- School of Modern Agriculture and Biotechnology, Ankang University, Ankang, China
Bacterial soft rot is a destructive disease that restricts the development of the konjac (Amorphophallus konjac K. Koch ex N.E.Br) industry. The objective of this study was to investigate how soft rot disease affects bacterial communities associated with the roots of konjac plants growing under a pure Robinia pseudoacacia plantation. Three sampling sites affected by different degrees of soft rot damage were selected based on the disease incidence [0%, non-diseased (ND); 4.2%, moderately diseased (MD); and 18.6%, highly diseased (HD)]. The variation in soil and root bacterial diversity and community composition among the sampling sites was determined by Illumina HiSeq sequencing of the V3–V4 hypervariable regions of the bacterial 16S rRNA gene. The results showed that the contents of soil organic matter and available nutrients (N, P, and K) increased with increasing damage degree, whereas higher damage degree resulted in lower soil pH and enzymatic activity (sucrase, urease, catalase, and polyphenol oxidase). The composition of root-associated bacterial communities differed among the three sampling sites. Proteobacteria was the most dominant bacterial phylum in all soil and root samples. Pseudomonas, Bacillus, Rhizobium, and Streptomyces were the most abundant in all samples from the ND sites, whereas Pectobacterium carotovorum and Serratia were predominant in the samples from the MD and HD sites. The abundance and alpha diversity of root-associated bacteria were significantly higher (p < 0.05) in the ND sites than in the diseased sites. The results suggested pronounced differences in the abundance, alpha diversity, and community composition of bacteria associated with the roots of konjac plants affected by different degrees of soft rot damage. Such differences in bacterial community structure were related to dynamic changes in soil variables, especially soil available potassium content, sucrase activity, and urease activity. Analysis of the dominant root-associated bacterial taxa offers an approach to predict the damage degree due to soft rot in konjac and provides evidence for the prevention of this soil-borne disease via microecological regulation.
Introduction
Konjac (Amorphophallus konjac K. Koch ex N.E.Br) is a perennial, herbaceous species extensively planted in Southeast Asia and Africa (Vasques et al., 2008). The corm of konjac is rich in glucomannan, a neutral polysaccharide with excellent biocompatibility and biodegradability. Konjac glucomannan has been widely used in food, medicine, agriculture, and chemical engineering (Li et al., 2010). China is a major producer of konjac and has the world’s largest konjac production capacity (Wu et al., 2010; Qiu, 2013). Langao (Shaanxi Province) is a national key county of konjac industry in China (Song and Liu, 2018). The planting area of konjac in Langao reached 62.67 km2 in 2019, accounting for approximately one-sixth of the total planting area of konjac in Shaanxi Province (The State Council of the People’s Republic of China, 2019).
To make full use of limited land resources, konjac intercropping is applied in various plantations, such as Robinia pseudoacacia, Juglans regia, Toxicodendron vernicifluum, and Hevea brasiliensis. Among them, the yield of konjac under R. pseudoacacia plantations is the highest, with an average increase of 71.9% compared with the konjac yield in corn fields (He et al., 2015c). So far, the practice of planting konjac under R. pseudoacacia plantations has been widely adopted throughout China, including Zhen’an County in Shaaxi Province (He et al., 2014) and other regions in Yunnan Province (Zhang, 2017). Despite the yield increase in intercropping, development of the konjac industry in China is still negatively affected by soft rot, a devastating soil-borne plant disease caused by Pectobacterium spp. (Wu et al., 2012), Dicyeya spp. (Czajkowski et al., 2014; Wu et al., 2016), and Enterobacter spp. (Wu et al., 2011b).
Outbreaks of konjac soft rot disease have caused irreparable damage to the agroecosystem with serious economic losses and ecological destruction, including the added costs of labor management and disease control (Wu et al., 2011a). Therefore, many studies have been dedicated to identifying the pathogens of konjac soft rot and developing new control methods (Wu et al., 2011b, 2012, 2015; Chen, 2013). Biological control, for instance, using Paenibacillus polymyxa and Pseudomonas fluorescens, is considered to be a promising method for the control of konjac soft rot (Helmy et al., 2008; Chen, 2013). However, the effects of available biological agents or bioorganic fertilizers are unstable in practice due to the influence of abiotic and biotic factors. Particularly, the microecosystem formed by microbes in the roots and root-associated soil of konjac is a vital factor affecting the incidence of soft rot and regulating the efficacy of biological control (He and Cui, 2017).
The microbial diversity and community composition in the root zone of a crop is indicative of the present situation and development tendency of the soil ecosystem, which is crucial for crop health (He et al., 2015c). It has been found that agricultural practices, including crop variety selection (Guan et al., 2016), fertilizer application (Su et al., 2014), continuous cropping (Liu et al., 2019), interplanting, and rotation (Zhuang et al., 2009), can cause variation in soil microbial diversity, microbial abundance, and enzymatic activity. Moreover, a previous study using culture-dependent technique has shown that the community composition of root-associated bacteria and fungi differs between konjac growing fields with and without soft rot (He et al., 2018). However, little information is available on the response of soil and root bacterial communities to different degrees of soft rot damage in konjac. Additionally, the relationships between root-associated bacteria and soil variables in konjac growing sites affected by different degrees of soft rot damage remain poorly understood. Detangling these relationships is essential to identify the key factors influencing the bacterial community structure in the root zone of konjac.
In the present study, we hypothesized that different degrees of soft rot damage in konjac could affect the abundance, alpha diversity, and community composition of root-associated bacteria through altering soil variables. To verify the hypothesis, we compared soil physicochemical properties and enzymatic activity, as well as soil and root bacterial community characteristics, among three konjac growing sites affected by soft rot disease to different degrees. The results could provide supportive evidence for the prevention and control of konjac soft rot via microecological regulation.
Materials and Methods
Study Area
This study was conducted on konjac plants growing under a pure black locust (R. pseudoacacia) plantation located in Lishuya, a village in Langao County, Shaanxi Province, Northwest China. Langao (32°N and 108°E) is situated in the northern subtropical continental monsoon climate zone, with a mean altitude of 1,486 m and a high forest coverage of 78.6%. The mean annual temperature is 16°C, and the frost-free period lasts 242 days. The mean annual dryness index and precipitation are 0.37 and 1,033 mm, respectively. Precipitation is highly seasonal, with ∼80% of the annual precipitation occurring from May to October and a dry season of 6 months. The major soil type in the study area is brown clay (FAO-UNESCO, 1988).
Experimental Design and Sample Collection
Three sampling sites were chosen in the study area based on the incidence and disease index of konjac soft rot (Supplementary Figure 1). One site was not infected with soft rot [non-diseased (ND)], while two sites were affected by soft rot to different degrees [moderately diseased (MD) and highly diseased (HD)]. All the sampling sites had been continuously planted with the same cultivar konjac (A. konjac K. Koch ex N.E.Br) for 2 years, and the current konjac plants were 7 months old. The canopy closure of R. pseudoacacia in each site was evaluated by an experienced forestry expert using a simple visual assessment technique (Jennings et al., 1999). Other information on the sampling sites was provided by the Bureau of Konjac Langao (Shaanxi, China).
In August 2017, three sampling plots (20 m × 20 m) were established at random in each of the ND, MD, and HD sites (NDS, MDS, and HDS). The MD plants were identified by maceration of corms, and the leaves were yellow on one side of the plants. The HD plants were identified by maceration and rotting of parenchymatous tissue in aboveground organs (stems and leaves) in addition to underground organs, which led to a total collapse of the aboveground parts of the plants (Wu et al., 2010). The incidence and disease index of konjac soft rot were calculated according to the method of Duan et al. (2018).
In each sampling plot, three 7-month-old konjac plants (70–90 cm tall) were selected at random for collecting soil and root samples simultaneously. After removal of leaf litter and stones on the soil surface (0- to 5-cm depth), root-associated soil samples (each ∼500 g) within 2 cm of the roots of selected konjac plants were collected by pulling the plant from the ground and shaking the roots gently (April and Keller, 1990). After that, fresh root samples of the same three konjac plants were obtained together with soil from the 5- to 30-cm depth. In total, three soil samples and three root samples per plot were collected separately. Subsamples were combined equally to give one composite sample per plot. The soil samples of the same three konjac plants were passed through a 2-mm mesh size sieve and then divided into two portions. One portion was stored at −80°C for DNA extraction, and the other portion was air-dried and stored at 25°C in resealable plastic bags until analysis for soil physicochemical properties and enzymatic activity. The root samples were washed five times with sterile water and then stored at −80°C prior to DNA extraction.
Soil Physicochemical Analysis and Enzymatic Activity Assay
Soil pH was measured in 1:5 (w/v) soil–water suspensions (Bao, 2000) using a PHS-3C digital pH meter (Lida Instrument Factory, Shanghai, China). Soil organic matter (OM) was determined by oxidization with 0.4 M of potassium dichromate–concentrated sulfuric acid solution (Nelson and Sommers, 1982). The alkaline hydrolysis diffusion method was used to determine soil available nitrogen (AN) content (Song et al., 2018). Soil available phosphorus (AP) was extracted with 0.5 M of NaHCO3 for 20 min and quantified by molybdenum-antimony anti-spectrophotometry (Page, 1982). Soil available potassium (AK) was extracted with 1.0 M of ammonium acetate for 30 min and determined by flame photometry (Oyedele et al., 2008).
Soil sucrase activity was assayed using the 3,5-dinitrosalicylic acid colorimetric method and calculated on the basis of the amount of reducing sugar liberated (Guan et al., 1986). Soil urease activity was assayed using the indophenol blue colorimetric method, which involved an incubation step with 5% urea (substrate) in borate buffer solution (pH 10) for 24 h, subsequent extraction, and filtration with 2 M of KCl (Kandeler and Gerber, 1988). Soil catalase activity was assayed by titration with 0.1 M of potassium permanganate (Stepniewska et al., 2009). Polyphenol oxidase activity was analyzed using the catechol colorimetric method with 0.5% catechol as a substrate (Adamczyk et al., 2009).
DNA Extraction and Illumina HiSeq Sequencing
Soil and root samples were homogenized in liquid nitrogen. Total genomic DNA was extracted from 500 mg of soil samples using the E.Z.N.A.® soil DNA kit (Omega Bio-Tek, Doraville, GA, United States) following the manufacturer’s instructions. Total DNA extraction from 80 mg of root samples was performed using a plant DNA extraction kit (Tiangen Biotech, Beijing, China) according to the manufacturer’s protocol. A total of 18 samples, consisting of nine root samples and nine soil samples (three biological replicates with three technical replicates for each treatment), were subjected to total genomic DNA extraction. DNA concentration and purity were determined using a SmartspecTM Plus spectrophotometer (Bio-Rad, Hercules, CA, United States), and the quality was monitored using 0.8% agarose gel electrophoresis.
