- 1College of Bioengineering, Sichuan University of Science and Engineering, Zigong, China
- 2Key Laboratory of Brewing Biotechnology and Application, Sichuan Province, Sichuan University of Science and Engineering, Zigong, China
- 3Sichuan Tuopai Shede Liquor Co., Ltd., Suining, Sichuan
Daqu is an important saccharifying and fermenting agent. It provides various microorganisms and enzymes for the fermentation of Baijiu and plays a vital role in the formation of Baijiu flavor. However, it is difficult to obtain information on microbial growth and metabolism in time for Daqu production. Therefore, the “Qu Xiang” obtained by smelling is an important index in the traditional production process to evaluate the microbial fermentation in the process of Daqu-making, “Qu Xiang” mainly represents the volatile flavor compounds in Daqu. The microbial diversity and volatile metabolites on 0, 6, 16, and 29 days of the fermentation process were measured using high-throughput sequencing and gas chromatography–mass spectrometry. Significant differences were found in the composition of the microbial community. Pseudomonas, Weissella, Bacillus, and Pelomonas were the main bacterial genera. Alternaria, Rhizopus, and Pichia are the main fungal genera. A total of 32 differential volatile metabolites were detected in samples at four time points using differential metabolic analysis. The correspondence of prevailing microorganisms with differential metabolites distinguished by Spearman correlation and two-way orthogonal partial least square analysis show that Saccharopolyspora exhibited a significant connection for the 12 differential metabolites. A significant positive correlation was observed between Rhizomucor and 13 different metabolites. These findings further understanding of the metabolism of microorganisms in Daqu fermentation and also help to control the microorganisms in the Daqu-making process, to obtain more stable Baijiu products.
Introduction
Fermentation is a traditional strategy that has been used to process and preserve foods all over the world. It has significance in cultural heritage and provides local economic products and rich microbiological resources (Liu and Tong, 2017). Chinese baijiu is a kind of prevalent beverage that is enjoyed around the world. Nongxiang baijiu is the most famous type and has a long history. The annual output accounts for more than 70% of Chinese liquor as a result of its unique flavor and aroma (Zou et al., 2018). Nongxiang Baijiu is brewed by solid-state fermentation, in which the production of Daqu and grain fermentation is mainly involved. Its characteristics are acquired from Daqu, a traditional fermentation starter (Du et al., 2019). Daqu is produced from raw materials in an open environment without sterilizing. During this period process, liquefaction enzymes, saccharifying enzymes, proteases, and esterifying enzymes were accumulated through microbial metabolism (Wu et al., 2020). In Baijiu liquor, the starch and protein in the Daqu can be degraded into small molecules by the aforementioned enzymes, hence serving as nutrients and substrates for microbial growth and flavor metabolism (He et al., 2020). These enzymes are mainly produced by some specific microorganisms, such as Aspergillus, Rhizopus, Bacillus, LactoBacillus, Wickerhamomyces, and Saccharomycoposis (Liu and Sun, 2018; Li et al., 2020). The interaction between microbes, microorganisms, and the environment in the manufacturing process of Daqu making eventually forms a Daqu microbial group that is involved in the subsequent Baijiu brewing (Chen et al., 2020; Fan et al., 2020). Previous research found that the proportion of bacteria and fungi from Daqu was 81.5% and 79.2% respectively, in the fermented grain (Wang P. et al., 2017). They not only affect the accumulation of enzymes in the fermentation process but also determine the final community composition (Wang et al., 2021). Therefore, the Daqu microorganism group has a crucial effect on the quality formation of Nongxiang Baijiu. If we master the rule of microbial succession in the production process of Nongxiang Daqu, we can get high-quality Daqu.
However, it is difficult to obtain timely information on microbial growth and metabolism in time and quickly during the production process (Fan et al., 2019). Therefore, the “Qu Xiang” obtained by smelling is an important index to evaluate microbial fermentation in the process of Daqu making. As a sensory index, “Qu Xiang” mainly represents the volatile flavor compounds in Daqu. Meanwhile, metabolism is the most downstream of life activities and the change in the upstream signal can be easily reflected in the metabolic phenotype through the cascade amplification effect, so metabolism has high sensitivity as a marker (Mousavian et al., 2016; Noda-Garcia et al., 2018; Slot and Gluck-Thaler, 2019). It was reported that the volatile components of Daqu exhibit diversity. For instance, a previous study shows that hexanal, 4-guaiacol, and phenylacetaldehyde are crucial aroma-active components in Daqu, which may be related to the Daqu quality. There are multiple and diverse species inhabiting Daqu, LactoBacillus, Bacillus, Aspergillus, and some Saccharomyces genera (saccharomycoposis, Wickerhamomyces, and Pichia) dominate different types of Daqu (Xiao et al., 2017; Jin et al., 2019). Researchers have learned little about the change of the microbial communities that are associated with flavor formation during the production of Daqu and how these microbial communities produce flavor substances through metabolic pathways. The use of Daqu with defined standard quality is one of the most effective potential methods for standardizing fermentation and ensuring the stable quality of Nongxiang Baijiu. Therefore, the study of microbial flora changes during the Daqu making process, combining this with metabonomics analysis could characterize microbial metabolic activity by different metabolites and provide a theoretical basis for the real-time monitoring of microbial growth and metabolism in the Daqu making process.
The primary aims of the present study were as follows: (1) using a culture-independent strategy during the producing process of fermentation to explore the structures of the bacterial and fungal communities; (2) to determine the content of volatile metabolites during fermentation; (3) to reveal potential correlations between microbial communities and metabolites. Then, to prove that the change of the relative abundance of the microorganisms can be reflected by the change of metabolite content. If we achieve these objectives it would further our understanding of the relationship between microorganisms and metabolites in Daqu and provide us with a theoretical basis for the quality monitoring of Daqu fermentation.
Materials and Methods
Sampling
We collected Daqu samples from a Nongxiang Baijiu enterprise in Suining, Sichuan, China. They were collected from five locations within the same incubating room and they were then mixed as one sample. The collection times were on days 0, 6, 16, and 29, respectively. The details of the sample are shown in Figures 1A-C. First, crushed barley and pea was mixed with water and then pressed to fall into a cuboid brick shape (Daqu bricks). Then, the bricks were placed into an incubating room, cultured for about 30 days according to the enterprise operation regulations. The culture temperature of Daqu needs to be controlled in line with the following procedure. (i) During the low-temperature period, microbiota start to grow and the temperature gradually increases, reaching 30–40°C in 2–3 days. (ii) In the high-temperature incubation stage, the temperature increases by 5–8°C/day to a maximum of 55–62°C. In this period, the relative humidity of the incubating room is increased to 90%, doors and windows are properly opened to ventilate and lower the humidity. (iii) For the temperature dropping stage, the temperature and relative humidity decrease gradually, and the aroma compounds are accumulated by the metabolic conversion. Finally, Daqu were stacked in another incubating room and deposited for more than three months until maturation. The samples were conveyed to the laboratory on ice in an insulation container. Samples were stored at −20°C before analysis of volatile compounds and were deposited at −80°C before DNA extraction. All analyses were conducted in biological quadruplicate.
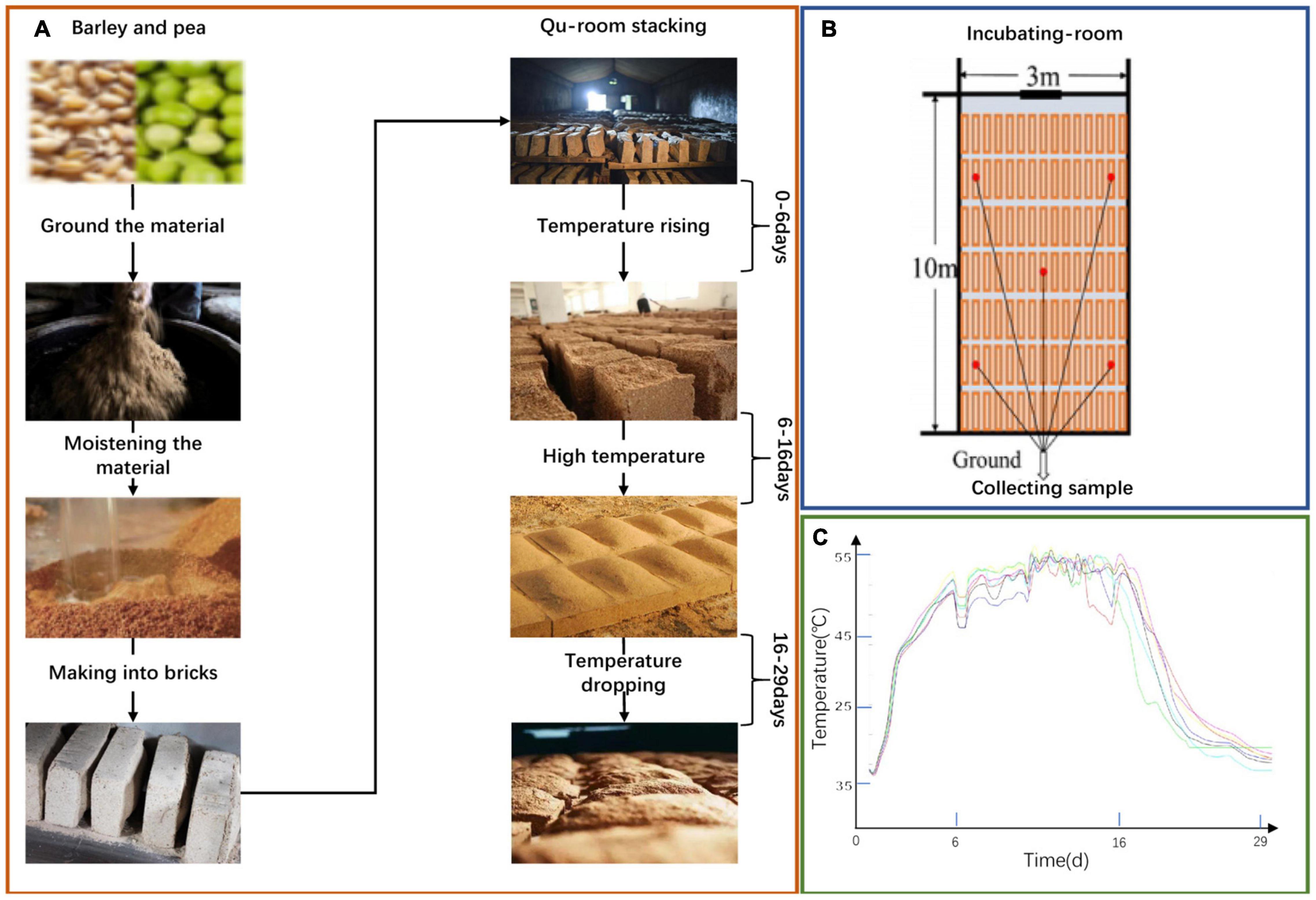
Figure 1. A schematic of the production process of NongXiang Daqu (A). A Schematic diagram of increasing room (B). Temperature curve of the fermentation process (C).
