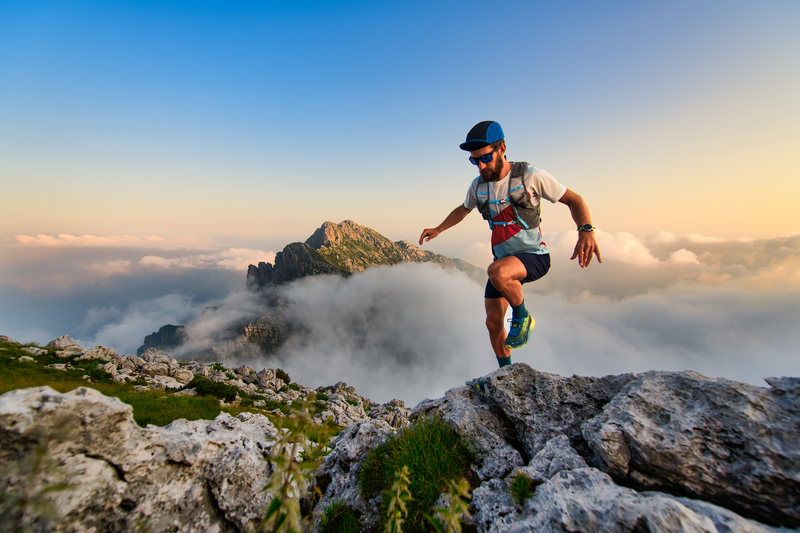
95% of researchers rate our articles as excellent or good
Learn more about the work of our research integrity team to safeguard the quality of each article we publish.
Find out more
ORIGINAL RESEARCH article
Front. Microbiol. , 25 March 2021
Sec. Evolutionary and Genomic Microbiology
Volume 12 - 2021 | https://doi.org/10.3389/fmicb.2021.649466
This article is part of the Research Topic The Uncultured Microorganisms: Novel Technologies and Applications View all 7 articles
The oriental white stork (Ciconia boyciana) is considered an endangered species based on the International Union for Conservation of Nature (IUCN) Red List. This study presents the first evidence on comparative analysis of gut microbial diversity of C. boyciana from various breeding conditions. To determine the species composition and community structure of the gut microbiota, 24 fecal samples from Tianjin Zoo and Tianjin Qilihai Wetland were characterized by sequencing 16S rRNA gene amplicons using the Illumina MiSeq platform. Firmicutes was found to be the predominant phylum. Analysis of community structure revealed significant differences in the species diversity and richness between the populations of the two breeding conditions. The greatest α-diversity was found in wild C. boyciana, while artificial breeding storks from Tianjin Zoo had the least α-diversity. Principal coordinates analysis showed that the microbial communities were different between the two studied groups. In conclusion, this study reveals the species composition and structure of the gut microbiota of oriental white storks under two breeding conditions, and our findings could contribute to the integrative conservation of this endangered bird.
Gut microbiota plays a crucial role in the health of animal hosts through many factors, such as genetics, geography, nutrition, diet, and immunity (Kohl, 2012; van Dongen et al., 2013; Kong et al., 2014; Waite and Taylor, 2014, 2015). It is well-established that the gut microbiota of an animal functions from the moment the animal is born, the amount and species of gut microbiota are not fixed (Xiong et al., 2019; Li et al., 2020). The external environment and the host itself influence the diversity of gut microorganisms (Ley et al., 2006; Mueller et al., 2006; Waite and Taylor, 2014). Thus, research on gut microbiomes can improve survival of animals in different living environments and an emerging suite of novel tools from this research is being applied for animal health and conservation field (Clemente et al., 2012; Lee and Hase, 2014; Waite and Taylor, 2015).
Studies of gut flora are being conducted in many animals, but among these, there is considerably less research on birds than mammals (Clemente et al., 2012). Many factors influence the intestinal flora of birds, such as population diversity and different life-history characteristics including migratory behavior, diet, flight ability, and mating system (Xenoulis et al., 2010; van Dongen et al., 2013; Stanley et al., 2015; Colston and Jackson, 2016).
Nowadays 16S rRNA high-throughput sequencing technologies has been used to determine composition and compare structures of the intestinal flora recent years (Cole et al., 2009; Wang et al., 2015; Wu et al., 2016; Cui et al., 2019). With the development of high-throughput sequencing, the study of avian gut microbiota has become feasible and economical (Salter et al., 2014; Hird et al., 2015). Consequently, the amount of data generated in this field has markedly increased, providing more information on the relationships among gut microbiomes across different bird species and habitats (Michel et al., 2018). For instance, it is found that cecal microbes of wild and captive western grouse are significantly different since the living environments, including diet and exercise intensity, differ between the two groups (Wienemann et al., 2011). Wang et al. (2020) compared the compositions and differences in gut microbiomes of black-necked cranes (Grus nigricollis) from six wintering areas in China, and found that the overwintering food sources might lead to specific gut microbes (Wang et al., 2020).
