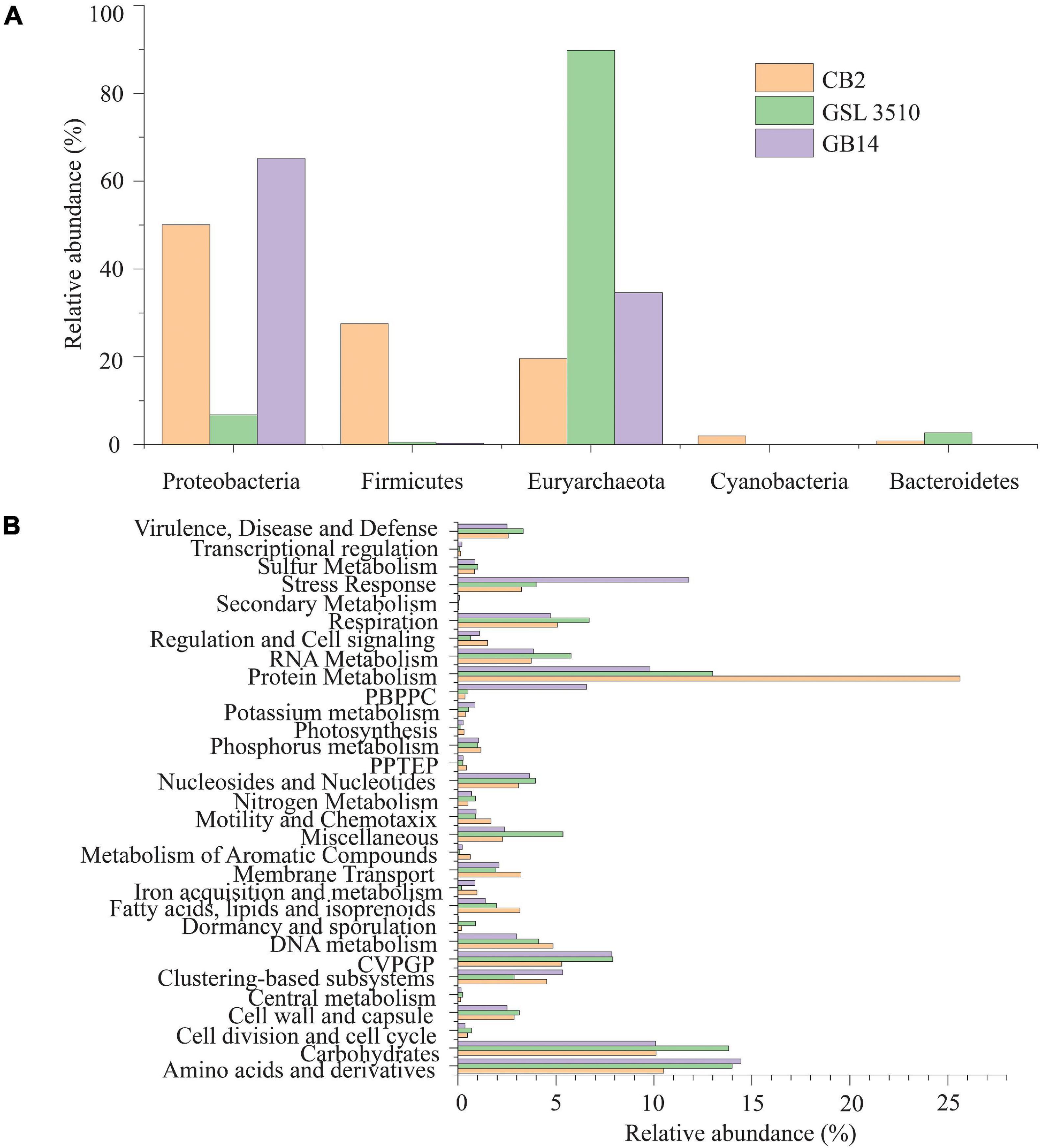
Figure 3. (A) Phylum level classification of the bacterial and archaeal community across the Great Salt Lake sediments. (B) Functional classification (SEED subsystem Level I) of the bacterial and archaeal open reading frames (ORFs). (PPTEP, phages, prophages, transposable elements, plasmids; CVPGP, cofactors, vitamins, prosthetic groups, pigments; PBPPC, predictions based on plant-prokaryote comparative analysis).
The predicted genes of the contigs were queried against the SEED subsystem database to predict the community’s metabolic potential. The relative abundances of the microbial sequences that had similarities to the SEED subsystem categories are depicted in Figure 3B. In GSL, a maximum number of predicted genes were affiliated to protein metabolism, SEED system category (Figure 3B). Amino acids and derivatives and carbohydrates comprised 12.97 ± 2.15 and 11.34 ± 2.15% (mean ± SD), respectively, of the genes. Genes affiliated to stress response were also identified among the contigs. Interestingly, 11.78% of predicted ORFs of GB14 (salinity 11.66%) were affiliated to stress response, greater than the other two study areas (Figure 3B). The bacterial and archaeal stress factors may not be solely attributed to salinity gradients. Gene for sulfur and nitrogen metabolism were identified for the bacteria and archaea involved in the GSL biogeochemical cycles.
Bacterial and Archaeal Metagenome-Assembled Genomes of the Great Salt Lake
A total of 95 MAGS were extracted from the bacterial and archaeal metagenomes of the GSL. Check M v1.0.7 (Parks et al., 2015) was used for the quality assessment of the MAGs. Seventy-six out of the ninety-five MAGs had contamination <5%. The MAGs with contamination ≥5% (19) were manually curated following the MAG refine protocols described in the “Materials and Methods” section. The curation of the contaminated MAGs via splitting resulted in the retention of 33 MAGS with less than 5% contamination. A total of 43 MAGs had completeness greater than 50% and thus were selected for phylogenetic analysis. The genome statistics of the 43 MAGs selected for phylogenetic analysis are shown in Table 2. The genome statistics for the MAGs with contamination <5% and completeness <50% (66) is shown in Supplementary Table S4. The phylogenetic position of the selected MAGs is shown in Figure 4. The relative abundance of the reconstructed MAGs was low (Figure 5A) because only a small proportion of reads (33.9 ± 4.61%) could be mapped back to the MAGs.
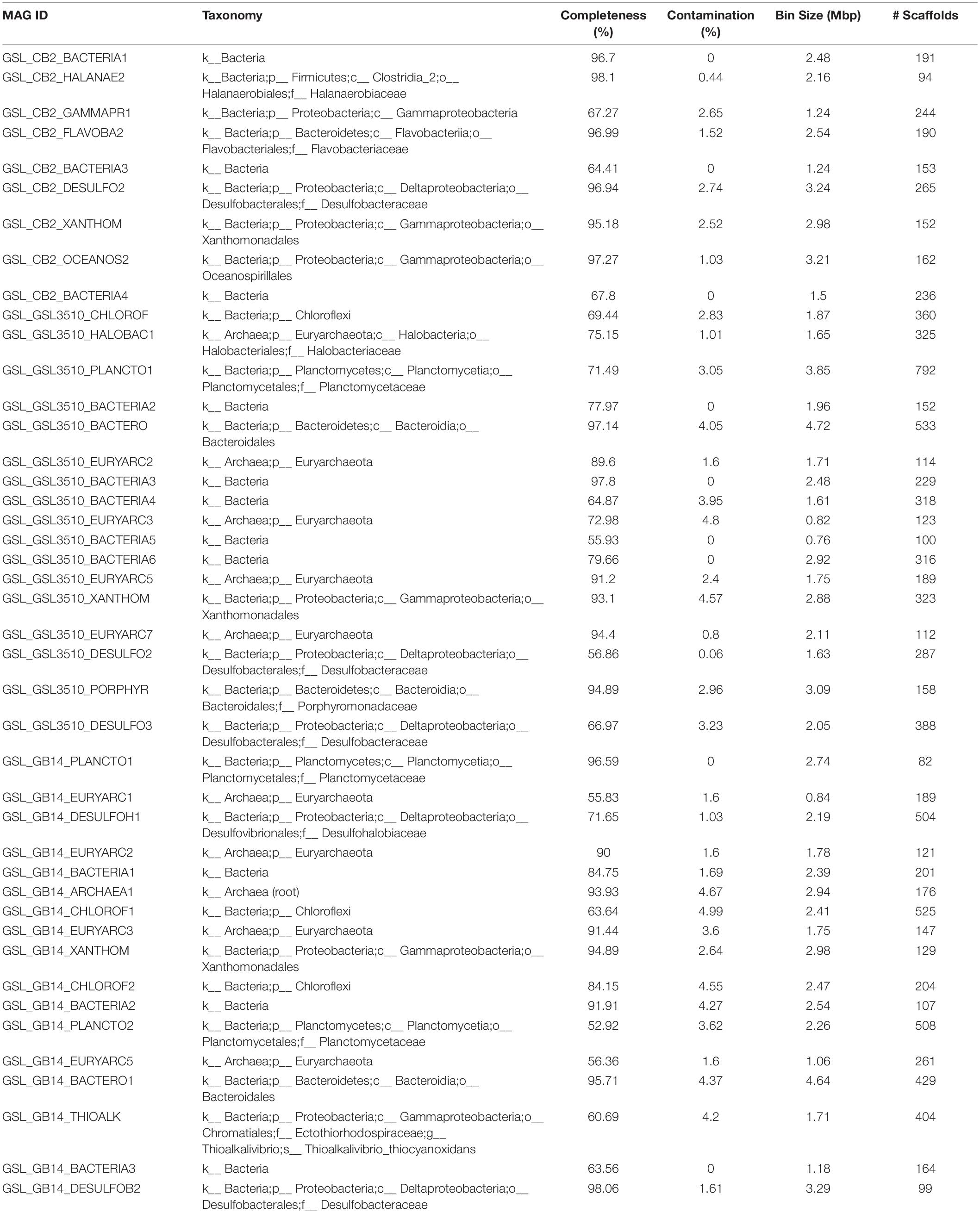
Table 2. Genome statistics of MAGs from the Great Salt Lake (Utah, United States) selected for phylogenetic analysis (completeness ≥ 50%).