The DNA extract was diluted to 1 ng⋅μl–1 and used as PCR template. The universal primers 319F (5′-ACTCCTACGGGAGGCAGCAG-3′) and 806R (5′-GGACTACHVGGGTWTCTAAT-3′) were used to amplify the V3–V4 hypervariable regions of the bacterial 16S rRNA gene (Zhou et al., 2011), which covered over 97% of the 16S gene sequences in the ribosomal database. Each primer included a linker sequence required for Illumina HiSeq 300-bp paired-end sequencing and a 12-bp heterogeneity spacer index sequence intended to reduce biases associated with low-diversity amplicon sequencing (Chopyk et al., 2017).
DNA samples were PCR-amplified in triplicate. The PCR mixture (25 μl) contained 13 μl of 2 × Taq Master Mix (CoWin Biotech, Beijing, China), 0.5 μl of each primer (10 μM; Invitrogen, Carlsbad, CA, United States), 1 μl of DNA template, and 10 μl of RNase-free water. The following thermal conditions were used: pre-denaturation at 95°C for 3 min; 37 cycles of degeneration at 95°C for 5 s, annealing at 58°C for 30 s, and extension at 72°C for 45 s; and a final extension step at 72°C for 10 min. Gel electrophoresis was performed to confirm successful amplification. Next, PCR products from the triplicate reactions were extracted from a 0.8% agarose gel and further purified using a universal DNA purification kit (Tiangen Biotech). The pellet of combined DNA was submitted to Beijing Biomics Biotechnology Institute (Beijing, China) for paired-end sequencing on the Illumina HiSeq 2500 Platform (Illumina Inc., San Diego, CA, United States).
Sequence Data Processing and Analysis
Raw tags generated by Illumina sequencing were transformed into clean tags using FLASH v1.2.7 (Magoč and Salzberg, 2011), Trimmomatic v0.33 (Wang et al., 2012), and UCHIME v4.2 (Edgar et al., 2011). The clean sequences were clustered into operational taxonomic units (OTUs) based on a 97% similarity cutoff using Usearch v7.1 in the QIIME pipeline (v1.8.0; http://qiime.org/). Representative sequences for each bacterial OTU were chosen for alignment and taxonomic classification using the Ribosomal Database Project classifier (Release 11.5; http://rdp.cme.msu.edu/). Rarefaction curve and alpha diversity indices were analyzed based on the OTU table by random sampling to the minimum number of sequences in the samples. The alpha diversity of bacteria in each sample was evaluated in terms of species richness (Chao1 and ACE) and diversity (Shannon and Simpson indices), and the index values were calculated using QIIME v1.8.0 (Caporaso et al., 2010). All raw sequences were submitted to the Sequence Read Archive of the National Center for Biotechnology Information database under accession numbers SRR11490401–SRR11490418.
Statistical Analysis
Significant differences between treatments were analyzed through a one-way analysis of variance (ANOVA) followed by least significant difference (LSD) tests (p < 0.05) using SPSS Statistics v22.0 (SPSS Inc., Chicago, IL, United States). Venn diagrams were created to visualize common and unique OTUs between samples (http://bioinformatics.psb.ugent.be/webtools/Venn/; Chen and Boutros, 2011). A heatmap of dominant bacterial genera (relative abundance > 1% of total sequences) was constructed using the package ‘‘pheatmap’’ in R v3.2.21. Principal component analysis (PCA) was conducted based on the relative abundance of dominant and pathogenic bacterial genera from the three sampling sites using Canoco v5.0 (Centre for Biometry, Wageningen, Netherlands). Redundancy analysis (RDA) was used to estimate the multivariate correlations between bacterial community structure and soil variables associated with different degrees of konjac soft rot using Canoco v5.0.
Results
General Characteristics of Konjac Growing Sites
The three sampling sites had distinctly different incidences and disease index of konjac soft rot: 0 and 0 for ND, 4.2% and 1.4 for MD, 18.6% and 18.6 for HD. The three sites were located at similar altitudes (963–1,043 m) and slopes (29°–32°). All the sites were derived from the same original vegetation (R. pseudoacacia), with the canopy closure of 57–60%, average plant height of 81.8–85.5 cm, and planting density of 5.56–6.25/m2 (Table 1).
Soil Physicochemical Properties and Enzymatic Activity
Soil pH decreased concomitantly with increasing degree of soft rot damage, and the lowest soil pH was observed in site HD (6.40). In contrast, soil OM, AN, AP, and AK contents all showed the opposite trend (Table 2) and reached the highest levels in site HD (28.38 g⋅kg–1, 127.36 mg⋅kg–1, 8.27 mg⋅kg–1, and 110.53 mg⋅kg–1, respectively). However, there were no significant differences in soil AN and AP contents between sites ND and MD (p > 0.05).

Table 2. Soil physicochemical properties in the three konjac growing sites affected by different levels of soft rot disease.
Among the three sampling sites, MD showed the highest soil sucrase activity (5.62 mg⋅g–1⋅day–1), whereas ND exhibited the highest catalase activity (1.43 μmol⋅g–1⋅day–1); however, catalase activity did not differ significantly between the MD and HD sites (Table 3). Soil urease and polyphenol oxidase activity gradually decreased with increasing degree of soft rot damage; their activity levels in the HD site were 58.4 and 63.1% lower, respectively, than those in the ND site (p < 0.05).

Table 3. Soil enzymatic activity in the three konjac growing sites affected by different levels of soft rot disease.
Bacterial Taxonomic Distribution
Sequencing Data
The rarefaction curves were used to indicate the volume of the sequencing data and the bacterial species richness for all the 18 samples (Song et al., 2018). The curves tended to flatten out (Figure 1), suggesting that the sequencing data can indeed represent the bacterial community in konjac soils and roots and signify the bacterial community diversity.
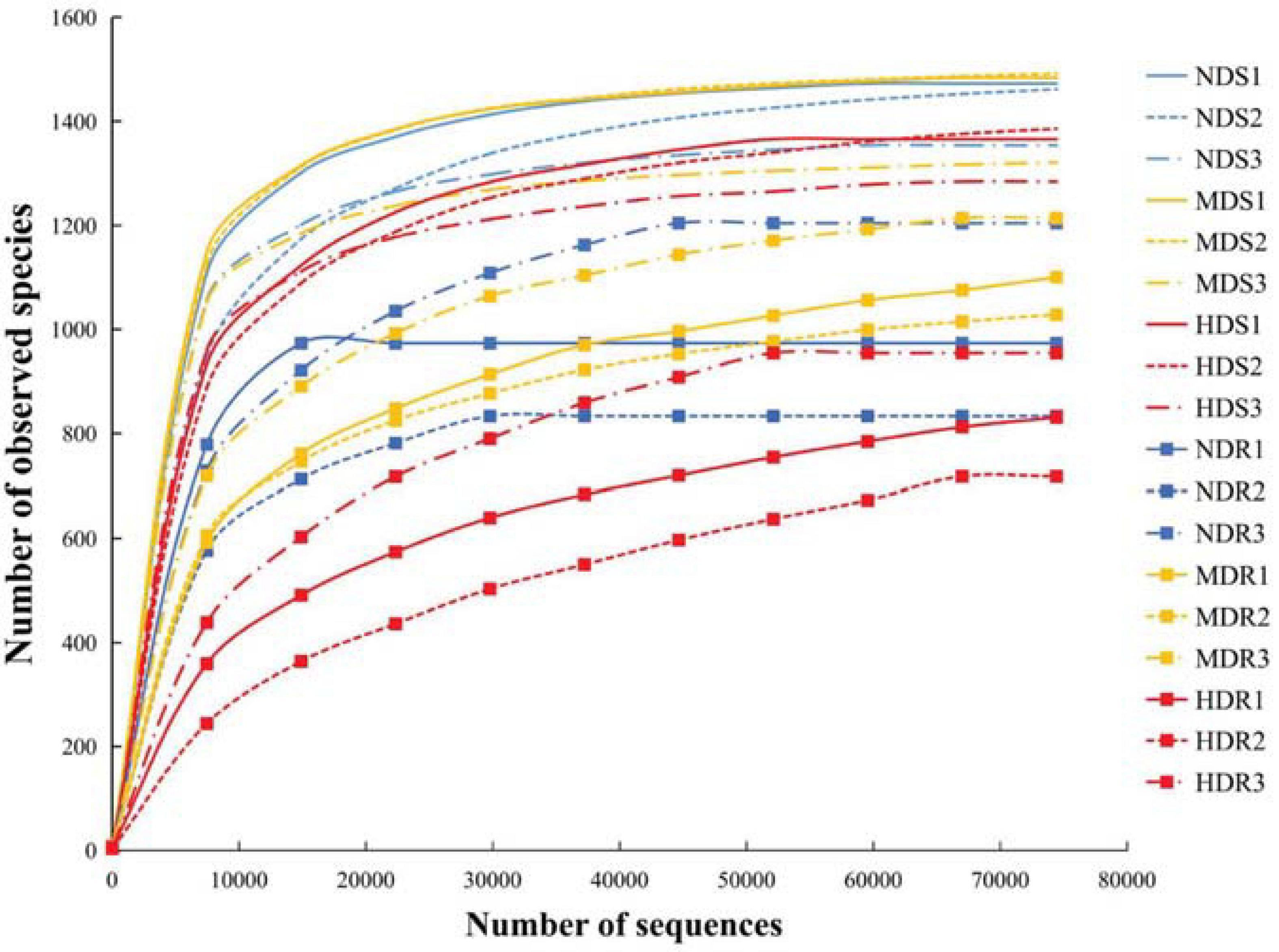
Figure 1. Rarefaction curves showing the relationships between the number of sequences and the number of observed species identified in the soil and root samples of konjac from the non-diseased (NDS and NDR), moderately diseased (MDS and MDR), and highly diseased (HDS and HDR) sites.
A total of 1,314,791 and 1,393,944 raw reads were collected from the nine soil samples and nine root samples, respectively; after paired-end assembly, 1,228,232 and 1,303,840 clean reads were obtained, respectively. In total, 987,130 and 982,774 bacterial sequences representing 12,676 and 9,235 OTUs were identified, respectively, from soil and root samples. In the soil samples from the three sampling sites (NDS, MDS, and HDS), 1,536, 1,539, and 1,503 OTUs were identified, including five, two, and one unique OTUs, respectively, with 1,490 common OTUs; the number of common OTUs was the highest (1,528) between the NDS and MDS samples. In the root samples from the three sampling sites (NDR, MDR, and HDR), fewer OTUs were identified: 1,352, 1,344, and 1,190 OTUs, respectively, with 1,057 common OTUs (Figure 2).
