- 1Department of Food Hygiene and Technology, Universidad de León, León, Spain
- 2Institute of Food Science and Technology, Universidad de León, León, Spain
Campylobacter spp. are the most frequent agent of human gastroenteritis worldwide, and the spread of multidrug-resistant strains makes the clinical treatment difficult. The current study presents the resistome analysis of 39,798 Campylobacter jejuni and 11,920 Campylobacter coli genomes available in public repositories. Determinants of resistance to β-lactams (Be) and tetracyclines (Te) were the most frequent for both species, with resistance to quinolones (Qu) as the third most important on C. jejuni and to aminoglycosides (Am) on C. coli. Moreover, resistance to Te, Qu, and Am was frequently found in co-occurrence with resistance to other antibiotic families. Geographical differences on clonal complexes distribution were found for C. jejuni and on resistome genotypes for both C. jejuni and C. coli species. Attending to the resistome patterns by isolation source, three main clusters of genomes were found on C. jejuni genomes at antimicrobial resistance gene level. The first cluster was formed by genomes from human, food production animals (e.g., sheep, cow, and chicken), and food (e.g., dairy products) isolates. The higher incidence of tet(O), associated with tetracycline resistance, and the gyrA (T86I) single-nucleotide polymorphism (SNP), associated with quinolone resistance, among genomes from this cluster could be due to the intense use of these antibiotics in veterinary and human clinical settings. Similarly, a high incidence of tet(O) genes of C. coli genomes from pig, cow, and turkey was found. Moreover, the cluster based on resistome patterns formed by C. jejuni and C. coli genomes of human, turkey, and chicken origin is in agreement with previous observations reporting chicken or poultry-related environments as the main source of human campylobacteriosis infections. Most clonal complexes (CCs) associated with chicken host specialization (e.g., ST-354, ST-573, ST-464, and ST-446) were the CCs with the highest prevalence of determinants of resistance to Be, Qu, and Te. Finally, a clear trend toward an increase in the occurrence of Te and Qu resistance determinants on C. jejuni, linked to the spread of the co-occurrence of the blaOXA–61 and tet(O)-tet(O/W/O) genes and the gyrA (T86I) SNP, was found from 2001 to date in Europe.
Introduction
Thermophilic Campylobacter species, and specially Campylobacter jejuni and Campylobacter coli, are the most frequent agents of human gastroenteritis both in industrialized countries (Kaakoush et al., 2015; European Food Safety Authority, 2018) and in low- and middle-income countries (Fischer Walker et al., 2013; Liu et al., 2015), with C. jejuni accounting for up to 80% of all human Campylobacter gastroenteritis cases (Bronnec et al., 2016), with estimations of over 800,000 cases per year between 2000 and 2008 (Scallan et al., 2011). Moreover, C. jejuni is the most frequent foodborne bacterial pathogen globally, with developing countries being the most affected (Yahara et al., 2017).
Campylobacter jejuni and C. coli are present in the digestive tract of different animals, including birds, cattle, sheep, pigs, and pets (Thépault et al., 2017), although chicken is recognized as the main source of human infection in most countries (Domingues et al., 2012; Newell et al., 2017; Cody et al., 2019). Despite consumption of contaminated raw or under-cooked poultry meat being the main source of human infection (Domingues et al., 2012), other sources of infection include contaminated water, unpasteurized/incompletely pasteurized milk, or the direct contact with animals and the environment (Lévesque et al., 2008; de Perio et al., 2013; Levallois et al., 2014; Fernandes et al., 2015; Mossong et al., 2016).
Antimicrobial-based therapy for campylobacteriosis is indicated only in patients with severe and invasive gastroenteritis. Macrolides (mainly erythromycin), fluoroquinolones (mainly ciprofloxacin), and tetracyclines are the most commonly used and effective antibiotics (Aarestrup et al., 2008; Silva et al., 2011). Unfortunately, these antimicrobial agents have analogs widely employed in veterinary settings, and their overuse or misuse have favored the emergence and spread of resistant Campylobacter isolates (Van Boeckel et al., 2015; Asuming-Bediako et al., 2019). Furthermore, the continuous increase in resistance to certain antimicrobial classes has been accompanied by a rise in the occurrence of multidrug-resistant (MDR) C. jejuni and C. coli from farm to fork (Mourkas et al., 2019).
Whole genome sequencing (WGS) is a powerful tool that offers high-resolution sub-typing of Campylobacter isolates, which is of value for outbreak investigations (Clark et al., 2016; Lahti et al., 2017). Apart from multilocus sequence typing (MLST) schemes, based on the analysis of the sequence of several house-keeping genes, WGS also allows to predict relevant phenotypes, such as antibiotic resistance, in Campylobacter (Zhao et al., 2016). Moreover, recent studies have found high concordance between the presence of antimicrobial resistance determinants in WGS data and phenotypic resistance among Campylobacter isolates from Latvia (Meistere et al., 2019), England and Wales (Painset et al., 2020), and Poland (Wysok et al., 2020), supporting the use of WGS data as a good predictor for phenotypical antimicrobial resistance.
Taking into account the increasing relevance of C. jejuni and C. coli as foodborne pathogens and the availability of a high amount of their genomes in different public repositories, with the corresponding information on the year, country, and source of isolation of the sequenced strains, the objectives of the current study are as follows: (i) to study the host association of the most abundant clonal complexes (CCs) of C. jejuni and the most abundant sequence types (ST) within the main CCs on C. jejuni and C. coli; (ii) to compare the prevalence of antimicrobial resistance (AR) factors within C. jejuni and C. coli genomes; (iii) to perform an exhaustive resistome genotypic analysis and link AR patterns to country/continent, source of isolation, and C. jejuni CCs; and (iv) to assess temporal changes in such AR patterns along the last 20 years.
Materials and Methods
Downloading Genomes and Metadata for Each Genome
Metadata associated to each genome was obtained by downloading the table obtained after using “Campylobacter jejuni” and “Campylobacter coli” as keywords (i) on PATRIC Taxon View website1; (ii) through the Export dataset section on the PubMLST-Campylobacter jejuni/coli website2, where all isolates were selected; and (iii) by using 02.NCBI_Download_metadata.R script3 for the NCBI repository. This script basically downloads the information obtained from https://www.ncbi.nlm.nih.gov/biosample/BIOSAMPLE?report=full&format=text, where BIOSAMPLE is substituted by the NCBI Biosample code for each genome analyzed, with these genomes being those of the C. jejuni and C. coli strains compiled in the prokaryotes.txt file in ftp://ftp.ncbi.nlm.nih.gov/genomes/GENOME_REPORTS/. The three metadata files obtained (for the NCBI, PATRIC, and PubMLST repositories) were manually inspected and adapted to merge them in a unique file. The collection_date, host_name, country, and continent columns were manually inspected to homogenize the collection date (to year of collection), isolation source, and location format (to country and continent).
C. jejuni and C. coli genomes publicly available at NCBI,4 PATRIC,5 and PubMLST6 repositories were downloaded on February 2021 using the download_genomes pipeline made for such task (see footnote 3). Briefly, the lists of available C. jejuni and C. coli genomes were constructed from metadata files for the PATRIC and PubMLST repositories and from the prokaryotes.txt file7 for the NCBI repository. Those genomes that were present on more than one repository were discarded from the NCBI or PubMLST dataset using 03.ena2ncbi.rb and 04.remove_repetition scripts (see footnote 3), and then, all selected genomes were downloaded using the corresponding script for each database from download_genomes github repository (see footnote 3).
Genome Analyses
All genomes were analyzed through MLST by using the mlst github pipeline (Seemann), which employs data from the PubMLST.org website (Jolley et al., 2018). Antimicrobial gene detection and detection of single-nucleotide polymorphisms (SNPs) conferring antimicrobial resistance were performed using the ResFinder database version updated at March 12, 2021 (Zankari et al., 2012) and PointFinder database updated at February 1, 2021 (Zankari et al., 2017), respectively. Both analyses, together with MLST, were performed for each genome by using the staramr pipeline (phac-nml/staramr) and 08.staramr_auto.rb script (see footnote 3) to automatize the analyses for the dozens of thousands genomes analyzed.
Statistical Analysis
The R-packages tidyr and dplyr were used to organize the data matrix, while the R-packages ggplot2 and pheatmap were employed for the representation of line plots, boxplots, and heatmap plots using R version 3.6.1 (R Core Team, 2019) and t-test to determine statistical differences. Heatmap plots were done using the percentage of genomes carrying each AR determinant (gene or SNP) that belonged to the same isolation source, clonal complex, or isolation year. The Euclidean distance and the complete (= UPGMA) clustering method were employed for the clustering of columns and rows.
Only those isolation sources with more than 50 genomes, excluding the unknown group, were selected for obtaining heatmap plots of antibiotic resistance genes (ARGs) or antibiotic families versus isolation source to avoid the introduction of biases due to the low number of genomes. Similarly, European genomes were uniquely selected for temporal variation analyses in the resistome, due to the fact that the highest number of genomes belonged to Europe and North America, but an important proportion of North-American genomes had missing information in relation to the year of isolation. Moreover, only European genomes from isolates obtained in those years when at least 100 genomes were available (i.e., years between 1997 and 2018, with the exception of 1999, 2000, and 2002) were selected for this task.
Results
Global Overview
A total of 39,798 C. jejuni and 11,920 C. coli genomes were analyzed. The C. jejuni genomes belonged to 41 CCs, with ST-21 CC being the most abundant (23.8% of the analyzed genomes) followed by ST-353 and ST-45 CCs (8.9 and 7.8% of the analyzed genomes, respectively), while C. coli genomes belonged to 21 CCs, with ST-828 CC as the main CC (84.8% of analyzed genomes). Remarkably, 13.7% of the C. jejuni and 13.43% of C. coli genomes were not assigned to any known CC. The main isolation source was humans (37.1% of C. jejuni genomes and 14.8% of C. coli genomes), followed by chicken, cows, and sheep for C. jejuni (10, 3, and 1.2% of genomes, respectively) and chicken, pigs, cows, and sheep for C. coli (9, 5.5, 2.3, and 1.3% of genomes, respectively). Unfortunately, 45.6 and 60.6% of C. jejuni and C. coli genomes, respectively, had missing information on the isolation source. A total of 18,812 C. jejuni (47.3%) and 7,959 C. coli (66.8%) genomes were from North-American isolates, while 18,787 C. jejuni (47.2%) and 3,328 C. coli (27.9%) genomes belonged to European isolates. The genome codes, collection year, continent, country, host and MLST information for all the analyzed C. jejuni and C. coli genomes were presented to Supplementary Tables 1, 2, respectively.
The most abundant AR factors were associated with resistance to β-lactams and tetracyclines for both species, although β-lactam resistance was most prevalent on C. jejuni (81.6 vs. 65.0%). AR factors against aminoglycosides and antibiotics of the macrolides-lincosamides-streptogramins-pleuromutilins group (MLSP) were most abundant on C. coli genomes (34.8 and 2.9%, respectively) than on C. jejuni (6.7 and 0.5%, respectively), while resistance to quinolones was much more frequent on C. jejuni (28.7%) then on C. coli (0.6%) genomes (Figure 1). Moreover, up to 7.8 and 23.3% of C. jejuni and C. coli genomes, respectively, did not harbor any known AR factor (Figure 1). Remarkably, 39.9 and 21% of C. jejuni and C. coli genomes, respectively, had only AR genes or SNPs associated with resistance to only β-lactams, while 41.7 and 44.0% of the genomes harbored determinants of resistance to β-lactams combined with determinants of resistance to other antibiotic families. Similar observations were made for resistance to tetracyclines, which were seen as unique resistance factors on 4.6 and 5.1% of C. jejuni and C. coli genomes, respectively, while 38.6 and 41.6% of genomes harbored resistance to tetracyclines combined with resistance to other antibiotic families (Figure 1). It is worth highlighting the high level of co-occurrence of resistance to aminoglycosides, β-lactams, and tetracyclines on C. coli genomes (20.2%) and to β-lactams, quinolones, and tetracyclines on C. jejuni genomes (15.4%) (Figure 1).