DNA Extraction and PCR Amplification Genomic
A 2 g portion of each of the crushed Daqu samples was ground into much tinier particles using a Medium-Flux Tissue Grinding System (Dinghaoyuan, China) combined with freezing in liquid nitrogen for homogenization. We used the Soil DNA Kit (Omega Bio-Tek, Norcross, GA, United States) to extract the DNA from the microbial communities of these samples. The DNA extract was tested for quality on 1% agarose gel, the concentration and purity of DNA were identified by using the Nano Drop 2000 UV–vis spectrophotometer (Thermo Scientific, DE, United States). The V3-V4 regions of the bacterial 16S rRNA gene and the internal transcribed spacer (ITS1) regions of fungal rRNA genes were amplified using the primers 338f/806r (5′-ACTCCTACGGGAGGCAGCAG-30 /50- GGACTACHVGGGTWTCTAAT-3′) and ITS1f/2043R (5′-CTTGGTCATTTAGAGGAAGTAA-3′/5′-GCTGCGTTCTTCATC GATGC-3′) with barcodes, respectively (Zhao et al., 2016). The polymerase chain reaction (PCR) amplification of the gene was performed and the initial denaturation was conducted at 95°C for 3 min, followed by 27 cycles of denaturation at 95°C for 30 s; annealing at 55°C for 30 s and extension at 72°C or 45 s; single extension at 72°C for 10 min; and ultimately at 4°C. The PCR mixtures contained the following: 4 μL of 5 × Trans Start Fastpfu buffer, 2 μL of 2.5 mM dNTPs, 0.8 μL of the forward primer (5 μM), 0.8 μL of the reverse primer (5 μM), 0.4 μL of Trans Start FastPfu DNA polymerase, 10 ng DNA of the template, and up to 20 μL of ddH2O.
Pyrosequencing and Bioinformatics Processing
Illumina MiSeq platform was used to carry out two terminal (Paired-end) sequencing of DNA fragments from the community. To integrate the original two terminal sequencing data, the quality of the two terminal sequences in fastq format was screened one by one by using the sliding window method. The size of the window was 10 bp, the step size was 1 BP, and the average quality of the base in the window was required to be ≥Q20 (that is, the average sequencing accuracy of the base was ≥99%). The length of the truncated sequence should be more than 150 BP, the existence of (mbiguous base) N is not allowed. Then, using Flash software (v1.2.71), pairing the two terminal sequences that passed the quality screening according to the overlapping base. The length of the overlapping base of read 1 and read 2 is required to be more than 10 bp, and the number of base mismatches is less than 10% of the length of the overlapping base. Finally, according to the index information of each sample (barcode sequence, which is a small base sequence used to identify the sample at the beginning of the sequence), the connected sequence recognition is assigned to the corresponding sample (index sequence is required to match completely), to obtain the effective sequence of each sample. Operational taxonomic units (OTUs) with 97% similarity cutoffs were clustered using UPARSE (version 7.1) (Edgar, 2013), chimeric sequences were identified and removed using the UCHIME algorithm. Taxonomic assignment was conducted using RDP Classifier (Wang et al., 2007). Alpha diversity indices Chao1, Shannon, and Goods coverage were performed in the software package mothur to reflect the diversity and richness of microbial communities in different samples (Schloss et al., 2011).
Quantitative Real-Time PCR
The qPCR reagent used was AceQ® qPCR SYBR® Green Master Mix of Applied Biosystems® by Vazyme (Carlsbad, CA, United States) and a final reaction volume of 20 μL was used. qPCR and melt curve analysis were performed on an Applied Biosystems®7500 Real-Time PCR System, Software v.2.0 (Foster City, CA, United States).1 ul of DNA was diluted several times and used as a template. The system includes an 8ul template and 8ul mixture of 2 × SYBR real-time PCR premixture and general primers what was mentioned in the previous PCR method. The qPCR protocol began with 1 cycle of 95°C for 5 mins, followed by 40 cycles of 95 C for 15 s, 60 C for 30 s was executed for 40 cycles. The melting curve stage (60–95°C) was added after the step of amplification.
Determination of Organic Acids in Daqu
Put a 1 g sample into a test tube, then add 10 ml ultra pure water. After ultrasonic treatment in a water bath for 30 min, centrifugation at 5000 rpm for 10 min, and filtering it through 0.22 μm PTFE membrane (allcrom, Sao Paulo, Brazil). A high-performance liquid chromatography system (LC- 20AT; Shimadzu, Kyoto, Japan) was used to analyze organic acids (malic acid, citric acid, oxalic acid, organic acid, succinic acid, acetic acid, acetic acid, and tartaric acid); Oven for controlling column temperature, UV relative detector; and a data acquisition system fitted with a CAPECELL PAK MG S5 C18 column (4.6 × 250 mm 2, 5 μm). The mobile phase consists of a 0.01M potassium dihydrogen phosphate buffer (ph2.55) and methanol (90:10, v/v) solution. The autosampler was adjusted to 20 μL. The isocratic elution method was applied to the mobile phase with a flow rate of 1.0 ml/min. The wavelength of 210 nm was selected for quantization. The calibration curves of different compounds were constructed by using pure standard curves of different concentrations (de Sena Aquino et al., 2015).
Determination of Volatile Metabolites
Gas chromatography-mass spectrometry (GC-MS) was used. Headspace solid phase microextraction (HS-SPME) combined with GC-MS (7890bgc, 5977ams) was used to analyze the volatile metabolites of fermented Daqu (Agilent Technologies, Santa Clara, United States). The sample (1 g) was accurately weighed into a 20 ml glass bottle containing 3ml saturated salt water, and then 50 μL 2 octanol (10 – 6 mol/L) was used as internal standard. The vials were immediately put into a heating block and equilibrated at 70c for 15 min, then DVB/car/pdmsspme fiber (2 cm, 50/30 μm) (Supelco, PA, United States) was exposed to the top of the samples for 40 min at 70°C. In the split free mode, the ejector removes the volatiles from the SPME fiber at 250°C for 5 min. Helium was used as carrier gas at a flow rate of 1.0 ml / min. Db-5ms column (30M × two hundred and fifty μm,0.25 μ M film thickness; Agilent, Santa Clara, United States) with column temperature set to 40°C (keep for 3 min) was increased to 150°C at 5°C /min, then 250°C at 10°C / min (keep at 10 min). MS was carried out at 230°C, M / Z 33-500 and 70 ev. The average concentration of each compound was calculated using the following formula: odorant = concentration (internal standard of the compound) × Peak area concentration) internal standard peak area.
Statistical Analysis
Data were analyzed using statistical products and services solutions (version 17.0; SPSS software in Chicago, IL, United States) and Duncan’s test. Differential metabolites were identified by using SIMCA-P software (version 11.5; Umetrics, Umeå, Sweden). To analyze the differences in volatile profiles among the samples; variables with a VIP value of 2 and a Student’s t test p value of 0.05 were considered differential. The quality of the OPLS-DA models was verified by the typical cross-validation procedure of leaving one seventh of the samples out of each round and then assessing the model. Data drawing also used the GraphPad prism (version 7; GraphPad Software, San Diego, CA, United States) and OriginPro (version 7.5; OriginLab Corporation, Northampton, United States) for plotting data. Correlation analysis was performed using R (version 3.2.2; The R Foundation for Statistical Computing, Vienna, Austria). The Phylogenetic Investigation of Communities by Reconstruction of Unobserved States (PICRUSt2) analysis was performed based on the 16S rRNA sequencing to gain additional insight into the metabolic potential of the differential fermentation period in Daqu. The gene family counts for each predicted sample were derived from the Kyoto Encyclopedia of Genes and Genome (KEGG) ortholog (KO)2. The estimated KEGG pathways related to the production of volatile metabolites were manually classified based on their KEGG identification (Yang et al., 2019). Statistically significant differences were accepted at p < 0.05. Spearman correlations coefficient (r) was calculated among the microbial genera and metabolites to detect the association between the microbiota and volatile compounds, r > 0.6 with statistical significance was considered as a strong correlation. Using Cytoscape 3.4.0 to visualize the correlation matrix.
Results
Quantitative Real-Time PCR Analysis
We operated qPCR for bacteria and fungi in the samples respectively and drew the melting curve (Supplementary Figure 1). During Daqu fermentation, the copy numbers of bacteria and fungi showed an obvious upward trend. The bacterial copy number ranged from 4262500 to 16401415, and the fungal copy number ranged from 21625000 to 225075000. The melting curve showed only one peak, which proved that the reaction was specific and there was no dimer.
Diversity and Composition of Microbial Community
The numbers of effective sequences of the fourteen samples were in the range of 37,082–100,172 and 27,082–91,208 for bacteria and fungi, respectively. The average number and ratio of high-quality sequences were 26818 and 85.97% for bacteria, and 60641 and 96.85% for fungi. The Specaccum curves based on OTU number (Supplementary Figure 2), approaching the saturation plateau, suggested that the sequencing depth in this research was adequate to represent the microbial structure of samples. We determined the diversity of bacterial and fungal communities with standardized sequences. The characteristics of microbial communities are listed in Table 1 (α Diversity index). According to the Shannon index, the bacterial diversity of Daqu in fermentation first decreased, then increased. Besides, the Simpson index showed an identical trend in the fermentation process.