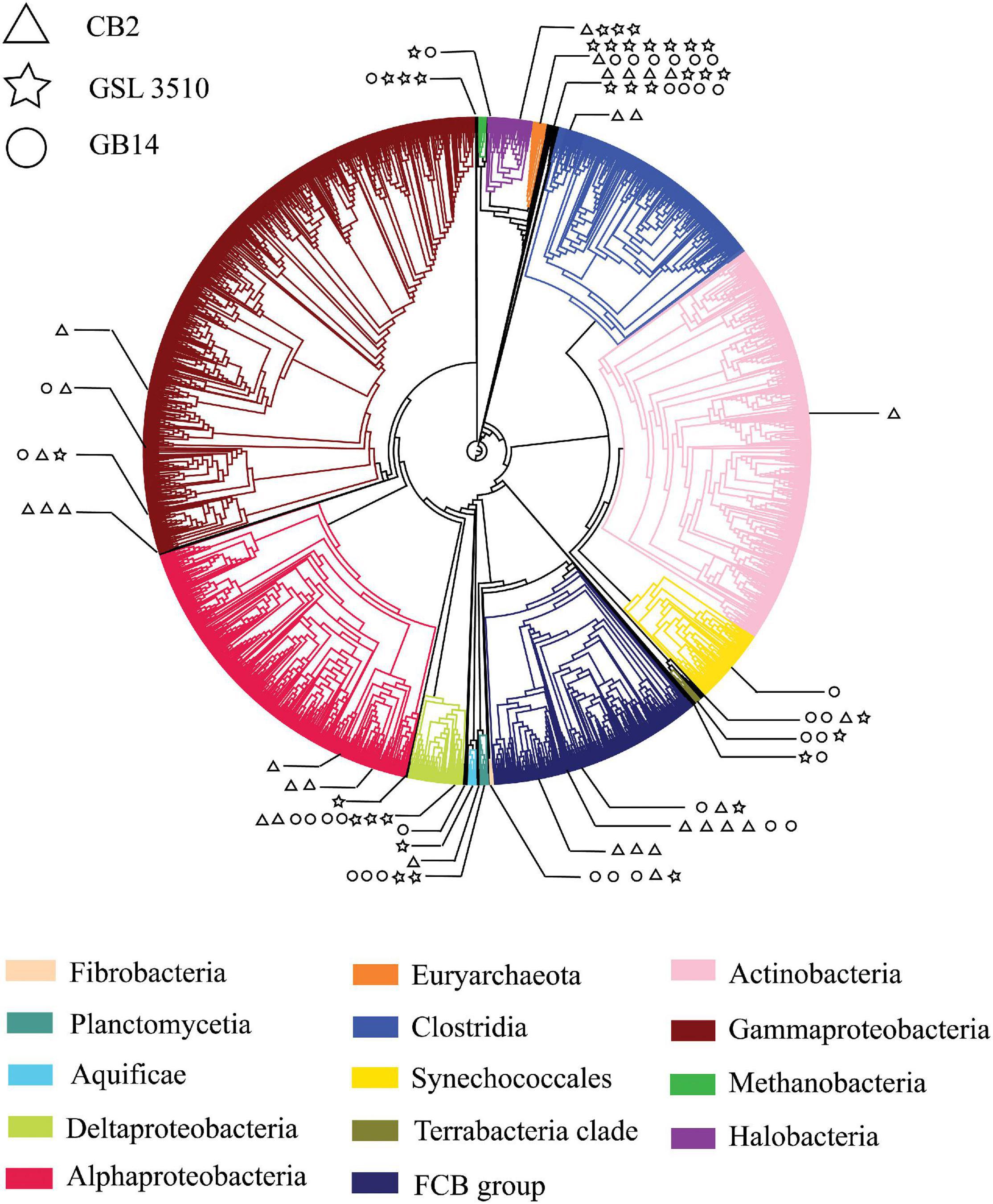
Figure 4. A phylogenetic tree of Great Salt Lake metagenome-assembled genomes (MAGs). The MAGs with contamination (>5%) assessed by CheckM v1.0.7 (Parks et al., 2015) were placed in the reference phylogenetic tree inferred with 43 conserved marker genes using CheckM v1.0.7 (Parks et al., 2015). The phylogenetic tree was annotated with CLC Genomics Workbench v.12 (CLC Bio, Denmark). The branch of the reference tree represents ‘Class,’ taxonomy. Each ‘Class’ is denoted with individual color. The branches with Great Salt Lake MAGs are expanded to show their taxonomic position on the phylogenetic tree. The Great Salt Lake MAGs from three sites on the Lake are distinguished with different symbols.
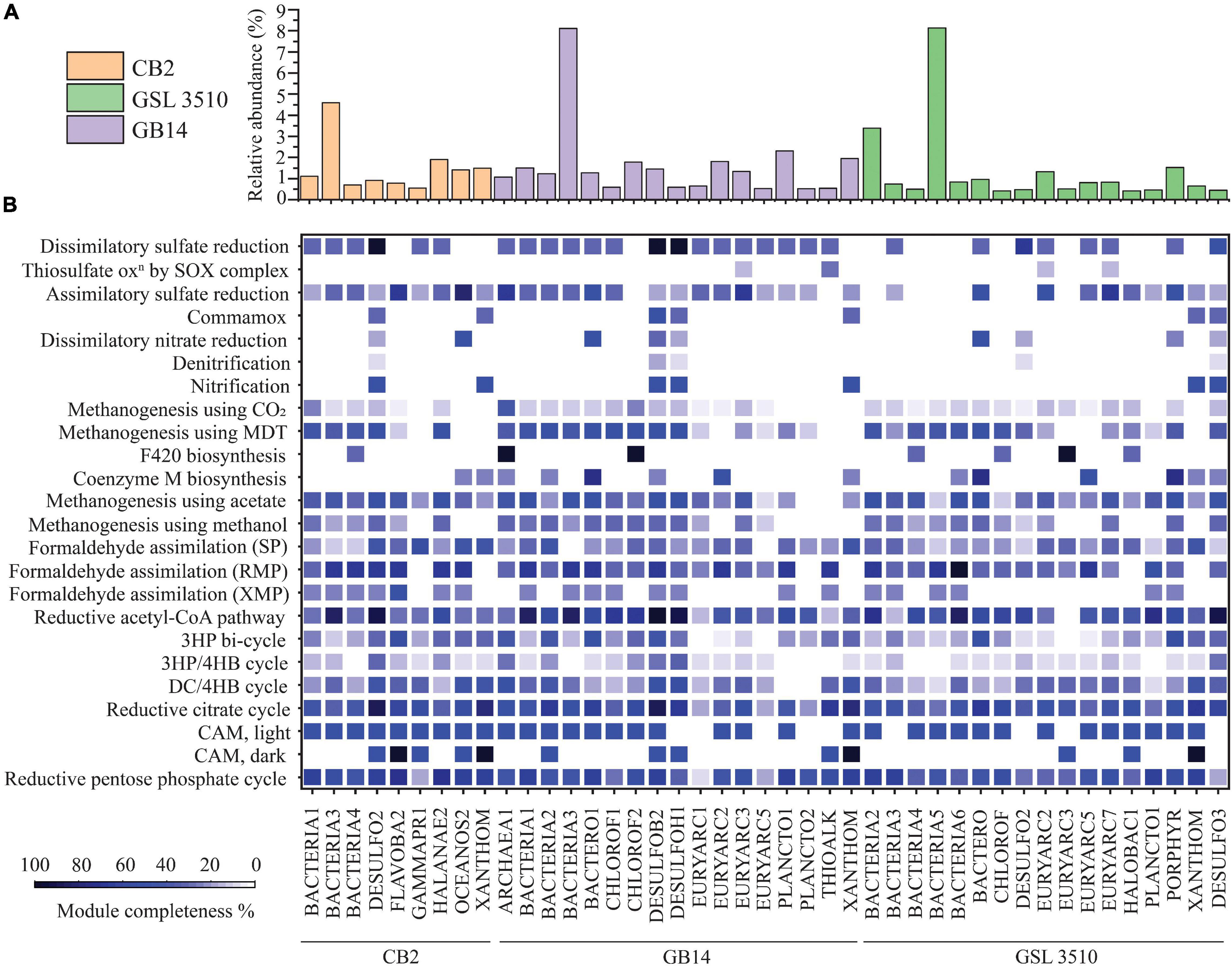
Figure 5. (A) The relative abundance of MAGs across the Great Salt Lake sediments is shown as bar graph. (B) Heatmap representing the percentage completion of KEGG metabolic modules of MAGs selected for phylogenetic analysis (completeness ≥ 50%, contamination < 5%). (MDT, methylamine–dimethylamine–trimethylamine; SP, serine pathway; RMP, ribulose monophosphate pathway; XMP, xylulose monophosphate pathway; 3HP, 3-hydroxypropionate; 3HP/4HB, 3-hydroxypropionate/4-hydroxybutyrate; DC/4HB, dicarboxylate/4-hydroxybutyrate cycle; CAM, Crassulacean acid metabolism).
Prokka v 1.14.6 (Seemann, 2014) was used for gene/ORF prediction and annotation of the 109 refined MAGs. The metabolism of the MAGs was assessed based on the completeness of different metabolic pathways (KEGG Pathway). The nitrogen, sulfur, methane, and carbon fixation pathways were studied in detail for 43 MAGs selected for phylogenetic analysis to elucidate the nutrient cycling in the GSL (Figure 5B). The findings are presented in the following sections.