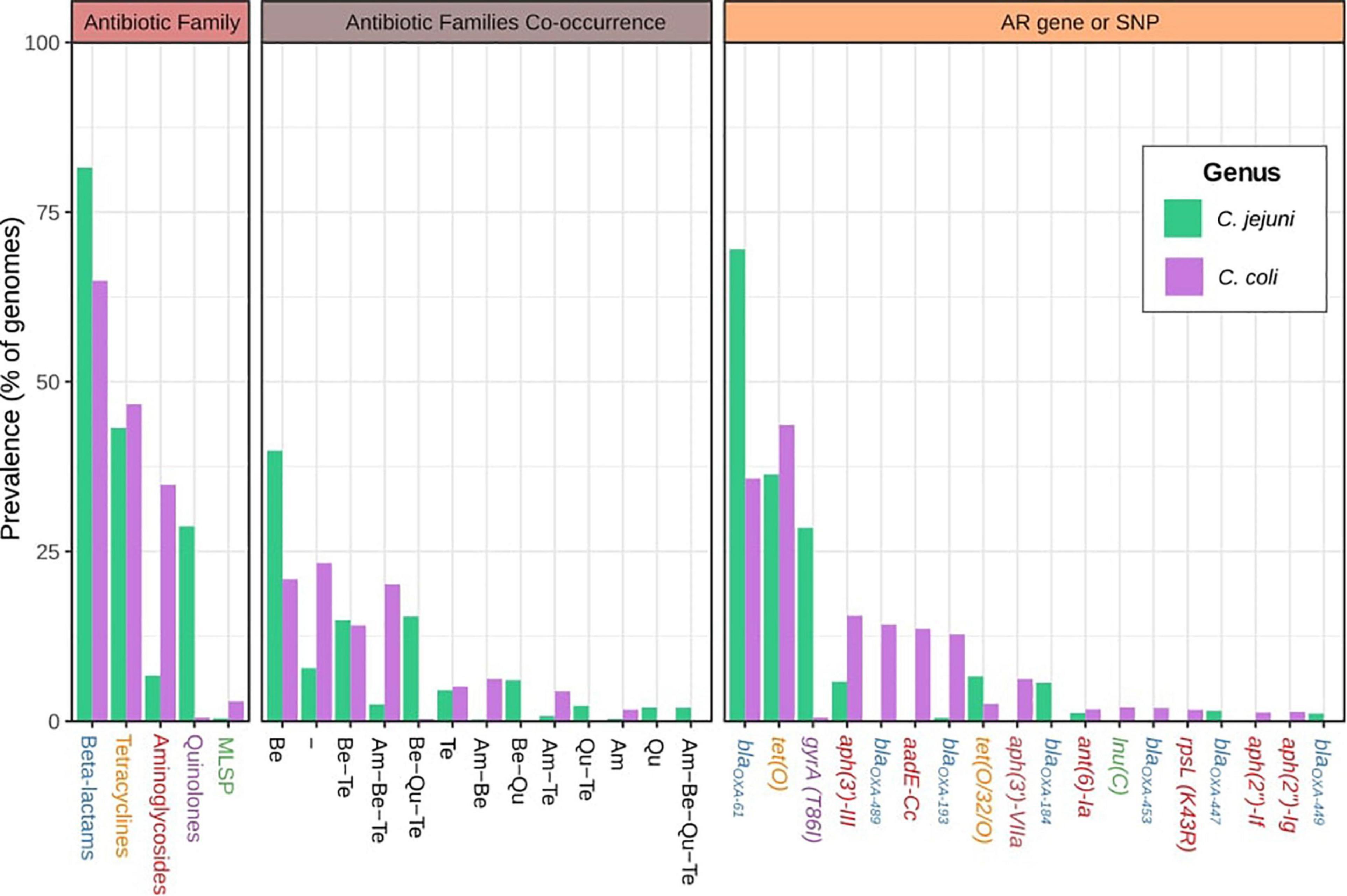
Figure 1. Global antibiotic resistance gene (ARG)-single-nucleotide polymorphism (SNP) distribution on C. jejuni and C. coli genomes. Antimicrobial resistance families, family co-occurrence, and most abundant ARGs or SNPs among the C. jejuni and C. coli genomes analyzed. Am, aminoglycosides; Be, beta-lactams; Qu, quinolones; Te, tetracyclines. Gene names are colored according to the corresponding antimicrobial family [blue: beta-lactams, orange: tetracyclines, red: aminoglycosides, purple: quinolones, and green: macrolides-lincosamides-streptogramins-pleuromutilins group (MLSP)]. Hyphen (-) indicates no ARGs or SNPs detected.
A total of 120 and 68 different AR genes and SNPs were detected for C. jejuni and C. coli genomes, respectively. blaOXA–61 and tet(O) genes were the most extended AR determinants on both C. jejuni and C. coli genomes (they were carried by 69.5 and 36.4% of C. jejuni and 35.8 and 43.6% of C. coli genomes, respectively). The gyrA (T86I) SNP was the third most prevalent AR factor on C. jejuni genomes (28.5%), while aph(3′)-III, blaOXA–489, aadE-Cc, and blaOXA–193 were present in more than 10% of the C. coli genomes (Figure 1).
Moreover, while 33.48% of C. jejuni genomes carried only the blaOXA–61 gene, 10.7% simultaneously harbored blaOXA–61 and tet(O), and 9.8% had gyrA (T86I), blaOXA–61, and tet(O) in combination, with these three ARG genotype patterns being the most abundant on C. jejuni genomes (Table 1). The presence of only the blaOXA–61 gene was observed on 10.4% of the C. coli genomes, with it being the main genotype pattern found, followed by only the blaOXA–489 gene and blaOXA–61 together with tet(O), with 6.9% and 6.6% of prevalence, respectively (Table 1).
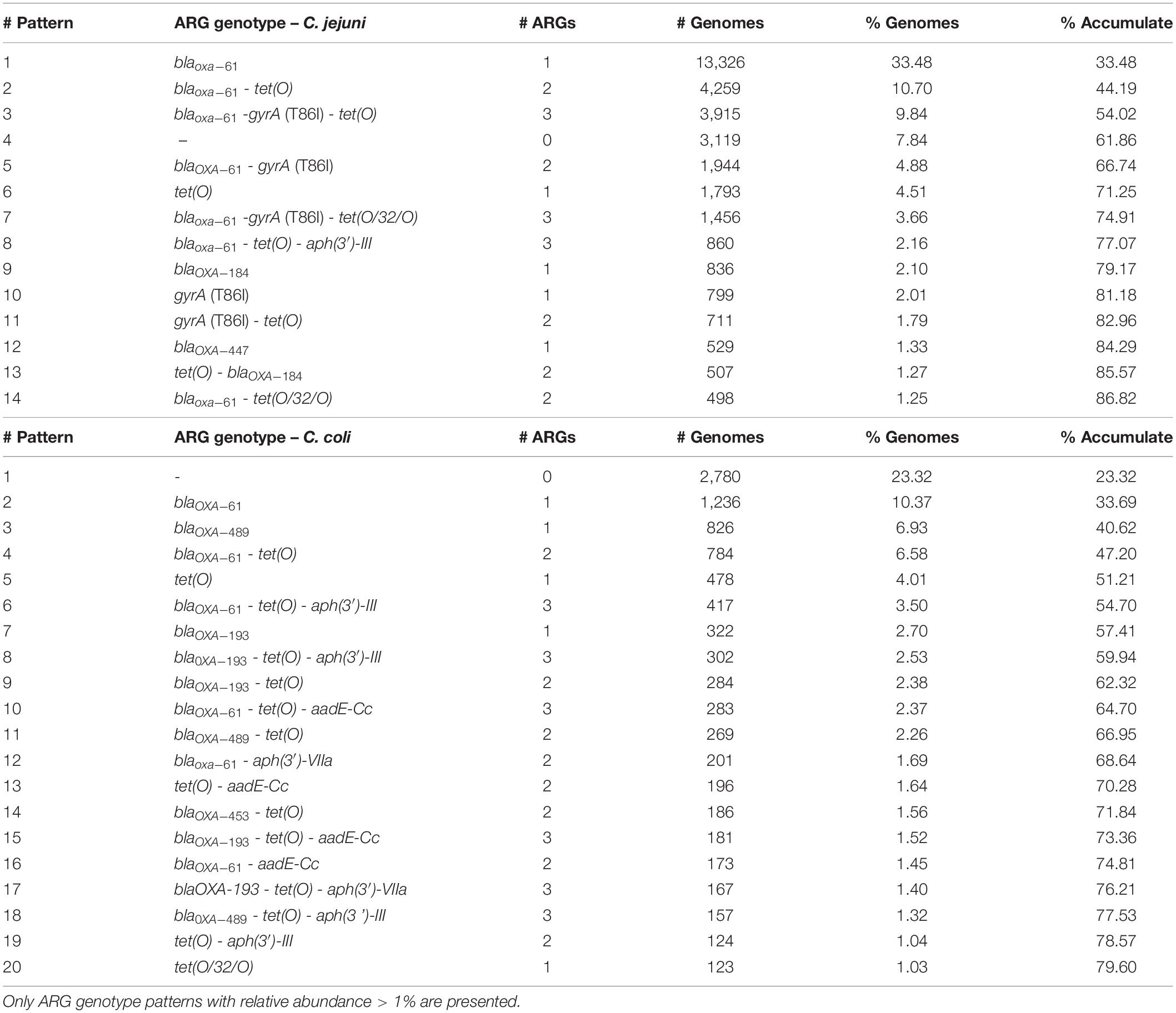
Table 1. Most abundant ARG genotypes on the C. jejuni and C. coli genomes analyzed, ordered by decreasing relative abundance.
Geographical Distribution of CCs and ARGs-SNPs
The first global analysis of C. jejuni CC distribution showed differences by continent. The ST-21 CC was the most abundant in Asia, Europe, and North America (26.9, 25.0, and 23.0% of genomes, respectively), while ST-353 CC was predominant in South America and Africa (23.7 and 15.6%, respectively) and ST-354 CC was dominant in Oceania (60.3%) (Figure 1). These patterns are not observed in all the countries from the same continent. For example, ST-353 CC was abundant in the United States and Brazil (12.8 and 33.5% of genomes, respectively), but it was less represented on Canadian and Peruvian genomes (2.2 and 7.0%, respectively), with Canada having a high prevalence of ST-45 CC (18.8%) (Figure 2). The main differences among countries within the same continent were found in Europe, with 93.3% of ST-21 CC genomes in Denmark; 34.4% of ST-45CC genomes in Finland; and 26.7, 25, and 39.5% of ST-21 CC genomes in France, United Kingdom, and Israel, as the main CCs (Figure 2).
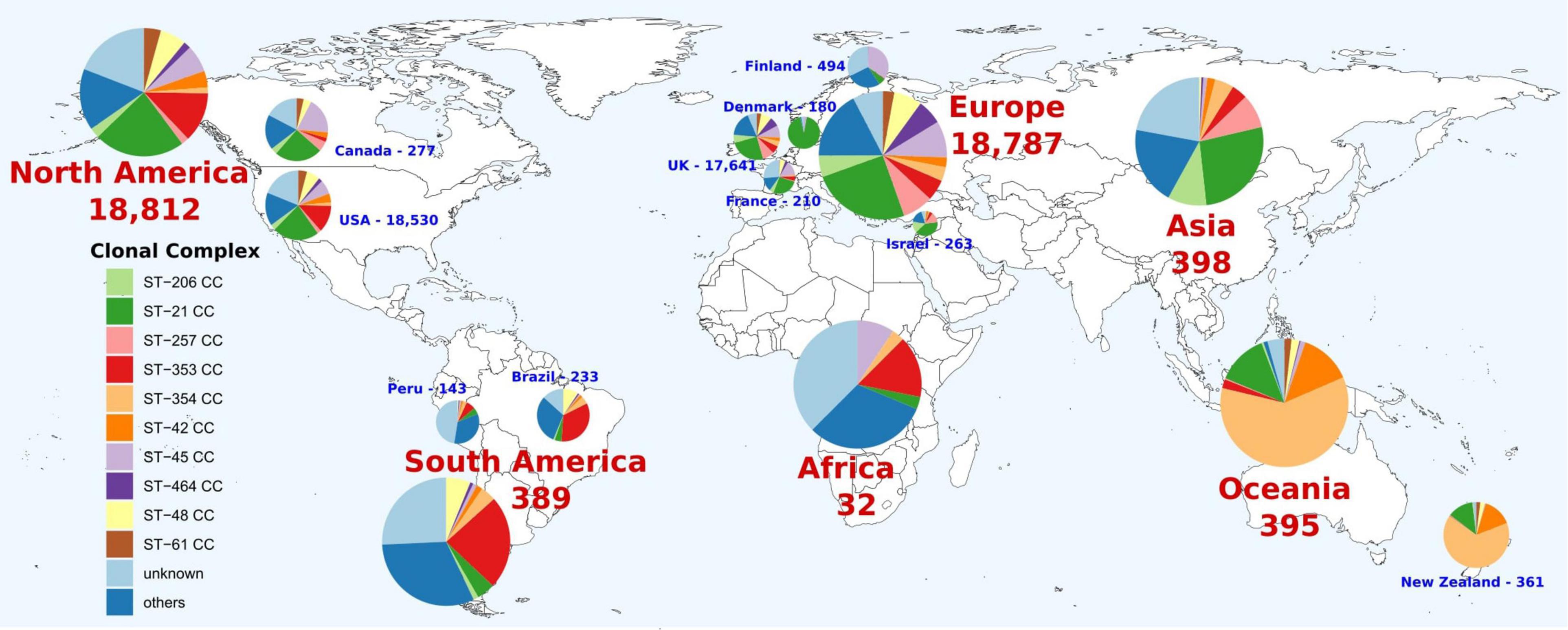
Figure 2. Geographical distribution of C. jejuni clonal complexes (CCs). Only countries with more than 100 genomes were represented. The number indicates the genomes available on the corresponding continent or country. Colors within pie charts represent the percentage of genomes belonging to the main CCs, with low abundant CCs grouped as “others” and genomes with unknown CCs as “unknown.”