The differences in microbial communities among the four Daqu samples became very obvious by using Circos (Ye et al., 2014) on the phylum level. Firmicutes, Proteobacteria, Cyanobacteria, Bacteroidetes and Actinobacteria were the main bacterial phyla (Figure 2A). Ascomycota, Mucoromycota and Basidiomycota were the predominant fungal phylum, comprising more than 98.68% of the total fungal population except for 0 day (Figure 2B). Meanwhile, the dissimilarities in genera in the four samples were apparent (Figures 2C,D). Bacillus, Thermoatinomyces, Pseudomonas, and Weissella were the main bacterial genera. However, the dominant bacteria genus were different in the four fermentation time samples. Initially, the relative abundance of Pseudomonas, Weissella and Staphylococcus was 37.86% in total. On the sixth day, the relative abundance of Weissella was 13.98%. On the 16 th day, the relative abundance of Bacillus was 67.34%, On the 29 th day, the relative abundance of Pseudomonas and Thermoatinomyces was 51.98% in total. In addition, Weissella, Leuconostoc, and Lactobacillus were the main Lactic acid bacteria in the fermentation process at four time points. The fungi community was dominated by Thermoascus, Thermomyces, Rhizopus, and Alternaria during the 29-day fermentation of Daqu. Thermoascus is the most dominant genus (more than 78.56%) except for 0 day. Nevertheless, the dominant genera were different in the four fermentation time samples. On the sixth day, the fungal community was dominated by Thermoascus (80.40%) and Rhizopus (8.79%). On the 16 th day, the main geuns were Thermoascus (87.53%) and Thermomyces (9.71%). Identically, On the 29 th day, the dominant genus were either Thermoascus (78.56%) and Thermomyces (19.01%). In addition, in unfermented Daqu samples, the most principal fungi in the microbial community were some unclassified fungi. This may be some unique microorganisms in the raw material.
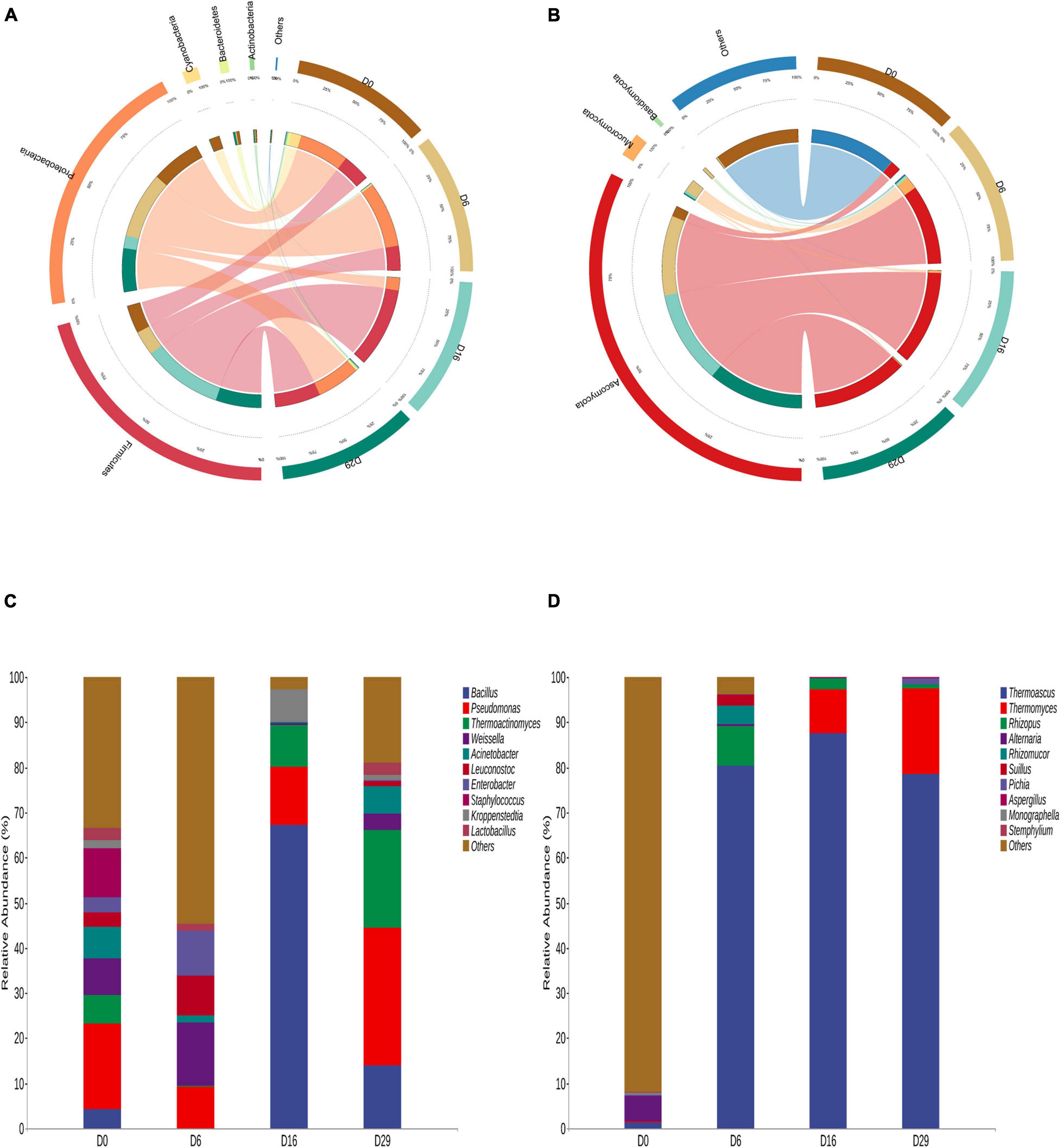
Figure 2. Distribution of bacteria (A) and the fungi (B) on phylum level during the fermentation process of Daqu. And showing the changes of major bacteria (C) and fungi (D) in the samples during the fermentation on genus level.
Comparison of Microbial Community Composition
The significance of the difference of bacterial and fungal communities in samples from different fermentation stages is shown as a principal component analysis (PCA) plot (Figures 3A,B). However, roughly, the differences between them are not obvious. This may be because Daqu fermentation is a complex system in which microorganisms interacted with each other. We used LEfSe analysis to calculate in addition to the main differential microorganisms in each group. In total, 17 bacterial genus and 17 fungi genus showed significant differences among samples (Figures 3C,D, LDA > 2, p < 0.05). In the beginning, the abundance of Aeromonas, pragia, Acinetobacter, and Mizugakiibacter was significantly higher than those at other time points. Finally, the abundance of Acinetobacter decreased remarkably, while Thermoactinomyces, Kroppenstedtia, and BreviBacillus were the dominant bacteria, which accounted for 67.62% of the total bacteria. In the early stage, Mycosphaerella, Alternaria, Kazachstania, Verticillium, and Fusarium were the main fungal genera, while the abundance of Mycosphaerella, Alternaria, and Kazachstania was significantly decreased. On the sixth day, Rhizomucor (81.32% of the total fungi) was the dominant fungal genus. In the later stage, Thermoascus (14.87%), Thermomyces, and Pichia (61.10%) were the main genera of the fungal community.
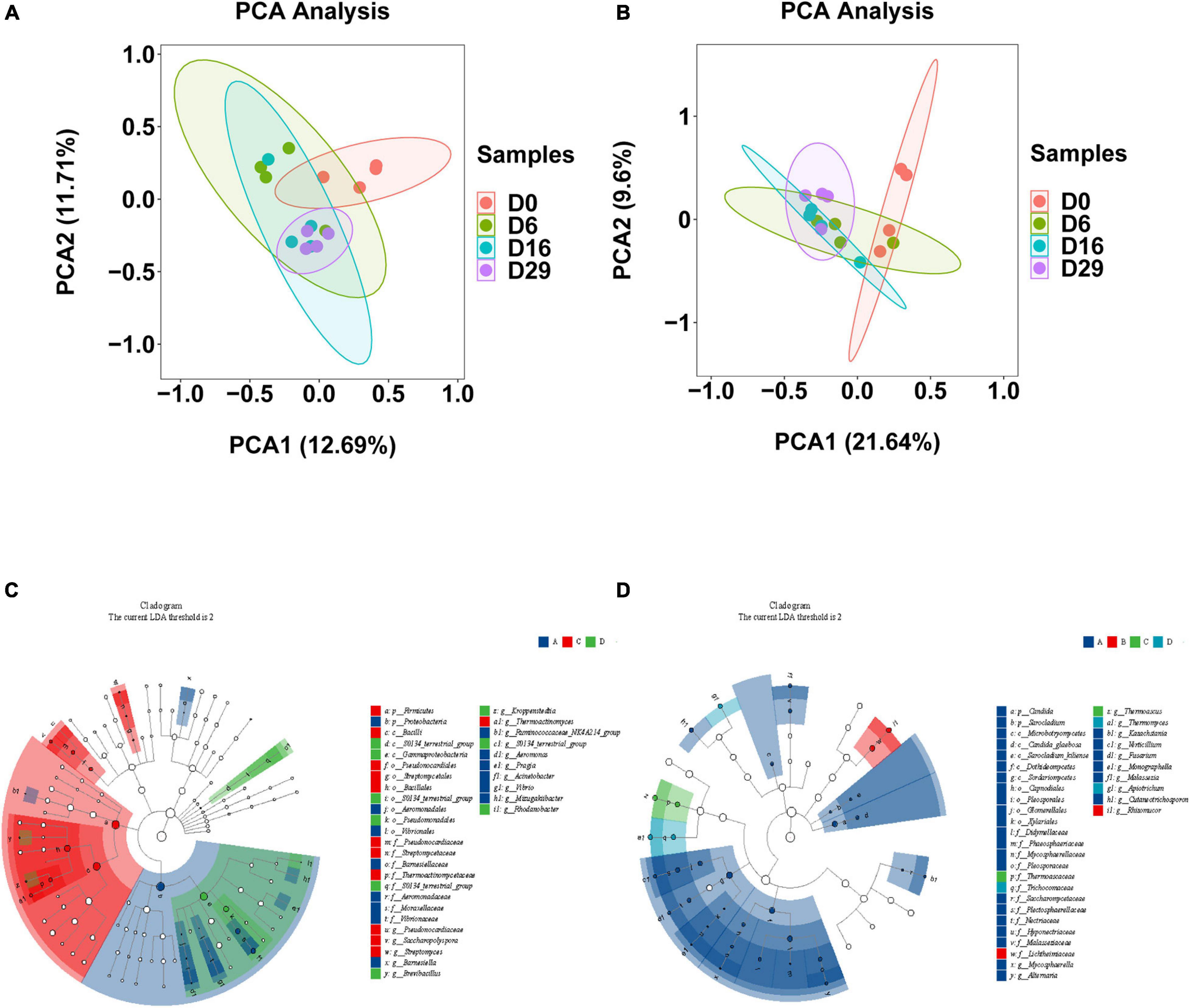
Figure 3. Clustering analysis of bacteria (A) and the fungi (B) in different fermented samples (days 0, 6, 16 and 29)using principal component analysis. The differential bacteria (C) and fungi (D) in each time point of Daqu by using LEfSe analysis (LDA > 2, p < 0.05) (Four groups were set according to four time points).