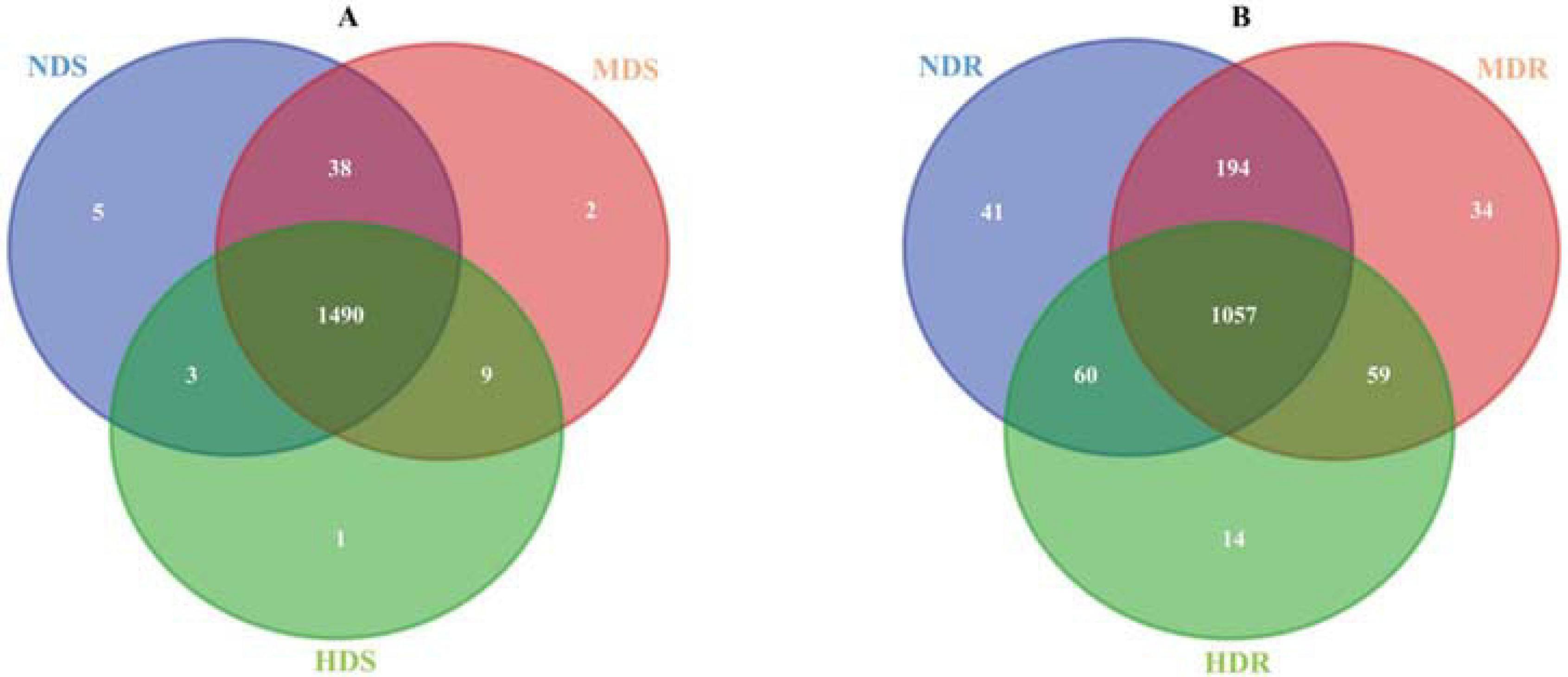
Figure 2. Venn diagram showing the common and unique bacterial operational taxonomic units in soil (A) and root (B) samples collected from the non-diseased (NDS and NDR), moderately diseased (MDS and MDR), and highly diseased (HDS and HDR) sites.
Bacterial Alpha Diversity
The soil and root samples both showed a similar tendency in the bacterial alpha diversity among the three sampling sites. One-way ANOVA showed that the bacterial species richness (ACE and Chao1) was significantly higher in the ND and MD sites than in the HD site (p < 0.05; Table 4). Similarly, the Shannon index suggested that the bacterial diversity decreased with increasing degree of soft rot damage, but the Simpson index presented an opposite trend. Generally, site ND had the highest bacterial species richness, whereas site HD had the lowest. Furthermore, site ND was more similar to site MD in terms of bacterial alpha diversity. Nonetheless, the Simpson index values of the soil and root samples from sites MD and HD were markedly higher than those of the samples from site ND (p < 0.05).
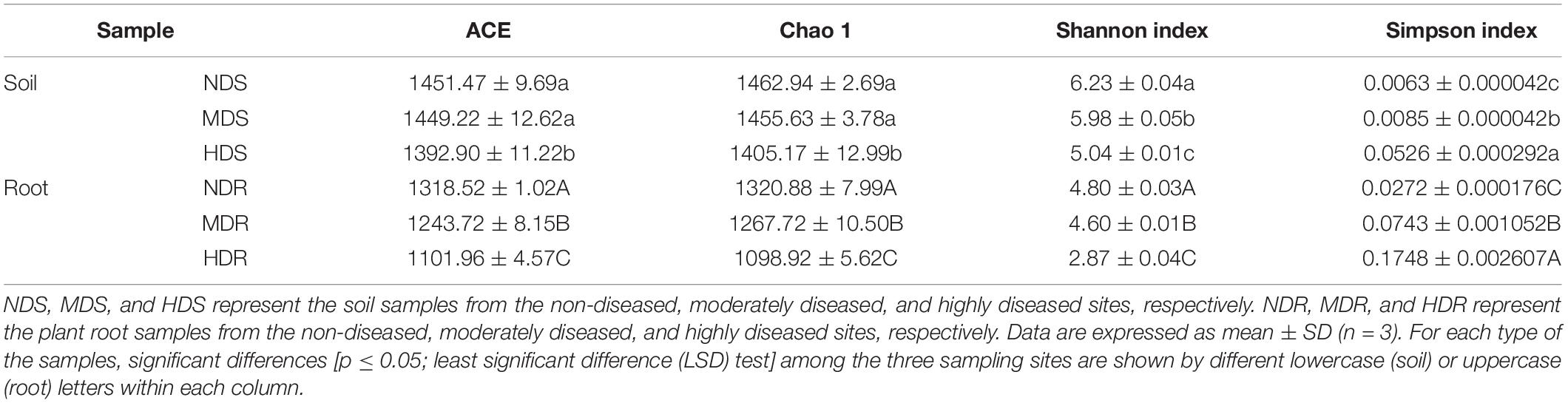
Table 4. Alpha diversity indices of soil and root bacterial communities in different konjac growing sites affected by different degrees of soft rot disease.
Bacterial Community Composition
The sequences obtained from the soil and root samples were classified into nine bacterial phyla (Figure 3A and Supplementary Table 1). In the soil samples, the most dominant bacterial phyla (relative abundance > 1%) included Proteobacteria (38.8% of all sequences), Firmicutes (16.8%), Actinobacteria (14.7%), Bacteroidetes (12.8%), Acidobacteria (7.6%), Gemmatimonadetes (3.6%), Chloroflexi (2.1%), Nitrospirae (1.9%), and Verrucomicrobia (1.2%). Among them, the relative abundance of Actinobacteria increased with increasing degree of soft rot damage, while the relative abundance of Bacteroidetes and Verrucomicrobia exhibited an opposite trend. Particularly, the abundance of Firmicutes, Acidobacteria, Chloroflexi, and Gemmatimonadetes was significantly higher (by 38.2, 12.1, 5.4, and 5.2%, respectively) in the HDS compared with NDS samples (p < 0.05). In contrast, Proteobacteria and Verrucomicrobia were less abundant (by 11.9% and 31.1%, respectively) in the HDS samples than in the NDS samples (p < 0.05). In the root samples, Proteobacteria (77.6%), Actinobacteria (13.6%), Bacteroidetes (3.8%), and Firmicutes (2.7%) were the dominant bacterial phyla. Specifically, the relative abundance of Proteobacteria increased with increasing damage degree, while the relative abundance of Acidobacteria, Actinobacteria, Gemmatimonadetes, Nitrospirae, and Verrucomicrobia showed an inverse trend. Bacteroidetes, Chloroflexi, and Firmicutes were less abundant (by 75.3, 66.0, and 84.7%, respectively) in the HDR than in NDR samples (p < 0.05).
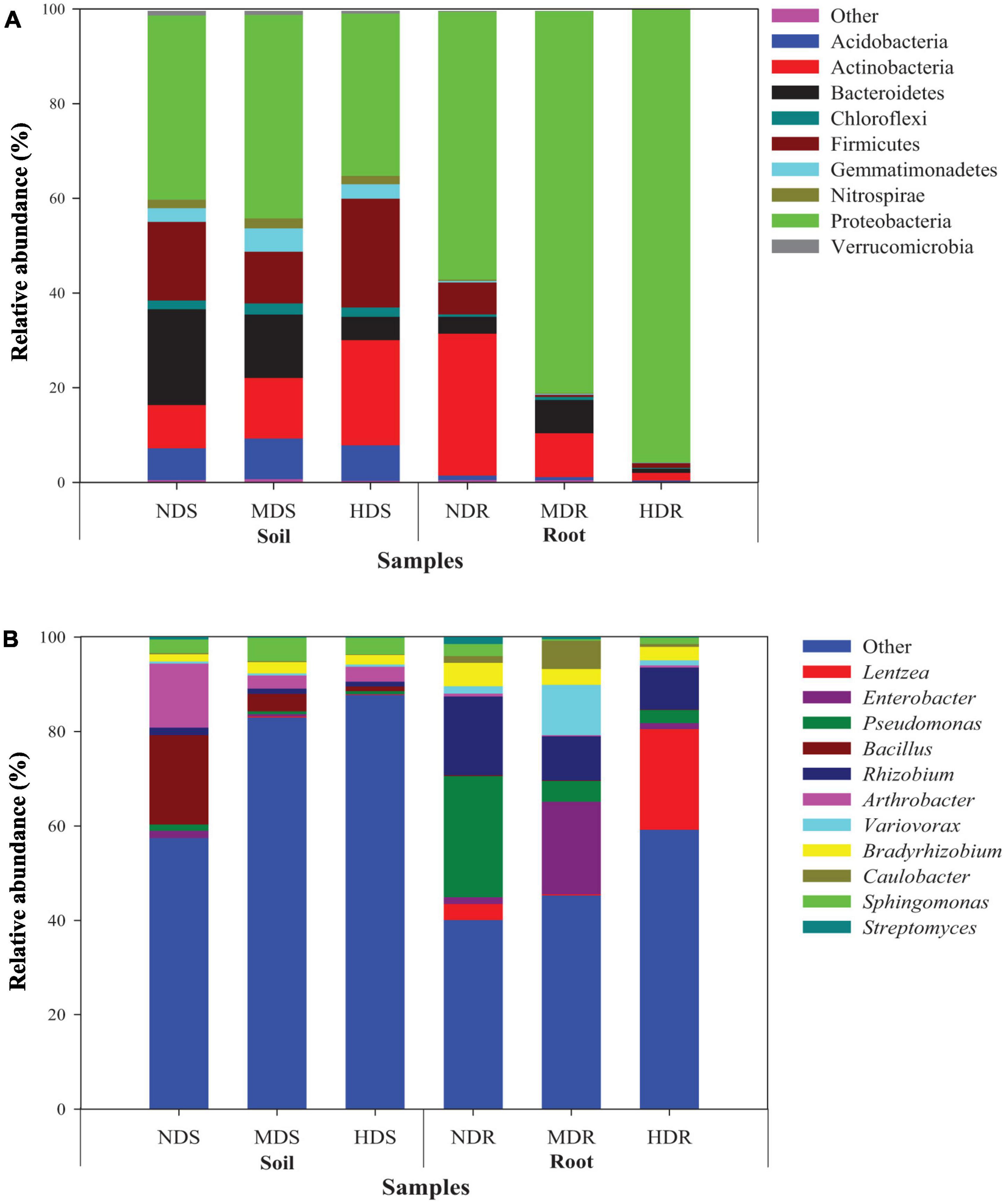
Figure 3. Relative abundance of dominant bacterial taxa (>1% of total sequences) in different soil and root samples. (A) Nine phyla and (B) 11 genera. NDS, MDS, and HDS represent the soil samples from the non-diseased, moderately diseased, and highly diseased sites, respectively. NDR, MDR, and HDR represent the plant root samples from the non-diseased, moderately diseased, and highly diseased sites, respectively. Data are expressed as mean ± SD (n = 3). For each type of samples, different lowercase (soil) or uppercase (root) letters within each group indicate significant differences among the three sampling sites [p ≤ 0.05; least significant difference (LSD) test].