The blaOXA–61 gene had the highest prevalence among C. jejuni genomes in all continents (from 36.5 to 92.4% of total genomes), except for South America, which had the gyrA (T86I) SNP as the main AR factor (39.1%) (Figure 3A). Oceania had the highest prevalence of tet(O), followed by North America and Asia (60, 46.9, and 42.7%, respectively), while the gyrA T86I SNP had the highest abundance on genomes obtained in Asia and Oceania (73.9 and 59.2%, respectively), which cluster together and separated from those from other continents (Figure 3A and Supplementary Figure 1A). There were some discrepancies at country level, where two main clusters were obtained, the second one containing genomes from Taiwan, Israel, New Zealand, Luxemburg, and China, countries with no geographical or even socio-economical relation, which harbored the highest prevalences of tet(O) gene and gyrA (T86I) SNP (Figure 3B and Supplementary Figure 1B).
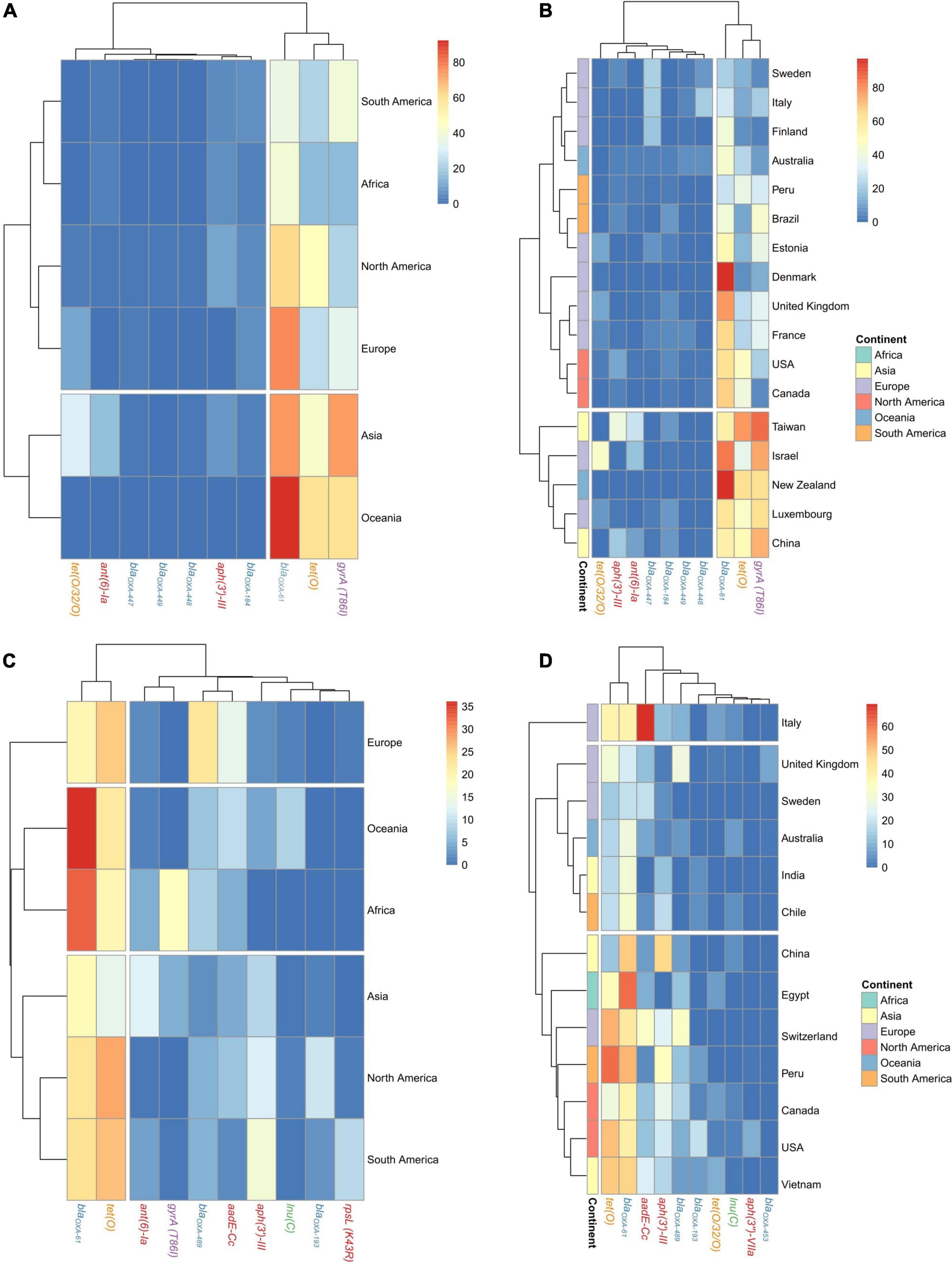
Figure 3. Geographical distribution of AR genes and SNPs on C. jejuni and C. coli genomes. Heatmap plots showing the distribution of the main AR genes and SNPs on C. jejuni genomes among (A) continents and (B) countries and on C. coli genomes among (C) continents and (D) countries. Countries with less than 20 genomes were discarded for this analysis. Gene names are colored according to the corresponding antimicrobial family (blue: beta-lactams, orange: tetracyclines, red: aminoglycosides, purple: quinolones, and green: MLSP).
The tet(O) and blaOXA–61 genes were the most abundant on C. coli regardless of the continent, with prevalences ranging from 16.9 to 36.1% and 13.7–28.1% of genomes, respectively. The aadE-Cc and aph(3′)-III genes showed an important presence on European genomes (22.6 and 13.6%, respectively), while ant(6’)-Ia had an important prevalence on North and South American genomes (11.8 and 15.6%, respectively) and the gyrA (T86I) SNP on Asian genomes (12.2%) (Figure 3C). At country level, using those countries with at least 20 genomes, the cluster formed by genomes from China, Egypt, Switzerland, Peru, Canada, United States, and Vietnam had a higher prevalence of aph(3′)-III, blaOXA–61, and tet(O) genes than the cluster formed by genomes from the United Kingdom, Sweden, Australia, India, and Chile (Figure 3D and Supplementary Figure 1).
Host Specialization and ARG-SNP Distribution by Source of Isolation
The analysis of the occurrence of C. jejuni CCs in each of the isolation sources, excluding human, showed three clear clusters of CCs. Chicken specialist and generalist CCs formed the biggest group, which was clearly subdivided into two sub-clusters according to host range. Interestingly, chicken was the most frequent source found on the generalist sub-cluster (Figure 4A). Previous studies (French et al., 2005; Griekspoor et al., 2010; Revez et al., 2011; Sheppard et al., 2014; Dearlove et al., 2016; Mourkas et al., 2020) have classified up to eight C. jejuni CCs as host generalist and 13 CCs as host specialist, including three CCs specialized in cattle infection, seven in chicken infection, and other three in wild bird infection or environmental persistence (Table 2). According to the clustering obtained, ST-460, ST-464, ST-574, ST-607, and ST-658 CCs, previously not classified according to their host specialist/generalist behavior, can be classified as chicken specialist CCs, while ST-52 CC can be classified as a generalist CC (Figure 4A and Table 2). On the other hand, ST-283 CC, previously described as a specialist chicken CC, was not located within the chicken specialist sub-cluster (Figure 4A), so a change to generalist host range can be proposed according to the data here presented. Another important observed cluster was the cluster of cattle specialist CCs, which also included the ST-22 CC, previously described as generalist (Figure 4A). In this case, the percentage of genomes assigned to the cow source among cattle specialist CCs, which ranged from 44.1 to 66.7% (excluding genomes isolated from human), was not as high as that of genomes assigned to chicken source for chicken specialist CCs, which was in all cases above 90%. In addition, cattle specialist CCs had similar percentages of abundance in the cow source than generalist CCs in the chicken source (Figure 4A and Supplementary Figure 2A). Therefore, it is important to remark the high level of specialization of those CCs considered as chicken specialist, compared to other specialist groups. Moreover, a ST host specialization analysis performed with the most abundant CCs showed that ST-45 CC harbors STs with medium levels of chicken specialization, while ST-353 and ST-354 CCs contain STs highly specialized for chicken (Figure 3B and Supplementary Figure 2B). Finally, the ST-21 CC contains three STs specialized in cattle infection (ST-8, ST-806, and ST-982), two specialized in chicken infection (ST-50 and ST-53), one ST specialized on sheep infection (ST-262), and two generalist STs (ST-19 and ST-21) (Figure 4B and Supplementary Figure 2B). The ST-858 CC, which was the main one found for C. coli genomes, harbors up to seven STs (ST-4425, ST-830, ST-1119, ST-872, ST-1614, ST-828, and ST-829) that could be considered as chicken infection specialist, three pig specialized STs (ST-1058, ST-854, and ST-887), one cattle specialist ST (ST-1068), and five host generalist STs (ST-1016, ST-1055, ST-827, ST-825, and ST-962) (Figure 4C and Supplementary Figure 2C). The complete list of STs analyzed, from both C. jejuni and C. coli genomes, and their host specilization were indicated on Supplementary Table 3.
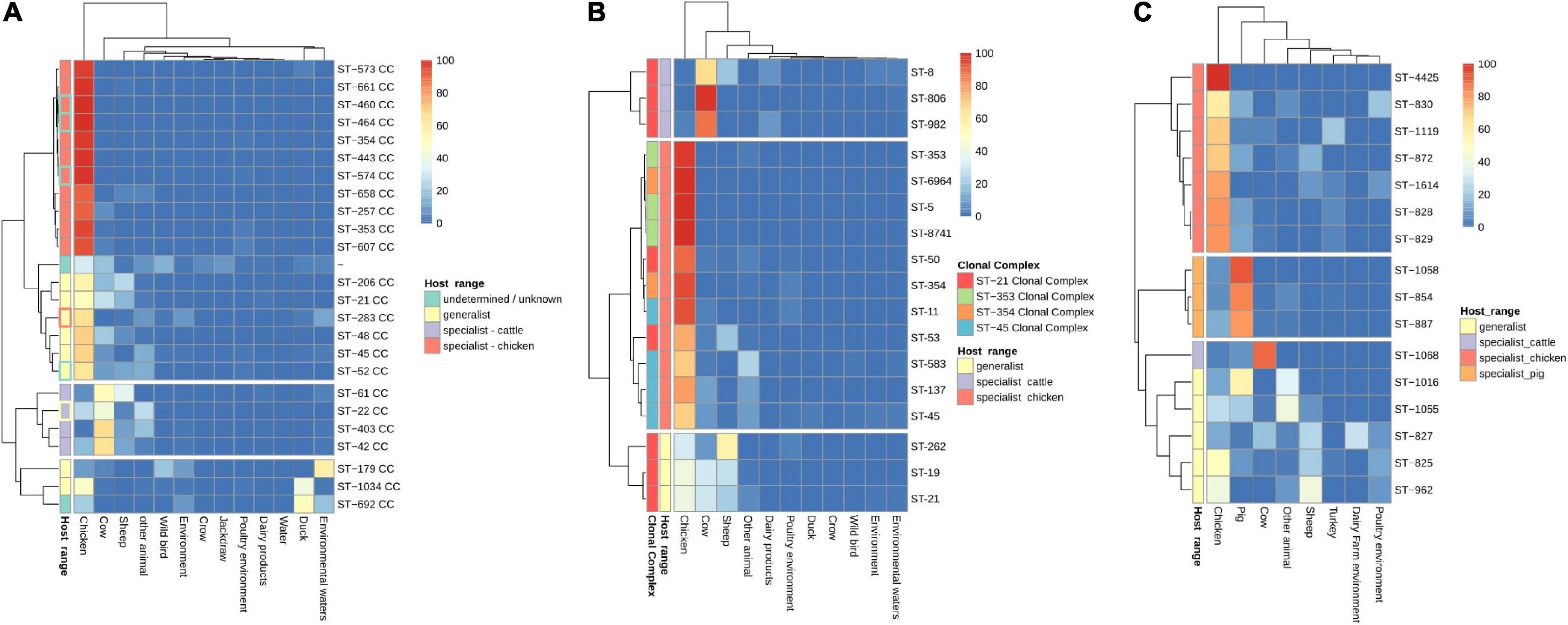
Figure 4. CC and sequence type (ST) host specialization. Heatmap plot showing (A) percentage of C. jejuni genomes per CC, (B) C. jejuni genomes per ST within the four main CCs, and (C) C. coli genomes per ST within ST-828 CC belonging to each isolation source. Genomes belonging to the human isolation source were removed before the calculation of the relative abundances, and CCs/STs with 20 or less genomes were removed to avoid biases due to the low number of genomes. The Host range classification of C. jejuni CCs is according to previous publications (see Table 1), except for those with highlighted edges, where edge color indicates previous classification while fill color indicates the new assignment, according to the results obtained in the current study.
