- 1Upland Flue-Cured Tobacco Quality and Ecology Key Laboratory of China Tobacco, Guizhou Academy of Tobacco Science, Guiyang, China
- 2College of Agriculture, Guizhou University, Guiyang, China
- 3Guizhou Institute of Plant Protection, Guizhou Academy of Agricultural Sciences, Guiyang, China
- 4Faculty of Science, Wilfrid Laurier University, Waterloo, ON, Canada
- 5College of Agriculture, Yangtze University, Jingzhou, China
- 6Key Laboratory of Crop Germplasm Resource, Department of Agronomy, College of Agriculture and Biotechnology, Zhejiang University, Hangzhou, China
A Myriad of biotic and abiotic factors inevitably affects the growth and production of tobacco (Nicotiana tabacum L.), which is a model crop and sought-after worldwide for its foliage. Among the various impacts the level of disease severity poses on plants, the influence on the dynamics of phyllospheric microbial diversity is of utmost importance. In China, recurring reports of a phyto-pathogen, Didymella segeticola, a causal agent of tobacco leaf spot, accentuate the need for its in-depth investigation. Here, a high-throughput sequencing technique, IonS5TMXL was employed to analyze tobacco leaves infected by D. segeticola at different disease severity levels, ranging from T1G (least disease index) to T4G (highest disease index), in an attempt to explore the composition and diversity of phyllospheric microbiota. In all healthy and diseased tobacco leaves, the most dominant fungal phylum was Ascomycota with a high prevalence of genus Didymella, followed by Boeremia, Meyerozyma and Alternaria, whereas in the case of bacterial phyla, Proteobacteria was prominent with Pseudomonas being a predominant genus, followed by Pantoea. The relative abundance of fungi, i.e., Didymella and Boeremia (Ascomycota) and bacteria, i.e., Pseudomonas and Pantoea (Proteobacteria) were higher in diseased groups compared to healthy groups. Healthy tissues exhibited relatively rich and diverse fungal communities in contrast with diseased groups. The infection of D. segeticola had a complex and significant effect on fungal as well as bacterial alpha diversity. FUNGuild analysis indicated that the relative abundance of pathotrophs and saprotrophs in diseased tissues proportionally increased with disease severity. PICRUSt analysis of diseased tissues indicated that the relative abundance of bacterial cell motility and membrane transport-related gene sequences elevated with an increase in disease severity from T1G to T3G and then tended to decrease at T4G. Conclusively, the current study shows the typical characteristics of the tobacco leaf microbiome and provides insights into the distinct microbiome shifts on tobacco leaves infected by D. segeticola.
Introduction
Given its commercial importance, tobacco is cultivated extensively all across the globe and categorized as a non-food agricultural crop, mainly consumed as cigarettes, cigars, snuff, etc. (Liu et al., 2017). Its leaves are susceptible to damage by fungal, bacterial, and viral pathogens during growth and development. Morphological deformations, such as leaf spots induced by microflora, could result in heavy economical losses (Zhu, 2002). As a new taxon, Didymella segeticola was first reported by Chen et al. (2015), while its pathological characteristics were explained by Zhao et al. (2018) as being a causal agent of leaf spot on tea plants in China. Likewise, a study carried out by Guo et al. (2020) revealed that D. segeticola causes leaf spot disease in tobacco plants as well. Symptomatically, diseased tobacco leaves affected by this pathogen exhibit sandy beige-colored lesions with dark-brown edges, circular, elliptical, or irregular in shape, usually surrounded by yellow halos (Guo et al., 2020).
The phyllosphere or phylloplane (leaf surface) of a plant is an important habitat for many potentially beneficial, pathogenic, or antagonistic microbes (including bacteria, fungi, protists, and viruses), forming complex plant-microbial interactions and contributing greatly to plant health as well as productivity (Lindow and Brandl, 2003; Xin et al., 2016; Luo et al., 2019). However, the phyllospheric microbial community and composition are subjected to change by plant diseases (Luo et al., 2017; Zhang Z. et al., 2019). Tobacco powdery mildew infected by Golovinomyces cichoracearum was reported to reduce the leaf fungal community abundance and diversity (Huang et al., 2020); whereas a similar response was observed by Xiang et al. (2020c) during the investigation of tobacco brown spot caused by Alternaria alternata. Chen Q. L. et al. (2020) noticed a much higher abundance of Rhizopus oryzae in cured tobacco leaves infected by tobacco pole rot than that of healthy leaves. The change of phyllosphere microbe potentially helps to evaluate the effects of efficient disease management. The microbiome of tobacco leaves during the epidemic season of tobacco brown spot was significantly affected by the foliage application of dimetachlone, especially for the major pathogen A. alternata (Chen X. Y. L. et al., 2020). The biological control agent Stenotrophomonas displayed better performance in decreasing the disease index of wildfire than that of kasugamycin (Liu et al., 2021). Additionally, phyllospheric microbes were associated with a particular stage of disease severity for powdery mildew in Euonymus japonicus (Zhang Z. et al., 2019) and in Cucurbita moschata (Zhang et al., 2018). Efficient disease management of tobacco leaf spot caused by D. segeticola demands much consideration of the phyllospheric microbial composition and diversity of tobacco leaves, especially during disease epidemic season.
In recent years, high-throughput sequencing technologies have been used to investigate the characterizations of phyllosphere microbial community and composition, including target sequencing of the phylogenetic markers encoding 16S rRNA for bacteria and Internal Transcribed Spacer (ITS) for fungi (Zhang et al., 2016; Song et al., 2017). Notably, high sequencing flux, accuracy, and cost performance are a few of their advantages among many, and have been applied in multiple fields (Li et al., 2018; Mboowa et al., 2018; Speranskaya et al., 2018), and progressively utilized by researchers to study the interactions between host plants and their respective environments (Espenshade et al., 2019; Singh et al., 2019; Zhang Z. et al., 2019). Particularly in tobacco, these tools have been employed in the investigation of leaf microbiome for both healthy and diseased foliage infected with pole rot (Chen et al., 2019), brown spot (Liu et al., 2019; Liu et al., 2020; Xiang et al., 2020c), and powdery mildew (Huang et al., 2020). Disease severity is an important biotic factor to affect leaf microbiome, however, its influence upon the dynamics of phyllosphere microbial diversity is largely unexplored, especially for the newly reported tobacco leaf spot caused by D. segeticola. After the invasion of the pathogen, tobacco leaves normally show both symptomatic and asymptomatic parts, which could vary significantly with the advancement of disease severity. It is imperative to gather knowledge of the typical tobacco leaf microbiome characteristics and inspect the distinct microbiome shifts on tobacco leaves infected by this pathogen. In this study, tobacco leaves at different levels of disease severity were collected from a commercial field. Both fungal and bacterial compositions and diversity of tobacco leaves (symptomatic and asymptomatic parts) were analyzed by IonS5TMXL high-throughput sequencing technique, which led to some interesting findings. This study will provide a novel perspective on D. segeticola infection and its influence on the leaf microbiome at different leaf spot disease severity stages in tobacco.
Materials and Methods
Sampling Sites and Sampling Strategy
Leaf samples were obtained from tobacco plants (cultivar Yunyan 87) with symptoms of leaf spot disease caused by D. segeticola in June 2019, Zheng’an county (28.55° N,107.44°E), Guizhou Province, China. Tobacco leaves were randomly selected and classified into four different disease severity levels (T1G–T4G) based on the Chinese National Standard (GB/T 23222—2008). For T1G, T2G, T3G, and T4G groups, diseased lesion area ranged from 6 to 10%, 11 to 20%, 21 to 40%, and 41 to 100% of a leaf (Zhang et al., 2018), respectively. A total of 24 samples were collected and are illustrated in Figure 1. Three biological replicates from each group (same disease severity, different individual) were separated into two parts, with and without visible leaf spots, and labeled as diseased and healthy samples, respectively as presented in Table 1. After classification, leaf samples were immediately taken to the laboratory and stored at −80°C until further use.
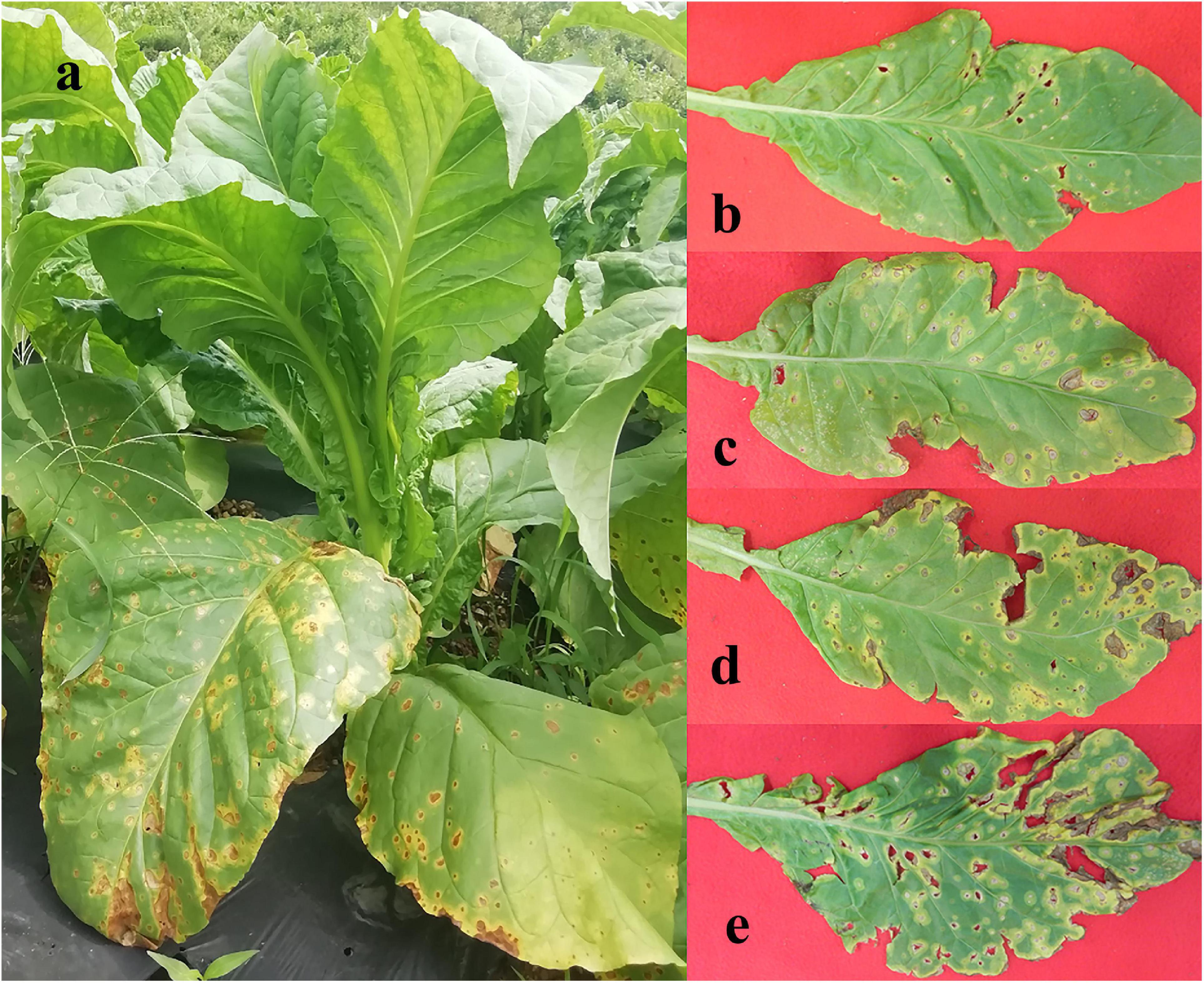
Figure 1. Whole tobacco plant (a) and leaves displaying disease severity at (b) T1G, (c) T2G, (d) T3G, and (e) T4G, respectively.