At the genus level (Figure 3B and Supplementary Table 2), the relatively abundant OTUs were distributed into 11 different taxa. In the soil samples, the dominant genera (relative abundance > 1%) were Bacillus (7.9%), Arthrobacter (6.5%), Sphingomonas (3.8%), Bradyrhizobium (2.0%), and Rhizobium (1.2%). The relative abundance of Enterobacter, Pseudomonas, Bacillus, Rhizobium, Arthrobacter, Caulobacter, and Streptomyces was significantly higher in the NDS samples than in the MDS or HDS samples. However, Bradyrhizobium (2.4%) and Sphingomonas (4.9%) showed the highest relative abundance in the MDS samples. In the root samples, Rhizobium (11.6%), Pseudomonas (10.9%), Lentzea (8.3%), Enterobacter (7.4%), Variovorax (4.4%), Bradyrhizobium (3.7%), Caulobacter (2.7%), and Sphingomonas (1.4%) were predominant. The relative abundance of Pseudomonas, Bacillus, Rhizobium, Bradyrhizobium, and Streptomyces in the root samples decreased with increasing degree of soft rot damage and showed the lowest values in the HDR samples (2.74, 0.11, 8.90, 2.87, and 0.11%, respectively). Compared with those in the NDR samples, Lentzea was 6.3-fold more abundant in the HDR samples, whereas Enterobacter, Variovorax, and Caulobacter were 13.6-, 6.8-, and 4.1-fold more abundant in the MDR samples, respectively. Moreover, the relative abundance of Arthrobacter and Sphingomonas in the HDR samples was 24.6% and 48.6% lower than in the NDR samples (p < 0.05).
As expected, bacterial sequences of soft rot pathogens were identified in both soil and root samples (Figures 4A,B). The relative abundance of Pectobacterium carotovorum and Serratia increased with increasing damage degree and reached the highest values in the HD site (HDS: 1.5 and 0.16%, respectively; HDR: 1.0 and 0.01%, respectively).
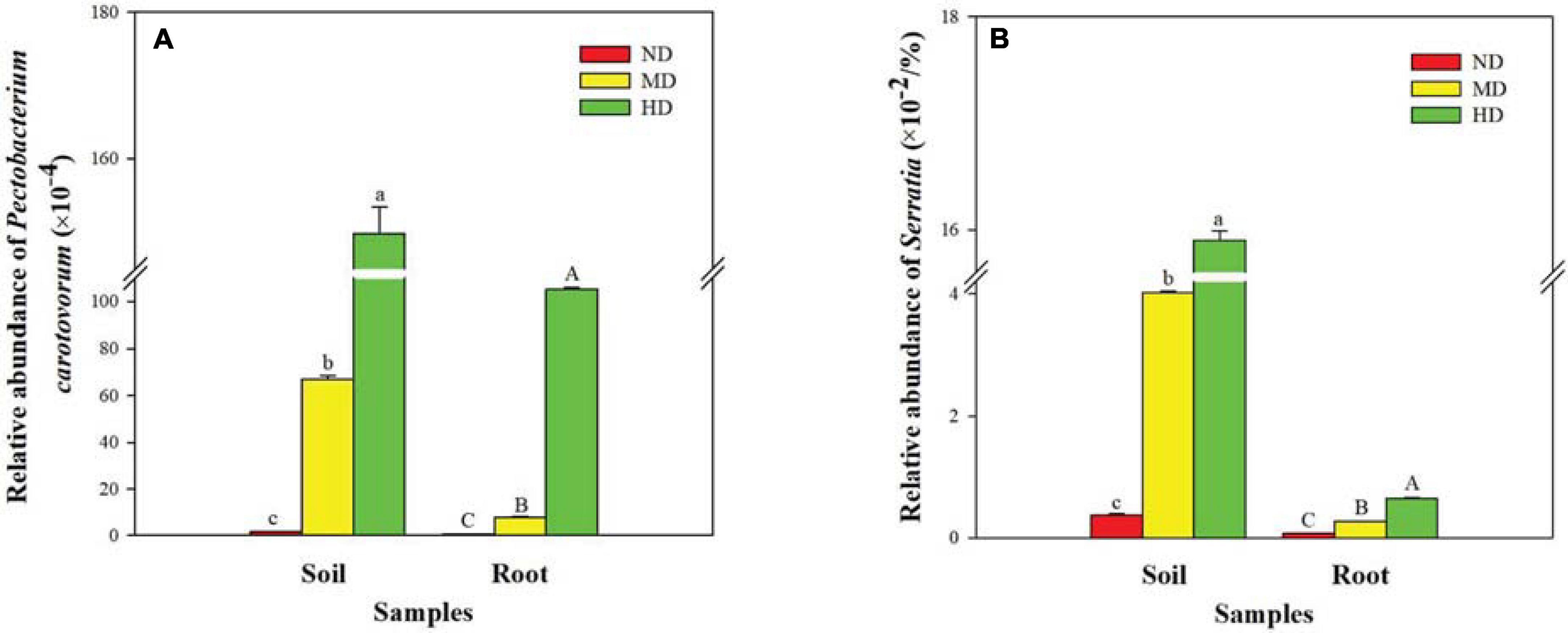
Figure 4. Relative abundance of soft rot pathogens in different soil and root samples. (A) Pectobacterium carotovorum and (B) Serratia. ND, MD, and HD represent the non-diseased, moderately diseased, and highly diseased sites, respectively. Data are expressed as mean ± SD (n = 3). For each type of samples, different lowercase (soil) or uppercase (root) letters within each group indicate significant differences among the three sampling sites [p ≤ 0.05; least significant difference (LSD) test].
Factors Associated With Bacterial Community Structure
The PCA plot based on the relative abundance of the 11 dominant and two pathogenic bacterial genera shows a separation between soil and root samples, as well as between NDS and MDS/HDS samples, or between the NDR/MDR and HDR samples on the first component axis, which explained 49.4% of the total variation (Figure 5). The second component explained 30.6% of the total variation. The component loading plot shows groups of OTU associated with the different konjac samples. In the root samples, a large group of OTUs, including those belonging to genera Enterobacter, Pseudomonas, Caulobacter, Variovorax, Rhizobium, and Bradyrhizobium, were associated with the MDR samples. Moreover, OTUs belonging to Streptomyces, Enterobacter, and Pseudomonas were associated with the NDR samples, while those from Lentzea and P. carotovorum were associated with the HDR samples. In the soil samples, a large group of OTUs, including those belonging to genera Bacillus, Arthrobacter, and Sphingomonas, were associated with the NDS samples. Additionally, OTUs from Sphingomonas and Serratia were associated with the MDS samples, while those from Serratia and P. carotovorum were associated with the HDS samples (Figure 5).