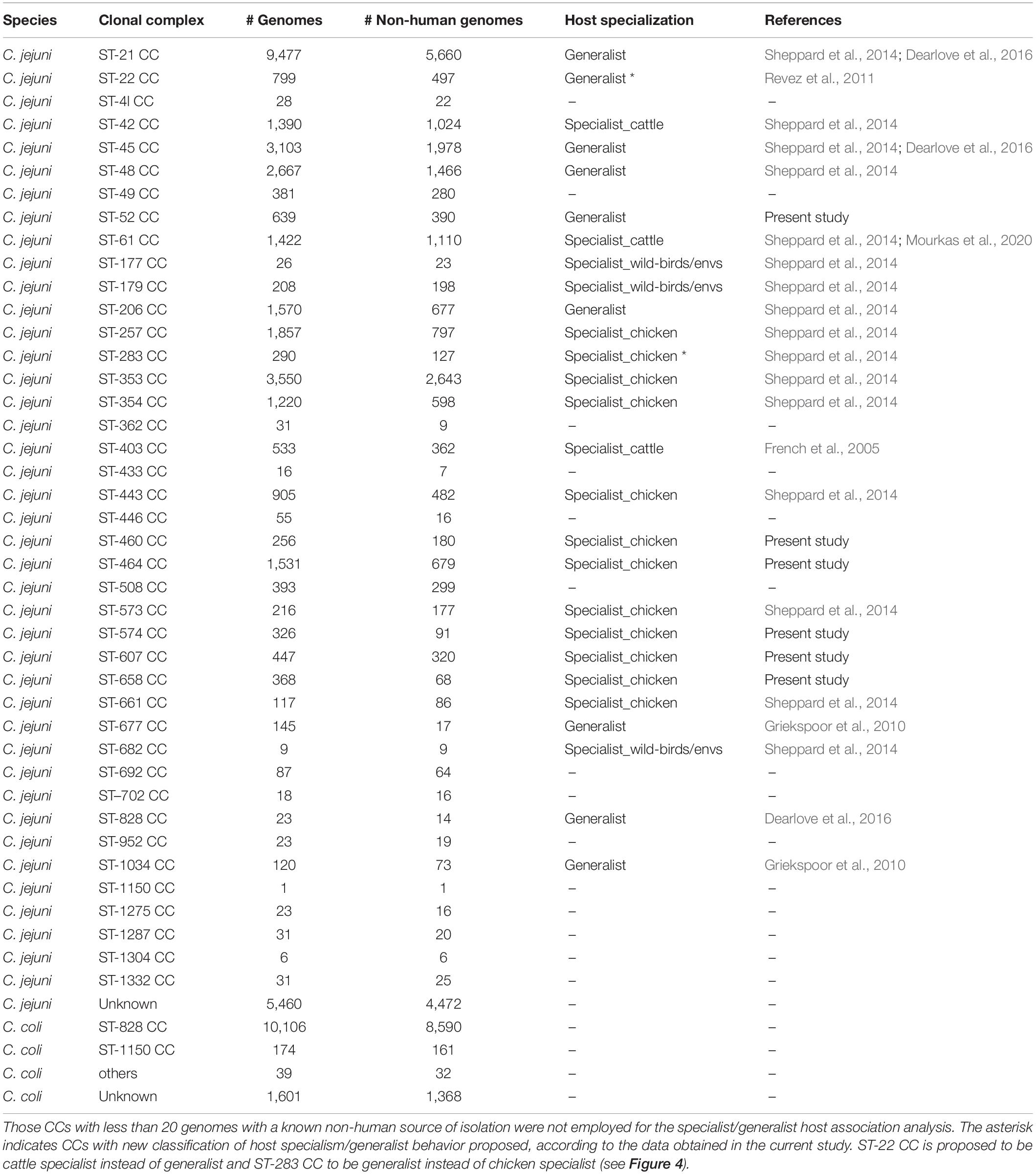
Table 2. Clonal complex classification by their host specialization according to previous publications and to the results of the current study.
The analysis of the occurrence of AR determinants in genomes from each of the isolation sources revealed three main clusters of both C. jejuni and C. coli genomes. The main cluster of C. jejuni genomes related to food production sources, which showed a higher prevalence of blaOXA–61 (from 44.7 to 100%) and of the gyrA (T86I) SNP (from 1.5 to 41.1%) than genomes from the second cluster obtained [from 0 to 12.84% for blaOXA–61; from 1.3 to 4.6% for the gyrA (T86I) SNP] (Figure 5A and Supplementary Figure 3A). This second cluster harbored genomes from isolates coming from host animals not linked to industrialized food production, such as jackdaws, crows, and other wild birds (Figure 5B). The lower prevalence of blaOXA–61 and the higher diversity of other genes associated with resistance to β-lactams among the genomes from this second cluster indicate a lower selection pressure to keep this prevalent blaOXA–61 gene in non-livestock animal populations. The last cluster of C. jejuni genomes was formed by genomes from duck, other animals, and environmental waters and presented an intermediate prevalence of blaOXA–61 compared to the other two clusters obtained (Figure 5A and Supplementary Figure 3A). Similar clusters were found for C. coli genomes, with pig, cow, and turkey forming the cluster with the highest prevalence of tet(O) and blaOXA–61, with tet(O) being more prevalent than on C. jejuni genomes while blaOXA–61 was less prevalent (Figure 5B and Supplementary Figure 3B). A second cluster of C. coli genomes was formed by genomes from dairy products, humans, chicken, and other animals and was characterized by an intermediate prevalence of tet(O) and blaOXA–61 genes, compared to the other clusters (Figure 5B and Supplementary Figure 3B). Finally, the third cluster, with the lowest prevalence of AR determinants, was formed by genomes from environmental samples, sheep, and duck. It is important to highlight the high prevalence of aadE-Cc on genomes from pigs and blaOXA–489 on genomes from sheep (Figure 5B).
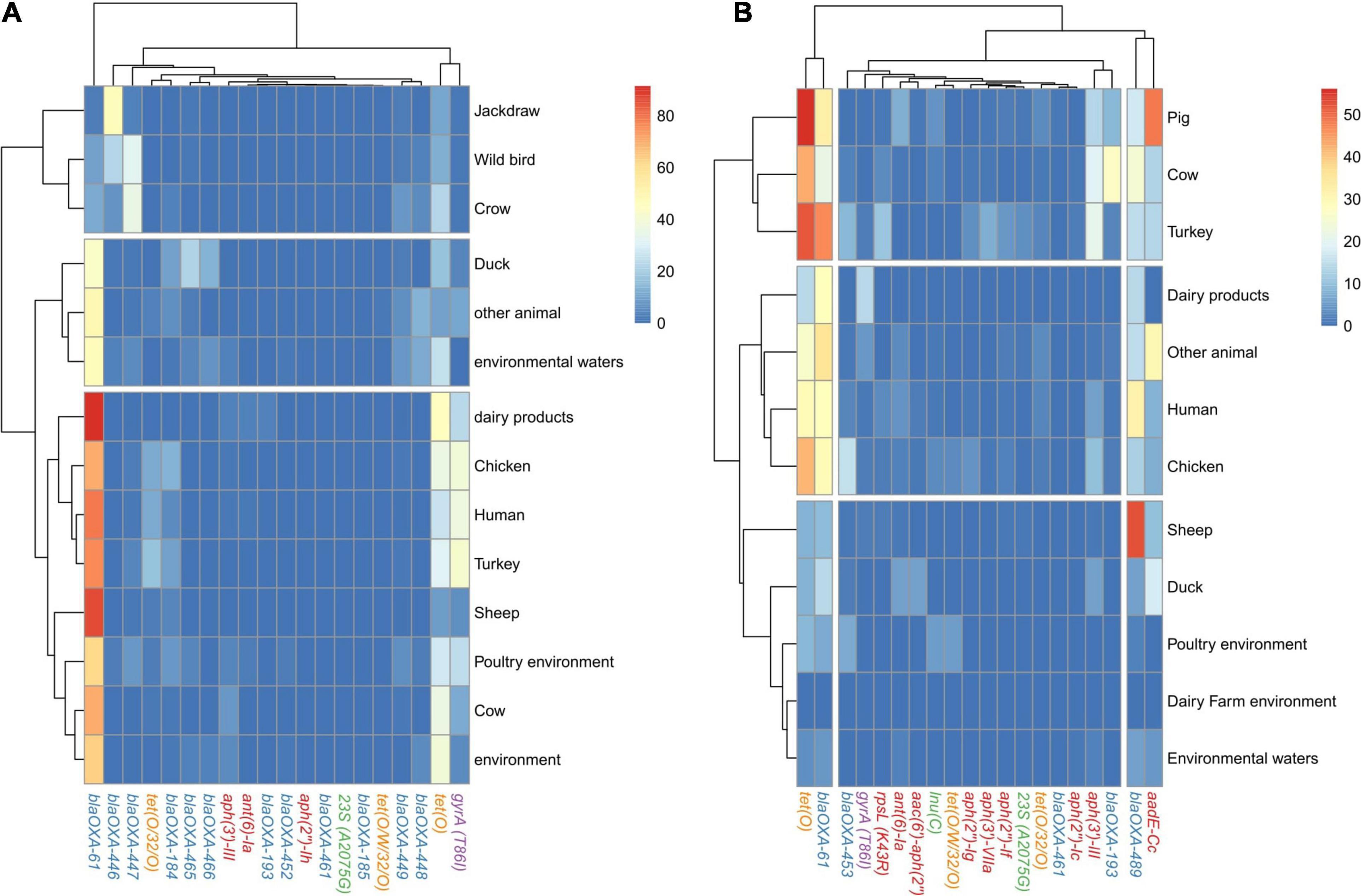
Figure 5. AR genes and SNP distribution among C. jejuni and C. coli genomes by source of isolation. Heatmap plot showing the percentage of (A) C. jejuni genomes and (B) C. coli genomes per isolation source carrying ARGs or SNPs associated with AR, showed in columns. Only the main 20 ARGs or SNPs are presented, because the clustering by isolation source remained the same as when the 120 and 68 AR determinants were used for C. jejuni and C. coli, respectively (data not shown). Only isolation sources containing more than 50 genomes are presented. Gene names are colored according to the corresponding antimicrobial family (blue: beta-lactams, orange: tetracyclines, red: aminoglycosides, purple: quinolones, and green: MLSP).
ARG-SNP Distribution Among C. jejuni CCs
Up to four main clusters were obtained in the analyses performed at antibiotic family level. Cluster 1 harbored four CCs with the highest prevalence of determinants of resistance to tetracyclines and quinolones, while cluster 2, formed by 20 CCs, had the second main prevalence for tetracycline and quinolone resistance determinants (Figure 6A and Supplementary Figure 4A). Cluster 3, formed by seven CCs, had the lowest prevalence of quinolone and tetracycline resistance determinants (Figure 6A and Supplementary Figure 4A), and cluster 4, formed by six CCs, had the lowest abundance of β-lactam resistance determinants (Figure 6A and Supplementary Figure 4A). At gene level, three clusters were found: cluster 1, harboring four CCs with a high prevalence of gyrA (T86I) SNP, blaOXA–61, and tet(O); cluster 2, composed of 20 CCs with a high prevalence of blaOXA–61; and cluster 3, with 14 CCs showing the lowest prevalence of gyrA (T86I) SNP, blaOXA–61, and tet(O) (Figure 6B and Supplementary Figure 4B). No clear association between genotype patterns at antibiotic family level and host specialist/generalist status was observed. As an example, cluster 1, characterized by a high prevalence of ARGs linked to resistance to tetracyclines and quinolones, was mainly formed by chicken specialist CCs, but other chicken specialist CCs were located across the other three remaining clusters (Figure 6A). A similar absence of a clear association with the host range of each CC was observed at gene level.