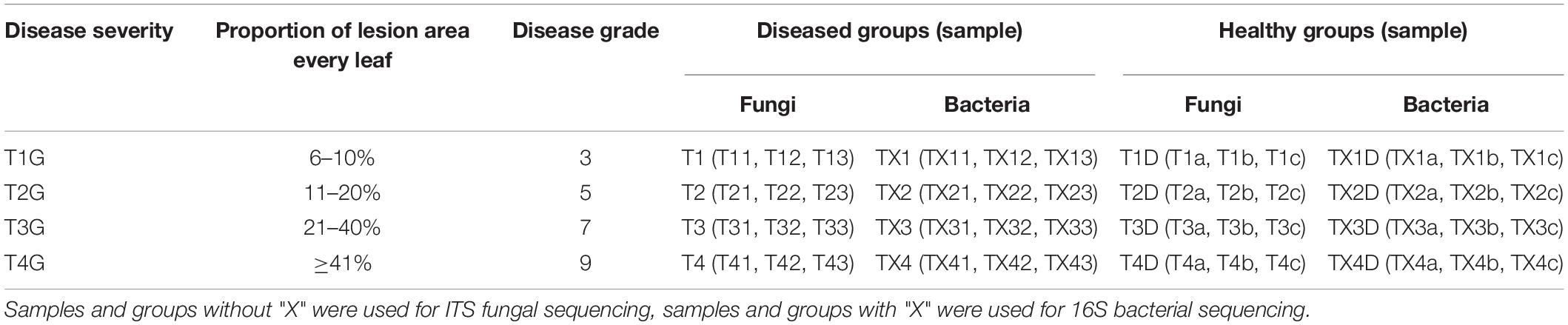
Table 1. Sample information for both diseased and healthy tobacco leaves infected by Didymella segeticola at different disease severity.
DNA Extraction, PCR Amplification, and High-Throughput Sequencing
Each of the 0.5 g samples (stored at −80°C) was ground and FastDNA® Spin kit for Soil (MP Biochemicals, Solon, OH, United States) was used according to the manufacturer’s protocol to extract the total DNA. Briefly, the powdered sample was added to Lysing Matrix E Tube, followed by the addition of sodium phosphate buffer (978 μL) and MT buffer (122 μL). After vortexing for 10–15 s, the samples were secured in FastPrep® Instrument and processed for 30 s at 5.5 speed, then supernatants were transferred to a clean tube, and PPS reagent (250 μL) was added. To obtain the pellet, centrifugation (14,000 × g for 5 min) was performed, then the same amount of binding matrix suspension was added. To allow binding of DNA to the matrix, tubes were inverted to let silica matrix settle, then the supernatant was discarded. The binding matrix with the remaining amount of supernatant was re-suspended and approximately 600 μL of that mixture was transferred to a SPINTM Filter and centrifuged for 1 min (at 14,000 × g). SEWS-M (500 μL) was added to the SPINTM Filter and centrifuged again (at 14,000 × g) for 1 min. After decanting the flow-through, SPINTM Filter was replaced in a collection tube and centrifuged at 14,000 × g for 2 min to “dry” the matrix of residual SEWS-M wash solution. A fresh collection tube was employed to place the SPINTM Filter in, then left for air drying at room temperature (5 min). DNase/Pyrogen Free Water (DES; 50 μL) was added and the matrix on the filter membrane was gently vortexed to re-suspend the silica for efficient elution of DNA. Centrifugation at 14,000 × g for 1 min was performed to transfer eluted DNA to the collection tube. The total DNA concentration was adjusted to 30 ng μL–1 (Zhang et al., 2018), and the purity A260:A280 ratio was regulated within 1.8–2.2 by NanoDrop ND-2000 (Thermo Fisher Scientific, Waltham, MA, United States) before conducting PCR (Xiang et al., 2020a).
Following total DNA extraction, the fungal ITS region was amplified using the eukaryotic primers ITS1F primer (5′-CTTGGTCATTTAGAGGAAGTAA-3′) and ITS2R (5′-GCTGCGTTCTTCATCGATGC-3′) (Xiang et al., 2020a). To amplify the V3-V4 hypervariable regions of the 16S rRNA gene, 338F (5′-ACTCCTACGGGAGGCAGCAG-3′) and 806R (5′-GGACTACHVGGGTWTCTAAT-3′) primer pair were used (Xiang et al., 2020b). The PCR (amplification of the ITS region or V3-V4 region) was performed with a total volume of 20 μL (4 μL of 5 × FastPfu Buffer, 2 μL of 2.5 mM dNTPs, 0.8 μL of each primer, 0.4 μL of FastPfu Polymerase, and 1 μL DNA). The fungal PCR reaction was performed on a peqSTAR thermal cycler (PEQLAB Ltd., United Kingdom) with the following settings: 94°C for 5 min, followed by 35 cycles of 94°C for 1 min, 57°C for 1 min, and 72°C for 1 min, and finally 5 min at 72°C. The 16S rRNA gene reactions included the following steps: 94°C for 3 min, followed by 30 cycles of 94°C for 45 s, 55°C for 45 s and 72°C for 90 s, and finally at 72°C for 7 min. PCR products were checked by 2% agarose gel electrophoresis and purified with Gene JET (Thermo Fisher Scientific, Waltham, MA, United States), then sent for sequencing (250 bp paired-end sequencing) according to the standard protocol. The Ion S5 XL platform (Thermo Fisher Scientific, Waltham, MA, United States) was used for high-throughput sequencing at Novogene Bioinformatics Technology Co., Beijing, China.
Data Processing
Single-end reads were generated (i.e., 400 bp/600 bp for fungi and 1,000 bp/1,200 bp for bacteria), followed by qualitative filtering and merging of raw reads by Cutadapt version 1.9.1.1 Afterward, the remaining unique reads were clustered into operational taxonomic units (OTUs) by UPARSE version 7.0.1001 software2 with a 97% similarity cutoff. Subsequently, the OTUs of each sample were annotated to different classifiers based on the UNITE version 7.2 fungal ITS and SILVA 132 rRNA database (Chen Q. L. et al., 2020; Xiang et al., 2020a). Finally, the estimates of diversity (Shannon and Simpson index), richness (Chao1 index), and beta diversity of microbial community were performed for different disease severity samples by Qiime software (Version 1.9.1). Generally, a higher Shannon and Simpson index represents a higher community diversity. In addition, higher Chao1 indices signify a higher community abundance (Zhang D. X. et al., 2019). Beta diversity on weighted and unweighted unifrac distance matrix was calculated by Qiime software (Version 1.9.1). Principal co-ordinate analysis (PCoA) was analyzed by WGCNA, stats, and ggplot2 package in R software (Version 1.9.1). Principal Component Analysis (PCA) was performed by using ade4 and ggplot2 packages in R software (Version 1.9.1). PCoA and PCA were used to determine the differences between community structures; samples with high similar communities tend to cluster together, on the contrary, samples become dispersed in the case of largely different communities (Zhang D. X. et al., 2019). Dilution curve, rank abundance curve, and species accumulation were drawn by R software (Version 2.15.3). The Venn diagrams were drawn by VennDiagram of software R (Version 3.0.3). FUNGuild database was used to analyze Fungal trophic mode (Nguyen et al., 2016). Bioinformatics software package PICRUSt was used to analyze bacterial OTUs for their metabolic functions according to Langille et al. (2013).
Statistical Analysis
IBM SPSS Statistics 23 (IBM Corp., New York, United States of America) was used to analyze the data and to compare the differences of alpha-diversity indexes of fungal and bacterial communities (Mao et al., 2020). The mean values were compared and P-value at ≤ 0.05 was considered to be statistically significant.
Results
Quality of Total Fungal and Bacterial Sequence Data
After quality control processing and de-noising, 1,713,851 fungal sequences were obtained from the 24 samples. The sequences were classified into 812 operational taxonomic units (OTUs) at a 97% similarity level. The fungal sequence of each sample was deposited in the SRA database under the accession number PRJNA714770. For bacteria, a total of 1,900,408 sequences were classified into 301 OTUs across the 24 samples. The bacterial sequence of each sample was deposited in the SRA database under the accession number PRJNA714984. When the number of sequences reached approximately 40,000, the rarefaction curves for all samples showed that they approached the plateau phase (Figure 2), suggesting that the sequence coverage accurately described the fungal and bacterial composition. For further analyses, the observed OTUs were used directly.
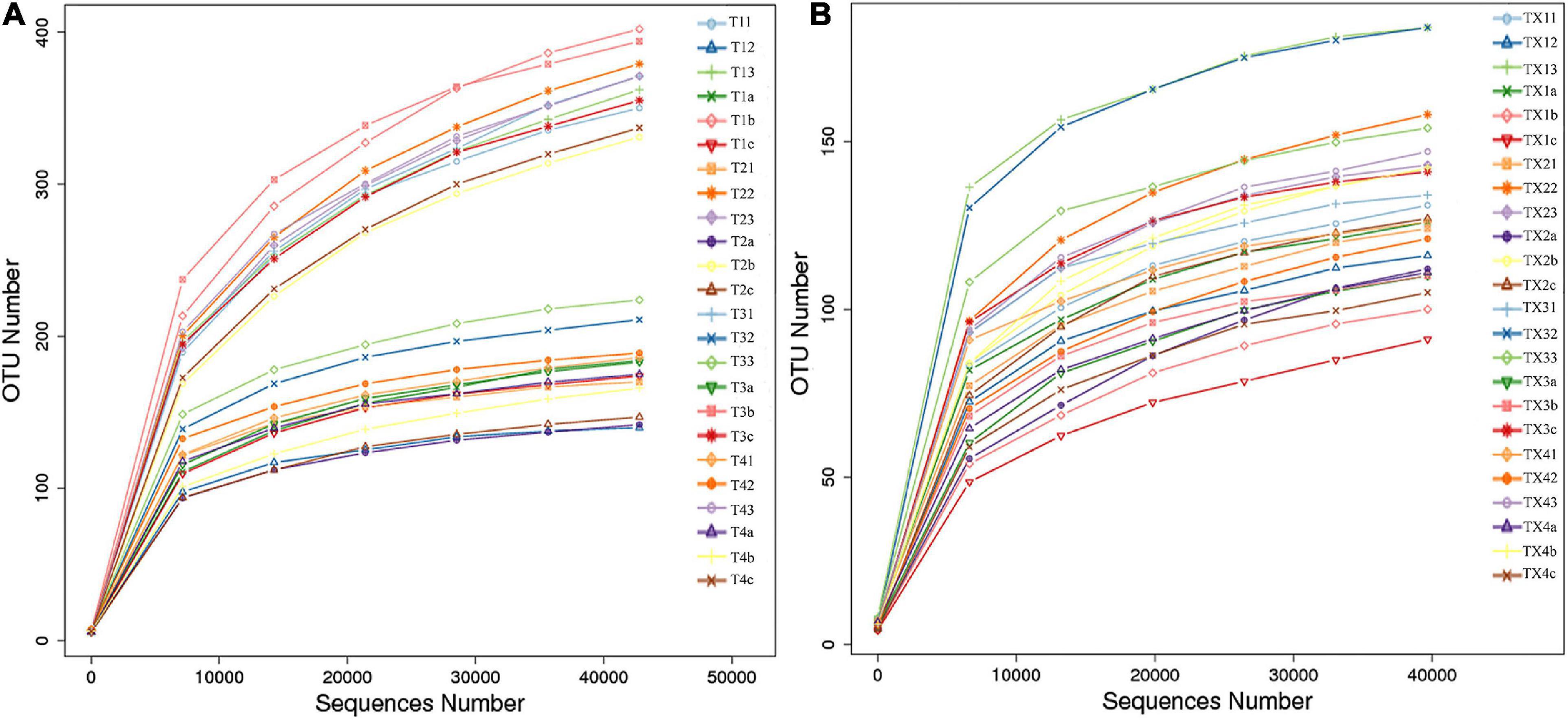
Figure 2. Rarefaction curves of fungal (A) and bacterial (B) OTUs across different tobacco leaf samples.