Prediction of Functional Genes in the Daqu Microbial Community
The analysis using the PICRUSt2 software package compared the functional characteristics of the microbial community in Daqu (He et al., 2020). The accuracy of the functional prediction using PICRUSt2 is reported so it could reach 85%–90% for microorganisms. The PICRUSt2 software package. Make metabolism as the level-1 pathway and 115 level-3 pathways are identified in the KEGG pathways. We screened the pathways of the top 30 abundances and made a heatmap (Figure 4). Generally, the relative rate of pentose and glucuronate interconversions was the highest. Especially at the beginning and end of fermentation. The relative abundances of starch and sucrose metabolism gradually increased during the fermentation as well as Glycolysis/Gluconeogenesis. The relative abundances of Glycolysis/Gluconeogenesis were low at the beginning of fermentation. The relative abundances of Glyoxylate and Dicarboxylate metabolism have a high relative abundance, which increased at the beginning of fermentation and reduced gradually, whereas, the relative abundances of the Citrate cycle (TCA cycle) and Lipid biosynthesis first increase and then decrease. It is noteworthy that not only the relative abundances of phenylalanine and Tyrosine biosynthesis gradually increase during the fermentation of Daqu; but also in the whole process, its abundance is at a high level.
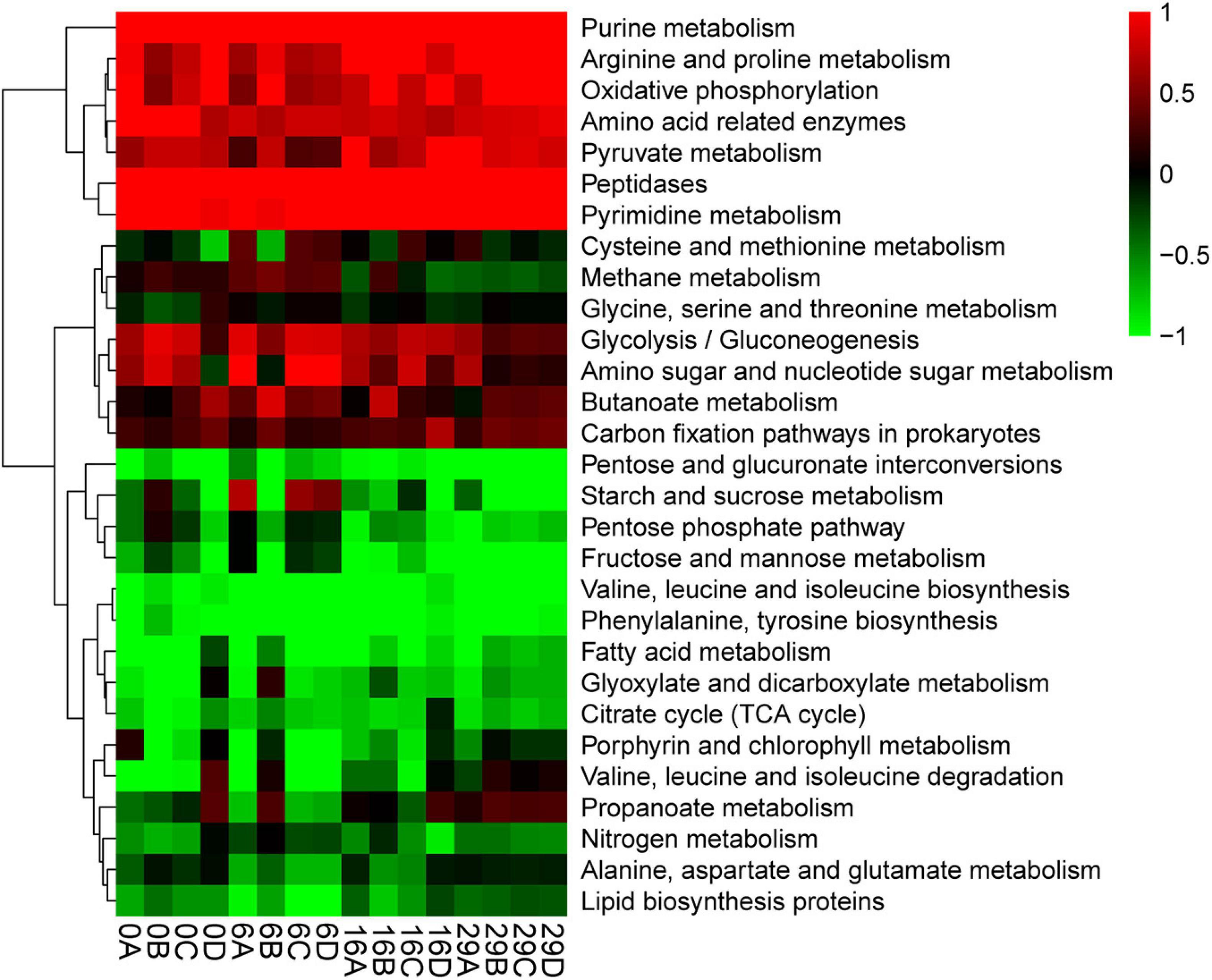
Figure 4. Potential function prediction (based on the level-3 pathway in metabolite of KEGG database).
Metabolomics Analysis of Fermented Daqu
In non targeted metabonomics analysis (based on GC-MS), 102 volatile compounds were found in the fermentation process. These compounds were divided into esters, alcohols, aldehydes, hydrocarbons, pyrazines, ketones, and other heterocyclic compounds (Figure 5A). Among them, 35 esters accounted for the highest proportion, followed by 22 aldehydes. During the whole fermentation process, the total volatile content of Daqu increased to 0.6882 mg/kg, which was about 5 times that of non fermented Daqu. Esters were the dominant volatile substances during the fermentation process of Daqu; their contents reached 0.0706 mg/kg on day 29 of the fermentation process. The ester content increased from 0.0129 mg/kg in the unfermented Sample to 0.0706 mg/kg on day 29 of the fermentation process. The scatter diagram (using OPLS-DA analysis) shows the samples with different fermentation times according to the concentration and type of each substance (Figure 5B). Differential metabolites (DMs) were are defined as the product with variable importance (VIP) value of ≥ 1 in the prediction and P value of ≤ 0.05 in the t-test. Figure 5C shows that there were 32 DMs among the samples and their scores of VIP, including 10 Esters, 6 Hydrocarbons, 10 Aldehydes, and 6 Alcohols. Among these, 16 Volatile metabolites were detected, which contributed to flavor characteristics. During the fermentation of Daqu, the concentrations of most of the esters and the alcohols significantly increased after fermentation, such as those of Amyl acetate (0 to 0.0113 mg/kg), Ethyl octanoate (0 to 0.0009 mg/kg), Ethyl pentadecanoate (0 to 0.0004 mg/kg), Ethyl palmitate (0 to 0.0224 mg/kg), Ethanol (0.0086 to 0.0737 mg/kg), N-amyl alcohol (0 to 0.0028 mg/kg), and phenylethanol (0 to 0.0047 mg/kg).
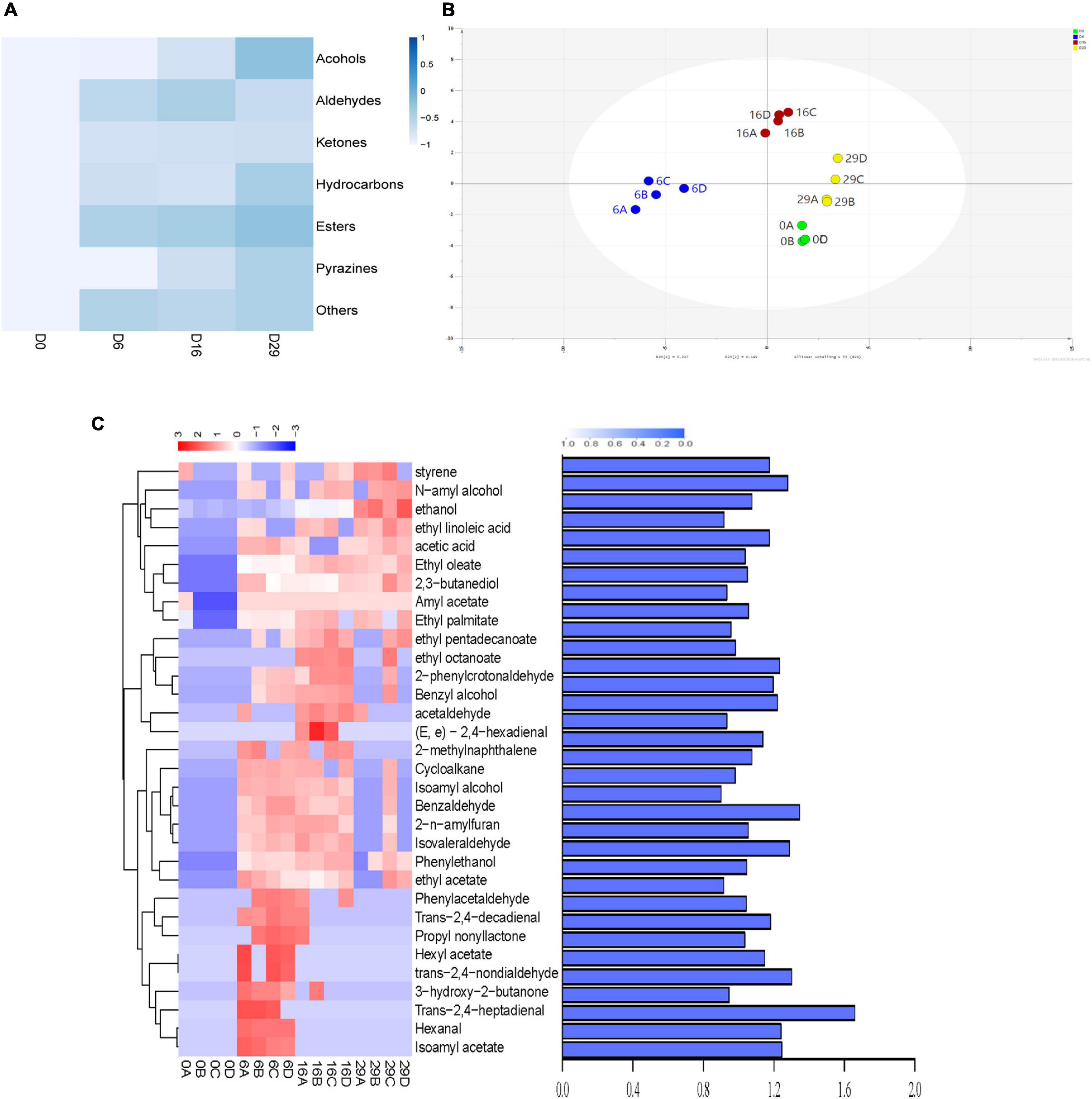
Figure 5. Kinetic comparison of volatile metabolites during fermentation. (A) Heatmap showing the Changes of different types of compounds in the fermentation process. (B) Clustering analysis of the volatile compounds in different fermented samples by orthogonal partial least squares analysis. (C) Distribution of different metabolites and value of variable importance in metabolite projection.