Carbon Fixation
Ten GSL MAGs showed a substantially complete (>70% complete) reductive acetyl-CoA module (Wood–Ljungdahl pathway) across the GSL (Figure 5B). At all three sites, MAGs of Desulfobacteraceae (CB2_DESULFO2, GSL3510_DESULFO3, GB14_DESULFOB2) were observed to take part in the reductive acetyl-CoA pathway. Similarly, a MAG classified as member of Desulfohalobiaceae from site GB14 (GB14_DESULFOH1) and another MAG classified as member of Planctomycetaceae in site GSL 3510 (GSL3510_PLANCTO1) contained more than 70% complete reductive acetyl-CoA pathway (Figure 5B). Only two MAGs assigned to Flavobacteriaceae (CB2_FLAVOBA2) and Halanaerobiaceae (CB2_HALANAE2) from site CB2 showed a substantially complete module (≥70%) for the reductive pentose phosphate cycle (Calvin–Benson–Bassham cycle). Although the reductive pentose phosphate cycle is the predominating CO2 fixation pathway globally (Wolfe and McBride, 1971; Pride et al., 2003; Bergmann et al., 2005), the anoxia within the sediments of the GSL supports the reductive acetyl-CoA pathway. These results suggest that the reductive acetyl-CoA pathway may be the primary pathway for carbon fixation in the GSL sediments.
One Carbon Metabolism
Metagenome-assembled genomes of Chloroflexi (GB14_CHLOROF1, GB14_CHLOROF2) contained at least half of the required methanogenesis genes using acetate and methylamine–dimethylamine–trimethylamine substrate, respectively (Figure 5B). MAGs of Desulfobacteraceae (CB2_DESULFO2, GB14_DESULFOB2), Desulfohalobiaceae (GB14_DESULFOH1), Bacteroidales (GB14_BACTERO1, GSL3510_BACTERO), Halanaerobiaceae (CB2_HALANAE2) contained genes for methanogenesis using both acetate and methylamine–dimethylamine–trimethylamine (Figure 5B). MAGs of Chloroflexi (GB14_CHLOROF2) and Euryarchaeota (GB14_ARCHAEA1) contained enzymes for F420 synthesis, an essential catabolic cofactor in methanogens. Similarly, MAGs of Bacteroidales (GB14_BACTERO1, GSL3510_BACTERO), Euryarchaeota (GB14_EURYARC2, GSL3510_EURYARC5), and Porphyromonadaceae (GSL3510_PORPHYR) contained genes coding for coenzyme M biosynthesis. Coenzyme M plays a vital role in methanogenesis by functioning as a C1 carrier Wolfe and McBride, 1971).
Metagenome-assembled genomes of Desulfobacteraceae (CB2_DESULFO2, GB14_DESULFOB2), Flavobacteriaceae (CB2_FLAVOBA2), Halanaerobiaceae (CB2_HALANAE2), Oceanospirillales (CB2_OCEANOS2), Bacteroidales (GB14_BACTERO1), Euryarchaeota (GB14_EURYARC2, GSL3510_EURYARC5), Planctomycetaceae (GB14_PLANCTO1) contained more than two-thirds of genes responsible for formaldehyde assimilation via the ribulose monophosphate pathway. None of the MAGs showed a substantial complete module (>50%) for formaldehyde assimilation via serine and xylulose monophosphate pathways (Figure 5B).
Sulfur Metabolism
The GSL sediments harbor a diverse population of sulfate reducers. Desulfobacteraceae at sites CB2 and GB14 and Desulfohalobiaceae at site GB14 are dissimilatory sulfate reducers of GSL. Some MAGs of Desulfobacteraceae (CB2_DESULFO2, GB14_DESULFOB2) and Desulfohalobiaceae (GB14_DESULFOH1) contained all the necessary genes for a dissimilatory sulfate reduction lifestyle (Figure 5B). In addition, Desulfobacteraceae (GSL3510_DESULFO2), sulfate reducer from the site GSL 3510, have genes of dissimilatory sulfate reduction pathway (66.67% of KEGG module completion). The MAGs of Flavobacteria (CB2_FLAVOB2) and Oceanospirillales (CB2_OCEANOS2) from the site CB2 and Euryarchaeota from the sites GSL 3510 (GSL3510_EURYARC2, GSL3510_EURYARC7) and GB14 (GB14_EURYARC3) have at least half of the genes for assimilatory sulfate reduction. MAGs of Bacteroidales (GSL3510_BACTERO, GB14_BACTERO1) and Porphyromonadaceae (GSL3510_PORPHYR) also contained substantially complete modules for assimilatory sulfate reduction. MAGs of Euryarchaeota and Thioalkalivibrio contained few genes for thiosulfate oxidation (<50% complete KEGG modules) (Figure 5B).
Nitrogen Metabolism
Bacteria and archaea are involved in nitrogen cycling connect carbon, phosphorous, and sulfur metabolism. Nitrogen metabolizing bacteria and archaea have been shown to be present in the GSL ecosystem (Motlagh et al., 2017). Multiple phylogenetically classified refined MAGs from the GSL possessed genes for nitrification (7), denitrification (5), and dissimilatory nitrate reduction (9) (Figure 5B).
The Xanthomonadales MAGs were found across GSL sediments. MAGs of Xanthomonadales (CB2_XANTHOM, GSL3510_XANTHOM, GB14_XANTHOM) contained hao gene but lack genes that encode ammonia mono-oxygenase subunits for nitrification. Sulfate reducers Desulfobacteraceae (CB2_DESULFO2, GB14_DESULFOB2, GSL3510_DESULFO3) and Desulfohalobiaceae (GB14_DESULFOH1) have a hao gene that encodes a potentially functional HAO protein. The HAO protein is not involved in nitrification but a result of horizontal gene transfer (Bergmann et al., 2005; Figure 5B). A MAG of Desulfobacteraceae (GB14_DESULFOB2) additionally showed a nitrite oxidoreductase gene for converting nitrite to nitrate. MAGs of Bacteroidales and Oceanospirillales had half of the genes required for dissimilatory nitrate reduction. MAGs of Bacteroidales (GB14_BACTERO1; GSL3510_BACTERO) contained nrfA and nrfH genes, while MAG of Oceanospirillales (CB2_OCEANOS2) was detected with nirB and nirD genes.
The absence of genes can also be due to the incomplete construction of MAGs.
Viral Ecology of the Great Salt Lake Sediments
The metavirome analysis showed that the viruses belonging to the Siphoviridae family of order Caudovirales were dominant across the GSL (39.12 ± 19.8%) (Figure 2B). Nearly 60.42% of the viruses at the anoxic site CB2 in the GSL belonged to the Siphoviridae family. Viruses assigned to Myoviridae (13.7 ± 6.6%) and Podoviridae (5.43 ± 0.64%) were the other dominant families within GSL viromes. Siphoviridae, Myoviridae, and Podoviridae are dominant viral families in the aquatic ecosystems (Gong et al., 2018; Kallies et al., 2019). One-third (33.15 ± 20.3%) of the total viruses across the GSL were categorized as unclassified DNA viruses (Figure 2B). At the site GSL 3510, over half (54.77%) of the total viruses were unclassified DNA viruses. This is due to the under representation of taxonomically classified viruses from hypersaline ecosystem in reference databases such as GenBank or RefSeq. Haloviruses, viruses that infect halophilic archaea, were found in the GSL sediments. The Haloviruses at sites CB2, GSL 3510, and GB14 comprised 14.23%, 52.6%, 29.39%, respectively, of total viral abundance. Viruses of genus Peduovirus were detected at two sites, GSL 3510 (5.54%), GB14 (11.2%). Additionally, Nonlabens virus P12024L were also recognized at three sites, CB2 (6.65%), GB14 (1.76%) and GSL 3510 (0.3%).
Comparison of IMG/VR database (Paez-Espino et al., 2019) showed that 67.41 and 42.12% of viruses from the sites GSL 3510 and GB14 had been previously identified in hypersaline environments (Figure 2C). Saline viruses were also observed in sediments from GSL 3510 and GB14, 5.38 and 12.02%, respectively. At site CB2, the abundance of viruses from hypersaline and saline, 28.57 and 25.44%, respectively, were similar (Figure 2C).
Post metavirome assembly with SPAdes v3.13.0 (Bankevich et al., 2012) and Prodigal v2.6.3 (Hyatt et al., 2010) for gene/ORF prediction and annotations, we used SUPER-FOCUS (Silva et al., 2016) for functional prediction. As anticipated, for viral genomes, a significant proportion of the predicted viral ORF annotations were phages, prophages, transposable elements, plasmids (Figure 2D). Genes of the stress response were also identified within the viral ORFs. The stress response genes included genes encoding cold shock proteins and sigmaB stress response regulation. The ORFs identified from the CVCs (Supplementary Figure S2) were also annotated with Prokka v1.14.6 (Seemann, 2014). Genome map of the viral genomes with auxiliary metabolic genes (AMGs) are shown in Supplementary Figure S3. AMGs psbA; rbcL, cbbL; gene encoding SHMT and nitric oxide reductase NorQ were identified across the GSL. Viruses in CB2 contained psbA gene for the Photosystem II module and rbcL, cbbL gene involved in reductive pentose phosphate cycle (Calvin cycle) for carbon fixation. Gene encoding SHMT was observed in viral genomes from CB2 and GSL 3510 sediments. SHMT is an enzyme involved in the assimilation of formaldehyde into intermediates of central metabolic pathways. Gene encoding nitric oxide reductase NorQ protein was observed in viral genomes from GSL 3510 sediments. NorQ is a membrane protein required in denitrification (Kahle et al., 2018).
Based on the lifestyle assessment of the viruses using BACPHLIP (Hockenberry and Wilke, 2021), a total of 68 viruses were identified as lysogenic out of 3316 viral genomes (Supplementary Table S9). These predictions should be interpreted with caution because many sequences are incomplete genomes which can underestimate which viruses are lysogenic.