Fungal and Bacterial Operational Taxonomic Unit Distribution and Diversity
A total of 812 fungal OTUs were obtained in Venn diagrams, including 437 belonging to the diseased group and 583 to the healthy group (Figure 3). The number of shared OTUs among the 4 diseased and healthy groups was 79 and 166, respectively. There were 47, 79, 38, and 34 OTUs found only in the T1, T2, T3, and T4 of the diseased group, respectively. Meanwhile, the healthy group T1D, T2D, T3D, and T4D only had 61, 35, 72, and 40 unique OTUs, respectively. The number of fungal OTUs in the healthy groups was more than those of the diseased groups. The Shannon index of diseased and healthy samples ranged from 3.31 ± 0.04 (mean ± SD) to 3.83 ± 0.07 and 4.45 ± 0.48 to 5.47 ± 0.37, respectively, whereas the Simpson index ranged from 0.73 ± 0.05 to 0.77 ± 0.05 for diseased, and 0.88 ± 0.01 to 0.95 ± 0.02 for healthy samples. The Chao1 index ranged from 153 ± 66 to 227 ± 101 and 192 ± 98 to 303 ± 103 for diseased and healthy samples, respectively. Correspondingly, the Shannon, Simpson and Chao1 indices indicated that the diversity and richness of the fungal community in the healthy groups were higher than those in the diseased groups (Table 2).
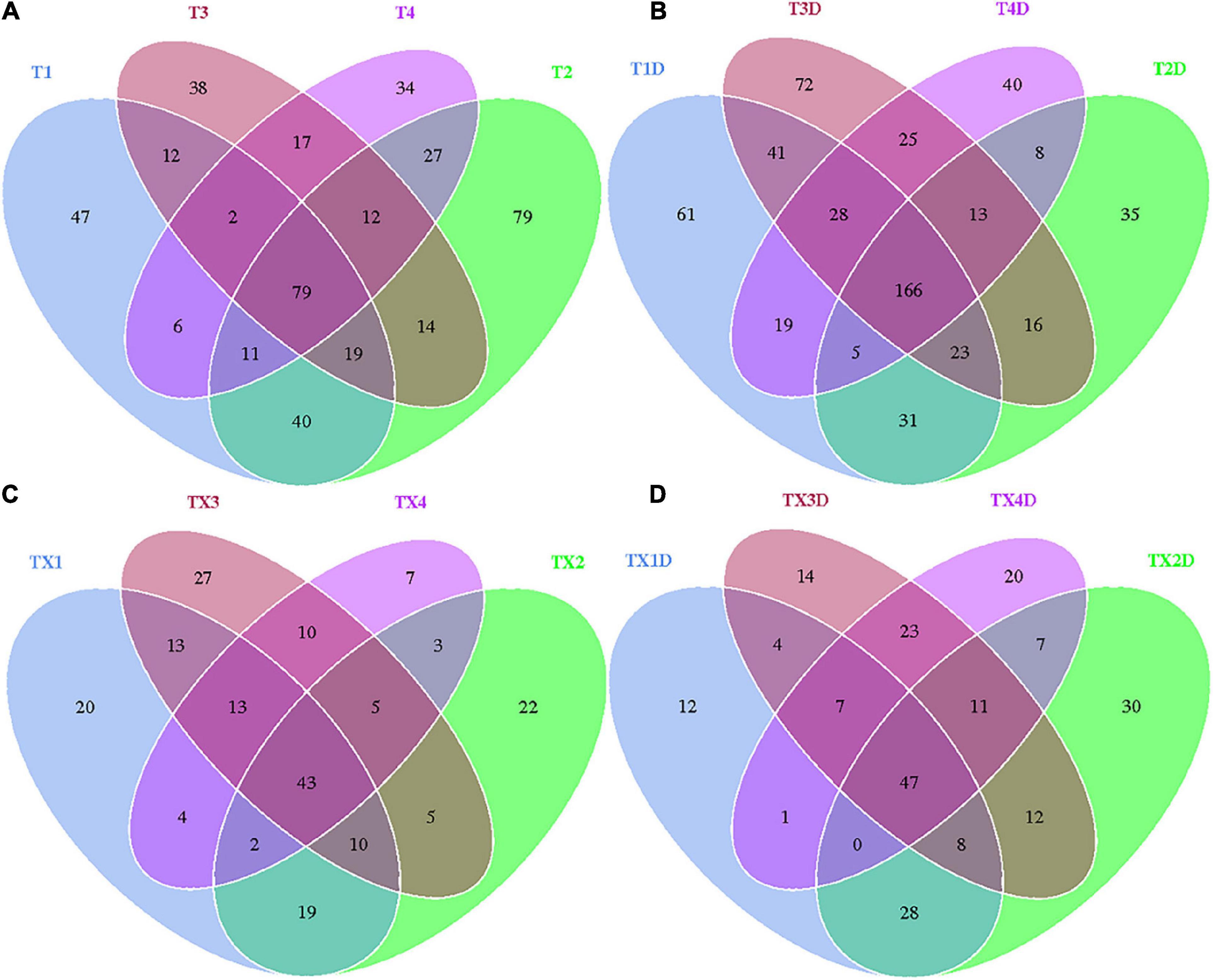
Figure 3. Venn diagram showing the number of fungal and bacterial OTUs detected in different groups at four different disease severity. Fungal Venn diagram in diseased (A) and healthy (B) groups at four different disease severities. Bacterial Venn diagram in diseased (C) and healthy (D) groups at four different disease severities. Numbers in the core section indicated shared OTUs for each group at four different disease severities. Numbers in the overlapping region indicated unique OTUs for two or three samples. Numbers in the non-overlapping regions indicated unique OTUs for the group.
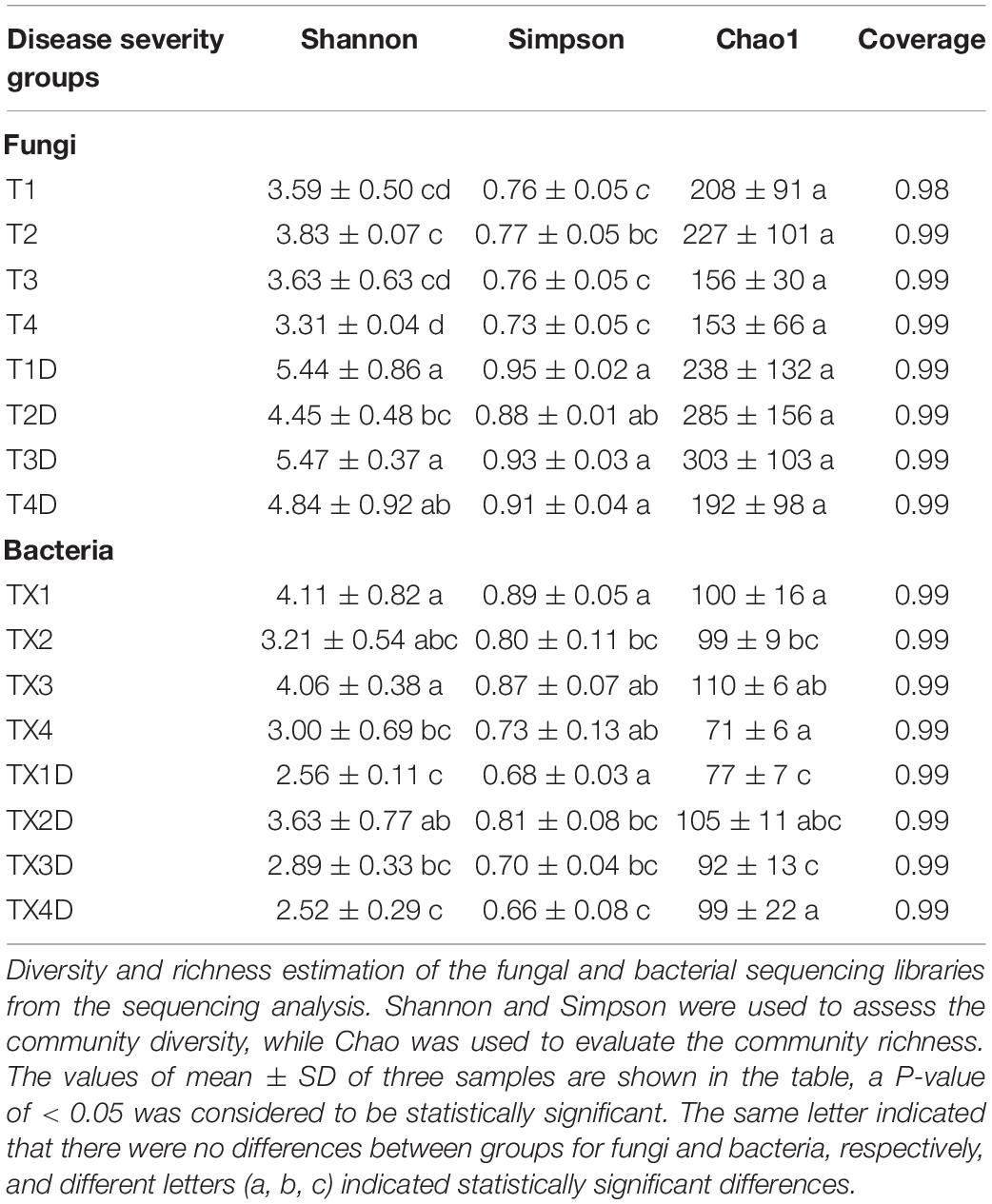
Table 2. Alpha-diversity indexes of fungal and bacterial community based on high-throughput sequencing in different samples.