Changes in Organic Acids During Fermentation
During the fermentation process, the change of organic acids is an important physical and chemical index that can reflect the important activity of microorganisms indirectly. Table 2 shows that the total content of organic acids increased from 7.1667 mg/g to 9.5964 mg/g. In the fermentation of Daqu, Acetic acid was the most important organic acid, accounting for 84.24% of the total organic acids on the 29 th day of fermentation. After 29 days of fermentation, its content increased from 2.3629 mg/g to 8.0842 mg/g. However, with time, the content of lactic acid and fumaric acid increased first and then decreased. Their content both reached the highest point on the sixth day at 3.2688 mg/g, 0.1302 mg/g respectively. Then the content of lactic acid began to decrease significantly and reached 0.6319 mg/g at the end of fermentation. In addition, the content of citric acid was always at a low level, only 0.2963 mg/g at most (The sixth day of fermentation), and could not be detected after 16 days of fermentation.
The Correlation Between Differential Microbial Communities and DMs
The correlation between different microorganisms and DMS in the fermentation process was analyzed. In the key bacterial communities (Figure 6A), 17 microbes exhibited an association with DMs (| ρ| > 0.6). Only eight bacteria have a significant correlation with DMs (p < 0.005). As Figure 6C shows, the abundance of Streptomyces has a very significant positive correlation with Acetaldehyde, Ethyl octanoate, (E, e)-2,4-hexadienal, Ethyl oleate, Ethanol, Benzyl alcohol, and Phenylethanol. In addition, the abundance of Bacillus was positively correlated with only 2,3-butanediol. However, the abundance of Acinetobacter had a significantly negative correlation with Ethanol, Ethyl oleate, N-amyl alcohol, Ethyl palmitate, and Ethyl linoleic acid. It is noteworthy that the abundance of Saccharopolyspora, Streptomyces, and Thermoactinomyces have a simultaneously extremely significantly positive correlation with Ethyl octanoate, Phenylethanol, and Benzyl alcohol. Whereas, the abundance of Acinetobacter, Aeromonas, Luteibacter and Chryseobacterium positively correlated with ethanol and Ethyl oleate contents.
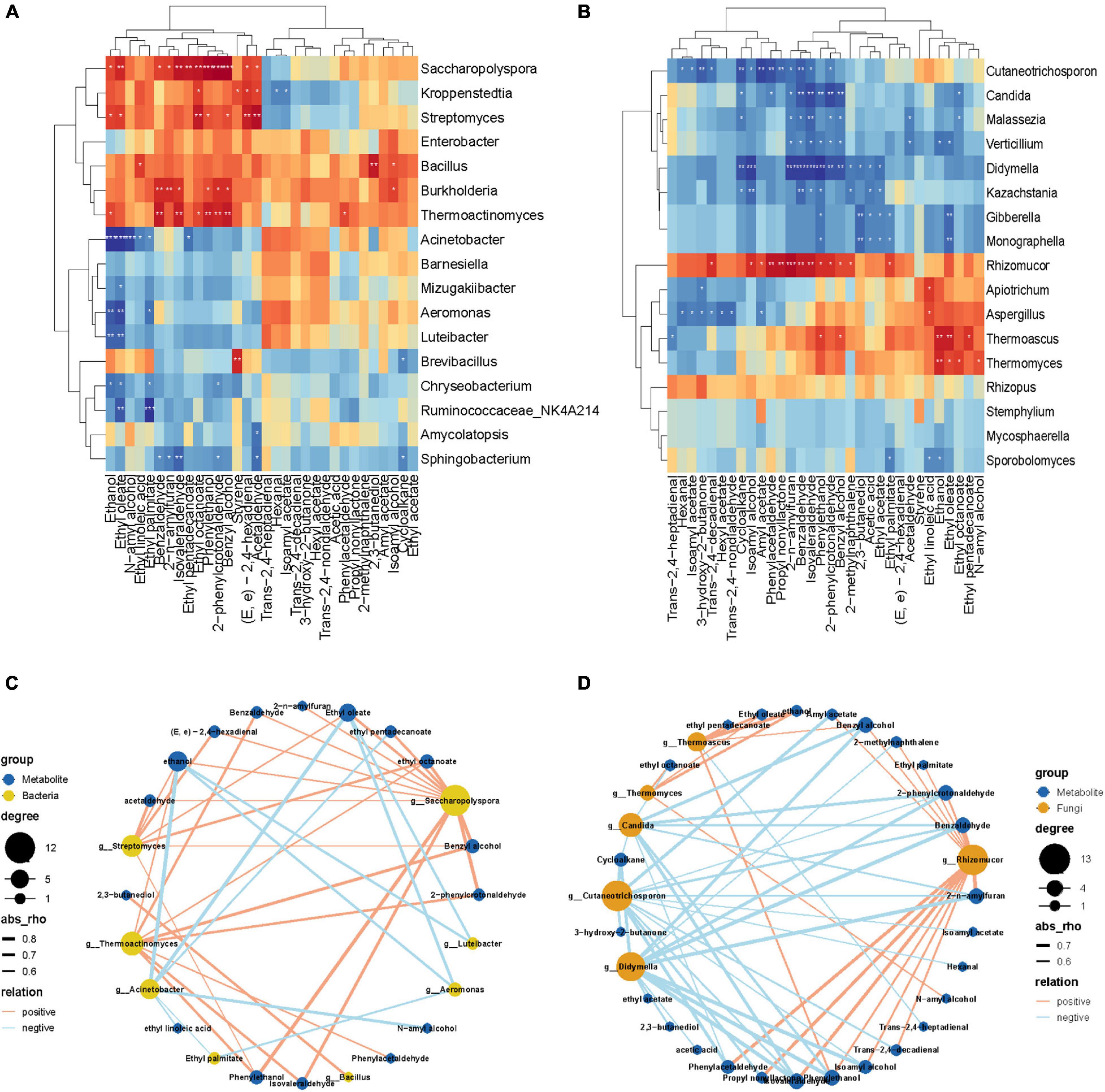
Figure 6. Heatmaps of Spearman correlations between dominant (A) bacterial and (B) fungal genera and differential metabolites during the fermentation process. Levels of significance are shown as follows: *0.01 < p ≤ 0.05; **0.001 < p ≤ 0.01***p ≤ 0.001. The co-occurrence network diagram of a highly significant correlation between differential metabolites and differential bacteria (C) and fungi (D).
In the key fungal communities (Figure 6B), 17 microbes exhibited a high association with DMs (| ρ| > 0.6)). In fungi genera, Rhizomucor was positively correlated with 12 DMs, including Trans-2,4-decadienal, Iso-amyl alcohol, Amyl acetate, Phenylacetaldehyde, Propyl nonyllactone, 2-n-amylfuran, Benzaldehyde, Iso-valeraldehyde, Phenylethanol 2-Phenylcrotonaldehyde, Benzyl alcohol, 2-methylnaphthalene, and Ethyl palmitate. However, there was a significant positive correlation between Cutaneotrichosporon and 13 DMs, containing Hexanal, Isoamyl acetate, 3-hydroxy-2-butanone, Trans-2,4-decadienal, Cycloalkane, Isoamyl alcohol, Amyl acetate, Phenylacetaldehyde, Propyl nonyllactone, 2-n-amylfuran, Benzaldehyde, Isovaleraldehyde and 2-phenylcrotonaldehyde. As we can see from the correlation network (Figure 6D) diagram, which shows that the abundance of Candida and Didymella have a simultaneous extremely significant negative correlation with the contents of 2-n-amylfuran, Benzaldehyde, Isovaleraldehyde, Phenylethanol, 2-phenylcrotonaldehyde, and Benzyl alcohol. In the meantime, the abundance of Thermoascus and Thermomyces showed a significantly positive correlation with ethanol and ethyl oleate contents.
Pathway Enrichment Analysis
The KEGG database was analyzed to enrich the pathways of DMs (Xu et al., 2021). To further explore the differential expression of these aforementioned metabolites in the fermentation process. A total of 21 DMs were identified and measured, and their contents determined. Metabolites set enrichment pathways analysis displayed that there are 19 pathways in all, there are six pathways of significant enrichment (Figure 7). They are Glycolysis/Gluconeogenesis, Phenylalanine metabolism, Toluene and xylene degradation, Styrene degradation, Pyruvate metabolism, Glycosaminoglycan biosynthesis–heparan sulfate, Phosphonate and Phosphinate metabolism, 3-Chloroacrylic acid degradation, Tetrachloroethene degradation (p < 0.05). Glycolysis/Gluconeogenesis and Phenylalanine metabolism are highly enriched pathways, with a p value of 0.0003 and 0.0009, respectively. At the same time, these two pathways also contain the largest number of DMs. Interestingly, these two pathways are also highly significant in the previous PICRUSt2 function prediction results of different microbial communities.