Identification of Lysogenized Metagenome-Assembled Genomes in the Great Salt Lake Sediments
Contigs within the refined MAGs were checked for viral signal through Virsorter (Roux et al., 2015). A total of 43 and 103 contigs of viral origin were identified within 28 and 11 MAGs, respectively, extracted from the RMC and RVC (Supplementary Figure S2). The MAGs with viral contigs were referred as lysogens. The lysogens in the GSL accounted for 17.52 (±3.75)% of the bacterial and archaeal community (Supplementary Figure S5). The taxonomic lineage of the lysogenic MAGs was obtained with CheckMv1.0.7 (Parks et al., 2015). The taxonomic affiliation of contigs of viral origin was determined by results of tBLASTx using reference sequence database for viruses (RefSeq-viral database), National Center for Biotechnological Information (NCBI) (accessed on September 2020). Supplementary Table S5 shows the putative lysogenic virus–host associations identified.
Flavobacterium virus FCL2, Nonlabens virus P12024L, and Pseudomonas virus KPP25 were associated with bacterial MAGs of family Flavobacteriaceae from site CB2. Similarly, viruses of family Siphoviridae and Podoviridae were associated with bacterial MAGs of the order Bacteroidales from site GSL 3510 and GB14 (Supplementary Table S5). MAG of family Planctomycetaceae was observed to form a lysogenic association with the Myoviridae family virus (Supplementary Table S5). Across the GSL, 14.45%, 16.41%, 21.7% of the refined MAGs, respectively, were lysogenic in sites CB2, GSL 3510, and GB14 (Supplementary Figure S5). Additionally, the viruses’ bacterial and archaeal hosts were also identified with the Virus–Host database (Mihara et al., 2016).
The RVCs were binned into genomes with metaBAT v2.12.1 (Kang et al., 2019). The MAGs were checked if they contained contigs of viral origin. A total of 11 MAGs (Supplementary Table S6) contained viral genomes within it, and thus they were also termed lysogenic MAGs. Viruses of genus Hapunavirus, Peduovirus, Lessievirus, Kleczkowskavirus, and Detrevirus formed a lysogenic association with MAGs of genus Pseudomonas. Viruses belonging to Podoviridae, Escherichia virus mEpX2, and Siphoviridae were found in the MAG genus Stenotrophomonas. Virus belonging to genus Salterprovirus was associated with the MAG of genus Microbacterium.
CRISPRs of Great Salt Lake Bacterial and Archaeal Contigs
A total of 8, 16, and 21 valid CRISPR arrays with 29, 79, and 109 spacer sequences were predicted in bacterial and archaeal contigs of the cellular fraction from CB2, GSL 3510, and GB14 (Supplementary Figure S6). All the spacer sequences were unique at 97% nucleotide level similarity.
The CRISPR spacers identified in bacterial and archaeal sequences were linked to the viral genomes with BLASTn. Thirty-one putative virus–host associations were established (minimum percentage identity: 90% with length ≥ 15 bp, maximum mismatch of one nucleotide, and e-value of 0.1) (Supplementary Table S7). The CRISPR spacer identified in a contig of Marinilabilia from phylum Bacteroidetes matched the virus belonging to the family Siphoviridae with 100% query coverage. This was the only association with 100% query coverage. The average query coverage was low (49.29 ± 12.45%, mean ± SD). The spacer sequences were also matched with the reference viral genome (RVG) and environmental viral genome (EVG) databases (see the section “Taxonomic Classification and Open Reading Frame Prediction of Viral, Bacterial, and Archaeal Sequences”). A total of twenty-three virus–host associations were determined against the RVG database but no associations exhibiting query coverage greater than 95% were observed (average query coverage: 58.43 ± 9.31%). CRISPR spacers in the bacterial and archaeal sequences matched with viruses from marine ecosystems (Supplementary Figure S7).
Bacterial and Archaeal Host of the Viruses From the Great Salt Lake
Bacterial and Archaeal hosts for 151 putative GSL viruses were assigned to bacterial and archaeal genomes in the NCBI RefSeq database based on (a) homology matches, (b) shared tRNAs, and (c) CRISPR spacers (Supplementary Table S8). The majority of predicted hosts were Bacteria (142) with few Archaea (9). Among the 151 predicted virus–host pairings, Proteobacteria (54.3%), Firmicutes (16.56%), and Actinobacteria (15.89%) were the dominant hosts of the GSL viruses. Other hosts of the putative viruses were Euryarchaeota (8), Bacteroidetes (3), Acidobacteria (2), Fusobacteria (2), Tenericutes (2), Crenarchaeota (1), Chlorobi (1), Chloroflexi (1).
Discussion
Microbial and Viral Community Composition in the Great Salt Lake Is Driven by Nutrients
Spherical, head-tail, and lemon-shaped viral morphotypes have been previously identified in hypersaline environments such as solar salterns and salt flats (Garcia-Heredia et al., 2012; Santos et al., 2012; Ramos-Barbero et al., 2019). Lemon-shaped viruses are generally known to infect archaea, while spherical and head-tail viruses can infect bacteria and archaea (Atanasova et al., 2015). Viruses of family Siphoviridae, Myoviridae, and Podoviridae order Caudovirales are dominant among GSL sediments (Figure 2B). The GSL viral communities are similar to the viruses of hypersaline ponds/Lakes with varying salinity (8 to 36%) (Roux et al., 2016; Motlagh et al., 2017). A notable proportion of viral genomes (33.15 ± 20.3%) were identified as unclassified DNA viruses, presumably because the available database lacks a comprehensive catalog of viruses from hypersaline environments. The limitation is thought to conceal the viral diversity in the less studied extreme ecosystems such as the GSL (Atanasova et al., 2015; Dávila-Ramos et al., 2019; Ramos-Barbero et al., 2019). The current study elucidates the virosphere from underrepresented hypersaline GSL, an extreme environment. This study’s viral diversity will add to the growing public repositories of viral genome databases and facilitate hypersaline virus research.
Great Salt Lake sites GSL 3510 and CB2, with a salinity of 12.14% and 17.59% (Table 1), respectively, harbors viruses of saline and hypersaline environments. More than two-thirds (72.79%) of the total viral abundance at site GSL 3510 are known saline and hypersaline viruses (Figure 2C). At the site CB2, a little over half (54.01%) of the total viruses are known to be from saline and hypersaline environments (Figure 2C). Although the salinity increases between the GSL sites, GSL 3510 and CB2, the abundance of previously known viruses from saline and hypersaline environments decreases. The GSL site CB2 harbors viruses that previously were not known to be in hypersaline environments.
Viruses from saline and hypersaline environments are known as Haloviruses (Emerson et al., 2012; Santos et al., 2012). These constituted one-third (32.07 ± 19.33%) of the total viral community across the GSL. Haloviruses infect halophilic archaea (Atanasova et al., 2015). However, the abundance of Haloviruses of GSL does not change with increased salinity across the Lake (Figure 2B). The GSL site GSL 3510, with a salinity of 12.14%, harbors Haloviruses. More than half (52.6% abundance) of the total virosphere at site GSL 3510 are Haloviruses. Whereas the GSL site CB2, with a high salinity of 17.59%, has Haloviruses but less abundant (14.23%). The Haloviruses abundance across the GSL is in congruence with its host, halophilic archaea. At the GSL sites GSL 3510 and CB2, the halophilic archaeal abundance was 89.74 and 19.6%, respectively, of the total bacterial and archaeal abundance (Supplementary Figure S4A).
In the GSL ecosystem, the abundance of Haloviruses and their host, halophilic archaea, are not in congruence with the salinity gradient. This contrasts with Roux et al. (2016) findings that across hypersaline environments, the abundance of Haloviruses and their archaeal hosts increase with salinity (Roux et al., 2016). Salinity alone does not affect the viral, bacterial, and archaeal diversity across the hypersaline GSL. In addition to salinity, inorganic nutrients and available organic carbon affect bacterial and archaeal diversity. Among three GSL sites, site CB2 is rich in nutrients (Table 1) and has high salinity. This site harbors a diverse [Shannon diversity index (H), 0.89] bacterial and archaeal community. The availability of nutrients as organic carbon and inorganics supports a diverse viral, bacterial, and archaeal community at the GSL site CB2. Our results suggest that the viral, bacterial, and archaeal community composition in the southern arm of the GSL along the salinity gradient is primarily influenced by concentrations of organic carbon and inorganic nutrients, while salinity is a secondary factor.
Reductive Acetyl-CoA Pathway Is the Primary Carbon Fixation Pathway in the Great Salt Lake Sediments
Our study found ten MAGs including those classified as Desulfobacteraceae, Desulfohalobiaceae, Planctomycetaceae, encoding genes involved in the reductive acetyl-CoA, or WL pathway in GSL sediments (Figure 5B). In all three sites, most of the genomes with substantially complete reductive acetyl-CoA pathway had genes encoding for CO dehydrogenase/acetyl-CoA synthase (Figure 5B). CO dehydrogenase/acetyl-CoA synthase is a critical enzyme in the WL pathway to reduce CO2 to CO and synthesize acetyl-CoA from the methyl carbonyl residues (Pezacka and Wood, 1984; Ragsdale and Wood, 1985). This indicates that the reductive acetyl-CoA pathway (WL pathway) is a primary carbon fixation channel in the GSL sediments. Recognized sulfate reducers Desulfobacteraceae and Desulfohalobiaceae in the GSL ecosystem (Brandt and Ingvorsen, 1997; Jakobsen et al., 2006; Kuever, 2014a, b) were identified with genes required for dissimilatory sulfate reduction as well as the WL pathway (Figure 5B). This suggests that Desulfobacteraceae and Desulfohalobiaceae function via coupled exergonic sulfate reduction and endergonic acetate oxidation (reverse WL pathway), as reported before (Schauder et al., 1988; Spormann and Thauer, 1988; Ferry, 1992). In our study, the MAGs of sulfur oxidizers Oceanospirillales and Thioalkalivibrio thiocyanoxidans showed minimal or no genes responsible for the thiosulfate oxidation pathway (Figure 5B), although previous studies have identified the presence of that pathway in these genomes (Swan et al., 2011; Berben et al., 2017). The genomes of strict anaerobes Desulfobacteraceae and Desulfohalobiaceae displayed partially complete nitrification pathways. However, the possibility of nitrification within these anaerobes cannot be asserted due to the absence of ammonia mono-oxygenase subunits in the MAGs. This suggests that the nutrient cycling pathway in GSL sediments observed in our genome-based study is still not comprehensive.