In the case of bacterial communities, a total of 301 OTUs were obtained, including 203 belonging to the diseased group and 224 to the healthy group. There were 43 and 47 shared OTUs found in four diseased and healthy groups, respectively. The unique OTU numbers in diseased groups from TX1 to TX4 were 20, 22, 27, and 7, respectively, while in the healthy group the numbers were 12, 30, 14, and 20, respectively. Accordingly, the Shannon index of diseased and healthy samples ranged from 3.00 ± 0.69 to 4.11 ± 0.82 and 2.52 ± 0.29 to 3.63 ± 0.77, respectively. Moreover, the Simpson index ranged from 0.73 ± 0.13 to 0.89 ± 0.05 for diseased and 0.66 ± 0.08 to 0.81 ± 0.08 for healthy samples. The Chao1 index ranged from 71 ± 6 to 110 ± 6 and 77 ± 7 to 105 ± 11 for diseased and healthy samples, respectively (Table 2). In general, the diversity of the bacterial community in the healthy groups was lower than in diseased groups, except for TX2D.
Fungal and Bacterial Community Composition
Fungal Community Composition
The ITS dataset showed that a total of 55.67% OTUs could be classified into the phyla of Ascomycota, Basidiomycota, Mortierellomycota, Olpidiomycota, Chytridiomycota, Mucoromycota, Rozellomycota, Glomeromycota, Aphelidiomycota, and Monoblepharomycota (Figure 4A). For diseased and healthy groups, the dominant fungal phylum was Ascomycota, followed by Basidiomycota and Mortierellomycota. Combining leaf tissue types, the relative abundance of Ascomycota in all diseased and healthy samples were 85.8 and 51.0%, Basidiomycota were 1.2 and 4.6%, and Mortierellomycota were 0.3 and 2.0%, respectively. Combining leaf tissue types and disease severity levels, the relative abundance of Ascomycota in diseased group T1, T2, T3, and T4 were 80.1, 81.3, 89.6, and 92.3%, respectively, while in healthy group T1D, T2D, T3D, and T4D, the percentage (%) was 33.5, 52.9, 56.0, and 61.6, respectively. In contrast, the relative abundance of Basidiomycota was 1.0, 2.1, 1.1, and 0.6% in group T1, T2, T3, and T4, respectively; while group T1D, T2D, T3D, and T4D represented a percentage of 7.9, 2.7, 4.6, and 3.3%, respectively. The relative abundance of Mortierellomycota was 0.2, 0.6, 0.1, and 0.3% (in group T1, T2, T3, and T4, respectively); whereas, percent values of 3.7, 1.1, 1.8, and 1.5 were obtained for group T1D, T2D, T3D, and T4D, respectively. Overall, the results infer that the contents of Ascomycota in diseased tissues were higher than in healthy tissues. Conversely, the contents of Basidiomycota and Mortierellomycota in diseased tissues were less than those in healthy tissues. In addition, with the increase in disease severity, the relative abundance of Ascomycota increased regardless of tissues being diseased or healthy.
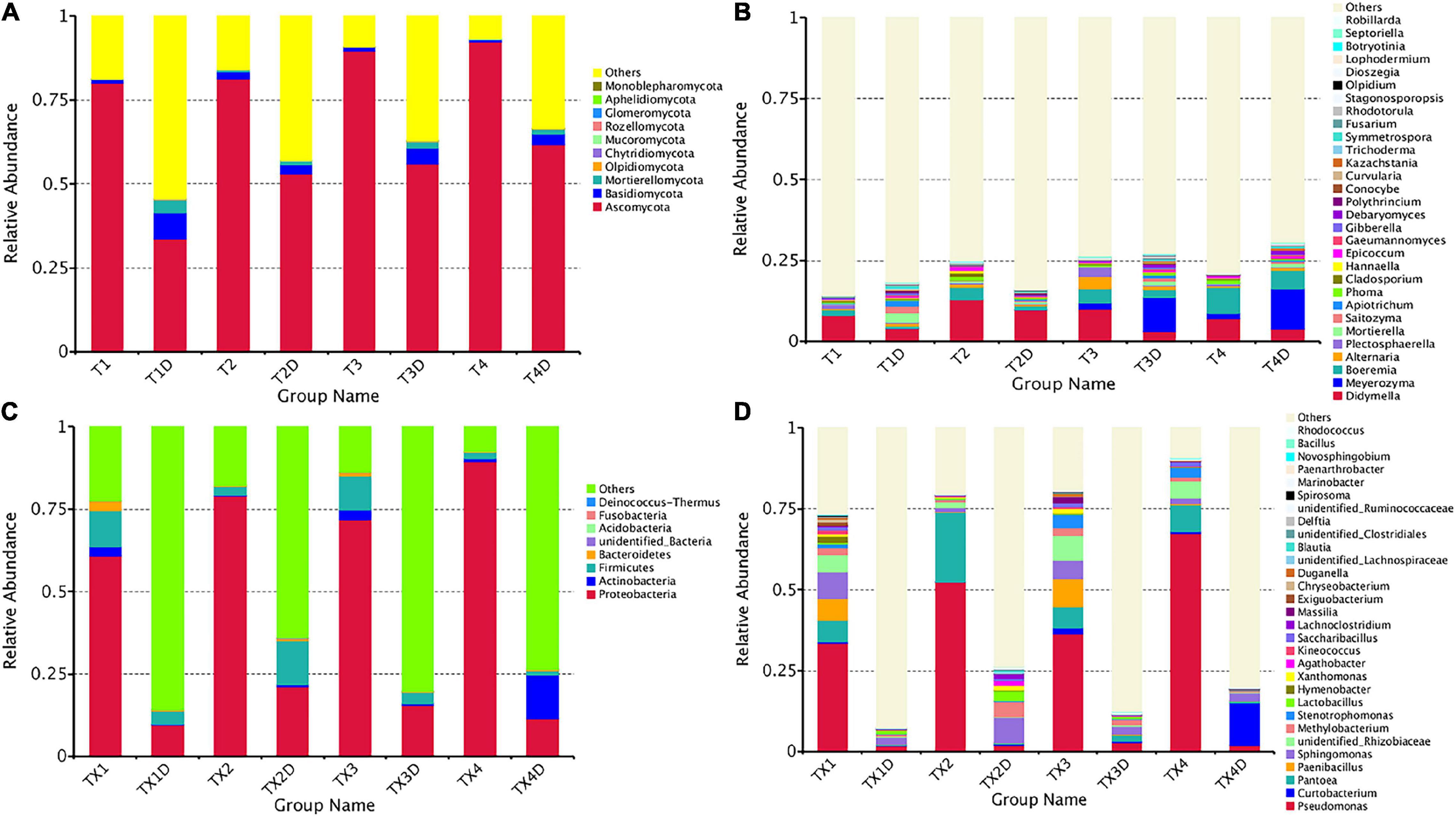
Figure 4. Microbial community composition of different groups at the phyla and genus levels. Fungal community composition of different groups at the phyla (A) and genus (B) levels. Bacterial community composition of different groups at the phyla (C) and genus (D) levels. Phyla making up less than 0.01 of total composition were classified as “Others”.
At the genus level, the 30 most common fungal genera are shown in Figure 4B. Among those, the top 10 genera were Didymella (with a relative abundance of 7.3%), Boeremia (3.5%), Alternaria (1.1%), Meyerozyma (6.6%), Plectosphaerella (0.8%), Mortierella (0.8%), Saitozyma (0.6%), Apiotrichum (0.4%), Phoma (0.5%), and Cladosporium (0.5%) (Table 3). Didymella was the dominant genus inboth diseased and healthy groups. A maximum-likelihood tree of the 100 most abundant genera showed that the dominant fungi belonged to Ascomycota, followed by Basidiomycota and Mortierellomycota. Didymella, Boeremia, Meyerozyma, Alternaria, Plectosphaerella, Phoma, Epicoccum, and Cladosporium were the dominant fungal genera of Ascomycota. For the Basidiomycota, the dominant fungal genera were Saitozyma, Apiotrichum, and Hannaella. For the Mortierellomycota, Mortierella was the dominant fungal genus (Figure 5A).
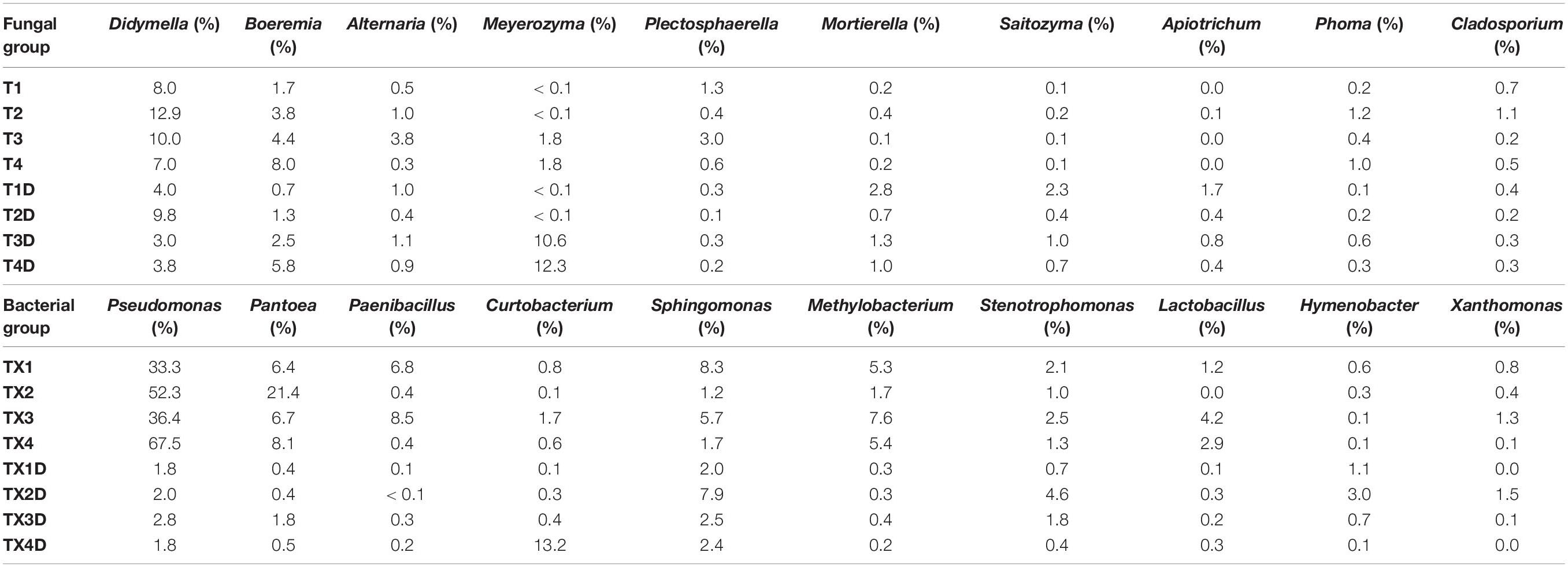
Table 3. List of top 10 dominant taxa and their relative abundance in the fungal and bacterial community of the group.