Discussion
This study aimed to investigate the relationship between metabolites and microbial community changes by using a combination of microbiomics and metabolomics, to provide a theoretical basis for the quality monitoring of Daqu fermentation (Zheng et al., 2011). We determined the metabolites of Daqu during the fermentation and then undertook a cluster analysis (OPLS-DA) that showed that there were significant differences on days 0, 6, 16, and 29. We found that this difference was similar to the succession of microbial communities during fermentation by sequencing the amplicon of the sample. The amplification and sequencing of 16S rRNA and ITS gene showed that the microbial community was mainly composed of three prokaryotic genera (Bacillus, Pseudomonas, and Enterobacter) and three eukaryotic genera (Aspergillus, Rhizopus, and Thermoascus), which are identical to many previous reports.
The key microorganisms in the samples of four fermentation time points were selected through LEfSe analysis. The early bacteria were mainly Aeromonas, pragia, Acinetobacter, and Mizugakiibacter. The late bacteria were mainly Thermoactinomyces, Saccharopolyspora, Streptomyces, and BreviBacillus. Whereas at the early stage, Mycosphaerella, Alternaria, Kazachstania, Verticillium, and Fusarium were the main genus of fungi. On the sixth day, Rhizomucor was the dominant fungal genus. We found that it showed significant positive correlations with five kinds of DMs (Isovaleraldehyde, Benzaldehyde, 2-n-amylfuran, Propyl nonyllactone, Phenylacetaldehyde), In previous studies, Rhizomuco was a popular genera in Daqu and are considered to be important functional micro organizations. As a powerful amylase producer, Rhizomucor is widely used in rice wine brewing and other traditional fermented foods by decomposing starch in Daqu raw materials, promoting the transformation of Pyruvic acid and Amino acid, providing nutrients for the growth of other microorganisms, and accumulating reducing sugar for later alcohol fermentation (Dung et al., 2007; Jiang et al., 2020). Additionally, the thermal fungus Rhizomucor has been used for producing industrial proteins and lipids (Yang et al., 2015; Wang et al., 2020). Rhizomucor can also secrete lipases and proteases, which play a key role in the process of synthesis of volatile substances, such as Esters, Aldehydes, Fatty acids, and so on. This explains that there are significant positive correlations between Rhizomucor and various DMs. Therefore, we can detect the concentration of changes of Isovaleraldehyde, Benzaldehyde, Phenylacetaldehyde, and other substances in the early stage to reflect the abundance changes of Rhizomucor in the early stage of fermentation in Nongxiang Daqu.
At the later stage, Thermoascus and Thermomyces were the differential genus of the fungal community. A higher abundance of Bacillus was observed in the 16 th to 29 th days of fermentation. First, this could be because the oxygen content is sufficient in the early stage of fermentation. The rapid growth of some aerobic microorganisms in Daqu had adverse effects on Bacillus. Second, Bacillus may compete with other bacteria for common ecological fields (Prosser et al., 2007). Third, Some bacteria may be inhibited by fungi, which reduce pH or produce ethanol. For example, S. cerevisiae can inhibit the growth of other strains by ethanol production (Meng et al., 2015). While for some yeasts, the growth of Pichia is inhibited by Bacillus (Deng et al., 2020). In the middle and later stages of this experiment, Bacillus dominated the microbial community. This is the same as the previous conclusion. Bacillus is a principal producer of Daqu flavor. It can secrete various hydrolases, including amylase, protease, and lipase to hydrolyze macromolecules, and produce flavor compounds in the brewing process (Zheng et al., 2011). More importantly, the production of hydrolytic enzymes and flavor precursors was improved after co-culture with Bacillus. The corresponding samples also exhibited higher contents of aromatic compounds, including, (2,2-diethoxyethyl)-benzene, Benzeneethanol, and Ethyl phenylacetate. Especially for Benzeneethanol and ethyl, they could provide rose-like and honey fragrances for liquor (Fan et al., 2007; Wang P. et al., 2017). We then found that there was only one significant positive correlation between Bacillus and 2,3-butanediol production, 2,3-Butanediol, a normal constituent of wine, is one of the rare aroma-producing polyols (Minebois et al., 2020). It might be synthesized from pyruvate by Bacillus (Wu et al., 2014). Furthermore, Bacillus, as a secretor of amylase, can continuously convert fermentable substances into Pyruvate. It may also be derived from diacetyl and acetoin in Leuconostoc (Escobar-Zepeda et al., 2016), but according to the microbial data of the Daqu fermentation process it can be observed that the metabolic activity of Leuconostoc is not evident. Therefore, 2,3-butanediol may be one of the biomarkers reflecting the growth of Bacillus.
In addition, the relative abundance of Thermoactinomyces increased significantly in the later stage of fermentation. These organisms are known to survive in harsh environments and metabolize at 45°C to produce various thermostable enzymes, such as amylase, serine, protease, and lipase (Pan et al., 2016). The existence of these bacteria can effectively promote the aerobic fermentation process of Daqu, which is consistent with the above results. During the aerobic fermentation of Daqu, the active metabolites of thermostable enzymes remained at a high level. Therefore, in the late stage of fermentation, we can determine that the relative abundance of Thermoactinomyces in Daqu samples could be determined by monitoring the contents of these metabolites. In addition, in the early stage of fermentation, Candida was selected as a differential microorganism. Candida is one of the producers of esters and alcohols (Han et al., 2009). It is the main microorganism responsible for lactic acid fermentation and alcohol fermentation (Sakandar et al., 2020). Ester production occurs in the downstream part of glycometabolism. Candida, Aspergillus, Rhizopus (Gandolfi et al., 2001) and Lactobacillales (Enterococcus, pediococcus, and LactoBacillus) participated in the production of esterase. In the amino acid branch, phenylethyl alcohol was produced from phenylalanine metabolism through the Ehrlich pathway of yeast. Candida and Monascus (Rahayu et al., 2017) participate in this process. In this study, we observed a significant positive correlation was observed between Aspergillus and ethyl linoleic acid. Previous studies have found that unsaturated fatty acids including linoleic acid, unsaturated fatty acid − rich fraction could be liberated by the action of Aspergillus oryzae lipases (Sakandar et al., 2020). It is noteworthy that Ethyl linoleate does not exist in Daqu, but appears in the middle and late stages of the fermentation process. Therefore, The increase of fatty acid ethyl ester content is obviously due to lipase catalyzed ester conversion as reported by Mitsuaki (1996), which indicated out that Fatty acid ethyl ester can be produced in fermented soybean paste when ethanol content reached 0.1% or more. In our case, the content of this ester was zero at the beginning, probably because yeast and Lactobacillus had no active metabolic activity, but its obvious formation result was produced a few days after. This shows that the enzyme reaction was effectively carried out in the fermentation process. This process is particularly crucial because fatty acid esters directly or indirectly promote the ripening flavor of any fermented food. It may thus be possible to follow up the fermentation process by monitoring the increase of ethyl linoleic acid content.
Previous research has suggested that the fermentation environment, microbial populations, and original raw materials notably influence the content of the organic acid. Acetic acid is the main organic acid at the end of Daqu fermentation, which is 8.0842 mg/g. On the one hand, this may be due to bacteria, on the other hand, it is the main end product in the high abundance pathways screened by PICRUSt2 function prediction and the pathway enrichment analysis of DMs. At the same time, as the intermediate product of some metabolic pathways, a part of Acetic acid will be metabolized by microorganisms in some communities (Nie et al., 2013), which could explain the phenomenon that the content of Acetic acid increases significantly and then decreases gradually during the fermentation process. In addition, most LactoBacillus species were capable of producing Lactic acid and Acetic acid (Rubing et al., 2020), The major citric acid accumulating microorganisms include several yeast strains from the genera Aspergillus and Candida, whereas the major malic acid producing microorganisms are from the genera Saccharomyces and Aspergillus (Ren et al., 2019). Staphylococcus and LactoBacillus could produce Lactic acid, and Saccharopolyspora might utilize Lactic acid, while Saccharomyces and Aspergillus were involved in both the production and utilization of Lactic acid (Liu et al., 2019). The interplay between these organisms was likely the reason for the fluctuation of the concentration of Lactic acid during the fermentation process, which may indicate that the determination of organic acid. content and its change can also reflect the growth and abundance of microbial flora related to organic acid production in the Daqu sample.
An integrated pathway map was used to show the metabolic pathways of some DMs in detail (Figure 8). Among the multiple pathways, Glycolysis/Gluconeogenesis pathway, Phenylalanine metabolism pathway, Pyruvate metabolic pathway, and TCA Cycle are directly involved in the production of Acetate, Acetaldehyde, Ethanol, Phenylacetaldehyde, and Phenylethyl alcohol (Jin et al., 2019; Liu et al., 2019). In the phenylalanine metabolism pathway, the levels of three proteins (Acetyl-CoA, Succinyl-CoA, and Phenyl-acetyl-CoA) and two metabolites (Phenylacetaldehyde, and Phenylethyl alcohol) were enriched. This result is consistent with the change of Phenylacetaldehyde and Phenylethyl alcohol contents in the previous volatile metabolite test during the fermentation of Daqu. Previous studies showed that the amylase and protease secreted by Bacillus changed starch and protein into glucose and amino acids, and promoted the formation of flavor compound precursors during the fermentation of Baijiu (Huang et al., 2020). They then also participated in the synthesis of a variety of volatile metabolites (Wang X. et al., 2017), However, many other microorganisms, such as some Lactic acid bacteria and thermophilic fungi also play this role (Jin et al., 2019; Yang et al., 2021). Therefore, we need to use more accurate omics sequencing and analysis technology to determine which microorganisms participate in specific metabolic pathways and which links are involved in different fermentation periods. It is a complex process, especially in Daqu fermentation. In such an open environment, the metabolic activities of various microorganisms form a very complex metabolic network. We still need to improve the understanding of flavor-producing core microorganisms.