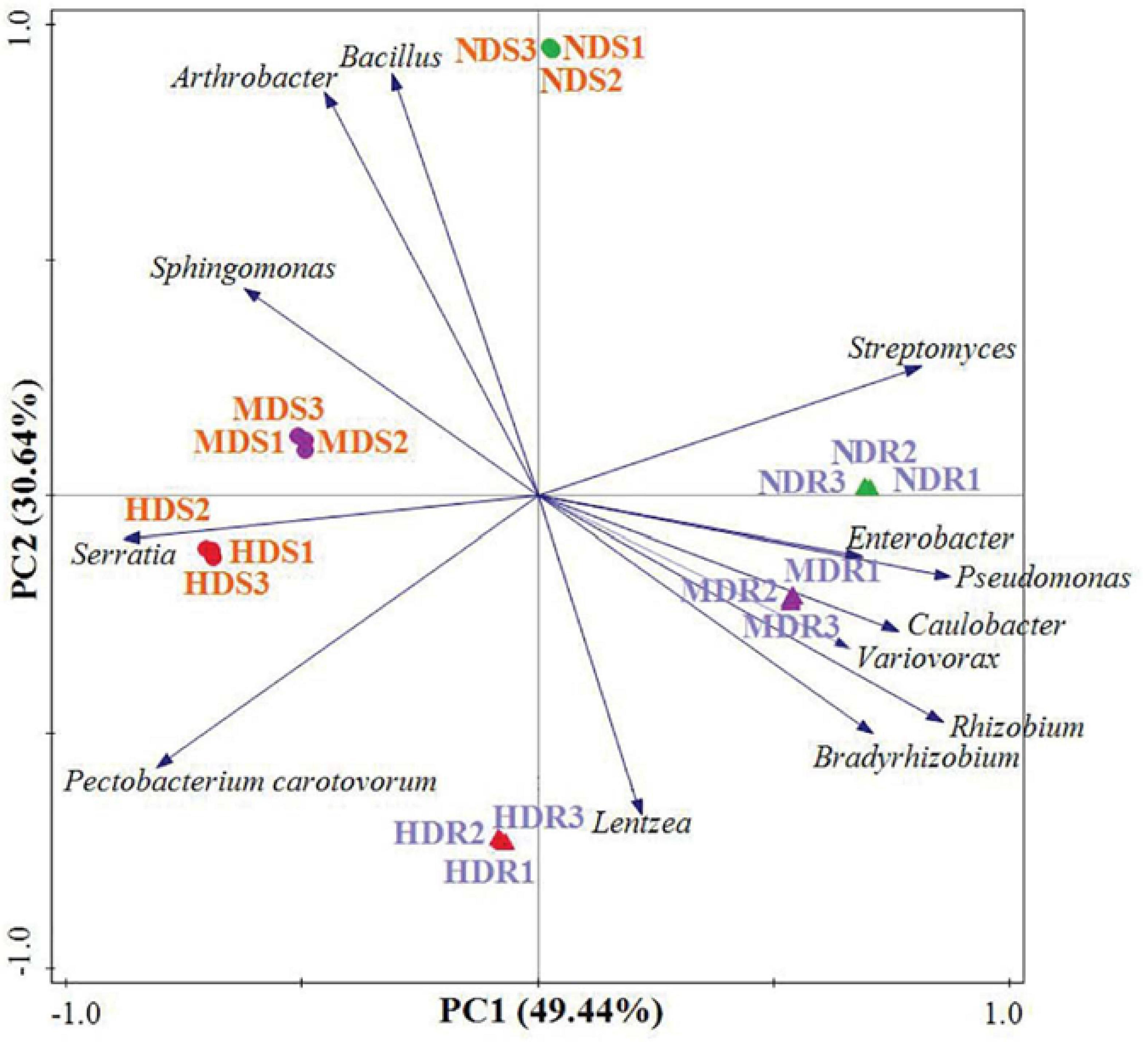
Figure 5. Principal component analysis of the dominant and pathogenic bacterial operational taxonomic units (genera) recovered from the root and soil samples in different konjac growing sites. NDS, MDS, and HDS represent the soil samples from the non-diseased, moderately diseased, and highly diseased sites, respectively. NDR, MDR, and HDR represent the plant root samples from the non-diseased, moderately diseased, and highly diseased sites, respectively.
The OTU data generated a high resolution for distinguishing the three sampling sites in terms of bacterial communities. All the bacterial communities recovered from each site clustered together with high identity (Figure 6). The clustering analysis showed a clear separation between the three sampling sites, indicating that different sites had distinctive characteristics and significantly influenced the bacterial communities. Generally, the MD and HD sites were more similar to each other than to the ND site in terms of the bacterial community structure in both soil and root samples. Furthermore, the RDA results showed that, in the soil samples, RDA1 separated NDS from MDS and HDS, whereas RDA2 separated MDS from HDS (Figure 7A); in the root samples, RDA1 separated MDR from NDR and HDR, whereas RDA2 separated NDR from HDR (Figure 7B). Soil AK, sucrase activity, and urease activity showed the largest effects on the bacterial community structure in both soil and root samples.
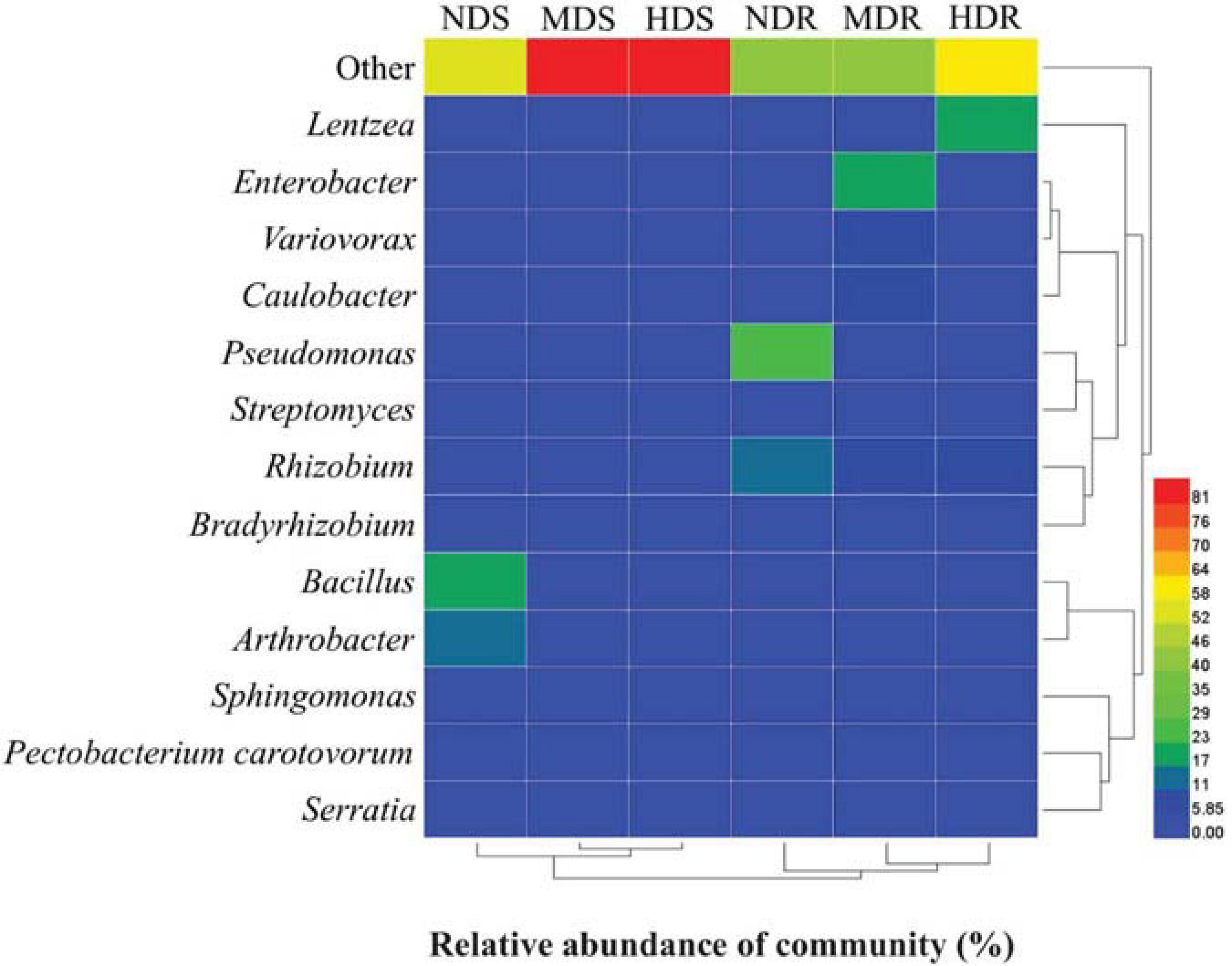
Figure 6. Heatmap of bacterial operational taxonomic units in soil and root samples of konjac from the non-diseased (NDS and NDR), moderately diseased (MDS and MDR), and highly diseased (HDS and HDR) sites.
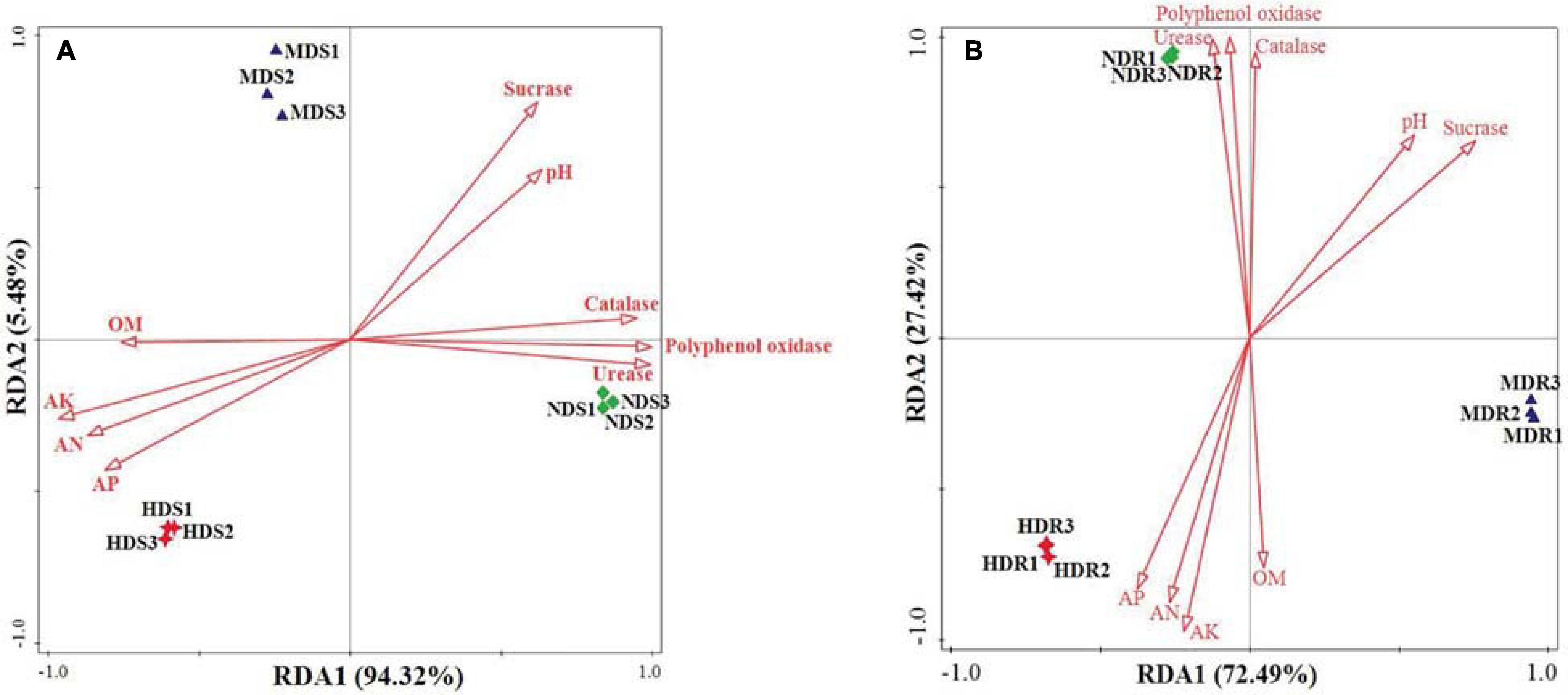
Figure 7. Redundancy analysis revealing the effects of different degrees of soft rot disease on soil variables and bacterial community structure (genus level) in the soil (A) and root (B) samples of konjac from the non-diseased (NDS and NDR), moderately diseased (MDS and MDR), and highly diseased (HDS and HDR) sites. OM, organic matter; AN, alkaline-hydrolyzable nitrogen; AK, available potassium; and AP, available phosphorus.