Lysogenic Metagenome-Assembled Genomes and Expanding Viral Host Range
In the GSL ecosystem, sequence reads that belong to phylum Euryarchaeota, and Proteobacteria has higher abundance of the total bacteria and archaea, respectively (Figure 3A). Across the GSL, we predicted eight viruses that infect MAGs affiliated to phylum Euryarchaeota (Supplementary Table S8). Such low numbers for viruses of Euryarchaeota are due to limitations in current viral-host prediction computational analysis. More than half (54.3%) of the 151 putative GSL viruses-host pairing were to MAGs of the phylum Proteobacteria (Supplementary Table S8).
The genetic content between the GSL viruses and the bacterial and archaeal genomes from the NCBI RefSeq database was compared based on homology matches, shared tRNAs, and CRISPR spacers. The prediction of the bacterial and archaeal hosts of the GSL viruses shows the association between four bacterial and archaeal genomes (lysogenic MAGs) with five GSL viruses are virus–host associations. These GSL viruses infect the bacteria and archaea that they co-binned with [Genus Stenotrophomonas (2), genus Pseudomonas (1), family Rhodobacteraceae (1), family Halanaerobiaceae (1)]. However, the host of two viruses co-binned with the MAGs of phylum Bacteroidetes and phylum Proteobacteria were genomes of phylum Firmicutes. The differences between the association between virus, bacteria, and archaea in our study and the virus–host prediction indicate expanding host range of the GSL viruses.
Great Salt Lake Viruses Encode Auxiliary Metabolic Genes Involved in Biogeochemical Cycles
The hypersaline viruses of GSL have AMGs. These AMGs could have been acquired from their immediate host during infection events (Mann et al., 2003; Thompson et al., 2011; Crummett et al., 2016). The historical acquisition of genes has been studied in cyanophage genomes. It has been shown that the selective pressure leads to incorporation of host-like genes in the viral genomes as Calvin cycle inhibitor CP12 was identified in cyanophage genomes to redirect host metabolism toward DNA biosynthesis. Also, there was a homology observed between the DNA sequences from bacteriophage S-PM2 and host Synechococcus encoding D1 proteins suggesting the horizontal acquisition of genes into the viral genome from their host (Thompson et al., 2011). These gene/s modulate host metabolism during infection events for the viruses to replicate efficiently (Breitbart et al., 2007). Viruses identified as Enterococcus phage EF24C, Lactococcus phage phiL47 (CB2) and Aeromonas virus 31 (GSL3510) encoding SHMT were identified. The SHMT enzyme is required for formaldehyde assimilation via the serine pathway (Chistoserdova et al., 1994). This enzyme catalyzes the reaction of formaldehyde and glycine for the formation of L- serine. Bacterial and archaeal host for the GSL virus with SHMT gene is unknown. The viruses with AMG SHMT likely acquired it from bacterial/archaeal host during previous infection event.
A viral genome co-binned with Halodesulfurarchaeum MAG (lysogenic archaea) displayed an AMG which encodes nitric oxide reductase (NorQ) protein. Nitric oxide reductase (NorQ) facilitates the insertion of non-heme Fe (FeB) into the cytochrome c dependent nitric oxide reductase (cNOR)- a vital membrane protein required for denitrification (Kahle et al., 2018). However, the virus may have acquired the AMG during past infection of the GSL denitrifier/s. Halodesulfurarchaeum depends on sulfur for respiration (Sorokin et al., 2017). In addition, the GSL draft Halodesulfurarchaeum MAG (GSL_GSL3510_HALOBAC1; 75.15% complete) lacks the genes for denitrification.
Viruses in GSL were also identified with AMGs responsible for carbon fixation and photosynthesis. The psbA gene required for Photosystem II and rbcL, cbbL gene involved in reductive pentose phosphate cycle (Calvin cycle) were detected within viruses from GSL site CB2. The Cyanobacteria MAG with photosynthetic metabolism and substantially complete Calvin cycle pathway from site CB2 had no association with the virus. We suspect that most sequences (66.1 ± 4.61%) did not get binned as draft bacterial and archaeal genomes. This led to failure to properly reconstruct the virus–host infection network of GSL. Without knowing the bacterial and archaeal host to these viruses, it is difficult to predict how these metabolic genes contribute to different biogeochemical processes. Nevertheless, the AMGs have ecological significance as they can be expressed or recombined with the host genes during infection thus altering host metabolisms.
Conclusion
Microbes (algae, bacteria, and archaea) are the primary producers in the GSL. They support other aquatic life forms such as Brine shrimps. Bacteria and archaea perform critical ecosystem functions by cycling nutrients (N, P, S, and C) (Motlagh et al., 2017). These microbes provide biological foundations upon which other unicellular and multi-cellular organisms establish themselves. Bacterial and archaea that inhabit the GSL extreme environments are often endemic to the region. The viruses of GSL that infect bacteria and archaea decide the fate of the host cell. During infection events, the viruses reprogram the host cellular metabolism for replication and propagation (Forterre, 2013; Rosenwasser et al., 2016). One-third of the GSL viruses are unclassified DNA viruses comprising Haloviruses. Two-third of the viruses belong to the order Caudovirales and spread across the families Siphoviridae, Myoviridae, and Podoviridae. The Haloviruses that infect halophilic archaea are low in numbers at the site with higher salinity, inorganic nutrients, and organic carbon. Diverging of the Roux et al. (2016) findings, the abundance of Haloviruses and its host are independent of salinity changes across the lake. In GSL, salinity alone does not determine the viral, bacterial, archaeal diversity. Instead, organic carbon, inorganic nutrients, and salinity fuel the bacterial and archaeal diversity across the lake. In addition, the microbes are equipped with genes for Calvin–Benson–Bassham (aerobic), and WL (anaerobic), carbon fixation pathways. The metabolic flexibility by two carbon fixation pathways supports bacterial and archaeal growth. The viruses that infect bacteria and archaea replicate and propagate by lysing the host. Lysogenic infection events are rare at this site (Supplementary Figure S5). The nutrient abundant regions of GSL support lytic lifestyle for the viruses. In GSL, the acetyl-coA pathway is the primary carbon fixation pathway. Sulfate reducers Desulfobacteraceae and Desulfohalobiaceae are prevalent across the GSL.
This study’s findings will provide important information regarding growth conditions and nutritional requirements for the isolation of a diverse set of bacteria, archaea, and viruses. This information will facilitate the culture-based study of GSL microbiome and viruses, thus elucidating their roles in biogeochemical cycling through detailed physiological studies.
Data Availability Statement
All DNA sequence FASTQ files are deposited in the NCBI Nucleotide Archive under SRR14023866 (CB2 Bacterial and Archaeal), SRR 14023937 (GSL 3510 Bacterial and Archaeal), SRR14023877 (GB14 Bacterial and Archaeal), SRR14023878 (CB2 Viral), SRR14023865 (GSL3510 Viral), and SRR14023938 (GB14 Viral). The Bioproject id is PRJNA714934. The NCBI genome accession numbers for the MAGs and viral assemblies are shown in Supplementary Table S10.
Author Contributions
BB conducted experiments, bioinformatic analysis, sequence data management, wrote the manuscript, and submissions to public repository. AB conducted bioinformatic analysis and assisted in writing the manuscript. FC contributed toward bioinformatic analysis (viral–host analysis, gene prediction and phage identification). RG supervised the GSL research, mentored students, and finalized the manuscript for submission.
Funding
The funding for this project was provided by the United States National Science Foundation with project number 1510255. However, the views and data discussion expresses are those of authors and not necessarily reflect any role of the funding agency.
Conflict of Interest
The authors declare that the research was conducted in the absence of any commercial or financial relationships that could be construed as a potential conflict of interest.
Publisher’s Note
All claims expressed in this article are solely those of the authors and do not necessarily represent those of their affiliated organizations, or those of the publisher, the editors and the reviewers. Any product that may be evaluated in this article, or claim that may be made by its manufacturer, is not guaranteed or endorsed by the publisher.
Acknowledgments
This study was supported by the National Science Foundation (NSF) Grant # 1510255. The opinions and views expressed are those of authors and do not necessarily reflect any association with the funding agency. We appreciate Ryan Newton at United States Geological Survey (USGS) for helping us in sampling at the GSL. We also thank Dr. Rakesh Kaundal at the Utah State University for valuable suggestions during the bioinformatic analysis. Graduate student Mr. Imdad Islam helped with sample collection and water chemistry parameter estimation. We positively acknowledge Imdad’s contribution.
Supplementary Material
The Supplementary Material for this article can be found online at: https://www.frontiersin.org/articles/10.3389/fmicb.2021.701414/full#supplementary-material
Supplementary Figure S1 | Vertical profile of physio-chemical data (specific conductivity, dissolved oxygen and oxidation–reduction potential) in three Great Salt Lake (GSL) study areas [(A) CB2, (B) GSL 3510, (C) GB14].
Supplementary Figure S2 | Workflow schematic of the viral, bacterial, and archaeal metagenome analysis.
Supplementary Figure S3 | Genome map of viral genomes (contigs) identified from the Great Salt Lake sediments with auxiliary metabolic genes (AMGs). The AMGs are denoted by asterisk (*). Arrow length in the genome maps is proportional to the size of the open reading frame (ORF).
Supplementary Figure S4 | Taxonomic profiling of the bacterial and archaeal communities in sediments across the Great Salt Lake in (A) Kingdom, (B) Class, (C) Order, (D) Family, (E) Genus, and (F) Species level.