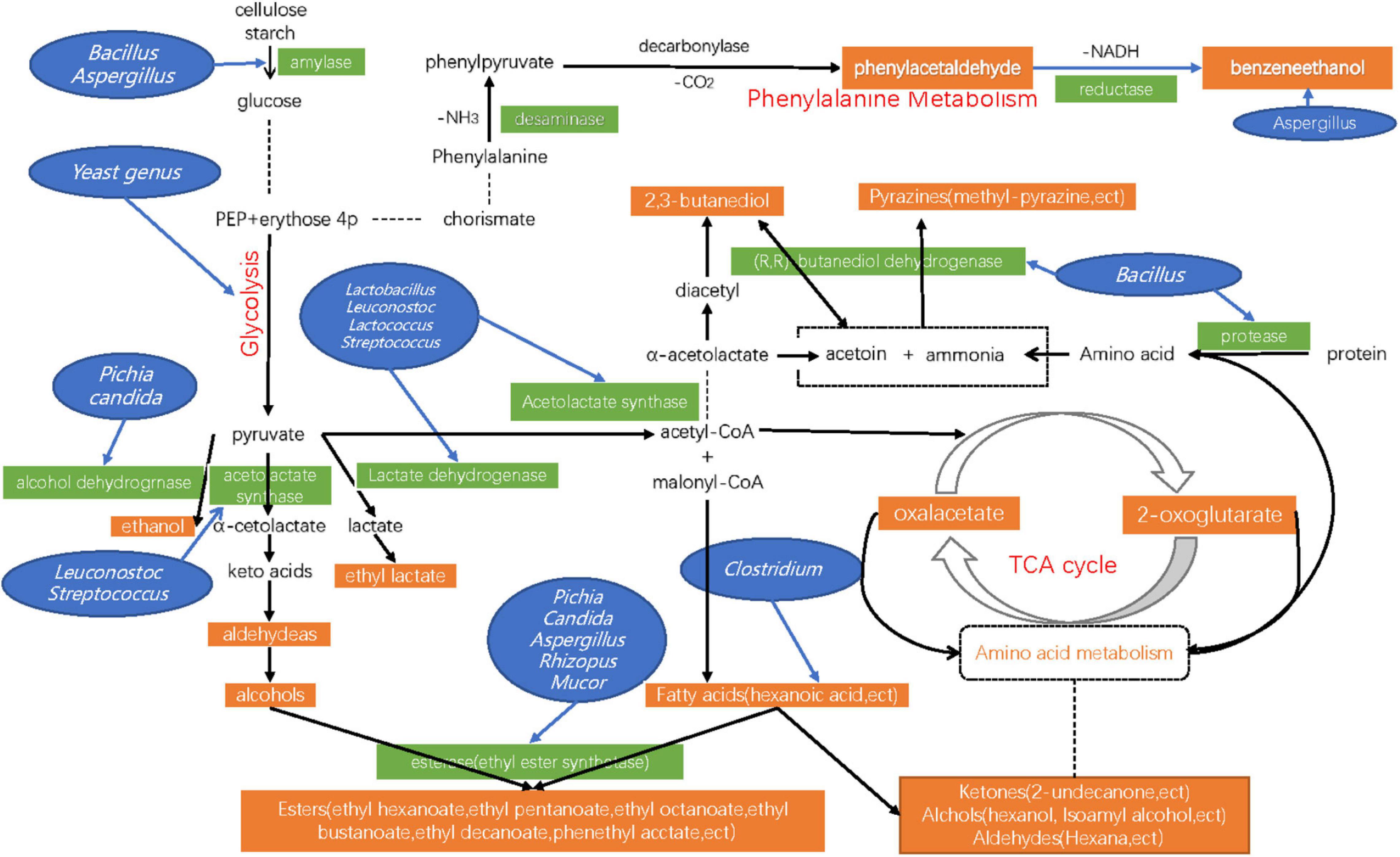
Figure 8. Schematic presentation of pathways, metabolites, enzymes, and genus related to the synthesis of Ethanol, Phenylethanol, Phenylacetaldehyde, and Lactic acids, ect in Daqu samples. The pathways were organized based on the KEGG (Kyoto Encyclopedia of Genes and Genomes).
Conclusion
In this study, we explored the correlation between the dominant microorganisms and different metabolites in different stages of the fermentation process, and then verified the relationship by using functional prediction and pathway enrichment. The result showed that the dynamic changes of microbial community were significantly correlated with the changes of some metabolites during the fermentation of Nongxiang Daqu. Thermoascus is the main fungus genus during the whole fermentation process. Its relative abundance was positively correlated with Ethanol and Ethyl oleate content. Meanwhile, in the middle and late stages of fermentation, the bacillus is the main bacteria. In addition, the change in the content of 2,3-butanediol was positively correlated with the change in the abundance of Bacillus, which can provide a new perspective for further exploring the relationship between microorganisms and metabolites in Daqu fermentation. Studying the changes of metabolites to reflect the changes of microorganisms in the fermentation process could provide a way to realize the rapid detection of Daqu quality strains and help control the microorganisms in the Daqu making process, to obtain more stable Baijiu products.
Data Availability Statement
The original contributions presented in the study are publicly available. This data can be found here: National Center for Biotechnology Information (NCBI) BioProject database under accession number PRJNA718914.
Author Contributions
HL, DH, and BH provided the idea, the framework, and support for the research. DY, YZ, HG, and WJ participated in the experiment. YD generated the data and performed the analysis of bioinformatics. YD wrote and edited the manuscript. All the authors contributed to the article and approved the submitted version.
Funding
This work was supported by the Sichuan Science and Technology Program (2019YJ0475) and the Key Laboratory of Wuliangye-flavor Liquor Solid-state Fermentation, China National Light Industry (2019JJ016). The authors declare no competing financial interest.
Conflict of Interest
DY, HG, YZ, and WJ were employed by company Sichuan Tuopai Shede Liquor Co., Ltd.
The remaining authors declare that the research was conducted in the absence of any commercial or financial relationships that could be construed as a potential conflict of interest.
Publisher’s Note
All claims expressed in this article are solely those of the authors and do not necessarily represent those of their affiliated organizations, or those of the publisher, the editors and the reviewers. Any product that may be evaluated in this article, or claim that may be made by its manufacturer, is not guaranteed or endorsed by the publisher.
Supplementary Material
The Supplementary Material for this article can be found online at: https://www.frontiersin.org/articles/10.3389/fmicb.2021.688981/full#supplementary-material
Footnotes
References
Chen, Y., Li, K., Liu, T., Li, R., Fu, G., Wan, Y., et al. (2020). Analysis of difference in microbial community and physicochemical indices between surface and central parts of chinese special-flavor baijiu daqu. Front. Microbiol. 11:592421. doi: 10.3389/fmicb.2020.592421
de Sena Aquino, A. C., Azevedo, M. S., Ribeiro, D. H., Costa, A. C., and Amante, E. R. (2015). Validation of HPLC and CE methods for determination of organic acids in sour cassava starch wastewater. Food Chem. 172, 725–730. doi: 10.1016/j.foodchem.2014.09.142
Deng, N., Du, H., and Xu, Y. (2020). Cooperative response of pichia kudriavzevii and saccharomyces cerevisiae to lactic acid stress in baijiu fermentation. J. Agric. Food Chem. 68, 4903–4911. doi: 10.1021/acs.jafc.9b08052
Du, H., Wang, X., Zhang, Y., and Xu, Y. (2019). Exploring the impacts of raw materials and environments on the microbiota in Chinese Daqu starter. Int. J. Food Microbiol. 297, 32–40. doi: 10.1016/j.ijfoodmicro.2019.02.020
Dung, N. T. P., Rombouts, F. M., and Nout, M. J. R. (2007). Characteristics of some traditional Vietnamese starch-based rice wine fermentation starters (men). LWT Food Sci. Technol. 40, 130–135. doi: 10.1016/j.lwt.2005.08.004
Edgar, R. C. (2013). UPARSE: highly accurate OTU sequences from microbial amplicon reads. Nat. Methods 10:996. doi: 10.1038/NMETH.2604
Escobar-Zepeda, A., Sanchez-Flores, A., and Baruch, M. Q. (2016). Metagenomic analysis of a Mexican ripened cheese reveals a unique complex microbiota. Food Microbiol. 57, 116–127. doi: 10.1016/j.fm.2016.02.004
Fan, G., Fu, Z., Sun, B., Zhang, Y., Wang, X., Xia, Y., et al. (2019). Roles of aging in the production of light-flavored Daqu. J. Biosci. Bioeng. 127, 309–317. doi: 10.1016/j.jbiosc.2018.08.005
Fan, W., Xu, Y., and Yanhong, Z. (2007). Characterization of pyrazines in some chineseliquors and their approximate concentrations. J. Agric. Food Chem. 55, 9956–9962. doi: 10.1021/jf071357q
Fan, Y., Huang, X., Chen, J., and Han, B. (2020). Formation of a mixed-species biofilm is a survival strategy for unculturable lactic acid bacteria and saccharomyces cerevisiae in daqu, a chinese traditional fermentation starter. Front. Microbiol. 11:138. doi: 10.3389/fmicb.2020.00138
Gandolfi, R., Converti, A., Pirozzi, D., and Molinari, F. (2001). Efficient and selective microbial esterification with dry mycelium of Rhizopus oryzae. J. Biotechnol. 92, 21–26. doi: 10.1016/s0168-1656(01)00345-5
Han, S. Y., Pan, Z. Y., Huang, D. F., Ueda, M., Wang, X. N., and Lin, Y. (2009). Highly efficient synthesis of ethyl hexanoate catalyzed by CALB-displaying Saccharomyces cerevisiae whole-cells in non-aqueous phase. J. Mol. Catalysis B 59, 168–172. doi: 10.1016/j.molcatb.2009.02.007
He, G., Huang, J., Wu, C., Jin, Y., and Zhou, R. (2020). Bioturbation effect of fortified Daqu on microbial community and flavor metabolite in Chinese strong-flavor liquor brewing microecosystem. Food Res. Int. 129:108851. doi: 10.1016/j.foodres.2019.108851
Huang, X., Fan, Y., Lu, T., Kang, J., and Chen, J. (2020). Composition and metabolic functions of the microbiome in fermented grain during light-flavor baijiu fermentation. Microorganisms 8:1281. doi: 10.3390/microorganisms8091281
Jiang, L., Su, W., Mu, Y., and Mu, Y. (2020). Major metabolites and microbial community of fermented black glutinous rice wine with different starters. Front. Microbiol. 11:593. doi: 10.3389/fmicb.2020.00593
Jin, Y., Li, D., Ai, M., Tang, Q., Huang, J., Ding, X., et al. (2019). Correlation between volatile profiles and microbial communities: a metabonomic approach to study Jiang-flavor liquor Daqu. Food Res. Int. 121, 422–432. doi: 10.1016/j.foodres.2019.03.021
Li, W., Fan, G., Fu, Z., Wang, W., Xu, Y., Teng, C., et al. (2020). Effects of fortification of Daqu with various yeasts on microbial community structure and flavor metabolism. Food Res. Int. 129:108837. doi: 10.1016/j.foodres.2019.108837
Liu, D., and Tong, C. (2017). Bacterial community diversity of traditional fermented vegetables in China. LWT 86, 40–48. doi: 10.1016/j.lwt.2017.07.040
Liu, H., and Sun, B. (2018). Effect of fermentation processing on the flavor of baijiu. J. Agric. Food Chem. 66, 5425–5432. doi: 10.1021/acs.jafc.8b00692
Liu, S., Chen, Q., Zou, H., Yu, Y., Zhou, Z., Mao, J., et al. (2019). A metagenomic analysis of the relationship between microorganisms and flavor development in Shaoxing mechanized huangjiu fermentation mashes. Int. J. Food Microbiol. 303, 9–18. doi: 10.1016/j.ijfoodmicro.2019.05.001