The oriental white stork (Ciconia boyciana) is a large water fowl that is endangered due to the loss of habitat and disturbance through human activities (Cheng et al., 2019; Fan et al., 2020). The main breeding areas of oriental white storks are limited to northern China and southeastern Russia (Liu et al., 2008; Zan et al., 2008; Yamada et al., 2019). The number of wild oriental white storks has declined sharply past decade, with approximately 1,000–2,499 mature individuals globally (IUCN, 2020), and this species is listed as nationally protected animals in China (Itoh et al., 1997; Cardoso et al., 2016; Han et al., 2016). Conservation of C. boyciana is of great significance and considerable efforts from various research fields have been conducted in this endangered species (e.g., Liu et al., 2008; Han et al., 2016; Wei et al., 2016; Cheng et al., 2020). However, the environmental influence on the intestinal flora of oriental white storks have yet to be elucidated. Considering the integrative conservation of endangered species, one related comparative analysis is therefore required to understand whether gut microbiota of oriental white storks differ between different regions.
The present study aimed to compare fecal microbial community compositions and diversity of oriental white storks from two breeding conditions by using the Illumina MiSeq platform for high-throughput sequencing for the first time. Differences in the community structure as well as species composition of the fecal microbiota in C. boyciana were compared, and the unique and shared bacteria were identified with the aim of finding relationships between different groups. Results of the study will facilitate understanding of the fecal microbiota in oriental white storks under two breeding conditions and could help improve population conservation of this endangered species.
All applicable international, national, and/or institutional guidelines for the care and use of animals were strictly followed. All animal sample collection protocols complied with the current laws of China. According to the Law of the People’s Republic of China on the Protection of Wildlife (Order of the President of the People’s Republic of China, No. 16, 2018), all experimental procedures carried out in this study were under permitted. The non-invasive genetic sampling taken in this work enables collecting samples (fecal samples) without the need to directly disturb or contact individuals of oriental white storks.
Twelve fecal samples of oriental white storks (Ciconia boyciana) were collected at Tianjin Zoo (Z Group) and Tianjin Qilihai Wetland (W group), respectively in 2019 (Table 1). Tianjin Qilihai Wetland is one of the main stopover habitats of wild C. boyciana during their migration periods. All samples were carefully taken from the inside of the fecal matter using sterilized equipment, and then were placed on dry ice and transported to the laboratory as soon as possible.
The total genomic DNA of all fecal samples was isolated by CTAB extraction method; Sodium dodecyl sulfate (SDS) extraction method; Guanidine thiocyanate (GuSCN) extraction method and TIANamp Stool DNA Kit (TIANGEN, China). For all methods, DNA extraction was conducted according to the references and manufacturer’s instructions (Hosomi et al., 2017; Kachiprath et al., 2018). The concentration and quality of DNA samples were determined by a NanoDrop 2000 spectrophotometer (Thermo Fisher Scientific, Waltham, MA, United States).
The V3–V4 region of the 16S rRNA gene was amplified based on the literature using specific PCR primers (Wu et al., 2016). Forward primer 338F (5′-ACTCCTACGGGAGGCAGCAG-3′) and reverse primer 806R (5′- GGACTACHVGGGTWTCTAAT-3′) were used (Caporaso et al., 2012). Amplification was carried out in 50-μL reactions with 10 ng template DNA and 25 μL Pfu DNA polymerase mix. The PCR cycle comprised an initial denaturation at 94°C for 5 min, followed by 30 cycles of 94°C for 15 s, 60°C for 15 s, and 72°C for 30 s.
Purified PCR amplicons were sequenced on an Illumina MiSeq platform at Majorbio Bio-Pharm Technology Co., Ltd., Shanghai, China. PE amplicon libraries were constructed, and all raw reads were screened according to the primer sequences.
Raw files of the total genomic DNA from fecal samples were demultiplexed by QIIME (version 1.9.1), and high-quality reads were clustered as an operational taxonomic unit (OTU) by UPARSE pipeline (version 7.1) when the sets of sequences shared at least 97% identity (Edgar, 2013). In this study, gene sequence taxonomy was analyzed by RDP Classifier (version 2.2)1 (Wang et al., 2007) using a confidence threshold of 80% against the Silva 16S rRNA database (Release 128)2 (Quast et al., 2013).
Rarefaction curves were plotted for each sample to determine the abundance of communities and sequencing data of each fecal sample (Amato et al., 2013). α-diversity and species accumulation curve were calculated by mothur software (version v.1.30.1), which showed the complexity of gut flora species in two groups. β-diversities analysis was performed and principal coordinate analyses (PCoA) based on OTU compositions were determined, in order to investigate structural variation in microbial communities of two groups using unweighted and weighted UniFrac distance metrics (Lozupone and Knight, 2005). The one-way analysis of variance ANOVA (Permutational multivariate analysis of variance) was performed to compare α-diversity estimates and p < 0.05 was considered statistically significant. Differences in the UniFrac distances for pairwise comparisons were determined using the Student’s t-test.