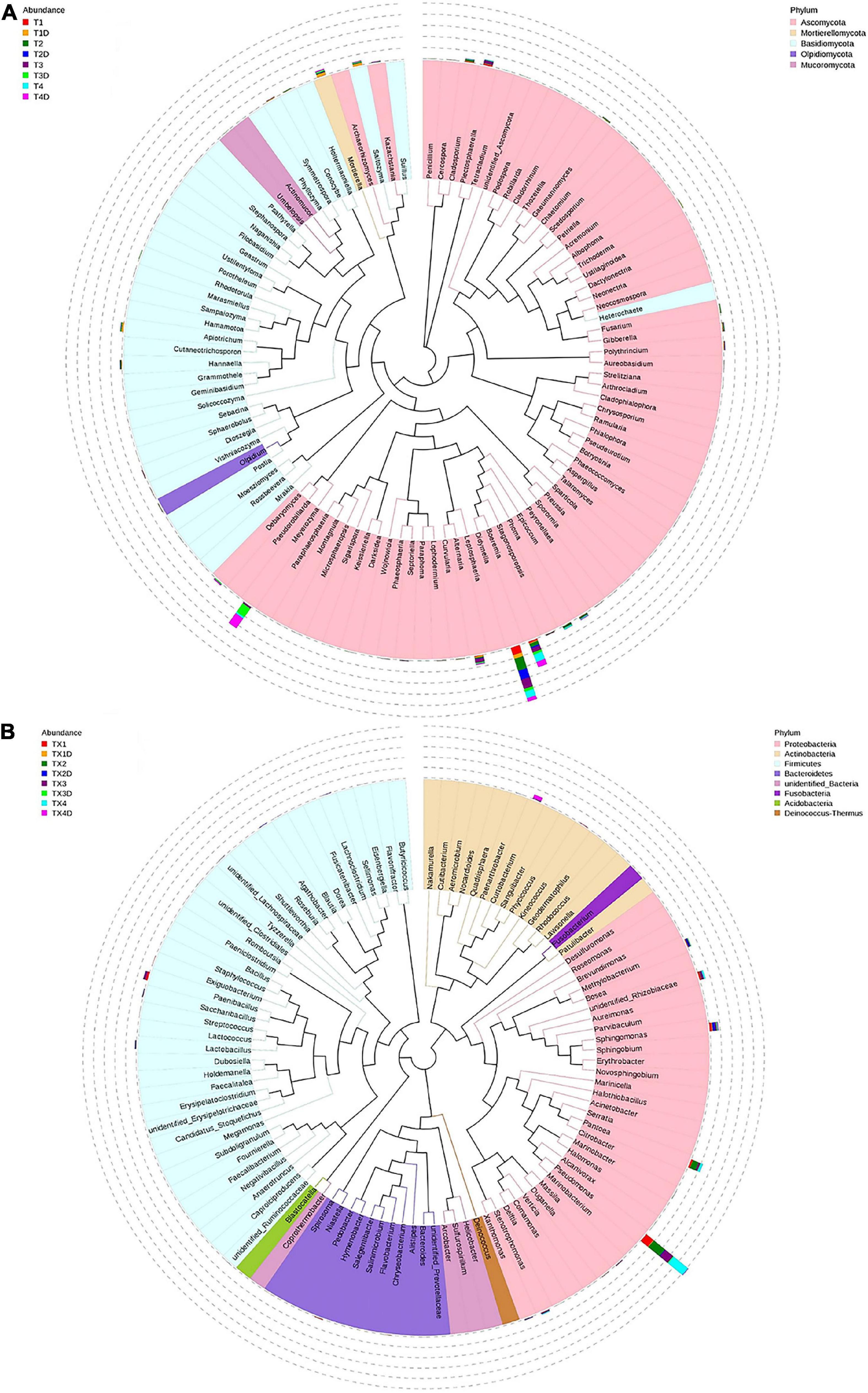
Figure 5. Maximum likelihood tree of the 100 most abundant fungal (A) and bacterial (B) genera in the eight group samples from tobacco leaves infected with D. segeticola. obtained by analysis of ITS rDNA and 16S rRNA pyrosequencing data. A color-coded bar plot shows the distribution of each fungal and bacterial genus in different groups.
The result related to the abundance of the top 10 fungal genera showed that Didymella in healthy groups was less compared to the diseased groups (Table 3). In diseased tissues, the relative abundance of Didymella increased with the progression of disease severity from T1G to T2G, and then decreased from T2G to T4G. Noticeably, healthy tissues exhibited the highest percentage of relative abundance for Didymella in T2D (9.8%), whereas for T1D, T3D, and T4D, it was found to be less (4.0%). Boeremia in healthy groups was less than those of the diseased groups, and with the increase of disease severity, the relative abundance of Boeremia increased. In diseased groups, the relative abundance of Alternaria increased proportionally with a disease severity level from T1G (0.5%) to T3G (3.8%), and then decreased in T4G (0.3%). In healthy groups, except for T2D (0.4%), the relative abundance of Alternaria was equal in T1D, T3D, and T4D. The relative abundance of Meyerozyma was higher in both diseased and healthy tissues of T3G and T4G. In contrast, Mortierella, Saitozyma, and Apiotrichum in healthy groups were higher than those of the diseased groups.
Bacterial Community Composition
The 16S dataset showed that a total of 94.68% OTUs could be classified in the Proteobacteria, Actinobacteria, Firmicutes, Bacteroidetes, Acidobacteria, Fusobacteria Deinococcus-Thermus, and unidentified-bacteria (Figure 4C). For diseased and healthy groups, the dominant bacterial phylum was Proteobacteria, followed by Firmicutes and Actinobacteria. Combining leaf tissue types, the relative abundance of Proteobacteria in all diseased and healthy samples were 75.2 and 14.4%, Firmicutes were 6.5 and 5.5%, and Actinobacteria were 1.8 and 3.7%, respectively. Combining tissue types and disease severity, the relative abundance of Proteobacteria in diseased group TX1, TX2, TX3, and TX4 were 60.7, 79.0, 71.8, and 89.4%, respectively, while in the healthy group TX1D, TX2D, TX3D, and TX4D were 9.6, 21.0, 15.4, and 11.5%, respectively. The relative abundance (%) of Firmicutes in diseased group TX1, TX2, TX3, and TX4 were 10.8, 2.6, 10.4, and 2.1, respectively; while in healthy group TX1D, TX2D, TX3D, and TX4D, were 3.9, 13.3, 3.4, and 1.3, respectively. Additionally, the relative abundance of Actinobacteria in diseased group TX1, TX2, TX3, and TX4 were 2.9, 0.3, 2.9, and 0.9%, respectively, while in healthy group TX1D, TX2D, TX3D, and TX4D were 0.2, 0.8, 0.6, and 13.2%, respectively.
In general, the results indicated that the contents of Proteobacteria and Firmicutes in diseased tissues were higher in comparison with healthy tissues. Relatively, the content of Actinobacteria in diseased tissues was less than those in healthy tissues. At the genus level, the 30 most common bacterial genera are shown in Figure 4D. The top 10 genera were Pseudomonas (24.7%), Pantoea (5.7%), Paenibacillus (2.4%), Curtobacterium (2.2%), Sphingomonas (4.0%), Methylobacterium (2.7%), Stenotrophomonas (1.8%), Lactobacillus (1.2%), Hymenobacter (0.8%), and Xanthomonas (0.5%) (Table 3). Pseudomonas and Pantoea were the dominant genera in the case of all diseased leaf groups, but not in the healthy groups. A maximum-likelihood tree of the 100 most abundant bacterial genera displayed that Pseudomonas, Pantoea, Sphingomonas, unidentified-Rhizobiaceae, and Methylobacterium were the dominant genera for the Proteobacteria, while Paenibacillus and Curtobacterium were the dominant bacterial genera for the Firmicutes and Actinobacteria, respectively (Figure 5B).
The abundance of the top 10 bacterial genera of all groups is presented in Table 3. The result revealed that Pseudomonas, Pantoea, Paenibacillus, and Methylobacterium in healthy groups were less than those of the diseased groups, indicating their prevalence in diseased tissues. In diseased groups, the relative abundance of Pseudomonas, Pantoea, Paenibacillus, and Methylobacterium ranged from 33.3 to 67.5%, 6.4 to 21.4%, 0.4 to 8.5%, and 1.7 to 7.6%, respectively, while in healthy groups, the abundance of Pseudomonas, Pantoea, Paenibacillus, and Methylobacterium was less than 3.0, 2, 0.3 and 0.4%, respectively.
Spatial Distribution of Fungal and Bacterial Communities
PCoA plots were used to determine the spatial distribution of fungal and bacterial communities (Figure 6). In fungal communities, all diseased samples from four different disease severity levels tended to cluster together, except for one distinctive fungal community, which was from the diseased samples at the T1G disease severity (T12). On the other hand, the communities of healthy groups were clearly separated from each other (Figure 6A). In bacterial communities, all diseased and healthy samples were respectively closer to each other (Figure 6B). These results suggest that there were significant differences between healthy and diseased tobacco leaves apropos of fungal and bacterial communities, respectively, as affected by the D. segeticola infection.
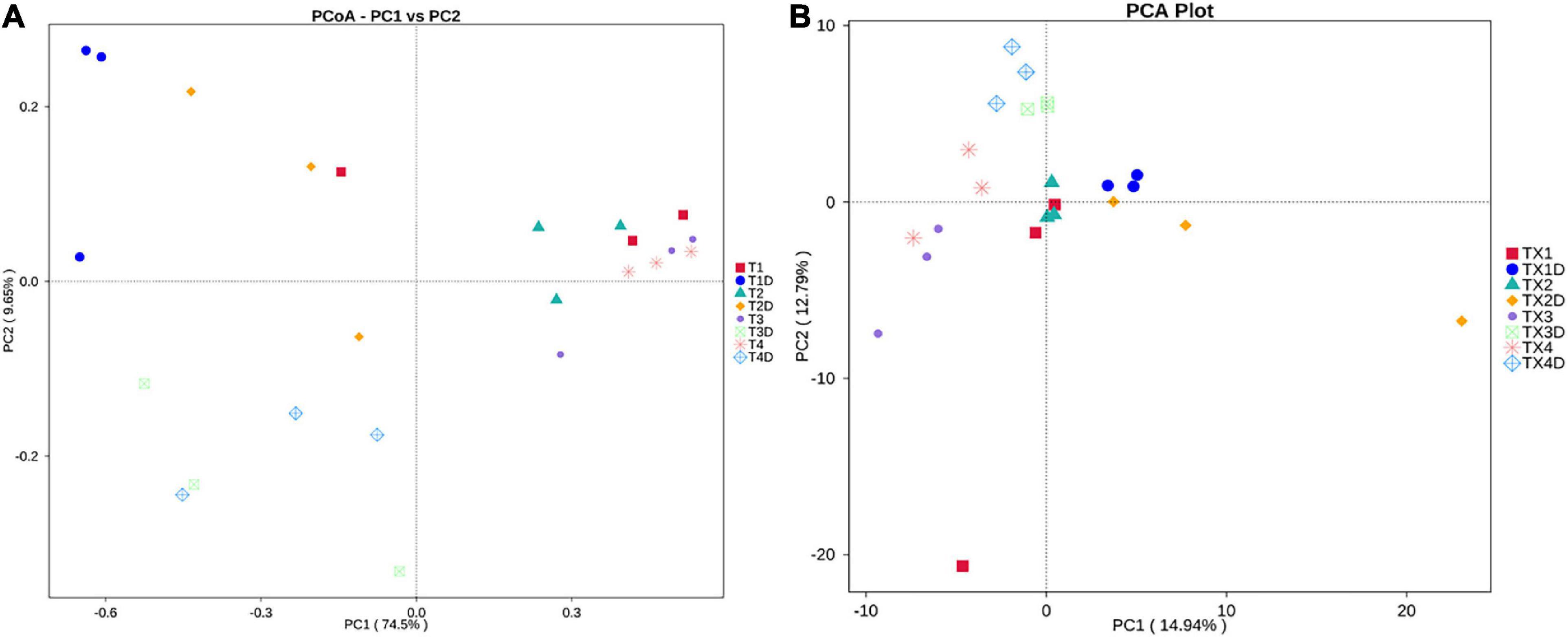
Figure 6. Principal Co-ordinate Analysis (PCoA) analysis of the fungal (A) and bacterial (B) communities in the different group samples.