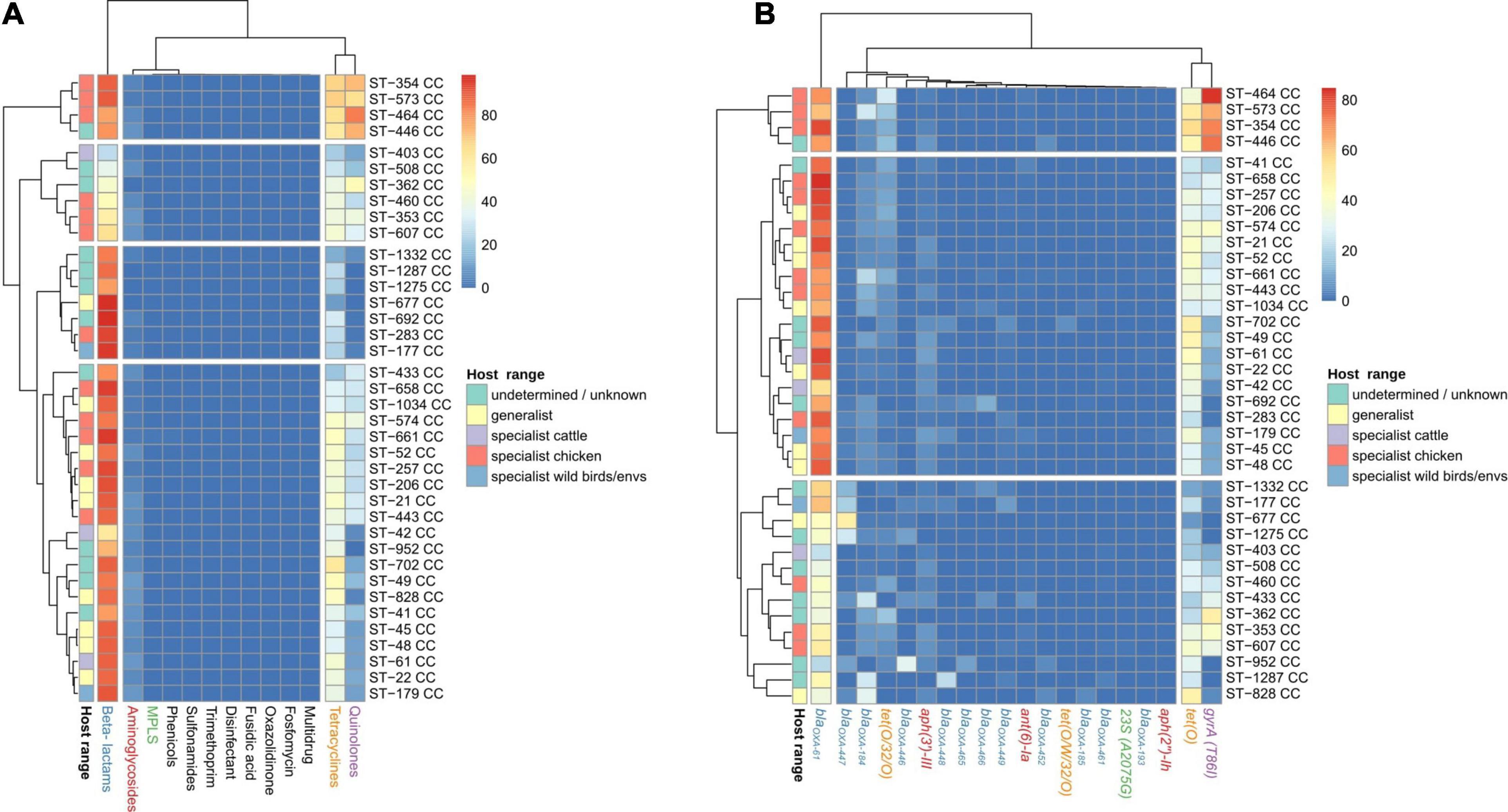
Figure 6. AR genes and SNP distribution among C. jejuni CCs. Heatmap plot showing the percentage of genomes per CC carrying (A) ARGs or SNPs associated with resistance to antibiotic families and (B) particular ARGs or SNPs, showed in columns. Only the main 20 ARGs or SNPs are presented, because the clustering by isolation source remained the same as when the 120 AR determinants were used (data not shown). The Host_range classification of CCs is according to previous publications (see Table 1), except for those with highlighted edges, where edge color indicates previous classification while fill color indicates the new assignment, according to the results showed in Figure 4. Gene names are colored according to the corresponding antimicrobial family (blue: beta-lactams, orange: tetracyclines, red: aminoglycosides, purple: quinolones, and green: MLSP).
Temporal Changes of AR Determinants on Europe
Focusing on temporal changes in the repertoire of AR determinants among the C. jejuni European genomes, there was a clear stability in the occurrence of β-lactam ARGs along time (with mean prevalence values per each 5-year period ranging from of 88.8 to 93.3%), while an important increase was observed in the prevalence of determinants of resistance to tetracyclines, from a mean value of 29.8% in the 2000–2004 5-year period to 44.0% in the 2015–2018 period, and quinolones, from 21.0% in the 2000–2004 5-year period to 42.4% in the 2015–2018 period (Figure 7A and Supplementary Figure 5A).
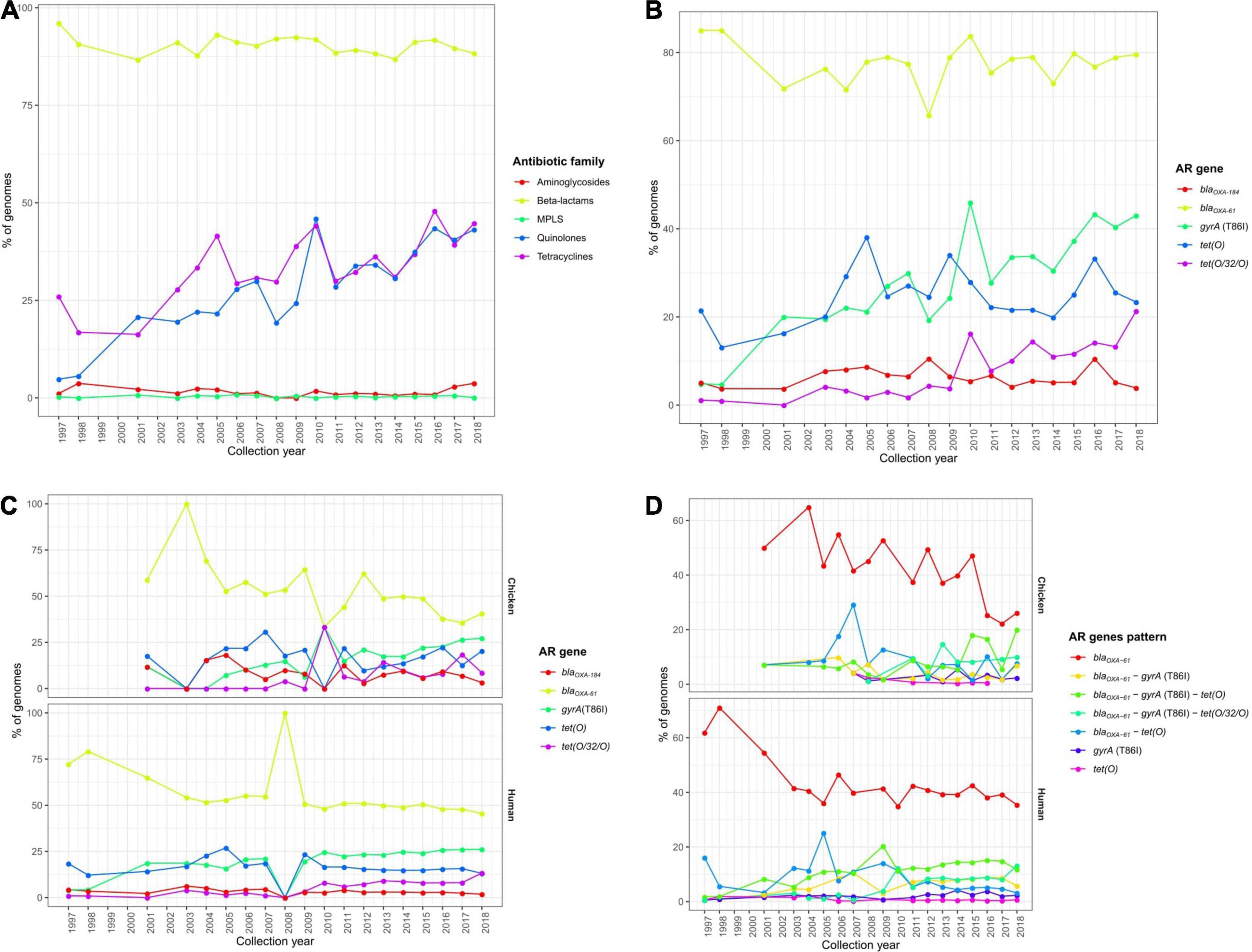
Figure 7. Temporal changes in the resistome of European C. jejuni genomes. Prevalence of (A) ARGs or SNPs associated with resistance to different antibiotic families and (B) the main ARGs or SNPs found on all European C. jejuni genomes and (C) main ARGs or SNPs and (D) AR patterns on C. jejuni genomes from chicken and human sources along the last 20 years.
These observations were linked to an increase in the occurrence of both the tet(O) and tet(O/W/O) genes and the gyrA (T86I) SNP (Figure 7B and Supplementary Figure 5B), which were the main determinants of resistance to tetracyclines and quinolones, respectively. Furthermore, the increases along time in the tet(O/W/O) gene and the gyrA (T86I) SNP were observed in both human and chicken genomes (Figure 7C and Supplementary Figures 5C,D). Finally, the enhanced occurrence over time of resistance to tetracyclines and quinolones was mainly correlated with a significant increase in blaOXA–61–tet(O)–gyrA (T86I) and blaOXA–61–tet(O/W/O)–gyrA (T86I) multi-drug resistance genotype patterns on C. jejuni genomes obtained from human and chicken, which showed mean prevalence values of 10.5 and 6.1% in the 2000–2004 5-year period and 25.6 and 29.9% in the 2015–2018 period, respectively, representing a significant increase in co-occurrence of such genes (Figure 7D and Supplementary Figures 5E,F).
Similar temporal analyses were performed on C. coli genomes, but no clear trends of AR determinant increase along time were detected. The observed slight increases in the prevalence of determinants of aminoglycoside and tetracycline resistance (Figure 8A), highly correlated with an increase of addE-Cc and tet(O) prevalence (Figure 8B), were associated with a differential prevalence on genomes from cows and pigs, with more C. coli genomes being sequenced on the last years (Figures 8C,D).
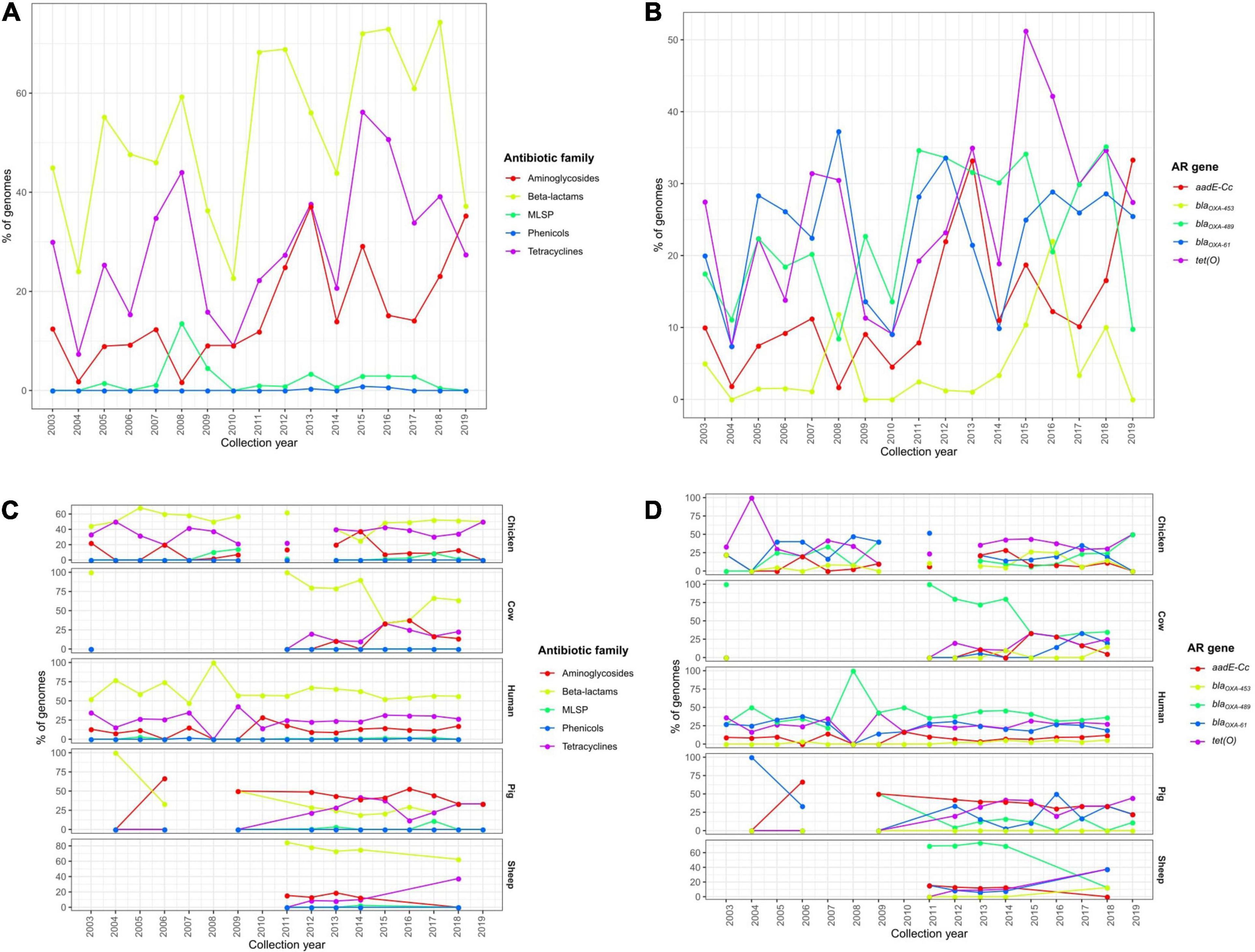
Figure 8. Temporal changes in the resistome of European C. coli genomes. Prevalence of (A) ARGs or SNPs associated with resistance to different antibiotic families and (B) the main ARGs or SNPs found on all European C. coli genomes and (C) the main ARGs or SNPs and (D) AR patterns on C. jejuni genomes from chicken, cow, human, pig, and sheep sources along the last 20 years.