Supplementary Figure S5 | Relative abundance of lysogenic MAGs represented by bar graphs.
Supplementary Figure S6 | Number of CRISPR arrays and spacer sequences in bacterial and archaeal contigs (per million reads) across the Great Salt Lake.
Supplementary Figure S7 | Association of CRISPR spacers from the bacterial and archaeal contigs with the viruses from the marine, saline, and hypersaline aquatic ecosystems (extracted from IMG/VR database).
Supplementary Figure S8 | Comparison of relative abundance between bacteria and archaeal phyla (based on bacterial and archaeal metagenome reads) with viruses that infects them (based on the Virus–Host database) across the Great Salt Lake sediments.
Supplementary Table S1 | Metagenomic read statistics.
Supplementary Table S2 | De novo assembly statistics of virome, bacterial, and archaeal metagenome.
Supplementary Table S3 | Summary of the number of viral contigs identified using VirSorter.
Supplementary Table S4 | Genome statistics of MAGs from the Great Salt Lake (Utah, United States) (completeness < 50%).
Supplementary Table S5 | Lysogenic MAGs identified in the bacterial and archaeal sequences.
Supplementary Table S6 | Lysogenic MAGs identified in the viral sequences.
Supplementary Table S7 | Summary of virus–host association based on CRISPR array.
Supplementary Table S8 | Computational host prediction of the viral contigs identified against the bacterial and archaeal genomes in the NCBI RefSeq database based on homology matches, shared tRNAs, and CRISPR spacers.
Supplementary Table S9 | Lifestyle prediction of viruses based on the conserved protein domains using BACPHLIP (Hockenberry and Wilke, 2021).
Supplementary Table S10 | NCBI accession numbers of the Great Salt Lake metagenome assembled genomes (MAGs) and viral assemblies.
Abbreviations
GSL, Great Salt Lake; MAG, metagenome assembled genome; AMG, auxiliary metabolic gene; USGS, United States Geological Survey; ORP, oxidation–reduction potential; DO, dissolved oxygen; MWCO, molecular weight cut-off; RASL, random amplified shotgun library; LASL, linker-amplified shotgun library; MDA, multiple displacement amplification; RMC, raw metagenome contigs; RVC, raw metavirome contigs; CMC, curated metagenome contigs; CVC, combined viral contigs; RVG, reference viral genomes; EVG, environmental viral genomes; ORF, open reading frame; NCBI, National Center for Biotechnology Information; LCB, locally collinear blocks; SHMT, glycine hydroxymethyltransferase; GTDB, Genomic Taxonomy Database; TOC, total organic carbon; TEM, transmission electron microscopy; WL, Wood–Ljungdahl.
References
Ahlgren, N. A., Fuchsman, C. A., Rocap, G., and Fuhrman, J. A. (2019). Discovery of several novel, widespread, and ecologically distinct marine Thaumarchaeota viruses that encode amoC nitrification genes. ISME J. 13, 618–631. doi: 10.1038/s41396-018-0289-4
Almeida-Dalmet, S., Sikaroodi, M., Gillevet, P., Litchfield, C., and Baxter, B. (2015). Temporal Study of the Microbial Diversity of the North Arm of Great Salt Lake, Utah, U.S. Microorganisms 3, 310–326. doi: 10.3390/microorganisms3030310
Anantharaman, K., Duhaime, M. B., Breier, J. A., Wendt, K. A., Toner, B. M., and Dick, G. J. (2014). Sulfur oxidation genes in diverse deep-sea viruses. Science 344, 757–760. doi: 10.1126/science.1252229
Anderson, R. E., Sogin, M. L., and Baross, J. A. (2014). Evolutionary strategies of viruses, bacteria and archaea in hydrothermal vent ecosystems revealed through metagenomics. PLoS One 9:e109696. doi: 10.1371/journal.pone.0109696
Andrews, S. (2010). FastQC: a Quality Control Tool for High Throughput Sequence Data. Cambridge, MA: Babraham Institute.
Angly, F. E., Felts, B., Breitbart, M., Salamon, P., Edwards, R. A., Carlson, C., et al. (2006). The marine viromes of four oceanic regions. PLoS Biol. 4:e0040368. doi: 10.1371/journal.pbio.0040368
Atanasova, N. S., Oksanen, H. M., and Bamford, D. H. (2015). Haloviruses of archaea, bacteria, and eukaryotes. Curr. Opin. Microbiol. 25, 40–48. doi: 10.1016/j.mib.2015.04.001
Bankevich, A., Nurk, S., Antipov, D., Gurevich, A. A., Dvorkin, M., Kulikov, A. S., et al. (2012). SPAdes: a new genome assembly algorithm and its applications to single-cell sequencing. J. Comput. Biol. 19, 455–477. doi: 10.1089/cmb.2012.0021
Baxter, B. K., and Butler, J. K. (2020). “Climate change and great Salt Lake,” in Great Salt Lake Biology, eds B. K. Baxter and J. K. Butler (Berlin: Springer International Publishing), 23–52. doi: 10.1007/978-3-030-40352-2_2
Baxter, B. K., Litchfield, C. D., Sowers, K., Griffith, J. D., Dassarma, P. A., and Dassarma, S. (2005). “Microbial diversity of great Salt Lake,” in Adaptation to Life at High Salt Concentrations in Archaea, Bacteria, and Eukarya, eds N. Gunde-Cimerman, A. Oren, and A. Plemenitaš (Dordrecht: Springer), 9–25.
Baxter, B. K., Mangalea, M. R., Willcox, S., Sabet, S., Nagoulat, M. N., and Griffith, J. D. (2011). “Haloviruses of Great Salt Lake: a model for understanding viral diversity,” in Halophiles and Hypersaline Environments, (Berlin: Springer), 173–190.
Berben, T., Overmars, L., Sorokin, D. Y., and Muyzer, G. (2017). Comparative genome analysis of three thiocyanate oxidizing Thioalkalivibrio species isolated from soda lakes. Front. Microbiol. 8:254. doi: 10.3389/fmicb.2017.00254
Bergh, Ø, Børsheim, K. Y., Bratbak, G., and Heldal, M. (1989). High abundance of viruses found in aquatic environments. Nature 340, 467–468. doi: 10.1038/340467a0
Bergmann, D. J., Hooper, A. B., and Klotz, M. G. (2005). Structure and sequence conservation of hao cluster genes of autotrophic ammonia-oxidizing bacteria: evidence for their evolutionary history. Appl. Environ. Microbiol. 71, 5371–5382. doi: 10.1128/AEM.71.9.5371-5382.2005
Bolger, A. M., Lohse, M., and Usadel, B. (2014). Trimmomatic: a flexible trimmer for Illumina sequence data. Bioinformatics 30, 2114–2120. doi: 10.1093/bioinformatics/btu170
Boyd, E. S., Hamilton, T. L., Swanson, K. D., Howells, A. E., Baxter, B. K., Meuser, J. E., et al. (2014). [FeFe]-hydrogenase abundance and diversity along a vertical redox gradient in great Salt Lake, USA. Int. J. Mol. Sci. 15, 21947–21966. doi: 10.3390/ijms151221947
Brandt, K. K., and Ingvorsen, K. (1997). Desulfobacter halotolerans sp. nov., a halotolerant acetate-oxidizing sulfate-reducing bacterium isolated from sediments of Great Salt Lake, Utah. Syst. Appl. Microbiol. 20, 366–373. doi: 10.1016/S0723-2020(97)80004-5
Breitbart, M., Thompson, L. R., Suttle, C. A., and Sullivan, M. B. (2007). Exploring the vast diversity of marine viruses. Oceanography 20, 135–139. doi: 10.5670/oceanog.2007.58
Bushnell, B. (2014). BBMap: a Fast, Accurate, Splice-Aware Aligner. Berkeley, CA: Lawrence Berkeley National Lab.(LBNL).
Cannon, J. S., and Cannon, M. A. (2002). The Southern Pacific Railroad trestle—past and present, in Great Salt Lake, an Overview of Change, ed. J. W. Gwynn (Salt Lake City: Department of Natural Resources) 283–294.