Fungal and Bacterial Functional Characteristics
The fungal OTUs were categorized into nine groups according to their trophic mode. Pathotroph-saprotroph, pathotroph, and saprotroph were the dominant trophic modes in the composition of the tobacco leaves (Figure 7). Generally, the abundance of pathotroph-saprotroph and pathotroph in diseased groups was found to be higher than those of healthy groups, while this was not the case for saprotroph abundance. In diseased groups, the relative abundance of pathotroph and saprotroph increased along with the increase in disease severity from T1G to T4G. For the variation of bacterial functional categories, the gene sequence in diseased and healthy groups (as shown in Figures 8A,B), were mostly involved in metabolism functions, followed by genetic information processing and environmental information processing. Under the category of metabolism functions, amino acid metabolism, carbohydrate metabolism, and energy metabolism were highly enriched in diseased samples, whereas in the case of healthy samples, in addition to the aforementioned categories, the metabolism of cofactor and vitamins were also enriched significantly with a declining trend from TX1D to TX4D. For environmental information, membrane transport activity in diseased leaves followed the pattern: TX3 > TX4 > TX2 > TX1, while in the context of healthy ones, TX4D was observed to be enriched more than the other three categories. The terms, i.e., translation, replication, and repair, under the category of genetic information processing displayed a gradual decline from TX1 to TX4 in diseased samples, albeit the healthy samples exhibited higher enrichment for TX3D and TX4D than TX1D or TX2D. The relative abundance of gene sequences was not corrected with increased disease severity.
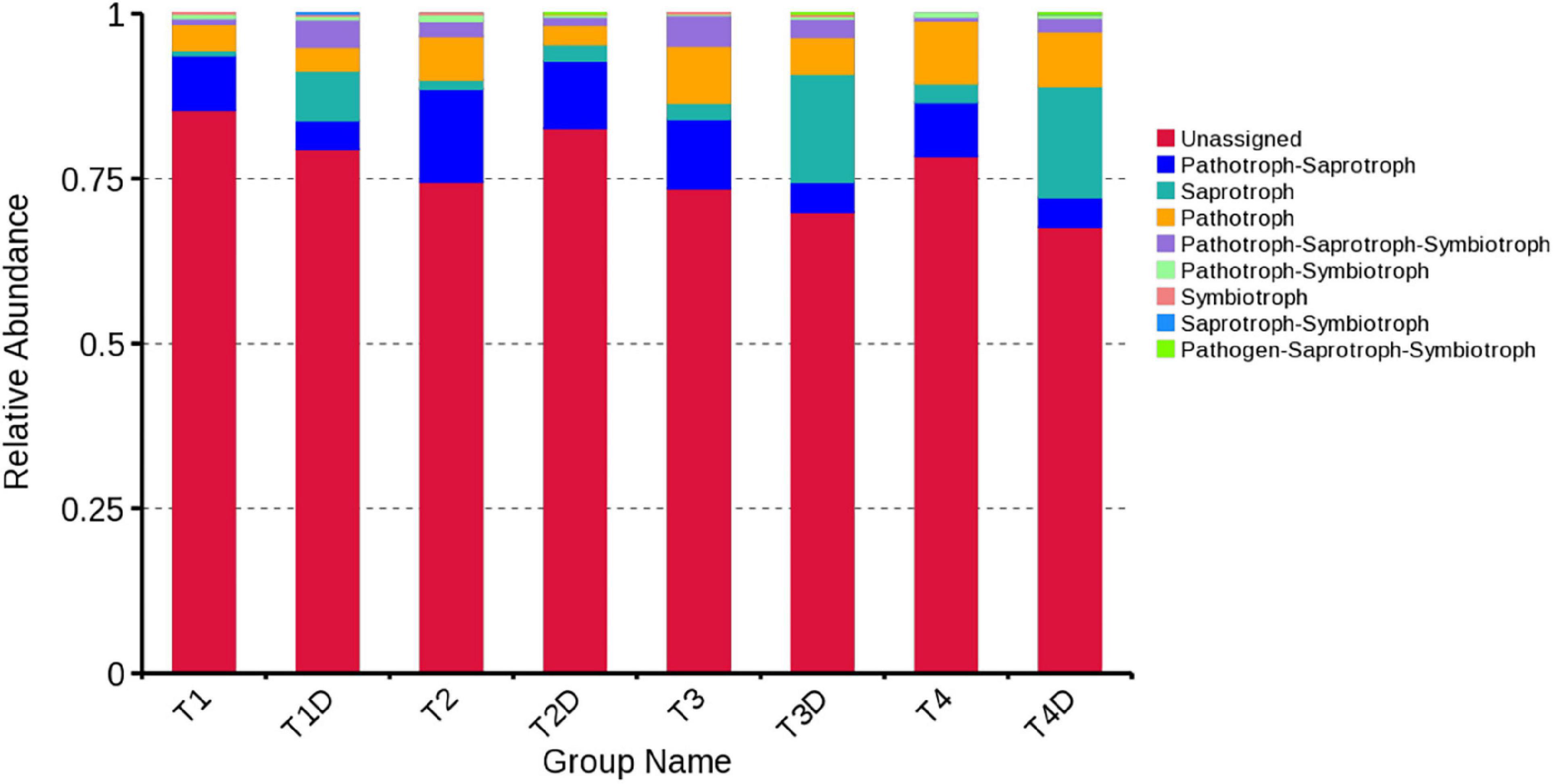
Figure 7. Relative abundance of fungal functional groups (guilds) based on OTU annotation table with disturbance frequency level.
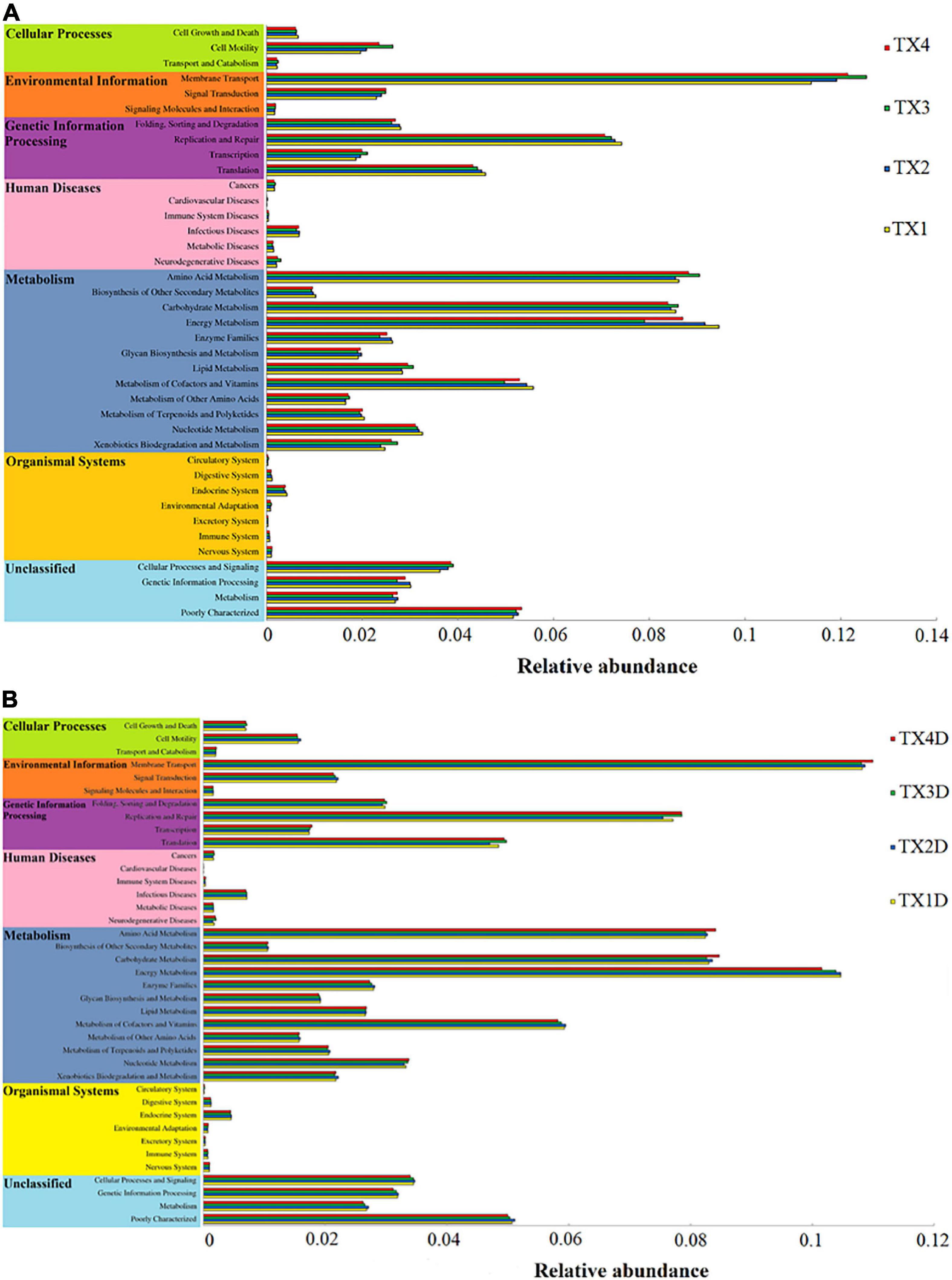
Figure 8. Variation of bacterial function categories in diseased (A) and healthy (B) groups at different disease severity analyzed by PICRUSt.
Discussion
In the present study, the ITS region of rDNA and V3-V4 hypervariable regions of the 16S rRNA were amplified to detect the dynamics of microbial diversity to different disease severity levels of D. segeticola infection, which manifests as leaf spots in tobacco. Tobacco leaves were separated into healthy and diseased categories for analysis; the relative abundance of fungi, i.e., Didymella and Boeremia (Ascomycota) and bacteria, i.e., Pseudomonas and Pantoea (Proteobacteria) were higher in diseased groups compared to healthy groups. Healthy tissues exhibited quite rich and diverse fungal communities compared with those of diseased groups. The analysis of Venn indicated that there were more fungal OTUs in the healthy groups than in diseased groups, which is in line with a previous study conducted by Rosenzweig et al. (2012) demonstrating a higher number of OTUs in the rhizosphere of healthy soil compared to diseased soil. Moreover, the number of fungal and bacterial OTUs was subject to change with increase in disease severity. In healthy groups, the dynamics of fungal OTUs were opposite to the bacterial OTUs with the disease severity advancement, which might be related to the factor of leaf nutrition leading toward the competitive relationship between microorganisms.