Discussion
In the present study, soil pH and nutrient levels changed at a small site scale due to the development of konjac soft rot under a R. pseudoacacia plantation. Specifically, soil pH significantly decreased with increasing degree of konjac soft rot, which is in agreement with the finding of Wu et al. (2017) that soil pH was higher in healthy soils than in diseased soils with continuously cropped konjac plants infected by Pectobacterium spp. The lower pH after soft rot development could be explained by the accumulation of organic acid through anaerobic decomposition and the associated environmental variation. For instance, the variation in soil conditions could be caused by weathering and biotic factors such as mycorrhizal development (Akema and Futai, 2005). Moreover, soil OM, AN, AP, and AK contents were significantly higher in the HD site than in the ND konjac site, which is probably attributed to increased OM decomposition, decreased carbon uptake, and reduced utilization of nutrients (N, P, and K) under the influence of soft rot disease.
Soil enzymatic activity is regarded as a crucial indicator of soil quality and agroecological stability (Acosta-Martinez et al., 2018). Sucrase catalyzes the hydrolysis of sucrose to produce fructose and glucose (Frankeberger and Johanson, 1983), while catalase catalyzes the decomposition of H2O2 into O2 and H2O (Kou et al., 2018). Here, soil sucrase and catalase activity exhibited a remarkable decrease in the HD site compared with the ND site of konjac. This result concurs with the findings from a previous study on notoginseng (Panax notoginseng) under no-till cultivation (You et al., 2014), indicating that an increase in the enzymatic activity of sucrase and catalase may be a response to soft rot disease and may enhance the protective effect on plant cells. Urease catalyzes the conversion of urea to ammonia and carbon dioxide (Phang et al., 2018), while polyphenol oxidase is mainly involved in the metabolism of aromatic organic carbon (Li et al., 2015). Soil urease and polyphenol oxidase activity exhibited a remarkable and continuous decrease with increasing incidence of konjac soft rot, which may be not advantageous to nitrogen utilization and carbon metabolism of plants affected by soft rot.
Proteobacteria and Actinobacteria, the most abundant phyla in various agroecosystems (Farmer et al., 2016; Huang, 2018), were the top two predominant bacterial phyla in both soil and root samples of konjac. And the relative abundance of Proteobacteria in the MD site (soil and root) and HD site (root) was significantly higher than that in the ND site. Similar results were obtained in watermelon (Citrullus lanatus) where the relative abundance of Proteobacteria in the soil of wilted watermelon was higher than that in healthy soil under both rotation and continuous cropping systems (Liang, 2018). However, these results contradict those of Lin et al. (2019), who reported that the abundance of Proteobacteria was negatively correlated with the incidence of banana (Musa nana) wilt under a banana–sugarcane rotation system (Lin et al., 2019). These conflicting results suggest that the relationship between the phylum Proteobacteria and the soft rot of konjac is dependent on the indirect effects of other factors such as various microbial taxa, plant developmental stage, and environmental conditions.
At the genus level, the relative abundance of Pseudomonas significantly decreased with increased incidence of konjac soft rot. Liu (2014) also reported that the abundance of Pseudomonas, which is closely associated with healthy plant growth, was significantly higher in the rhizosphere and non-rhizosphere soil of healthy plants than in diseased plants of ginger (Zingiber officinale; Liu, 2014). The relative abundance of Bacillus was significantly higher in the soil and root endosphere of the ND konjac plants than in the MD and HD plants, suggesting a vital role of Bacillus in healthy konjac growth. A previous study has demonstrated that Bacillus spp. are antagonistic to various potential phytopathogens including bacterial pathogens, fungal pathogens, systemic viruses, and root-knot nematodes (Kloepper et al., 2004). Similarly, the relative abundance of Rhizobium and Streptomyces was higher in the soil and root endosphere of healthy konjac plants. Owing to their high colonization ability, Rhizobium spp. could also be used in non-legumes for growth promotion (Qureshi et al., 2013). Moreover, Streptomyces are prolific producers of natural products with biological activities, such as antibiotics, which play a positive role in the plant rhizosphere microecosystem and control soil-borne pathogens (Jain and Jain, 2007; Xue et al., 2013).
In support of our previous culture-based study (He et al., 2018), here, we found that culturable dominant bacteria, including Bacillus thuringiensis, Rhizobium radiobacter, and Streptomyces cellulosae, were highly associated with the ND site, although it is difficult to determine the exact abundance of each species by the plate counting method. Additionally, the relative abundance of genera Bradyrhizobium and Sphingomonas was higher in the root endosphere of MD and HD konjac plants. Bradyrhizobium spp. and Sphingomonas spp. are reported to have high nitrogen-fixing ability and may enhance the drought and salinity tolerance of plants (Sun et al., 2010; Meng, 2016; Oliveira et al., 2017). These potentially beneficial bacteria, which were abundant in the root zone of ND konjac plants, could have enhanced their resistance to soft rot disease by producing antibacterial substances and inducing systemic resistance. Indeed, Streptomyces, Rhizobium, and Bacillus strains were previously used as biocontrol agents to protect konjac from soft rot (He et al., 2015a, b).
Estimations of the bacterial community composition in the soil and root endosphere of a crop could provide new opportunities for exploring the potential antagonistic microbes for suppression of soil-borne plant pathogens (Song et al., 2018). P. carotovorum is a bacterial pathogen causing soft rot disease in diverse plant species such as konjac (Wu et al., 2011a), pinellia (Pinellia ternata; Hu et al., 2008), cucumber (Cucumis sativus; Nazerian et al., 2011), and potato (Solanum tuberosum; Naas et al., 2018). In our study, the relative abundance of P. carotovorum was 1.498 and 1.051% in the soil and root endosphere of the HD konjac plants, respectively. These values were slightly higher than the relative abundance of Serratia, which is another group of potential pathogens causing soft rot disease (Gillis et al., 2013; Abu-Obeid et al., 2017) in the HD konjac plants (0.159 and 0.006%, respectively). Serratia spp. have been reported to cause soft rot of onion (Allium cepa; Lv et al., 2013) and pepper (Capsicum annuum; Gillis et al., 2013). As expected, the relative abundance of P. carotovorum and Serratia was lower in the ND site than in the MD and HD sites of konjac. Changes in the abundance of these pathogens inhabiting the soil and root endosphere of konjac plants may be associated with the incidence of soft rot.
The occurrence of disease pathogens and pests can cause shifts in root-associated bacterial communities (Kim et al., 2016; Silva et al., 2016). In the present study, the bacterial taxonomic distribution indicated that the relative abundance of dominant taxa was especially affected by different degrees of soft rot damage. Moreover, specific taxa could be related to the characteristics of distinct konjac growing sites. For instance, Bacillus, Streptomyces, Enterobacter, and Pseudomonas were associated with the ND site; Sphingomonas, Caulobacter, and Variovorax were associated with the MD site; and Serratia, P. carotovorum, and Lentzea were associated with the HD site. Therefore, these dominant genera may be used as indicators to assess the degree of damage in konjac plants due to soft rot disease. Furthermore, the bacterial alpha diversity indices were significantly lower in the MD and HD konjac sites. Zhang et al. (2013) also found that bacterial Shannon–Wiener and McIntosh indices were much lower in the soil of garlic (Allium sativum) infected by root rot disease than in those of healthy plants. The results clearly demonstrated that the infection of konjac plants due to P. carotovorum and Serratia altered the root-associated bacterial community structure. The effect of potentially beneficial bacteria on the resistance of konjac plants against soft rot merits further study.
Conclusion
In the present study, three sampling sites were investigated to explore the effects of soft rot damage on bacterial communities associated with the roots of konjac plants growing under a pure R. pseudoacacia plantation. Different degrees of soft rot disease altered soil physicochemical properties, with a large decrease in soil pH and an increase in soil OM, alkaline-hydrolyzable nitrogen, AP, and AK contents. Meanwhile, soil enzymatic activity was decreased, while the abundance and alpha diversity of root-associated bacteria were reduced due to soft rot damage of konjac plants. In conclusion, soft rot disease caused remarkable shifts in the community structure of root-associated bacteria of konjac mainly by altering the levels of soil AK, sucrase activity, and urease activity. Quantification of the dominant bacterial taxa provides an approach to predict the degree of damage due to konjac soft rot.
Data Availability Statement
The datasets presented in this study can be found in online repositories. The names of the repository/repositories and accession number(s) can be found below: https://www.ncbi.nlm.nih.gov/, PRJNA623222. All raw sequences were submitted to the Sequence Read Archive of the National Center for Biotechnology Information database under the accession number from SRR11490401 to SRR11490418.
Author Contributions
FH conceived, designed, performed the experiments, analyzed the data, and wrote the manuscript.
Funding
This research was supported by the National Natural Science Foundation of China (31901468), the Innovation Capacity Support Plan of Shaanxi Province (2020KJXX-003), the Key Research and Development Program of Shaanxi Province (2021NY-048), the Project of Key Laboratory of Se-enriched Products Development and Quality Control, Ministry of Agriculture and Rural Affairs (Se-2019B02), Se-enriched Special Plan of China Se-enriched Industry Research Institute (2019QCY-2.2), the Science and Technology Planning Project of Ankang City (AK2020-CQ01-1), and the Project of Ankang Agricultural Technology Extension Center (2017AYHX016).
Conflict of Interest
The author declares that the research was conducted in the absence of any commercial or financial relationships that could be construed as a potential conflict of interest.
Acknowledgments
The authors are grateful to the staff of the Bureau of Konjac Langao (Shaanxi, China) for their assistance in root and soil sampling.
Supplementary Material
The Supplementary Material for this article can be found online at: https://www.frontiersin.org/articles/10.3389/fmicb.2021.652758/full#supplementary-material
Supplementary Figure 1 | Growth conditions of konjac plants in the non-diseased (ND; A), moderately diseased (MD; B), and highly diseased (HD; C) sampling sites under a pure Robinia pseudoacacia plantation.
Supplementary Table 1 | Significant differences in the relative abundance of major bacterial phyla among three soil and three root samples of konjac collected from the non-diseased (NDS and NDR), moderately diseased (MDS and MDR), and highly diseased (HDS and HDR) sites. Different lowercase and uppercase letters indicate significant differences among the soil and root samples from different sampling sites, respectively (P ≤ 0.05; LSD test).