Chaumeil, P.-A., Mussig, A. J., Hugenholtz, P., and Parks, D. H. (2019). GTDB-Tk: a toolkit to classify genomes with the Genome Taxonomy Database. Hancock J, editor. Bioinformatics 36, 1925–1927. doi: 10.1093/bioinformatics/btz848
Chistoserdova, L. V., Lidstrom, M. E., and Keck, W. M. (1994). Genetics of the serine cycle in methylobacterium extorquens AM1: cloning, sequence, mutation, and physiological effect of glyA, the gene for serine hydroxymethyltransferase. J. Bacteriol. 176, 6759–6762. doi: 10.1128/jb.176.21.6759-6762.1994
Coutinho, F. H., Cabello-Yeves, P. J., Gonzalez-Serrano, R., Rosselli, R., López-Pérez, M., Zemskaya, T. I., et al. (2020). New viral biogeochemical roles revealed through metagenomic analysis of Lake Baikal. Microbiome 8, 1–15. doi: 10.1186/s40168-020-00936-4
Cram, J. A., Parada, A. E., and Fuhrman, J. A. (2016). Dilution reveals how viral lysis and grazing shape microbial communities. Limnol. Oceanogr. 61, 889–905. doi: 10.1002/lno.10259
Crummett, L. T., Puxty, R. J., Weihe, C., Marston, M. F., and Martiny, J. B. H. (2016). The genomic content and context of auxiliary metabolic genes in marine cyanomyoviruses. Virology 499, 219–229. doi: 10.1016/j.virol.2016.09.016
Daffonchio, D., Borin, S., Brusa, T., Brusetti, L., Van Der Wielen, P. W. J. J., Bolhuis, H., et al. (2006). Stratified prokaryote network in the oxic-anoxic transition of a deep-sea halocline. Nature 440, 203–207. doi: 10.1038/nature04418
Dávila-Ramos, S., Castelán-Sánchez, H. G., Martínez-ávila, L., Sánchez-Carbente, M. D. R., Peralta, R., Hernández-Mendoza, A., et al. (2019). A review on viral metagenomics in extreme environments. Front. Microbiol. 10:2403. doi: 10.3389/fmicb.2019.02403
Diemer, G. S., and Stedman, K. M. (2012). A novel virus genome discovered in an extreme environment suggests recombination between unrelated groups of RNA and DNA viruses. Biol. Direct 7, 1–4. doi: 10.1186/1745-6150-7-13
Emerson, J. B., Thomas, B. C., Andrade, K., Allen, E. E., Heidelberg, K. B., and Banfielda, J. F. (2012). Dynamic viral populations in hypersaline systems as revealed by metagenomic assembly. Appl. Environ. Microbiol. 78, 6309–6320. doi: 10.1128/AEM.01212-12
Eren, A. M., Esen, O. C., Quince, C., Vineis, J. H., Morrison, H. G., Sogin, M. L., et al. (2015). Anvi’o: an advanced analysis and visualization platformfor’ omics data. PeerJ 2015:e1319. doi: 10.7717/peerj.1319
Ferry, J. G. (1992). Biochemistry of methanogenesis. Crit. Rev. Biochem. Mol. Biol. 27, 473–503. doi: 10.3109/10409239209082570
Forterre, P. (2013). The virocell concept and environmental microbiology. ISME J. 7, 233–236. doi: 10.1038/ismej.2012.110
Fuhrman, J. A. (1999). Marine viruses and their biogeochemical and ecological effects. Nature 399, 541–548. doi: 10.1038/21119
Garcia-Heredia, I., Martin-Cuadrado, A.-B., Mojica, F. J. M., Santos, F., Mira, A., Antón, J., et al. (2012). Reconstructing viral genomes from the environment using fosmid clones: the case of haloviruses. Liles MR, editor. PLoS One. 7:e33802. doi: 10.1371/journal.pone.0033802
Gong, Z., Liang, Y., Wang, M., Jiang, Y., Yang, Q., Xia, J., et al. (2018). Viral diversity and its relationship with environmental factors at the surface and deep sea of prydz Bay, Antarctica. Front. Microbiol. 9:2981. doi: 10.3389/fmicb.2018.02981
Graham, E., Paez-Espino, D., Brislawn, C., Hofmockel, K., Wu, R., Kyrpides, N., et al. (2019). Untapped viral diversity in global soil metagenomes. bioRxiv [Preprint]. doi: 10.1101/583997
Herlemann, D. P. R., Labrenz, M., Jürgens, K., Bertilsson, S., Waniek, J. J., and Andersson, A. F. (2011). Transitions in bacterial communities along the 2000 km salinity gradient of the Baltic Sea. ISME J. 5, 1571–1579. doi: 10.1038/ismej.2011.41
Hockenberry, A. J., and Wilke, C. O. (2021). BACPHLIP: predicting bacteriophage lifestyle from conserved protein domains. PeerJ 9:e11396.
Hoehler, T. M., Bebout, B. M., and Des Marais, D. J. (2001). The role of microbial mats in the production of reduced gases on the early Earth. Nature 412, 324–327. doi: 10.1038/35085554
Hurwitz, B. L., and U’Ren, J. M. (2016). Viral metabolic reprogramming in marine ecosystems. Curr. Opin. Microbiol. 31, 161–168. doi: 10.1016/j.mib.2016.04.002
Hyatt, D., Chen, G. L., LoCascio, P. F., Land, M. L., Larimer, F. W., and Hauser, L. J. (2010). Prodigal: prokaryotic gene recognition and translation initiation site identification. BMC Bioinformatics 11:119. doi: 10.1186/1471-2105-11-119
Jakobsen, T. F., Kjeldsen, K. U., and Ingvorsen, K. (2006). Desulfohalobium utahense sp. nov., moderately halophilic, sulfate-reducing bacterium isolated from Great Salt Lake. Int. J. Syst. Evol. Microbiol. 56, 2063–2069. doi: 10.1099/ijs.0.64323-0
Ji, M., Kong, W., Yue, L., Wang, J., Deng, Y., and Zhu, L. (2019). Salinity reduces bacterial diversity, but increases network complexity in Tibetan Plateau lakes. FEMS Microbiol. Ecol. 95:190. doi: 10.1093/femsec/fiz190
Jiang, S. C., and Paul, J. H. (1998). Gene transfer by transduction in the marine environment. Appl. Environ. Microbiol. 64, 2780–2787. doi: 10.1128/AEM.64.8.2780-2787.1998
Kahle, M., ter Beek, J., Hosler, J. P., and Ädelroth, P. (2018). The insertion of the non-heme Fe B cofactor into nitric oxide reductase from P. denitrificans depends on NorQ and NorD accessory proteins. Biochim. Biophys. Acta 1859, 1051–1058. doi: 10.1016/j.bbabio.2018.05.020
Kallies, R., Hölzer, M., Brizola Toscan, R., Nunes da Rocha, U., Anders, J., Marz, M., et al. (2019). Evaluation of sequencing library preparation protocols for viral metagenomic analysis from pristine aquifer groundwaters. Viruses 11:484. doi: 10.3390/v11060484
Kang, D. D., Li, F., Kirton, E., Thomas, A., Egan, R., An, H., et al. (2019). MetaBAT 2: an adaptive binning algorithm for robust and efficient genome reconstruction from metagenome assemblies. PeerJ 2019:e7359. doi: 10.7717/peerj.7359
Keck, W., and Hassibe, W. (1979). The Great Salt Lake. New York, NY: Department of the Interior, Geological Survey.
Kuever, J. (2014a). “The family Desulfobacteraceae,” in The Prokaryotes: Deltaproteobacteria and Epsilonproteobacteria, eds E. Rosenberg, E.F. DeLong, S. Lory, E. Stackebrandt, and F. Thompson (Berlin: Springer-Verlag), 45–73.
Kuever, J. (2014b). “The family Desulfohalobiaceae,” in The Prokaryotes: Deltaproteobacteria and Epsilonproteobacteria, eds E. Rosenberg, E.F. DeLong, S. Lory, E. Stackebrandt, and F. Thompson (Berlin: Springer-Verlag), 87–95.
Langmead, B., and Salzberg, S. (2012). Fast gapped-read alignment with Bowtie 2. Nat. Methods 9:357. doi: 10.1038/nmeth.1923
Li, W., and Godzik, A. (2006). Cd-hit: a fast program for clustering and comparing large sets of protein or nucleotide sequences. Bioinformatics 22, 1658–1659. doi: 10.1093/bioinformatics/btl158
Lindell, D., Jaffe, J. D., Coleman, M. L., Futschik, M. E., Axmann, I. M., Rector, T., et al. (2007). Genome-wide expression dynamics of a marine virus and host reveal features of co-evolution. Nature 449, 83–86. doi: 10.1038/nature06130
Lindsay, M. R., Anderson, C., Fox, N., Scofield, G., Allen, J., Anderson, E., et al. (2017). Microbialite response to an anthropogenic salinity gradient in Great Salt Lake, Utah. Geobiology 15, 131–145. doi: 10.1111/gbi.12201
Logares, R., Lindström, E. S., Langenheder, S., Logue, J. B., Paterson, H., Laybourn-Parry, J., et al. (2013). Biogeography of bacterial communities exposed to progressive long-term environmental change. ISME J. 7, 937–948. doi: 10.1038/ismej.2012.168
Madison, R. J. (1970). Effects of a Causeway on the Chemistry of the Brine in Great Salt Lake, Utah. Utah: Utah Geological and Mineralogical Survey.
Mann, N. H., Cook, A., Millard, A., Bailey, S., and Clokie, M. (2003). Bacterial photosynthesis genes in a virus. Nature 424:741. doi: 10.1038/424741a
Meuser, J. E., Baxter, B. K., Spear, J. R., Peters, J. W., Posewitz, M. C., and Boyd, E. S. (2013). Contrasting Patterns of Community Assembly in the Stratified Water Column of Great Salt Lake, Utah. Microb. Ecol. 66, 268–280. doi: 10.1007/s00248-013-0180-9
Mihara, T., Nishimura, Y., Shimizu, Y., Nishiyama, H., Yoshikawa, G., Uehara, H., et al. (2016). Linking virus genomes with host taxonomy. Viruses 8:66. doi: 10.3390/v8030066
Motlagh, A. M., Bhattacharjee, A. S., Coutinho, F. H., Dutilh, B. E., Casjens, S. R., and Goel, R. K. (2017). Insights of phage-host interaction in hypersaline ecosystem through metagenomics analyses. Front. Microbiol. 8:352. doi: 10.3389/fmicb.2017.00352
Nasko, D. J., Ferrell, B. D., Moore, R. M., Bhavsar, J. D., Polson, S. W., and Wommack, K. E. (2019). Crispr spacers indicate preferential matching of specific virioplankton genes. mBio 10:e02651-18. doi: 10.1128/mBio.02651-18
Nooij, S., Schmitz, D., Vennema, H., Kroneman, A., and Koopmans, M. P. G. (2018). Overview of virus metagenomic classification methods and their biological applications. Front. Microbiol. 9:749. doi: 10.3389/fmicb.2018.00749
Paez-Espino, D., Roux, S., Chen, I. M. A., Palaniappan, K., Ratner, A., Chu, K., et al. (2019). IMG/VR v.2.0: an integrated data management and analysis system for cultivated and environmental viral genomes. Nucleic Acids Res. 47, D678–D686. doi: 10.1093/nar/gky1127
Parks, D. H., Imelfort, M., Skennerton, C. T., Hugenholtz, P., and Tyson, G. W. (2015). CheckM: assessing the quality of microbial genomes recovered from isolates, single cells, and metagenomes. Genome Res. 25, 1043–1055. doi: 10.1101/gr.186072.114
Pezacka, E., and Wood, H. G. (1984). Role of carbon monoxide dehydrogenase in the autotrophic pathway used by acetogenic bacteria. Proc. Natl. Acad. Sci. U.S.A. 81, 6261–6265. doi: 10.1073/pnas.81.20.6261
Pfaff, J. (1993). Determination of Inorganic Anions by Ion Chromatography (300.0), EPA Methods for Determination of Inorganic Substances in Environmental Samples. Ohio: U.S. Environmental Protection Agency.