Meng, X., Wu, Q., Wang, L., Wang, D., Chen, L., and Xu, Y. (2015). Improving flavor metabolism of Saccharomyces cerevisiae by mixed culture with Bacillus licheniformis for Chinese Maotai-flavor liquor making. J. Ind. Microbiol. Biotechnol. 42, 1601–1608. doi: 10.1007/s10295-015-1647-0
Minebois, R., Pérez-Torrado, R., and Querol, A. (2020). A time course metabolism comparison among Saccharomyces cerevisiae, S. uvarum and S. kudriavzevii species in wine fermentation - ScienceDirect. Food Microbiol. 90:103484. doi: 10.1016/j.fm.2020.103484
Mitsuaki, K. (1996). Formation of ethyl fatty acid ester in rice-koji and barley-koji miso. J. Brew. Soc. Jpn. 91, 222–227. doi: 10.6013/jbrewsocjapan1988.91.222
Mousavian, Z., Kavousi, K., and Masoudi-Nejad, A. (2016). Information theory in systems biology. Part I: gene regulatory and metabolic networks. Semin. Cell Dev. Biol. 51, 3–13. doi: 10.1016/j.semcdb.2015.12.007
Nie, Z., Yu, Z., Min, W., Yue, H., and Niu, D. (2013). Exploring microbial succession and diversity during solid-state fermentation of Tianjin duliu mature vinegar. Bioresour. Technol. 148C, 325–333. doi: 10.1016/j.biortech.2013.08.152
Noda-Garcia, L., Liebermeister, W., and Tawfik, D. S. (2018). Metabolite-enzyme coevolution: from single enzymes to metabolic pathways and networks. Annu. Rev. Biochem. 87, 187–216. doi: 10.1146/annurev-biochem-062917-012023
Pan, L., Lin, W., Xiong, L., Wang, X., and Luo, L. (2016). Environmental factors affecting microbiota dynamics during traditional solid-state fermentation of chinese daqu starter. Front. Microbiol. 7:1237. doi: 10.3389/fmicb.2016.01237
Prosser, J., Bohannon, B., Curtis, T., Ellis, R., Firestone, M., Freckleton, R., et al. (2007). The role of ecological theory in microbiology. Nat. Rev. Microbiol. 5:384.
Rahayu, Y., Yoshizaki, Y., Yamaguchi, K., Okutsu, K., Futagami, T., Tamaki, H., et al. (2017). Key volatile compounds in red koji-shochu, a Monascus-fermented product, and their formation steps during fermentation. Food Chem. 224, 398–406. doi: 10.1016/j.foodchem.2016.12.005
Ren, Q., Sun, L., Wu, H., Wang, Y., Wang, Z., Zheng, F., et al. (2019). The changes of microbial community and flavor compound in the fermentation process of Chinese rice wine using Fagopyrum tataricum grain as feedstock. Sci. Rep. 9:3365.
Rubing, D., Qun, W., and Yan, X. (2020). Chinese liquor fermentation: identification of key flavor-producing lactobacillus spp. by quantitative profiling with indigenous internal standards. Appl. Environ. Microbiol. 86: 20–e00456.
Sakandar, H. A., Hussain, R., Khan, Q. F., and Zhang, H. (2020). Functional microbiota in chinese traditional baijiu and mijiu qu (starters): a review. Food Res. Int. 138:109830.
Schloss, P. D., Gevers, D., and Westcott, S. L. (2011). Reducing the effects of PCR amplification and sequencing artifacts on 16S rRNA-based studies. PLoS One 6:e27310. doi: 10.1371/journal.pone.0027310
Slot, J. C., and Gluck-Thaler, E. (2019). Metabolic gene clusters, fungal diversity, and the generation of accessory functions. Curr. Opin. Genet. Dev. 58-59, 17–24. doi: 10.1016/j.gde.2019.07.006
Wang, P., Wu, Q., Jiang, X., Wang, Z., Tang, J., and Xu, Y. (2017). Bacillus licheniformis affects the microbial community and metabolic profile in the spontaneous fermentation of Daqu starter for Chinese liquor making. Int. J. Food Microbiol. 250, 59–67. doi: 10.1016/j.ijfoodmicro.2017.03.010
Wang, Q., Garrity, G. M., Tiedje, J. M., and Cole, J. R. (2007). Naive Bayesian classifier for rapid assignment of rRNA sequences into the new bacterial taxonomy. Appl. Environ. Microbiol. 73, 5261–5267. doi: 10.1128/aem.00062-07
Wang, X., Du, H., and Xu, Y. (2017). Source tracking of prokaryotic communities in fermented grain of Chinese strong-flavor liquor. Int. J. Food Microbiol. 244, 27–35. doi: 10.1016/j.ijfoodmicro.2016.12.018
Wang, Y. C., Hu, H. F., Ma, J. W., Yan, Q. J., Liu, H. J., and Jiang, Z. Q. (2020). A novel high maltose-forming alpha-amylase from Rhizomucor miehei and its application in the food industry. Food Chem. 305:125447. doi: 10.1016/j.foodchem.2019.125447
Wang, Y., Cai, W., Wang, W., Shu, N., Zhang, Z., Hou, Q., et al. (2021). Analysis of microbial diversity and functional differences in different types of high-temperature Daqu. Food Sci. Nutr. 9, 1003–1016. doi: 10.1002/fsn3.2068
Wu, Q., Ling, J., and Xu, Y. (2014). Starter culture selection for making chinese sesame-flavored liquor based on microbial metabolic activity in mixed-culture fermentation. Appl. Environ. Microbiol. 80, 4450–4459.
Wu, X., Jing, R., Chen, W., Geng, X., Li, M., Yang, F., et al. (2020). High-throughput sequencing of the microbial diversity of roasted-sesame-like flavored Daqu with different characteristics. 3 Biotech 10:502.
Xiao, C., Lu, Z. M., Zhang, X. J., Wang, S. T., Ao, L., Shen, C. H., et al. (2017). Bio-heat is a key environmental driver shaping the microbial community of medium-temperature daqu. Appl. Environ. Microbiol. 83:e01550–17. doi: 10.1128/aem.01550-17
Xu, X., Wu, B., Zhao, W., Lao, F., Chen, F., Liao, X., et al. (2021). Shifts in autochthonous microbial diversity and volatile metabolites during the fermentation of chili Daqu (Capsicum frutescens L.). Food Chem. 335:127512. doi: 10.1016/j.foodchem.2020.127512
Yang, Q., Wang, S., Dai, E., Zhou, S., Liu, D., Liu, H., et al. (2019). Pathway enrichment analysis approach based on topological structure and updated annotation of pathway. Brief Bioinform. 20, 168–177. doi: 10.1093/bib/bbx091
Yang, S. Q., Xiong, H., Yang, H. Y., Yan, Q. J., and Jiang, Z. Q. (2015). High-level production of beta-1,3-1,4-glucanase by Rhizomucor miehei under solid-state fermentation and its potential application in the brewing industry. J. Appl. Microbiol. 118, 84–91. doi: 10.1111/jam.12694
Yang, Y., Wang, S. T., Lu, Z. M., Zhang, X. J., Chai, L. J., Shen, C. H., et al. (2021). Metagenomics unveils microbial roles involved in metabolic network of flavor development in medium-temperature daqu starter. Food Res. Int. 140:110037. doi: 10.1016/j.foodres.2020.110037
Ye, L., Amberg, J., Chapman, D., Gaikowski, M., and Liu, W. T. (2014). Fish gut microbiota analysis differentiates physiology and behavior of invasive Asian carp and indigenous American fish. ISME J. 8, 541–551. doi: 10.1038/ismej.2013.181
Zhao, L., Li, Y., Jiang, L., and Deng, F. (2016). Determination of fungal community diversity in fresh and traditional Chinese fermented Daqu by pyrosequencing. FEMS Microbiol. Lett. 363:fnw273. doi: 10.1093/femsle/fnw273
Zheng, X. W., Tabrizi, M. R., Nout, M., and Han, B. Z. (2011). Daqu— a traditional chinese liquor fermentation starter. J. Inst. Brew. 117, 82–90. doi: 10.1002/j.2050-0416.2011.tb00447.x
Keywords: nongxiang daqu, microbial communities, differential metabolites, process of fermentation, correlation analysis
Citation: Deng Y, Huang D, Han B, Ning X, Yu D, Guo H, Zou Y, Jing W and Luo H (2021) Correlation: Between Autochthonous Microbial Diversity and Volatile Metabolites During the Fermentation of Nongxiang Daqu. Front. Microbiol. 12:688981. doi: 10.3389/fmicb.2021.688981
Received: 31 March 2021; Accepted: 05 July 2021;
Published: 22 September 2021.
Edited by:
Madhumita Barooah, Assam Agricultural University, IndiaCopyright © 2021 Deng, Huang, Han, Ning, Yu, Guo, Zou, Jing and Luo. This is an open-access article distributed under the terms of the Creative Commons Attribution License (CC BY). The use, distribution or reproduction in other forums is permitted, provided the original author(s) and the copyright owner(s) are credited and that the original publication in this journal is cited, in accordance with accepted academic practice. No use, distribution or reproduction is permitted which does not comply with these terms.
*Correspondence: Dan Huang, 632348827@qq.com; Huibo Luo, susehbluo@163.com