Discussion
At a first global view, the distribution of C. jejuni CCs was different among continents, with ST-21 CC as the main one in North America, Europe, and Asia; ST-353 CC in South America and Africa, and ST-354 CC in Oceania. Moreover, this distribution by continent was not maintained among all the countries within a given continent, i.e., Denmark harbored a strong dominance of ST-21 CC genomes while Finland had ST-45 CC as the most abundant CC. Surprisingly, a lack of main association within developing–developed countries and AR composition on C. jejuni and C. coli genomes was clearly found, maybe due to the unequal distribution of both CC-STs and host origin of isolation among countries and even the amount of sequenced genomes for each country.
Results obtained in the current study reveal the association of certain C. jejuni CCs and both C. jejuni and C. coli STs to particular isolation sources. In this regard, it is worth mentioning the high abundance of CCs linked to chicken as a host. Most of these C. jejuni CCs had been already classified as a chicken specialist (Sheppard et al., 2014), and our study confirmed these previous associations, while ST-283 CC, previously described as a chicken specialist (Sheppard et al., 2014), can be considered as a generalist according to our findings. Moreover, among previously not assigned CCs, ST-460, ST-464, ST-574, ST-607, and ST-658 CCs can be considered as a chicken specialist, ST-52 CC as a generalist, and ST-22 CC as a cattle specialist, according to the results here reported. The high abundance of genomes from generalist ST-21 and chicken specialist ST-353 CCs agrees with previous reports that have described these CCs as highly prevalent among clinical, food, and animal samples from Israel (Rokney et al., 2018); clinical cases, dairy cattle, and broiler products in Lithuania (Ramonaite et al., 2017); chicken in Senegal (Kinana et al., 2006); or human and broiler carcasses in Belgium (Elhadidy et al., 2018). The high abundance of ST-21, ST-48, ST-45, and ST-257 CCs on isolates obtained from human samples has been previously highlighted in the United Kingdom (Haldenby et al., 2020). Interestingly, the STs within ST-45 CC, previously considered as a generalist CC, could be considered as chicken specialist STs. Conclusions on host specialization have to be taken carefully. For example, the generalist ST-21 CC harbored STs considered as chicken specialist (ST-50 and ST-53), cow specialist (ST-8, ST-806, and ST-982), and generalist (ST-19, ST-21, and ST-262). This host specialization differences within ST-21 CC could be due to the paraphyletic phylogeny of this CC, which was found as the ancestor CC of the cattle specialist ST-61 CC (Sheppard et al., 2014; Mourkas et al., 2020). The prevalence of ST-353, ST-354, ST-50, and ST-45 on chicken hosts and ST-21 as a host generalist were previously reported by using all the profiles on PubMLST (Pascoe et al., 2020), which are twice the number of genomes available on that web repository and employed in the current study. That supports the proposed host specialization classification, despite the possible biases introduced due to the isolates selected or employed for whole genome sequencing within all the available collections of bacterial isolates.
Determinants of resistance to β-lactams and tetracyclines were the most extended among the 39,798 C. jejuni and 11,920 C. coli genomes analyzed, with blaOXA–61 as the main β-lactam resistance factor on C. jejuni; blaOXA–61, blaOXA–489, and blaOXA–193 on C. coli; and tet(O) for tetracycline resistance on both species. Determinants of resistance to quinolones [due mainly to the gyrA (T86I) SNP] and to aminoglycosides [by aph(3′)-III, aadE-Cc, and aph(3′)-VIIa genes, among others) were also highly prevalent on C. jejuni and C. coli genomes, respectively. Phenotypic resistance to β-lactam antibiotics in C. jejuni is frequently linked to the production of β-lactamase enzymes, encoded by blaOXA genes (Jonker and Picard, 2010), while tetracycline and quinolone resistance has been often associated with the tet(O) gene (Meistere et al., 2019) and the gyrA (T86I) SNP, respectively (Kinana et al., 2006; Meistere et al., 2019; Abraham et al., 2020; Joensen et al., 2020). Indeed, a high prevalence of blaOXA–61 and tet(O) has been found on C. jejuni isolated from human, chicken, and cattle in Spain in a study where SNPs were not screened (Mourkas et al., 2019) and on Campylobacter spp. in a global study analyzing 237 genomes from the RefSeq NCBI database (Rivera-Mendoza et al., 2020). Moreover, AR determinants of aminoglycoside resistance on C. coli and quinolone resistance on C. jejuni were mainly found on genomes associated with multidrug resistance, together with AR determinants for β-lactams and tetracyclines.
The increase in resistance to quinolones on Campylobacter isolates during the last years has been reported worldwide, including the United States (Nachamkin et al., 2002), China (Wang et al., 2016), France (Gallay et al., 2007), Vietnam (Nguyen et al., 2016), and Peru (Pollett et al., 2012), among other countries, and the usage of fluoroquinolones in poultry farms has been associated with an increase in resistance to quinolones in chicken and human Campylobacter isolates (Wieczorek and Osek, 2013). An increased occurrence of determinants of resistance to β-lactams, tetracyclines, and quinolones was found among those C. jejuni and C. coli genomes from human and food animal origin. The clear differences observed in the resistome between Campylobacter genomes from animals employed for food production and those from non-livestock animals can be likely related to the selective pressure exerted by antibiotic use in veterinary settings. Isolates from livestock species were characterized by a higher incidence of blaOXA–61, associated with resistance to β-lactam antibiotics, such as amoxicillin or ampicillin, antibiotics that are frequently employed for veterinary uses. Quinolones and tetracyclines are still available for treatment of livestock all over the world (WHO, 2017), so this is likely the main cause of the resistance levels found for such antibiotics on isolates from food-related animals. Antibiotic resistance represents a great concern mainly in livestock animals due to the misuse of antibiotics. As an example, erythromycin (macrolide), ampicillin (β-lactam), tetracycline (tetracycline), nalidixic acid, and ciprofloxacin (both belonging to the quinolones family) are the drugs toward which the most resistant isolates were found in a recent review on Campylobacter spp. in Sub-Saharan African countries (Hlashwayo et al., 2020), which is in agreement with results found globally or also with the temporal changes among European isolates described in the current study.
Although the current study does not involve a source attribution analysis, the similar ARG genotype patterns and levels of prevalence of blaOXA–61, tet(O), and gyrA (T86I) found among human, chicken, and turkey C. jejuni genomes, and of blaOXA–61 and tet(O) among human and chicken C. coli genomes, suggest a high level of relatedness among isolates from these origins. Poultry has been reported as the main source of human infection in studies carried out in different locations including Denmark (Joensen et al., 2020), Sub-Saharan Africa (Gahamanyi et al., 2020), or Lithuania (Ramonaite et al., 2017). On the other hand, other authors have identified as important sources of human infection both chicken and ruminants in France (Berthenet et al., 2019), the United States (Kelley et al., 2020), Israel (Rokney et al., 2018), and Sub-Saharan Africa (Hlashwayo et al., 2020) or chicken and wild birds in Baltic countries (Mäesaar et al., 2020). Moreover, the detection of no major differences in the resistance profiles among isolates from different points of the chicken meat processing chain (Dramé et al., 2020) suggests that AR spread is originated on upstream steps, such as the animal feeding, where the animals are exposed to antibiotics. A clear link between human and chicken Campylobacter isolates has been previously demonstrated by combining pulsed-field gel electrophoresis (PFGE) to subtype strains and antibiotic resistance phenotypic testing (Zhao et al., 2015).
The spread of AR genes among Campylobacter isolates from humans, animals, and the environment has been reported previously (Mourkas et al., 2019), and it should explain the presence of high levels of ARGs conferring resistance to tetracyclines in human isolates despite these antibiotics are not clinically employed in human medicine. Moreover, tetracycline is broadly employed in the poultry industry worldwide (Van Boeckel et al., 2015), which could be an important reservoir for resistant strains. Furthermore, higher levels of resistant C. jejuni and C. coli isolates were observed among isolates from pigs than from wild boars in Italy (Marotta et al., 2020), where an important amount of multidrug-resistant strains were isolated from pigs, being the over-use of antibiotics the main cause of the spread of multidrug resistance among pig isolates.
The blaOXA–61 gene is highly prevalent among Campylobacter spp. isolated from poultry (Griggs et al., 2009). The high diversity and abundance of determinants of resistance to β-lactams different to blaOXA–61 among isolates from non-food-related animals and environments could be due to the ability of Campylobacter spp. for intrinsic production of β-lactamases in the absence of selective pressure (Cody et al., 2012). The blaOXA–61 gene, chromosomally encoded (Rivera-Mendoza et al., 2020), can generate many variants with few SNPs, differing from other blaoxa-encoded proteins in only one (e.g., blaOXA–193) or two amino acids (e.g., blaOXA–489). The blaOXA–61 gene and aminoglycoside resistance genes are confined to C. jejuni and C. coli genomes within Campylobacter species, while gyrA T86I SNP was mostly presented on C. jejuni (Rivera-Mendoza et al., 2020). Unfortunately, ACT to ATT mutation on gyrA position 86, the most common on C. coli strains with resistance to quinolones (Zirnstein et al., 2000), was not included on PointFinder database, which includes ACA to ATA mutation, the most prevalent on C. jejuni. So that, the prevalence of quinolone resistance among C. coli genomes found on the present study can be due to this technical issue.
The clonal complexes ST-354, ST-573, ST-464, and ST-446, the first three considered chicken specialist, were the CCs with the highest prevalence of ARGs linked to β-lactam, tetracycline, and quinolone resistance, due to the presence of the blaOXA–61, tet(O), and gyrA (T86I) determinants for all of them. Some of these CCs (ST-354, ST-446, and ST-464) have been previously associated with ciprofloxacin (quinolone) resistance in human isolates (Cody et al., 2012). ARGs were more randomly distributed among CCs than among isolation sources. Hence, ARG genotype patterns were more closely related to the source of isolation (and probably the use of antibiotics) than to the lineage of the strains, as also remarked previously (Cody et al., 2012).
An increase in the occurrence of genotypes of resistance to tetracyclines and quinolones, mainly due to the spread of blaOXA–61–gyrA (T86I)–tet(O) and blaOXA–61–gyrA (T86I)–tet(O/W/O) multi-drug resistance genotypes, was observed along the last 20 years in European C. jejuni genomes. The main reason for this temporal trend could be the continuous misuse of tetracyclines and quinolones, still broadly employed on livestock all over the world (WHO, 2017). Interestingly, fluoroquinolones are not utilized in Australian livestock, where Campylobacter isolates from chicken meat show very scarce levels of resistance to fluoroquinolones, always due to gyrA (T86I) SNPs (Abraham et al., 2020). On the other hand, an increase over time in the prevalence of the gyrA (T86I) SNP among isolates of C. jejuni associated with human gastrointestinal disease has been reported in the United Kingdom from 1993–1996 to 2008–2009 and even to 2016–2017 (Haldenby et al., 2020). This spread of resistance to quinolones is an important concern in some countries, such as Latvia, where resistance to quinolones was found, due to gyrA (T86I), in 90% of the C. jejuni isolates obtained from clinical and food-related sources from 2008 to 2016 (Meistere et al., 2019). Remarkably, it has been demonstrated that high levels of fluoroquinolone resistance can persist in poultry even after discontinued use of these antibiotics (Price et al., 2005) and that resistance can rapidly emerge in poultry flocks (Humphrey et al., 2005). Some fitness benefits linked to the gyrA (T86I) SNP could be the reason for such high resistance prevalence in settings where the use of fluoroquinolones has been reduced, as has been proposed by Haldenby et al. (2020). Another example of temporal increases in fluoroquinolone resistance among Campylobacter species was observed on fecal samples from cattle in France between 2002 and 2006, with the fluoroquinolone resistance rate rising from 29.7% to 70.4% (Châtre et al., 2010).