In our study, results suggested a higher fungal alpha diversity in healthy groups than diseased groups, which is in agreement with previous studies (Shang et al., 2016; Zhang Z. et al., 2019). The analysis revealed that the fungal alpha diversity of diseased groups decreased with an increased disease severity level from T2G to T4G. Research conducted by Manching et al. (2014) validates our results, where they reported an inverse correlation between alpha diversity and severity of southern leaf blight disease under immense disease pressure. The trend of fungal alpha diversity change was the same as the relative abundance of Didymella with increased disease severity in diseased groups, attributing the changes in fungal alpha diversity to the pathogen invasion. The ITS dataset showed that the fungal community of the tobacco phyllosphere was altered by disease severity. In both healthy and diseased groups, Ascomycota was the most dominant phylum, where its relative abundance increased gradually with disease severity level, a trend similar to which has been reported by Zhang et al. (2018) while working with pumpkin leaves affected by powdery mildew. At the genus level, fungal OTUs were mostly dominated by Didymella, followed by Boeremia and Alternaria. Didymella was the most dominant genus in all diseased groups and some earlier reports are consistent with this finding (Chen et al., 2019; Zhang Z. et al., 2019). Our study indicated that the highest relative abundance of this pathogen was observed in T2G rather than T4G, which contradicts with the study of Zhang et al. (2018) who indicated that the abundance of the pathogen genus Podosphaera was positively correlated with a spike in pumpkin powdery mildew disease severity. We corroborate that the parasitic ability of Didymella is inferior to Podosphaera, which might benefit the growth of other microorganisms post-Didymella genus invasion. Therefore, the relative abundance of Alternaria rose with an increase in disease severity from T1G to T3G and then decreased at T4G in diseased groups. It could be deduced that genus Alternaria could benefit from the disease pressure caused by Didymella. The relative abundance of Boeremia was found to be positively correlated with disease severity in diseased groups. Previous studies have reported the Boeremia genus as an endophytic fungus and a pathogen of tobacco (Ibrahim et al., 2017). It may be explained with the disease severity increase from T2G to T4G in the diseased group, where the relative abundance of Didymella decreased, while the relative abundance of Boeremia increased.
Tobacco phyllosphere fungal composition in our study was mostly identified as Pathotroph-saprotroph, saprotroph, and pathotroph. With disease severity increase, the trend of change in the relative abundance of pathotroph was the same as those of saprotroph in both diseased and healthy groups. This result indicated that there were many fungi with potential pathogenic characteristics on tobacco phyllosphere, as well as that most of those fungi are saprotrophs, which include genera whose species are well-known tobacco pathogens, for instance, Alternaria alternata (Wang et al., 2016), Didymella segeticola (Guo et al., 2020), Phoma sorghina, and Phoma omnivirens causing leaf spot on tobacco (Yuan et al., 2016; Jiang et al., 2018), as well as Cladosporium cladosporioides causing seed disease in tobacco (Wang et al., 2014). In general, most of these pathogens that infect tobacco leaves could grow both pathogenically inside the leaves or saprophytically on leaf surfaces. However, the abundance of microorganisms could be affected by many factors, such as sampling time, sampling place and plant species, etc. The 16S dataset showed that the bacterial alpha diversity became complicated with increased disease severity, owing to the tobacco leaf spot caused by D. segeticola, which may influence other fungal communities. The lowest bacterial alpha diversity under T4G in diseased groups may be attributed to the high disease pressure, inhibiting bacterial growth. Except for the T2G, the bacterial community diversity in the healthy groups was lower than those in the diseased groups. This result resonates with a previous study, where the bacterial diversity in the stem of black shank tobacco plants was found to be higher than that of healthy stems (Xiang et al., 2020b). The bacterial community diversity of TX2D was higher than the TX2, owing to the fact that T2G had a large Pantoea and Didymella population, consequently inhibiting the growth of other bacteria in the diseased part.
Regarding the bacterial community, Proteobacteria was the most abundant phylum in all groups and has been reported as a dominant bacterial taxon in many phyllospheric communities and in tobacco stems infected by fungal diseases (Liu et al., 2020; Xiang et al., 2020b). At the genus level, Pseudomonas and Pantoea were the dominant genera in diseased groups, which is in correspondence with an earlier report (Liu et al., 2020). In this study, the pattern of change in the relative abundance of Pseudomonas and Pantoea was the same as the Didymella from disease severity T1G to T3G in diseased groups. There may be a synergistic or antagonistic relationship between Didymella and two bacterial genera of Pseudomonas and Pantoea. Genus Pseudomonas potentially causes diseases in tobacco, such as wildfire (caused by Pseudomonas syringae pv. tabaci), and angular leaf spot (caused by Pseudomonas syringae pv. angula), and while looking at the bigger picture, D. segeticola and Pseudomonas syringae pv. tabaci could work hand-in-hand for their mutual benefit, as tobacco leaf spot caused by D. segeticola (Guo et al., 2020) might mix with tobacco wildfire or angular tobacco leaf spot (Cole, 1960; Zhu, 2002; Guo et al., 2017). More work should be conducted to verify this hypothesis. However, some studies reported that Pantoea spp. elicited a hypersensitive reaction in tobacco leaves (Mondal et al., 2011; Wang, 2018). The relative abundance of Pantoea in diseased groups were found higher than those of healthy groups, combined with the trend of change in the relative abundance of Pantoea being the same as the Didymella from disease severity T1G to T3G in diseased groups, supporting the evidence that the Pantoea could produce a hypersensitive reaction to prevent attack by pathogens. Results from PICRUSt analysis indicated that the metabolism of energy, cofactors, and vitamins declined with an increase in disease severity level from T1G to T3G in diseased groups, which may be associated with the factor of disease pressure. However, cell motility and membrane transport increased with disease severity level from T1G to T3G in diseased groups, the trend of change was the same as the relative abundance of phototrophs with increased disease severity from T1G to T3G. The results indicate that many microorganisms could provide corresponding material for pathogen growth. The reason why the cell motility and membrane transport were decreased at T4G may be attributed to some of the compounds produced by microorganisms which inhibit biofilm development in the diseased group. Morales et al. (2013) reported bacterial molecules such as Pseudomonas aeruginosa produced phenazines or compounds with similar activities inhibiting biofilm development.
Conclusion
The obtained data from this study helps to infer that the composition and diversity of phyllospheric microbiota differed markedly on D. segeticola-infected tobacco leaves at different disease severity levels, particularly in the case of the fungal community. Noticeably, in all tobacco leaf samples, the most dominant fungal phylum was Ascomycota with a high prevalence of genus Didymella, co-existing with bacterial phylum Proteobacteria with predominant genus Pseudomonas, however, their relative abundance was found to be higher in diseased groups compared to healthy counterparts. Conclusively, healthy tissues exhibited quite rich and diverse fungal communities in contrast with diseased groups. The infection of D. segeticola had a significant but varying effect on bacterial alpha-diversity. The relative abundance of pathotrophs and saprotrophs proportionally increased in diseased tissues with the progression of disease severity.
Data Availability Statement
The datasets presented in this study can be found in online repositories. The names of the repository/repositories and accession number(s) can be found in the article/supplementary material.
Author Contributions
YH, H-CW, and IS contributed to conception and design of the study. YH organized the database and performed the statistical analysis. YH and H-CW wrote the first draft of the manuscript. IS and ZL wrote sections of the manuscript. All authors contributed to manuscript revision, read, and approved the submitted version.
Funding
This research was supported by the China National Tobacco Corporation (110202001035(LS-04)), the National Natural Science Foundation of China (31960550 and 32160522), the Guizhou Science Technology Foundation (ZK[2021]Key036), the Guizhou Excellent Young Talent Platform ([2017]5619), the International Science and Technology Cooperation Base ([2020]4102), the Guizhou Provincial Academician Workstation of Microbiology and Health ([2020]4004), the Guizhou Tobacco Company (201914, 2020XM22, 2020XM03), the Sino-Pakistan Project NSFC (31961143008), the National Natural Science Foundation of China, International (Regional) Cooperation and Exchange Program, Research fund for International young scientists (31750110462), and the Jiangsu Collaborative Innovation Center for Modern Crop Production (JCIC-MCP) China.
Conflict of Interest
The authors declare that this study received funding from Guizhou Tobacco Company Project. The funder was not involved in the study design, collection, analysis, interpretation of data, the writing of this article or the decision to submit it for publication.
Publisher’s Note
All claims expressed in this article are solely those of the authors and do not necessarily represent those of their affiliated organizations, or those of the publisher, the editors and the reviewers. Any product that may be evaluated in this article, or claim that may be made by its manufacturer, is not guaranteed or endorsed by the publisher.
Footnotes
References
Chen, Q. L., Cai, L., Wang, H. C., Cai, L. T., Goodwin, P., Ma, J., et al. (2020). Fungal composition and diversity of the tobacco leaf phyllosphere during curing of leaves. Front. Microbiol. 11:554051. doi: 10.3389/fmicb.2020.554051
Chen, Q. L., Li, Z., Wang, H. C., Huang, Y., Cai, L. T., Xie, H. L., et al. (2019). Fungal composition and diversity of tobacco phyllosphere from cured tobacco leaves. Acta Microbiol. Sin. 59, 2401–2409. doi: 10.13343/j.cnki.wsxb.20190065
Chen, Q., Zhang, K., Zhang, G. Z., and Cai, L. (2015). A polyphasic approach to characterise two novel species of Phoma (Didymellaceae) from China. Phytotaxa 197, 267–281. doi: 10.11646/phytotaxa.197.4.4
Chen, X. Y. L., Wicaksono, W. A., Berg, G., and Cernavaet, T. (2020). Bacterial communities in the plant phyllosphere harbour distinct responders to a broad-spectrum pesticide. Sci. Total Environ. 751, 141799. doi: 10.1016/j.scitotenv.2020.141799
Cole, J. S. (1960). Field spray trials against wildfire and angular leaf spot of tobacco in Rhodesia. Ann. Appl. Biol. 48, 291–298. doi: 10.1111/j.1744-7348.1960.tb03525.x
Espenshade, J., Thijs, S., Gawronski, S., Boye, H., Weyens, N., and Vangronsveld, J. (2019). Influence of urbanization on epiphytic bacterial communities of the Platanus × hispanica tree leaves in a biennial study. Front. Microbiol. 10:675. doi: 10.3389/fmicb.2019.00675
Guo, Y. S., Su, X. K., Cai, L. T., and Wang, H. C. (2017). Phenotypic characterization of pseudomonas syringae pv. tabaci, the causal agent of tobacco wildfire. J. Plant Pathol. 99, 499–504.
Guo, Z. N., Xie, H. L., Wang, H. C., Huang, Y., Chen, Q. L., Xiang, L. G., et al. (2020). Leaf Spot Caused by Didymella segeticola on tobacco in China. Plant Dis. 104, 1559–1560.
Huang, Y., Wang, H. C., Chen, Q. L., Xiang, L. G., Guo, Z. N., Li, Z., et al. (2020). Community structure and diversity of fungi in leaves of tobacco plant infected with powdery mildew. Guizhou Agri. Sci. 48, 54–58.