Supplementary Table 2 | Significant differences in the relative abundance of dominant bacterial genera among three soil and three root samples of konjac collected from the non-diseased (NDS and NDR), moderately diseased (MDS and MDR), and highly diseased (HDS and HDR) sites. Different lowercase and uppercase letters indicate significant differences among the soil and root samples from different sampling sites, respectively (P ≤ 0.05; LSD test).
Footnotes
References
Abu-Obeid, I., Khlaif, H., and Salem, N. (2017). Detection and identification of bacterial soft rot of potato Pectobacterium carotovorum subsp. carotovorum using specific PCR primers in Jordan. Afr. J. Agric. Res. 12, 2910–2918. doi: 10.5897/AJAR2017.12620
Acosta-Martinez, V., Cano, A., and Johnson, J. (2018). Simultaneous determination of multiple soil enzyme activities for soil health-biogeochemical indices. Appl. Soil Ecol. 126, 121–128. doi: 10.1016/j.apsoil.2017.11.024
Adamczyk, B., Kitunen, V., and Smolander, A. (2009). Polyphenol oxidase, tannase and proteolytic activity in relation to tannin concentration in the soil organic horizon under silver birch and Norway spruce. Soil Biol. Biochem. 41, 2085–2093. doi: 10.1016/j.soilbio.2009.07.018
Akema, T., and Futai, K. (2005). Ectomycorrhizal development in a Pinus thunbergii stand in relation to location on a slope and effect on tree mortality from pine wilt disease. J. Forest Res. 10, 93–99. doi: 10.1007/s10310-004-0101-3
April, R., and Keller, D. (1990). Mineralogy of the rhizosphere in forest soils of the eastern United States. Biogeochemistry 9, 1–18. doi: 10.1007/BF00002714
Bao, S. D. (2000). Soil Agricultural Chemistry Analysis. (Beijing: China Agriculture Press), 34–107.
Caporaso, J. G., Kuczynski, J., Stombaugh, J., Bittinger, K., Bushman, F. D., and Costello, E. K. (2010). QIIME allows analysis of high-throughput community sequencing data. Nat. Methods 7, 335–336. doi: 10.1038/nmeth.f.303
Chen, G. H. (2013). Screening of antagonistic bacterium to control konjac soft rot. Adv. Mater. Res. 72, 4427–4430. doi: 10.4028/www.scientific.net/amr.726-731.4427
Chen, H. B., and Boutros, P. C. (2011). VennDiagram: a package for the generation of highly-customizable Venn and Euler diagrams in R. BMC Bioinformatics 12:35. doi: 10.1186/1471-2105-12-35
Chopyk, J., Chattopadhyay, S., Kulkarni, P., Smyth, E. M., Hittle, L. E., and Paulson, J. N. (2017). Temporal variations in cigarette tobacco bacterial community composition and tobacco-specific nitrosamine content are influenced by brand and storage conditions. Front. Microbiol. 8:358. doi: 10.3389/fmicb.2017.00358
Czajkowski, R., Pérombelon, M. C. M., Jafra, S., Lojkowska, E., Potrykus, M., and van der Wolf, J. M. (2014). Detection, identification and differentiation of Pectobacterium and Dickeya species causing potato blackleg and tuber soft rot: a review. Ann. Appl. Biol. 166, 1–21. doi: 10.1111/aab.12166
Duan, L. F., Guo, B. L., Cai, Y. G., Qin, J. F., Chen, G. A., and Liu, C. P. (2018). Effects of different maize density as interplant crops on the yield and plant morbidity of Amorphophallus konjac. J. Shanxi Agric. 38, 22–25. doi: 10.13842/j.cnki.issn1671-8151.201804019
Edgar, R. C., Haas, B. J., Clemente, J. C., Quince, C., and Knight, R. (2011). UCHIME improves sensitivity and speed of chimera detection. Bioinformatics 27, 2194–2200. doi: 10.1093/bioinformatics/btr381
Farmer, J., Zhang, B., Jin, X. X., Zhang, P., and Wang, J. K. (2016). Long-term effect of plastic film mulching and fertilization on bacterial communities in a brown soil revealed by high through-put sequencing. Arch. Agron. Soil Sci. 63, 230–241. doi: 10.1080/03650340.2016.1193667
Frankeberger, J. R. W. T., and Johanson, J. B. (1983). Method of measuring invertase activity in soils. Plant Soil 74, 301–311. doi: 10.1007/BF02181348
Gillis, A., Rodriguez, M., and Santana, M. A. (2013). Serratia marcescens associated with bell pepper (Capsicum annuum L.) soft-rot disease under greenhouse conditions. Eur. J. Plant Pathol. 138, 1–8. doi: 10.1007/s10658-013-0300-x
Guan, S. M., Zhang, D. S., and Zhang, Z. M. (1986). The Soil Enzyme Research. (Beijing: Agriculture Press), 274–338.
Guan, Z. J., Lu, S. B., Huo, Y. L., Guan, Z. P., Liu, B., and Wei, W. (2016). Do genetically modified plants affect adversely on soil microbial communities? Agric. Ecosyst. Environ. 235, 289–305. doi: 10.1016/j.agee.2016.10.026
He, F., and Cui, M. (2017). Research advances in biological control of soft rot disease occurrence in Amorphophallus konjac. Shaanxi J. Agric. Sci. 63, 64–67. doi: 10.3969/j.issn.0488-5368.2017.01.019
He, F., Xue, Q. H., Zhang, Z. L., and Duan, J. L. (2014). Microbial ecological study about Amorphophallus konjac root-zone soil under walnut grove. J. Beijing Union Univ. 28, 26–32. doi: 10.16255/j.cnki.ldxbz.2014.02.001
He, F., Zhang, Z. L., Cui, M., Liu, L. P., and Xue, Q. H. (2018). Soft rot disease alters soil characteristics and root-associated, culturable microbial community of Amorphophallus konjac. J. Gen. Plant Pathol. 84, 44–57. doi: 10.1007/s10327-017-0759-y
He, F., Zhang, Z. L., Cui, M., Wang, D. S., and Xue, Q. H. (2015a). Identification, disease resistance and growth-promoting effect of dominant bacteria in the rhizosplane soil of healthy Amorphophallus konjac. Acta Agric. Boreali-Occidentalis Sin. 24, 75–83. doi: 10.7606/j.issn.1004-1389.2015.08.013
He, F., Zhang, Z. L., Cui, M., Xue, Q. H., and Wang, D. S. (2015b). Disease prevention and growth promotion effects of actinomycete strain D74 on Amorphophallus konjac. Acta Hortic. Sin. 42, 367–376. doi: 10.16420/j.issn.0513-353x.2014-0766
He, F., Zhang, Z. L., Liu, L. P., Cui, M., and Xue, Q. H. (2015c). Microecological mechanism for healthy growth and higher yield of Amorphophallus konjac under acacia forest. Acta Bot. Boreali-Occidentalis Sin. 35, 364–372. doi: 10.7606/j.issn.1000-4025.2015.02.0364
Helmy, M., Baddar, D., and El’ Masry, M. H. (2008). Affinity purification of a siderophore that exhibits an antagonistic effect against soft rot bacterium. Biochemistry 73, 776–782. doi: 10.1134/S0006297908070055
Hu, X. F., Ying, F. X., He, Y. B., Gao, Y. Y., Chen, H. M., and Chen, J. S. (2008). Characterization of Pectobacterium carotovorum subsp. carotovorum causing soft-rot disease on Pinellia ternata in China. Eur. J. Plant Pathol. 120, 305–310. doi: 10.1007/s10658-007-9219-4
Huang, Y. H. (2018). Comparison of rhizosphere and endophytic microbial communities of Chinese leek through high-throughput 16S rRNA gene Illumina sequencing. J. Integr. Agric. 17, 359–367. doi: 10.1016/S2095-3119(17)61731-3
Jain, P. K., and Jain, P. C. (2007). Isolation, characterization and antifungal activity of Streptomyces sampsonii GS 1322. Indian J. Exp. Biol. 45, 203–206. doi: 10.1049/el:20031169
Jennings, S. B., Brown, N. D., and Sheil, D. (1999). Assessing forest canopies and understorey illumination: canopy closure, canopy cover and other measures. Forestry 72, 59–74. doi: 10.1093/forestry/72.1.59
Kandeler, E., and Gerber, H. (1988). Short-term assay of soil urease activity using colorimetric determination of ammonium. Biol. Fertil. Soils 6, 68–72. doi: 10.1007/BF00257924
Kim, B., Song, G. C., and Ryu, C. M. (2016). Root exudation by aphid leaf infestation recruits root-associated Paenibacillus spp. to lead plant insect susceptibility. J. Microbiol. Biotechnol. 26, 549–557. doi: 10.4014/jmb.1511.11058
Kloepper, J. W., Ryu, C. M., and Zhang, S. (2004). Induced systemic resistance and promotion of plant growth by Bacillus spp. Phytopathology 94, 1259–1266. doi: 10.1094/phyto.2004.94.11.1259
Kou, T. J., Lam, S. K., Chen, D. L., and Yu, W. W. (2018). Soil urease and catalase responses to ozone pollution are affected by the ozone sensitivity of wheat cultivars. J. Agron. Crop Sci. 204, 424–428. doi: 10.1111/jac.12268
Li, J. J., Liu, F., and Zhou, X. M. (2015). Effects of different reclaimed scenarios on soil microbe and enzyme activities in mining areas. Environ. Sci. 36, 1836–1841. doi: 10.13227/j.hjkx.2015.05.044
Li, T., Wei, W. G., Shao, W., Chen, B. L., and Huang, Y. P. (2010). Lead and other elemental content of normal and Erwinia carotovora infected Amorphallus konjac corms. Bull. Environ. Contam. Toxicol. 84, 744–748. doi: 10.1007/s00128-010-9996-x
Liang, Z. H. (2018). Watermelon rhizosphere soil bacterial diversity affects the occurrence of Fusarium wilt. Chinese Agric. Sci. Bull. 34, 69–76. doi: cnki:sun:zntb.0.2018-17-012
Lin, W. P., Zeng, L. S., Lv, S., Wu, C. R., Wang, F., and Zhou, J. K. (2019). Continual effect and mechanism of banana-sugarcane plantation on control of Fusarium wilt (II). Chinese J. Eco-Agric. 27, 348–357. doi: 10.13930/j.cnki.cjea.180362
Liu, H. J. (2014). The Relationship Between Soil Factors and Bacterial Wilt Root of ginger in Qianwei Sichuan. Sichuan: Sichuan Agricultural University.