Post, F. J. (1981). “Microbiology of the Great Salt Lake north arm”, in Salt Lakes. Netherlands: Springer, 59–69.
Pride, D. T., Meinersmann, R. J., Wassenaar, T. M., and Blaser, M. J. (2003). Evolutionary implications of microbial genome tetranucleotide frequency biases. Genome Res. 13, 145–158. doi: 10.1101/gr.335003
Ragsdale, S. W., and Wood, H. G. (1985). Acetate biosynthesis by acetogenic bacteria. Evidence that carbon monoxide dehydrogenase is the condensing enzyme that catalyzes the final steps of the synthesis. J. Biol. Chem. 260, 3970–3977. doi: 10.1016/S0021-9258(18)89217-1
Ramos-Barbero, M. D., Martínez, J. M., Almansa, C., Rodríguez, N., Villamor, J., Gomariz, M., et al. (2019). Prokaryotic and viral community structure in the singular chaotropic salt lake Salar de Uyuni. Environ. Microbiol. 21, 2029–2042. doi: 10.1111/1462-2920.14549
Rodriguez-Brito, B., Li, L. L., Wegley, L., Furlan, M., Angly, F., Breitbart, M., et al. (2010). Viral and microbial community dynamics in four aquatic environments. ISME J. 4, 739–751. doi: 10.1038/ismej.2010.1
Rodriguez-R, L. M., and Konstantinidis, K. T. (2016). The enveomics collection: a toolbox for specialized analyses of microbial genomes and metagenomes. PeerJ [Epub ahead of print]. doi: 10.7287/peerj.preprints.1900v1
Rosenwasser, S., Ziv, C., van Creveld, S. G., and Vardi, A. (2016). Virocell metabolism: metabolic innovations during host–virus interactions in the Ocean. Trends Microbiol. 24, 821–832. doi: 10.1016/j.tim.2016.06.006
Roux, S., Enault, F., Hurwitz, B. L., and Sullivan, M. B. (2015). VirSorter: mining viral signal from microbial genomic data. PeerJ 2015:e985. doi: 10.7717/peerj.985
Roux, S., Enault, F., Ravet, V., Colombet, J., Bettarel, Y., Auguet, J. C., et al. (2016). Analysis of metagenomic data reveals common features of halophilic viral communities across continents. Environ. Microbiol. 18, 889–903. doi: 10.1111/1462-2920.13084
Ruiz-Perez, C. A., Conrad, R. E., and Konstantinidis, K. T. (2020). MicrobeAnnotator: a user-friendly, comprehensive mi-crobial genome annotation pipeline. bioRxiv [Preprint]. doi: 10.1101/2020.07.20.211847
Santos, F., Yarza, P., Parro, V., Meseguer, I. I., Rosselló-Móra, R., and Antón, J. (2012). Culture-independent approaches for studying viruses from hypersaline environments. Appl. Environ. Microbiol. 78, 1635–1643. doi: 10.1128/AEM.07175-11
Schauder, R., Preuß, A., Jetten, M., and Fuchs, G. (1988). Oxidative and reductive acetyl CoA/carbon monoxide dehydrogenase pathway in Desulfobacterium autotrophicum. Arch. Microbiol. 151, 84–89. doi: 10.1007/BF00444674
Seemann, T. (2014). Prokka: rapid prokaryotic genome annotation. Bioinformatics 30, 2068–2069. doi: 10.1093/bioinformatics/btu153
Sencilo, A., and Roine, E. (2014). A Glimpse of the genomic diversity of haloarchaeal tailed viruses. Front. Microbiol. 5:84. doi: 10.3389/fmicb.2014.00084
Shen, P. S., Domek, M. J., Sanz-Garcia, E., Makaju, A., Taylor, R. M., Hoggan, R., et al. (2012). Sequence and structural characterization of great salt lake bacteriophage CW02, a member of the T7-like supergroup. J. Virol. 86, 7907–7917. doi: 10.1128/JVI.00407-12
Silva, G. G. Z., Green, K. T., Dutilh, B. E., and Edwards, R. A. (2016). SUPER-FOCUS: a tool for agile functional analysis of shotgun metagenomic data. Bioinformatics 32, 354–361. doi: 10.1093/bioinformatics/btv584
Sorokin, D. Y., Messina, E., Smedile, F., Roman, P., Damsté, J. S. S., Ciordia, S., et al. (2017). Discovery of anaerobic lithoheterotrophic haloarchaea, ubiquitous in hypersaline habitats. ISME J. 11, 1245–1260. doi: 10.1038/ismej.2016.203
Spormann, A. M., and Thauer, R. K. (1988). Anaerobic acetate oxidation to CO2 by Desulfotomaculum acetoxidans. Arch. Microbiol. 150, 374–380. doi: 10.1007/BF00408310
Stephens, D. W. (1990). Changes in lake levels, salinity and the biological community of Great Salt Lake (Utah, USA), 1847–1987. Hydrobiologia. 197, 139–146. doi: 10.1007/BF00026946
Sternai, P., Caricchi, L., Garcia-Castellanos, D., Jolivet, L., Sheldrake, T. E., and Castelltort, S. (2017). Magmatic pulse driven by sea-level changes associated with the Messinian salinity crisis. Nat. Geosci. 10, 783–787. doi: 10.1038/ngeo3032
Sullivan, M. B., Lindell, D., Lee, J. A., Thompson, L. R., Bielawski, J. P., and Chisholm, S. W. (2006). Prevalence and evolution of core photosystem II genes in marine cyanobacterial viruses and their hosts. Moran NA, editor. PLoS Biol. 4:e234. doi: 10.1371/journal.pbio.0040234
Swan, B. K., Martinez-Garcia, M., Preston, C. M., Sczyrba, A., Woyke, T., Lamy, D., et al. (2011). Potential for chemolithoautotrophy among ubiquitous bacteria lineages in the dark ocean. Science 333, 1296–1300. doi: 10.1126/science.1203690
Thompson, L. R., Zeng, Q., Kelly, L., Huang, K. H., Singer, A. U., Stubbe, J. A., et al. (2011). Phage auxiliary metabolic genes and the redirection of cyanobacterial host carbon metabolism. Proc. Natl. Acad. Sci. U.S.A. 108, 757–764. doi: 10.1073/pnas.1102164108
Truong, D. T., Franzosa, E. A., Tickle, T. L., Scholz, M., Weingart, G., Pasolli, E., et al. (2015). MetaPhlAn2 for enhanced metagenomic taxonomic profiling. Nat. Methods 12, 902–903. doi: 10.1038/nmeth.3589
Ventosa, A., Fernández, A. B., León, M. J., Sánchez-Porro, C., and Rodriguez-Valera, F. (2014). The Santa Pola saltern as a model for studying the microbiota of hypersaline environments. Extremophiles. 18, 811–824. doi: 10.1007/s00792-014-0681-6
Weinbauer, M. G., and Rassoulzadegan, F. (2004). Are viruses driving microbial diversification and diversity? Environ. Microbiol. 6, 1–11. doi: 10.1046/j.1462-2920.2003.00539.x
Wilde, F. D. (2011). Water-quality sampling by the U.S. Geological Survey – Standard protocols and procedures: U.S. Geological Survey Fact Sheet 2010-3121, 2. Available at: https://pubs.usgs.gov/fs/2010/3121
Wolfe, R. S., and McBride, B. C. (1971). A new coenzyme of methyl transfer, coenzyme M. Biochemistry 10, 2317–2324. doi: 10.1021/bi00788a022
Wu, Q. L., Zwart, G., Schauer, M., Kamst-Van Agterveld, M. P., and Hahn, M. W. (2006). Bacterioplankton community composition along a salinity gradient of sixteen high-mountain lakes located on the Tibetan Plateau, China. Appl. Environ. Microbiol. 72, 5478–5485. doi: 10.1128/AEM.00767-06
Keywords: viral diversity, virus–host association, hypersaline lake, lysogens, auxiliary metabolic genes
Citation: Bhattarai B, Bhattacharjee AS, Coutinho FH and Goel RK (2021) Viruses and Their Interactions With Bacteria and Archaea of Hypersaline Great Salt Lake. Front. Microbiol. 12:701414. doi: 10.3389/fmicb.2021.701414
Received: 27 April 2021; Accepted: 06 September 2021;
Published: 28 September 2021.
Edited by:
Eva Ortega-Retuerta, UMR 7621 Laboratoire d’Océanographie Microbienne (LOMIC), FranceReviewed by:
Claire Geslin, Université de Bretagne Occidentale, FrancePradeep Ram Angia Sriram, UMR 6023 Laboratoire Microorganismes Génome Et Environnement (LMGE), France
Copyright © 2021 Bhattarai, Bhattacharjee, Coutinho and Goel. This is an open-access article distributed under the terms of the Creative Commons Attribution License (CC BY). The use, distribution or reproduction in other forums is permitted, provided the original author(s) and the copyright owner(s) are credited and that the original publication in this journal is cited, in accordance with accepted academic practice. No use, distribution or reproduction is permitted which does not comply with these terms.
*Correspondence: Ramesh K. Goel, cmFtLmdvZWxAdXRhaC5lZHU=
†Present address: Ananda S. Bhattacharjee, Department of Environmental Sciences, The University of California, Riverside, Riverside, CA, United States; USDA-ARS, United States Salinity Laboratory, Riverside, CA, United States; Felipe H. Coutinho, Department of Marine Biology and Oceanography, Institute of Marine Sciences, Consejo Superior de Investigaciones Científicas, Barcelona, Spain