In conclusion, the current work represents the biggest study to date, as far as we know, of the resistome markers harbored in C. jejuni/coli genomes, with 39,978 C. jejuni and 11,920 C. coli genomes in silico screened to search both for antimicrobial resistance genes and SNPs conferring antimicrobial resistance. The results obtained suggest the association between antimicrobial use in veterinary settings, in particular in poultry production, and the spread of resistance to Be, Qu, and Te and evidence the rapid expansion in Europe in the last two decades of determinants of resistance to these antibiotic families, especially among poultry and human isolates. The workflow employed is available to be used in future global resistome analyses for other species of interest, or even to be adapted to other kinds of microbiome analyses.
Data Availability Statement
The original contributions presented in the study are included in the article/Supplementary Material, further inquiries can be directed to the corresponding author.
Author Contributions
AÁ-O and JC-D created the study design. JC-D and PG wrote and employed the Ruby and R scripts and downloaded genomes, made data analysis, and plotted the figures. JC-D and PG wrote the first draft of the manuscript and AÁ-O revised it. All authors revised the manuscript and approved the final version.
Funding
Research in the laboratory of AÁ-O was funded by the European Commission under European Union’s Horizon 2020 Research and Innovation Program under grant agreement no. 818368 and the Ministry of Science and Innovation of the Spanish Government (AGL2016- 78085-P).
Conflict of Interest
The authors declare that the research was conducted in the absence of any commercial or financial relationships that could be construed as a potential conflict of interest.
Acknowledgments
We acknowledge the teams involved in the maintenance of PUBMLST, NCBI, and PATRIC web-sites, for making available such amount of information and data for the entire scientific community.
Supplementary Material
The Supplementary Material for this article can be found online at: https://www.frontiersin.org/articles/10.3389/fmicb.2021.662144/full#supplementary-material
Footnotes
- ^ https://patricbrc.org/view/Taxonomy/2#view_tab=genomes
- ^ https://pubmlst.org/bigsdb?db=pubmlst_Campylobacter_isolates&page=plugin&name=Export
- ^ https://github.com/JoseCoboDiaz/download_genomes
- ^ ftp://ftp.ncbi.nlm.nih.gov/genomes/
- ^ ftp://ftp.patricbrc.org/
- ^ https://pubmlst.org
- ^ ftp://ftp.ncbi.nlm.nih.gov/genomes/GENOME_REPORTS/prokaryotes.txt
References
Aarestrup, F. M., McDermott, P. F., and Wegener, H. C. (2008). Transmission of Antibiotic Resistance from Food Animals to Humans, 3rd Edn. Washington, DC: American Society for Microbiology. doi: 10.1128/9781555815554
Abraham, S., Sahibzada, S., Hewson, K., Laird, T., Abraham, R., Pavic, A., et al. (2020). Emergence of fluoroquinolone-resistant Campylobacter jejuni and Campylobacter coli among Australian chickens in the absence of fluoroquinolone use. Appl. Environ. Microbiol. 86:2765. doi: 10.1128/AEM.02765-19
Asuming-Bediako, N., Kunadu, A. P.-H., Abraham, S., and Habib, I. (2019). Campylobacter at the human-food interface: the african perspective. Pathogens 8:87. doi: 10.3390/pathogens8020087
Berthenet, E., Thépault, A., Chemaly, M., Rivoal, K., Ducournau, A., Buissonnière, A., et al. (2019). Source attribution of Campylobacter jejuni shows variable importance of chicken and ruminants reservoirs in non-invasive and invasive French clinical isolates. Sci. Rep. 9:8098. doi: 10.1038/s41598-019-44454-2
Bronnec, V., Turoòová, H., Bouju, A., Cruveiller, S., Rodrigues, R., Demnerova, K., et al. (2016). Adhesion, biofilm formation, and genomic features of Campylobacter jejuni bf, an atypical strain able to grow under aerobic conditions. Front. Microbiol. 7:1002. doi: 10.3389/fmicb.2016.01002
Châtre, P., Haenni, M., Meunier, D., Botrel, M. A., Calavas, D., and Madec, J. Y. (2010). Prevalence and antimicrobial resistance of Campylobacter jejuni and Campylobacter coli isolated from cattle between 2002 and 2006 in France. J. Food. Prot. 73, 825–831. doi: 10.4315/0362-028x-73.5.825
Clark, C. G., Berry, C., Walker, M., Petkau, A., Barker, D. O. R., Guan, C., et al. (2016). Genomic insights from whole genome sequencing of four clonal outbreak Campylobacter jejuni assessed within the global C. jejuni population. BMC Genomics 17:990. doi: 10.1186/s12864-016-3340-8
Cody, A. J., McCarthy, N. M., Wimalarathna, H. L., Colles, F. M., Clark, L., Bowler, I. C. J. W., et al. (2012). A longitudinal 6-year study of the molecular epidemiology of clinical Campylobacter isolates in Oxfordshire, United Kingdom. J. Clin. Microbiol. 50, 3193–3201. doi: 10.1128/JCM.01086-12
Cody, A. J., Maiden, M. C. J., Strachan, N. J. C., and McCarthy, N. D. (2019). A systematic review of source attribution of human campylobacteriosis using multilocus sequence typing. Eurosurveillance 24:1800696. doi: 10.2807/1560-7917.ES.2019.24.43.1800696
Dearlove, B., Cody, A., Pascoe, B., Méric, G., Wilson, D. J., and Sheppard, S. K. (2016). Rapid host switching in generalist Campylobacter strains erodes the signal for tracing human infections. ISME J. 10, 721–729.
de Perio, M. A., Niemeier, R. T., Levine, S. J., Gruszynski, K., and Gibbins, J. D. (2013). Campylobacter infection in poultry-processing workers, Virginia, USA, 2008 -2011. Emerg. Infect. Dis. 19, 286–288. doi: 10.3201/eid1902.121147
Domingues, A. R., Pires, S. M., Halasa, T., and Hald, T. (2012). Source attribution of human campylobacteriosis using a meta-analysis of case-control studies of sporadic infections. Epidemiol. Infect. 140, 970–981. doi: 10.1017/S0950268811002676
Dramé, O., Leclair, D., Parmley, E. J., Deckert, A., Ouattara, B., Daignault, D., et al. (2020). Antimicrobial resistance of Campylobacter in broiler chicken along the food chain in Canada. Foodborne Path. Dis. 17, 512–520. doi: 10.1089/fpd.2019.2752
Elhadidy, M., Arguello, H., Álvarez-Ordóñez, A., Miller, W. G., Duarte, A., Martiny, D., et al. (2018). Orthogonal typing methods identify genetic diversity among Belgian Campylobacter jejuni strains isolated over a decade from poultry and cases of sporadic human illness. Int. J. Food Microbiol. 275, 66–75. doi: 10.1016/j.ijfoodmicro.2018.04.004
European Food Safety Authority (2018). The European Union summary report on trends and sources of zoonoses, zoonotic agents and food-borne outbreaks in 2017. EFSA J. 16:5500. doi: 10.2903/j.efsa.2018.5500
Fernandes, A. M., Balasegaram, S., Willis, C., Wimalarathna, H. M. L., Maiden, M. C., and McCarthy, N. D. (2015). Partial failure of milk pasteurization as a risk for the transmission of Campylobacter from cattle to humans. Clin. Infect. Dis. 61, 903–909. doi: 10.1093/cid/civ431
Fischer Walker, C. L., Rudan, I., Liu, L., Nair, H., Theodoratou, E., Bhutta, Z. A., et al. (2013). Global burden of childhood pneumonia and diarrhoea. Lancet 381, 1405–1416. doi: 10.1016/S0140-6736(13)60222-6
French, N., Barrigas, M., Brown, P., Ribiero, P., Williams, N., Leatherbarrow, H., et al. (2005). Spatial epidemiology and natural population structure of Campylobacter jejuni colonizing a farmland ecosystem. Environ. Microbiol. 7, 1116–1126. doi: 10.1111/j.1462-2920.2005.00782.x
Gahamanyi, N., Mboera, L., Matee, M. I., Mutangana, D., and Komba, E. (2020). Prevalence, risk factors, and antimicrobial resistance profiles of thermophilic Campylobacter species in humans and animals in Sub-Saharan Africa: a systematic review. Int. J. Microbiol. 2020:2092478. doi: 10.1155/2020/2092478
Gallay, A., Prouzet-Mauleon, V., Kempf, I., Lehours, P., Labadi, L., Camou, C., et al. (2007). Campylobacter antimicrobial drug resistance among humans, broiler chickens, and pigs, France. Emerg. Infect. Dis. 13, 259–266. doi: 10.3201/eid1302.060587
Griekspoor, P., Engvall, E. O., Olsen, B., and Waldenström, J. (2010). Multilocus sequence typing of Campylobacter jejuni from broilers. Vet. Microbiol. 140, 180–185. doi: 10.1016/j.vetmic.2009.07.022
Griggs, D. J., Peake, L., Johnson, M. M., Ghori, S., Mott, A., and Piddock, L. J. V. (2009). β-lactamase-mediated β-lactam resistance in Campylobacter species: prevalence of Cj0299 (blaOXA-61) and evidence for a novel β-lactamase in C. jejuni. Antimicrob. Agents Chemother. 53, 3357–3364. doi: 10.1128/AAC.01655-08
Haldenby, S., Bronowski, C., Nelson, C., Kenny, J., Martinez-Rodriguez, C., Chaudhuri, R., et al. (2020). Increasing prevalence of a fluoroquinolone resistance mutation amongst Campylobacter jejuni isolates from four human infectious intestinal disease studies in the United Kingdom. PLoS One 15:e0227535. doi: 10.1371/journal.pone.0227535
Hlashwayo, D. F., Sigaúque, B., and Bila, C. G. (2020). Epidemiology and antimicrobial resistance of Campylobacter spp. in animals in Sub-Saharan Africa: a systematic review. Heliyon 6:e03537. doi: 10.1016/j.heliyon.2020.e03537
Humphrey, T. J., Jørgensen, F., Frost, J. A., Wadda, H., Domingue, G., Elviss, N. C., et al. (2005). Prevalence and subtypes of ciprofloxacin-resistant Campylobacter spp. in commercial poultry flocks before, during, and after treatment with fluoroquinolones. Antimicrob. Agents Chemother. 49, 690–698. doi: 10.1128/AAC.49.2.690-698.2005
Joensen, K. G., Kiil, K., Gantzhorn, M. R., Nauerby, B., Engberg, J., Holt, H. M., et al. (2020). Whole-genome sequencing to detect numerous Campylobacter jejuni outbreaks and match patient isolates to sources, Denmark, 2015-2017. Emerg. Infect. Dis. 26, 523–532. doi: 10.3201/eid2603.190947
Jolley, K. A., Bray, J. E., and Maiden, M. C. J. (2018). Open-access bacterial population genomics: BIGSdb software, the PubMLST.org website and their applications. Wellcome Open Res. 3:124. doi: 10.12688/wellcomeopenres.14826.1
Jonker, A., and Picard, J. A. (2010). Antimicrobial susceptibility in thermophilic Campylobacter species isolated from pigs and chickens in South Africa. J. S. Afr. Vet. Assoc. 81, 228–236. doi: 10.4102/jsava.v81i4.153
Kaakoush, N. O., Castaño-Rodríguez, N., Mitchell, H. M., and Man, S. M. (2015). Global epidemiology of Campylobacter infection. Clin. Microbiol. Rev. 28, 687–720. doi: 10.1128/CMR.00006-15
Kelley, B. R., Ellis, J. C., Large, A., Schneider, L. G., Jacobson, D., and Johnson, J. G. (2020). Whole-Genome Sequencing and bioinformatic analysis of environmental, agricultural, and human Campylobacter jejuni isolates from East Tennessee. Front. Microbiol. 11:571064. doi: 10.3389/fmicb.2020.571064
Kinana, A. D., Cardinale, E., Tall, F., Bahsoun, I., Sire, J.-M., Garin, B., et al. (2006). Genetic diversity and quinolone resistance in Campylobacter jejuni isolates from poultry in Senegal. Appl. Environ. Microbiol. 72, 3309–3313. doi: 10.1128/AEM.72.5.3309-3313.2006