Liu, J. J., Yao, Q., Li, Y. S., Zhang, W., Mi, G., and Chen, X. L. (2019). Continuous cropping of soybean alters the bulk and rhizospheric soil fungal communities in a Mollisol of Northeast PR China. Land Degrad. Dev. 30, 1725–1738. doi: 10.1002/ldr.3378
Lv, H. P., Wei, H., Qi, Y. H., Zhang, Y. M., Cao, S. F., and Yang, F. R. (2013). Pathogen identification from soft rot disease of onion bulbs in Jiayuguan, Gansu province. Acta Pratac. Sin. 22, 153–159. doi: 10.11686/cyxb20130419
Magoč, T., and Salzberg, S. L. (2011). Flash: fast length adjustment of short reads to improve genome assemblies. Bioinformatics 27, 2957–2963. doi: 10.1093/bioinformatics/btr507
Meng, N. (2016). The Mechanism Study on Rhizobia Symbiosis Promoting Effect of the Salt Resistance of Soybean Seedlings. Nanjing: Nanjing Agricultural University.
Naas, H., Sebaihia, M., Orfei, B., Rezzonico, F., Buonaurio, R., and Moretti, C. (2018). Pectobacterium carotovorum subsp. brasiliense and Pectobacterium carotovorum subsp. carotovorum as causal agents of potato soft rot in Algeria. Eur. J. Plant Pathol. 151, 1027–1034. doi: 10.1007/s10658-018-1438-3
Nazerian, E., Sijam, K., Zainal Abidin, M. A., and Vadamalai, G. (2011). First report of soft rot caused by Pectobacterium carotovorum subsp. Carotovorum on cucumber in Malaysia. Plant Dis. 95:1474. doi: 10.1094/PDIS-10-10-0754
Nelson, D. W., and Sommers, L. E. (1982). “Total carbon, organic carbon and organic matter,” in Methods of Soil Analysis, Part 2, Chemical and Microbiological Properties, ed. A. L. Page (Madison, WI: American Society of Agronomy), 539–579.
Oliveira, R. S., Carvalho, P., Marques, G., Ferreira, L., Pereira, S., and Nunes, M. (2017). Improved grain yield of cowpea (Vigna unguiculata) under water deficit after inoculation with Bradyrhizobium elkanii and Rhizophagus irregularis. Crop Pasture Sci. 68, 1052–1059. doi: 10.1071/CP17087
Oyedele, D. J., Gasu, M. B., and Awotoye, O. O. (2008). Changes in soil properties and plant uptake of heavy metals on selected municipal solid waste dump sites in Ile-Ife, Nigeria. Afr. J. Environ. Sci. Technol. 3, 106–115.
Page, A. L. (1982). “Chemical and microbiological properties,” in Methods of Soil Analysis, Part 2, Chemical and Microbiological Properties, ed. A. L. Page (Madison, WI: American Society of Agronomy), 625–641.
Phang, I. R. K., Chan, Y. S., Wong, K. S., and Lau, S. Y. (2018). Isolation and characterisation of urease-producing bacteria from tropical peat. Biocatal. Agric. Biotechnol. 13, 168–175. doi: 10.1016/j.bcab.2017.12.006
Qiu, B. T. (2013). Experimental Research and Modeling of Flaky Konjac Drying. Chongqing: Southwest University.
Qureshi, M. A., Shahzad, H., Imran, Z., Mushtaq, M., Akhtar, N., and Ali, M. A. (2013). Potential of Rhizobium species to enhance growth and fodder yield of maize in the presence and absence of L-Tryptophan. J. Anim. Plant Sci. 23, 1448–1454.
Silva, T. F. D., Estebanez Vollú, R., Marques, J. M., Falcão Salles, J., and Seldin, L. (2016). The bacterial community associated with rose-scented geranium (Pelargonium graveolens) leaves responds to anthracnose symptoms. Plant Soil 414, 1–11. doi: 10.1007/s11104-016-3102-z
Song, X. H., Pan, Y., Li, L. Y., Wu, X. L., and Wang, Y. (2018). Composition and diversity of rhizosphere fungal community in Coptis chinensis Franch. continuous cropping fields. PLoS One 13:e0193811. doi: 10.1371/journal.pone.0193811
Song, Z. M., and Liu, C. A. (2018). Four counties in Shaanxi Province won the title of national key county of konjac industry. Mod. Enterp. 65.
Stepniewska, Z., Wolinska, A., and Ziomek, J. (2009). Response of soil catalase activity to chromium contamination. J. Environ. Sci. 8, 128–133. doi: 10.1016/S1001-0742(08)62394-3
Su, J. Q., Ding, L. J., Xue, K., Yao, H. Y., and Zhu, Y. G. (2014). Long-term balanced fertilization increases the soil microbial functional diversity in a phosphorus-limited paddy soil. Mol. Ecol. 24, 136–150. doi: 10.1111/mec.13010
Sun, J. G., Zhang, Y. C., Xu, J., and Hu, H. Y. (2010). Isolation, identification and inoculation effect of nitrogen-fixing bacteria Sphingomonas GD542 from maize rhizosphere. Chin. J. Eco-Agric. 18, 89–93. doi: 10.3724/SP.J.1011.2010.00089
The State Council of the People’s Republic of China (2019). Data from: Xinhua News. Beijing: State Council of the People’s Republic of China.
Vasques, C. A., Rossetto, S., Halmenschlager, G., Linden, R., Heckler, E., and Fernandez, M. S. (2008). Evaluation of the pharmacotherapeutic efficacy of Garcinia cambogia plus Amorphophallus konjac for the treatment of obesity. Phytother. Res. 22, 1135–1140. doi: 10.1002/ptr.2323
Wang, Y., Sheng, H. F., He, Y., Wu, J. Y., Jiang, Y. X., and Tam, N. F. Y. (2012). Comparison of the levels of bacterial diversity in freshwater, intertidal wetland, and marine sediments by using millions of illumina tags. Appl. Environ. Microb. 78, 8264–8271. doi: 10.1128/AEM.01821-12
Wu, J. P., Diao, Y., Gu, Y. C., and Hu, Z. L. (2010). Infection pathways of soft rot pathogens on Amorphophallus konjac. Afr. J. Microbiol. Res. 4, 1495–1499. doi: 10.1016/j.mrgentox.2010.05.014
Wu, J. P., Diao, Y., Gu, Y. C., and Hu, Z. L. (2011a). Molecular detection of Pectobacterium species causing soft rot of Amorphophallus konjac. World J. Microbiol. Biotechnol. 27, 613–618. doi: 10.1007/s11274-010-0496-2
Wu, J. P., Ding, Z. L., Diao, Y., and Hu, Z. L. (2011b). First report on Enterobacter sp. causing soft rot of Amorphophallus konjac in China. J. Gen. Plant Pathol. 77, 312–314. doi: 10.1007/s10327-011-0330-1
Wu, J. P., Jiao, Z. B., Guo, F. L., Chen, L. F., Ding, Z. L., and Qiu, Z. M. (2016). Constitutive and secretory expression of the Aiia in Pichia pastoris inhibits Amorphophallus konjac soft rot disease. Am. J. Mol. Biol. 6, 79–87. doi: 10.4236/ajmb.2016.62009
Wu, J. P., Jiao, Z. B., Zhou, J., Guo, F. L., Ding, Z. L., and Qiu, Z. M. (2017). Analysis of bacterial communities in rhizosphere soil of continuously cropped healthy and diseased konjac. World J. Microbiol. Biotechnol. 33:134. doi: 10.1007/s11274-017-2287-5
Wu, J. P., Liu, X. Y., Diao, Y., Ding, Z. L., and Hu, Z. L. (2012). Authentication and characterization of a candidate antagonistic bacterium against soft rot of Amorphophallus konjac. Crop Prot. 34, 83–87. doi: 10.1016/j.cropro.2011.12.008
Wu, J. P., Yang, C. Z., Jiao, Z. B., Hu, Z. L., Ding, Z. L., and Qiu, Z. M. (2015). Genetic relationships of soft rot bacteria isolated from konjac in China by amplified fragment length polymorphism (AFLP) and 16S rDNA gene sequences. Agric. Sci. 06, 717–723. doi: 10.4236/as.2015.67069
Xue, L., Xue, Q. H., Chen, Q., Lin, C. F., Shen, G. H., and Zhao, J. (2013). Isolation an evaluation of rhizosphere actinomycetes with potential application for biocontrol of Verticillium wilt of cotton. Crop Prot. 43, 231–240. doi: 10.1016/j.cropro.2012.10.002
You, C. M., Lu, X. J., and Guan, H. L. (2014). The relevance of notoginseng root rot to the enzyme activity in soil. J. Yunnan Normal Univ. 34, 25–29. doi: 10.3969/j.issn.1007-9793.2014.06.005
Zhang, L. J., Mao, J., Zhang, Z. D., and Xie, Y. Q. (2013). Study of microbial community diversity in rhizosphere soil from garlic infected by root rot disease. Xinjiang Agric. Sci. 50, 2109–2117. doi: 10.6048/j.issn.1001-4330.2013.11.021
Zhang, L. Z. (2017). High-yielding cultivation technology of konjac under forest in Yun County, Yunnan Province. Agric. Eng. Technol. 4, 59–62. doi: 10.16815/j.cnki.11-5436/s.2017.11.050
Zhou, J. Z., Wu, L. Y., Deng, Y., Zhi, X. Y., Jiang, Y. H., and Tu, Q. C. (2011). Reproducibility and quantitation of amplicon sequencing-based detection. ISME J. 5, 1303–1313. doi: 10.1038/ismej.2011.11
Keywords: Amorphophallus konjac, bacterial community composition, Illumina high-throughput sequencing, root-associated bacteria, soft rot disease
Citation: He F (2021) Response of Root-Associated Bacterial Communities to Different Degrees of Soft Rot Damage in Amorphophallus konjac Under a Robinia pseudoacacia Plantation. Front. Microbiol. 12:652758. doi: 10.3389/fmicb.2021.652758
Received: 21 January 2021; Accepted: 09 June 2021;
Published: 08 July 2021.
Edited by:
Lucy N. Moleleki, University of Pretoria, South AfricaReviewed by:
Zongzhuan Shen, Nanjing Agricultural University, ChinaTeresa Ann Coutinho, University of Pretoria, South Africa
Copyright © 2021 He. This is an open-access article distributed under the terms of the Creative Commons Attribution License (CC BY). The use, distribution or reproduction in other forums is permitted, provided the original author(s) and the copyright owner(s) are credited and that the original publication in this journal is cited, in accordance with accepted academic practice. No use, distribution or reproduction is permitted which does not comply with these terms.
*Correspondence: Fei He, aGVmZWk2MDAwQDE2My5jb20=