Ibrahim, M., Sieber, T. N., and Schlegel, M. (2017). Communities of fungal endophytes in leaves of Fraxinus ornus are highly diverse. Fungal Ecol. 29, 10–19. doi: 10.1016/j.funeco.2017.05.001
Jiang, Y., Sang, W. J., Zeng, E. L., Wang, Y., Wang, D. F., and Qin, K. (2018). Identification of pathogenic fungus causing tobacco black spot stalk in Guizhou Province. Jiangsu Agric. Sci. 10, 92–95.
Langille, M. G., Zaneveld, J., Caporaso, J. G., Mcdonald, D., Knights, D., Reyes, J., et al. (2013). Predictive functional profiling of microbial communities using 16S rRNA marker gene sequences. Nat. Biotechnol. 31, 814–821. doi: 10.1038/nbt.2676
Li, Y., Feng, Y. W., Yu, Y., Ren, D., Ye, Y., and Li, M. Q. (2018). A bioinformatics study on the application of high-throughput sequencing technology to pathogen detection in patients with sepsis. J. Med. Imaging Health Inform. 8, 344–350. doi: 10.1166/jmihi.2018.2281
Lindow, S. E., and Brandl, M. T. (2003). Microbiology of the phyllosphere. Appl. Environ. Microbiol. 69, 1875–1883.
Liu, C., Wang, H. C., Xie, H. L., Chen, Q. L., Yu, Z. H., and Sun, G. J. (2019). Fungal community structure analysis of tobacco leaf infected with brown spot disease. Guizhou Agric. Sci. 47, 54–59.
Liu, C., Wang, H. C., Xie, H. L., Xiang, L. G., Huang, Y., Chen, Q. L., et al. (2020). Biodiversity analysis of phyllosphere bacterial genus from tobacco leaves infected by brown spot disease. Tobacco Sci. Technol. 53, 8–14.
Liu, L. P., Qu, J. W., Yi, X. Q., and Huang, H. H. (2017). Genome-wide identification, classification and expression analysis of the mildew resistance locus o (MLO) gene family in sweet orange (citrus sinensis). Braz. Arch. Biol. Technol. 60, 1678–4324.
Liu, T. B., Gu, Y. B., Zhou, Z. C., Liu, Z. H., Yin, H. Q., Qin, C., et al. (2021). Ecological strategies of biological and chemical control agents on wildfire disease of tobacco (Nicotiana tabacum L.). BMC Microbiol. 21:184. doi: 10.1186/s12866-021-02237-8
Luo, L. Y., Zhang, Z., Jin, D. C., Tan, X. Q., Zhang, D. Y., Tian, Y., et al. (2017). Phyllosphere bacterial diversities and community structures on pumpkin with different severities of powdery mildew. Acta Phytopathol. Sin. 47, 688–695.
Luo, L. Y., Zhang, Z., Wang, P., Han, Y. Q., Jin, D. C., Su, P., et al. (2019). Variations in phyllosphere microbial community along with the development of angular leaf-spot of cucumber. AMB Express 9:76. doi: 10.1186/s13568-019-0800-y
Manching, H. C., Balint-Kurti, P. J., and Stapleton, A. E. (2014). Southern leaf blight disease severity is correlated with decreased maize leaf epiphytic bacterial species richness and the phyllosphere bacterial diversity decline is enhanced by nitrogen fertilization. Front. Plant Sci. 5:403. doi: 10.3389/fpls.2014.00403
Mao, H. L., Wang, K., Wang, Z., Peng, J., and Ren, N. Q. (2020). Metabolic function, trophic mode, organics degradation ability and influence factor of bacterial and fungal communities in chicken manure composting. Bioresour. Technol. 302, 122883. doi: 10.1016/j.biortech.2020.122883
Mboowa, G., Sserwadda, I., Amujal, M., and Namatovu, N. (2018). Human genomic loci important in common infectious diseases: role of high-throughput sequencing and genome-wide association studies. Can. J. Infect. Dis. Med. Microbiol. 6010, 1–9. doi: 10.1155/2018/1875217
Mondal, K. K., Mani, C., Singh, J., Kim, J. G., and Mudgett, M. B. (2011). A new leaf blight of rice caused by Pantoea ananatis in India. Plant Dis. 95, 1582–1583. doi: 10.1094/PDIS-06-11-0533
Morales, D. K., Grahl, N., Okegbe, C., Dietrich, L. E. P., Jacobs, N. J., and Hogan, D. A. (2013). Control of candida albicans metabolism and biofilm formation by Pseudomonas aeruginosa phenazines. mBio 4:e00526-12. doi: 10.1128/mBio.00526-12
Nguyen, N. H., Song, Z. W., Bates, S. T., Branco, S., Tedersoo, L., Menke, J., et al. (2016). FUNGuild: an open annotation tool for parsing fungal community datasets by ecological guild. Fungal Ecol. 20, 241–248. doi: 10.1016/j.funeco.2015.06.006
Rosenzweig, N., Tiedje, J. M., Quensen, J. F., Meng, Q. X., and Hao, J. J. (2012). Microbial communities associated with potato common scab-suppressive soil determined by pyrosequencing analyses. Plant Dis. 96, 718–725. doi: 10.1094/PDIS-07-11-0571
Shang, Q., Yang, G., Wang, Y., Wu, X. K., Zhao, X., Hao, H., et al. (2016). Illumina-based analysis of the rhizosphere microbial communities associated with healthy and wilted Lanzhou lily plants grown in the field. World J. Microbiol. Biotechnol. 32, 95. doi: 10.1007/s11274-016-2051-2
Singh, P., Santoni, S., Weber, A., This, P., and Peros, J. P. (2019). Understanding the phyllosphere microbiome assemblage in grape species (Vitaceae) with amplicon sequence data structures. Sci. Rep. 9:14294. doi: 10.1038/s41598-019-50839-0
Song, W. F., Li, M. C., and Gao, Z. (2017). Research progress on in situ detection methods of microorganisms. Biotechnol. Bull. 33, 26–32.
Speranskaya, A. S., Krinitsina, A. A., Shipulin, G. A., Khafizov, K. F., and Logacheva, M. D. (2018). High-throughput sequencing for the authentication of food products: problems and perspectives. Russ. J. Genet. 54, 1003–1012. doi: 10.1134/S1022795418090132
Wang, H. C., Huang, Y. F., Wang, J., Chen, X. J., Wei, K. S., Wang, M. S., et al. (2016). Activities of azoxystrobin and difenoconazole against Alternaria alternata and their control efficacy. Crop Prot. 90, 54–58. doi: 10.1016/j.cropro.2016.08.022
Wang, H. C., Wang, J., Li, W. H., Huang, Y. F., Xia, H. Q., Wang, M. S., et al. (2014). Cladosporium cladosporioides identified in China on tobacco seeds. Plant Dis. 98, 1002–1002. doi: 10.1094/PDIS-12-13-1203-PDN
Wang, Q. (2018). Isolation and Identification of a New Pathogen Pantoea Ananatis Causing Rice Leaf Blight. Hefei: Anhui Agricultural University.
Xiang, L. G., Wang, H. C., Zheng, P., Cai, L. T., and Yu, Z. H. (2020c). Analysis of endophytic fungi and phyllosphere fungi of flue cured tobacco leaves with brown spot disease. Acta Tabacaria Sin. 26, 93–100.
Xiang, L. G., Guo, H., Zhou, H., Xie, H. L., Cai, L. T., Wang, H. C., et al. (2020a). Fungal community structure and diversity of the rhizosphere soil and stems of healthy and diseased tobacco plants infected with bacterial wilt. Plant Prot. 46, 189–196,228.
Xiang, L. G., Guo, H., Zhou, H., Xie, H. L., Wang, H. C., Cai, L. T., et al. (2020b). Bacterial community structure and diversity of rhizosphere soil and stem of healthy and black shank tobacco plants. Acta Tabacaria Sin. 26, 100–108.
Xin, X. F., Nomura, K., Aung, K., Aung, K., Velasquez, A. C., Yao, J., et al. (2016). Bacteria establish an aqueous living space in plants crucial for virulence. Nature 539, 524–529. doi: 10.1038/nature20166
Yuan, G. Q., Liao, T., Tan, H. W., Li, Q. Q., and Lin, W. (2016). First report of leaf spot caused by Phoma sorghina on tobacco in China. Plant Dis. 100, 1790–1790. doi: 10.1094/PDIS-11-15-1377-PDN
Zhang, D. X., Yang, X. M., Li, D. K., Li, L., Zhang, D. P., and Peng, Y. H. (2019). Effects of different modes of delivery and feeding on intestinal flora of newborns and infants with different ages. Iran. J. Pediatr. 29:e88329. doi: 10.5812/ijp.88329
Zhang, Y. J., Skaar, I., Sulyok, M., Liu, X. Z., Rao, M. Y., and Taylor, J. W. (2016). The microbiome and metabolites in fermented Pu-erh tea as revealed by high-throughput sequencing and quantitative multiplex metabolite analysis. PLoS One 11:e0157847. doi: 10.1371/journal.pone.0157847
Zhang, Z., Kong, X., Jin, D. C., Yu, H., Zhu, X., Su, X. F., et al. (2019). Euonymus japonicus phyllosphere microbiome is significantly changed by powdery mildew. Arch. Microbiol. 201, 1099–1109. doi: 10.1007/s00203-019-01683-3
Zhang, Z., Luo, L. Y., Tan, X. Q., Kong, X., Yang, J. G., Wang, D. H., et al. (2018). Pumpkin powdery mildew disease severity influences the fungal diversity of the phyllosphere. PeerJ 6:e4559. doi: 10.7717/peerj.4559
Zhao, X. Z., Wang, Y., Li, D. X., Ren, Y. F., and Chen, Z. (2018). Morphological characterization and phylogenetic analysis of the pathogen Phoma segeticola var. camelliae causing a new tea disease. Acta Phytopathol. Sin. 48, 556–559.
Keywords: microbial diversity, disease severity, Didymella segeticola, tobacco, high-throughput sequencing
Citation: Huang Y, Wang H-C, Cai L-T, Li W, Pan D, Xiang L, Su X, Li Z, Adil MF and Shamsi IH (2021) Phyllospheric Microbial Composition and Diversity of the Tobacco Leaves Infected by Didymella segeticola. Front. Microbiol. 12:699699. doi: 10.3389/fmicb.2021.699699
Received: 24 April 2021; Accepted: 08 September 2021;
Published: 14 October 2021.
Edited by:
Puneet Singh Chauhan, National Botanical Research Institute (CSIR), IndiaReviewed by:
Nikolaos Remmas, Democritus University of Thrace, GreeceAnandham Rangasamy, Tamil Nadu Agricultural University, India
Kunal Singh, Institute of Himalayan Bioresource Technology (CSIR), India
Copyright © 2021 Huang, Wang, Cai, Li, Pan, Xiang, Su, Li, Adil and Shamsi. This is an open-access article distributed under the terms of the Creative Commons Attribution License (CC BY). The use, distribution or reproduction in other forums is permitted, provided the original author(s) and the copyright owner(s) are credited and that the original publication in this journal is cited, in accordance with accepted academic practice. No use, distribution or reproduction is permitted which does not comply with these terms.
*Correspondence: Han-Cheng Wang, eGlhb2JhaXlhbmcxMjZAaG90bWFpbC5jb20=; Zhong Li, emhvbmd6aG9uZ2xpZ3p1QDE2My5jb20=; Imran Haider Shamsi, ZHJpbXJhbkB6anUuZWR1LmNu