Lahti, E., Rehn, M., Ockborn, G., Hansson, I., Ågren, J., Engvall, E. O., et al. (2017). Outbreak of campylobacteriosis following a dairy farm visit: confirmation by genotyping. Foodborne Pathog. Dis. 14, 326–332. doi: 10.1089/fpd.2016.2257
Levallois, P., Chevalier, P., Gingras, S., Dery, P., Payment, P., Michel, P., et al. (2014). Risk of infectious gastroenteritis in young children living in Quebec rural areas with intensive animal farming: results of a case-control study (2004 -2007). Zoonoses Public Health 61, 28–38. doi: 10.1111/zph.12039
Lévesque, S., Frost, E., Arbeit, R. D., and Michaud, S. (2008). Multilocus sequence typing of Campylobacter jejuni isolates from humans, chickens, raw milk, and environmental water in Quebec, Canada. J. Clin. Microbiol. 46, 3404–3411. doi: 10.1128/JCM.00042-08
Liu, L., Oza, S., Hogan, D., Perin, J., Rudan, I., Lawn, J. E., et al. (2015). Global, regional, and national causes of child mortality in 2000-13, with projections to inform post-2015 priorities: an updated systematic analysis. Lancet 385, 430–440. doi: 10.1016/S0140-6736(14)61698-6
Mäesaar, M., Tedersoo, T., Meremäe, K., and Roasto, M. (2020). The source attribution analysis revealed the prevalent role of poultry over cattle and wild birds in human campylobacteriosis cases in the Baltic States. PLoS One 15:e0235841. doi: 10.1371/journal.pone.0235841
Marotta, F., Di Marcantonio, L., Janowicz, A., Pedonese, F., Di Donato, G., Ardelean, A., et al. (2020). Genotyping and antibiotic resistance traits in Campylobacter jejuni and coli from pigs and wild boars in Italy. Front. Cell. Infect. Microbiol. 10:592512. doi: 10.3389/fcimb.2020.592512
Meistere, I., Íibilds, J., Eglîte, L., Alksne, L., Avsejenko, J., Cibrovska, A., et al. (2019). Campylobacter species prevalence, characterisation of antimicrobial resistance and analysis of whole-genome sequence of isolates from livestock and humans, Latvia, 2008 to 2016. Eur. Surveill 24:1800357. doi: 10.2807/1560-7917.ES.2019.24.31.1800357
Mossong, J., Mughini-Gras, L., Penny, C., Devaux, A., Olinger, C., Losch, S., et al. (2016). Human campylobacteriosis in Luxembourg, 2010-2013: a case-control study combined with Multilocus Sequence Typing for source attribution and risk factor analysis. Sci. Rep. 6:20939. doi: 10.1038/srep20939
Mourkas, E., Florez-Cuadrado, D., Pascoe, B., Calland, J. K., Bayliss, S. C., Mageiros, L., et al. (2019). Gene pool transmission of multidrug resistance among Campylobacter from livestock, sewage and human disease. Environ. Microbiol. 21, 4597–4613. doi: 10.1111/1462-2920.14760
Mourkas, E., Taylor, A. J., Méric, G., Bayliss, S. C., Pascoe, B., Mageiros, L., et al. (2020). Agricultural intensification and the evolution of host specialism in the enteric pathogen Campylobacter jejuni. Proc. Natl. Acad. Sci. U.S.A. 117, 11018–11028. doi: 10.1073/pnas.1917168117
Nachamkin, I., Ung, H., and Li, M. (2002). Increasing fluoroquinolone resistance in Campylobacter jejuni, Pennsylvania, USA,1982–2001. Emerg. Infect. Dis. 8, 1501–1503. doi: 10.3201/eid0812.020115
Newell, D. G., Mughini-Gras, L., Kalupahana, R. S., and Wagenaar, J. A. (2017). “Campylobacter epidemiology - Sources and routes of transmission for human infection,” in Campylobacter: Features. Detection, and Prevention on Foodborne Disease, ed. G. Klein (Amsterdam: Elsevier), 85–110. doi: 10.1016/B978-0-12-803623-5.00005-8
Nguyen, T. N., Hotzel, H., El-Adawy, H., Tran, H. T., Le, M. T., Tomaso, H., et al. (2016). Genotyping and antibiotic resistance of thermophilic Campylobacter isolated from chicken and pig meat in Vietnam. Gut Pathog. 8:19. doi: 10.1186/s13099-016-0100-x
Painset, A., Day, M., Doumith, M., Rigby, J., Jenkins, C., Grant, K., et al. (2020). Comparison of phenotypic and WGS-derived antimicrobial resistance profiles of Campylobacter jejuni and Campylobacter coli isolated from cases of diarrhoeal disease in England and Wales, 2015-16. J. Antimicrob. Chemother. 75, 883–889. doi: 10.1093/jac/dkz539
Pascoe, B., Schiaffino, F., Murray, S., Méric, G., Bayliss, S. C., Hitchings, M. D., et al. (2020). Genomic epidemiology of Campylobacter jejuni associated with asymptomatic pediatric infection in the Peruvian Amazon. PLoS Negl. Trop. Dis. 14:e0008533. doi: 10.1371/journal.pntd.0008533
Pollett, S., Rocha, C., Zerpa, R., Patiño, L., Valencia, A., Camiña, M., et al. (2012). Campylobacter antimicrobial resistance in Peru: a ten-year observational study. BMC Infect. Dis. 12:193. doi: 10.1186/1471-2334-12-193
Price, L. B., Johnson, E., Vailes, R., and Silbergeld, E. (2005). Fluoroquinolone-resistant Campylobacter isolates from conventional and antibiotic-free chicken products. Environ. Health Perspect. 113, 557–560. doi: 10.1289/ehp.7647
Ramonaite, S., Tamuleviciene, E., Alter, T., Kasnauskyte, N., and Malakauskas, M. (2017). MLST genotypes of Campylobacter jejuni isolated from broiler products, dairy cattle and human campylobacteriosis cases in Lithuania. BMC Infect. Dis. 17:430. doi: 10.1186/s12879-017-2535-1
R Core Team (2019). R: A Language and Environment for Statistical Computing. Vienna: R Foundation for Statistical Computing.
Revez, J., Rossi, M., Ellström, P., de Haan, C., Rautelin, H., and Hänninen, M.-L. (2011). Finnish Campylobacter jejuni strains of multilocus sequence type ST-22 complex have two lineages with different characteristics. PLoS One 6:e26880. doi: 10.1371/journal.pone.0026880
Rivera-Mendoza, D., Martínez-Flores, I., Santamaría, R. I., Lozano, L., Bustamante, V. H., and Pérez-Morales, D. (2020). Genomic analysis reveals the genetic determinants associated with antibiotic resistance in the zoonotic pathogen Campylobacter spp. distributed Globally. Front. Microbiol. 11:513070. doi: 10.3389/fmicb.2020.513070
Rokney, A., Valinsky, L., Moran-Gilad, J., Vranckx, K., Agmon, V., and Weinberger, M. (2018). Genomic epidemiology of Campylobacter jejuni transmission in Israel. Front. Microbiol. 9:2432. doi: 10.3389/fmicb.2018.02432
Scallan, E., Hoekstra, R. M., Angulo, F. J., Tauxe, R. V., Widdowson, M. A., Roy, S. L., et al. (2011). Foodborne illness acquired in the United States – major pathogens. Emerg. Infect. Dis. 17, 7–15. doi: 10.3201/eid1701.P11101
Seemann, T. mlst. Github. Available online at: https://github.com/tseemann/mlst
Sheppard, S. K., Cheng, L., Méric, G., De Haan, C. P. A., Llarena, A.-K., Marttinen, P., et al. (2014). Cryptic ecology among host generalist Campylobacter jejuni in domestic animals. Mol. Ecol. 23, 2442–2451. doi: 10.1111/mec.12742
Silva, J., Leite, D., Fernandes, M., Mena, C., Gibbs, P. A., and Teixeira, P. (2011). Campylobacter spp. as a foodborne pathogen: a review. Front. Microbiol. 2:200. doi: 10.3389/fmicb.2011.00200
phac-nml/staramr Staramr. Available online at: https://github.com/phac-nml/staramr
Thépault, A., Méric, G., Rivoal, K., Pascoe, B., Mageiros, L., Touzain, F., et al. (2017). Genome-wide identification of host-segregating epidemiological markers for source attribution in Campylobacter jejuni. Appl. Environ. Microbiol. 83, e03085-16. doi: 10.1128/AEM.03085-16
Van Boeckel, T. P., Brower, C., Gilbert, M., Grenfell, B. T., Levin, S. A., Robinson, T. P., et al. (2015). Global trends in antimicrobial use in food animals. Proc. Natl. Acad. Sci. U.S.A. 112, 5649–5654. doi: 10.1073/pnas.1503141112
Wang, Y., Dong, Y., Deng, F., Liu, D., Yao, H., Zhang, Q., et al. (2016). Species shift and multidrug resistance of Campylobacter from chicken and swine, China, 2008–14. J. Antimicrob. Chemother. 71, 666–669. doi: 10.1093/jac/dkv382
Wieczorek, K., and Osek, J. (2013). Antimicrobial resistance mechanisms among Campylobacter. Biomed. Res. Int. 2013:340605. doi: 10.1155/2013/340605
WHO (2017). WHO Publishes List of Bacteria for Which New Antibiotics are Urgently Needed. Geneva: WHO Media Centre.
Wysok, B., Wojtacka, J., Hänninen, M.-L., and Kivistö, R. (2020). Antimicrobial resistance and virulence-associated markers in Campylobacter strains from diarrheic and non-diarrheic humans in Poland. Front. Microbiol. 11:1799. doi: 10.3389/fmicb.2020.01799
Yahara, K., Méric, G., Taylor, A. J., de Vries, S. P. W., Murray, S., Pascoe, B., et al. (2017). Genome-wide association of functional traits linked with Campylobacter jejuni survival from farm to fork. Environ. Microbiol. 19, 361–380. doi: 10.1111/1462-2920.13628
Zankari, E., Hasman, H., Cosentino, S., Vestergaard, M., Rasmussen, S., Lund, O., et al. (2012). Identification of acquired antimicrobial resistance genes. J. Antimicrob. Chemother. 67, 2640–2644. doi: 10.1093/jac/dks261
Zankari, E., Allesøe, R., Joensen, K. G., Cavaco, L. M., Lund, O., and Aarestrup, F. (2017). PointFinder: a novel web tool for WGS-based detection of antimicrobial resistance associated with chromosomal point mutations in bacterial pathogens. J. Antimicrob. Chemother. 72, 2764–2768. doi: 10.1093/jac/dkx217
Zhao, S., Mukherjee, S., Chen, Y., Li, C., Young, S., Warren, M., et al. (2015). Novel gentamicin resistance genes in Campylobacter isolated from humans and retail meats in the USA. J. Antimicrob. Chemother. 70, 1314–1321. doi: 10.1093/jac/dkv001
Zhao, S., Tyson, G. H., Chen, Y., Li, C., Mukherjee, S., Young, S., et al. (2016). Whole-genome sequencing analysis accurately predicts antimicrobial resistance phenotypes in Campylobacter spp. Appl. Environ. Microbiol. 82, 459–466. doi: 10.1128/AEM.02873-15
Keywords: Campylobacter jejuni, resistome, whole genome sequencing, host specialization, livestock and human sources, Campylobacter coli
Citation: Cobo-Díaz JF, González del Río P and Álvarez-Ordóñez A (2021) Whole Resistome Analysis in Campylobacter jejuni and C. coli Genomes Available in Public Repositories. Front. Microbiol. 12:662144. doi: 10.3389/fmicb.2021.662144
Received: 31 January 2021; Accepted: 19 April 2021;
Published: 05 July 2021.
Edited by:
Aloysius Wong, Kean University-Wenzhou, ChinaReviewed by:
Mohamed Elhadidy, Helmy Institute for Medical Sciences, University of Science and Technology at Zewail City, EgyptBen Pascoe, University of Bath, United Kingdom
Copyright © 2021 Cobo-Díaz, González del Río and Álvarez-Ordóñez. This is an open-access article distributed under the terms of the Creative Commons Attribution License (CC BY). The use, distribution or reproduction in other forums is permitted, provided the original author(s) and the copyright owner(s) are credited and that the original publication in this journal is cited, in accordance with accepted academic practice. No use, distribution or reproduction is permitted which does not comply with these terms.
*Correspondence: José F. Cobo-Díaz, jcobd@unileon.es