Taxa abundances in two groups at the phylum, class, order, family, and genus levels were statistically compared by Metastats (an improved statistical method for analysis of metagenomic data) (White et al., 2009). Microbial functions were predicted by PICRUSt based on high-quality sequences and the linear discriminant analysis effect size (LEfSe) was used to present bacterial taxonomic distributions of sample communities (Segata et al., 2011).
DNA extraction is a very critical step for high-throughput sequencing and the choice of a DNA extraction method has an important impact on DNA yield and purity. As the results shown, the GuSCN extraction method and the TIANamp Stool DNA Kit produced much higher DNA yields than the CTAB and SDS methods (Table 2). The obtained DNA yields by all the four extraction methods were higher than 10 ng/μl. The 260/230 absorbance ratios GuSCN extraction method and TIANamp Stool DNA Kit were above 1.8, indicating a low contamination with proteins. However, the average 260/280 absorbance ratios detected for the CTAB and SDS were below 1.8 which might suggest protein contamination from the two methods. In the study of oriental white stork’s fecal sample, the DNA obtained by GuSCN extraction method and TIANamp Stool DNA Kit could both be used successfully in library preparation for high-throughput sequencing. Moreover, DNA extraction using the GuSCN protocol was more cost efficient compared to the commercially TIANamp Stool DNA Kit.
Table 2. DNA yield and DNA purity (absorbance ratios of 260/280 nm) of four different DNA extraction methods.
Across the 24 samples of oriental white storks, a total of 1,263,339 raw reads with an average length of 415 bp were obtained after denoising by Illumina MiSeq sequencing. The total number of raw reads, base pairs, and the mean length of the reads were compared in all the 24 samples (Supplementary Table 1). The number of sequences varied from 42,700 to 70,383, and a total of 2,390 OTUs with 97% sequence similarity threshold representing 43 bacterial phyla were identified in the feces of C. boyciana. The α-diversity indices for bacteria, including Sobs, Shannon, Chao1, ACE, and Good’s coverage are shown in Table 3. The total number of OTUs in the W group (2,134) was much higher than that in the Z group (1,555), these values include 1,299 OTUs shared by both groups. There was a significant difference in α-diversity indices between Z group and W group (p > 0.05) (Supplementary Figure 1). Average Good’s coverage was 99.28 ± 1.57% (mean ± SD) around all 24 fecal samples, and thus the majority of the bacterial diversity in feces was obtained thereby ensuring accuracy of the analyses (Table 3).
Table 3. Estimated OTU richness and diversity indices for each fecal sample of oriental white storks.
To assess whether the depth of sequencing in this study was large enough to estimate the richness of gut microflora, the observed species rarefaction curves were calculated. According to species accumulation curves and Good’s coverage estimator, the curve tended to gradually flatten, indicating that the sample size collected was large enough to identify the majority of OTUs in the gut of oriental white storks (Supplementary Figure 2), and the high Good’s coverage indicated that the majority of bacterial phylotypes in all the samples were identified.
The taxonomic composition of each fecal sample clustering at the 97% phylotype similarity level was outlined at phylum, class, order, family, and genus levels (Supplementary Table 2). Overall gut microbiomes of the oriental white storks comprised of 43 phyla, 96 classes, 188 orders, 338 families, 675 genera, and 1,222 species; microbial compositions at these different levels are shown in Figure 1 and Supplementary Figures 3–5, respectively.
Figure 1. Microbial structure of all fecal samples at phylum and genus levels. (A) Bar-plots showing the abundance and distribution of the 11 most abundant phyla. (B) Bar-plots showing the abundance and distribution of the 11 most abundant genera.
At the phylum level, 43 prokaryotic phyla were identified from the 16S rRNA gene sequences (Figure 1A). Gut microbiota of C. boyciana from Z group was dominated by Firmicutes (65.59%), Actinobacteria (17.23%), and Proteobacteria (10.48%). These three dominant phyla accounted for 93.30% of all sequences across all the samples, while unclassified bacterial sequences only occupied 0.01% (Figure 1A and Supplementary Table 2). The gut microbiota from W group was dominated by Firmicutes (28.43%), Proteobacteria (23.58%), Actinobacteria (14.71%), Chloroflexi (11.57%), and unclassified bacterial sequences accounted for 0.04% of all sequences in the W group (Figure 1A and Supplementary Table 2).
At the genus level, 15 genera had abundances greater than 2% in all the sequences (Figure 1B). The most abundant genera in W group were Lactobacillus (8.33%), norank_f_Anaerolineaceae (5.92%), norank_f_Xanthomonadales_Incertae_Sedis (3.91%), norank_f_Bacteroidales_S24-7_group (3.38%), norank_c_ Cyanobacteria (3.35%), and Thiobacillus (2.943%). The most abundant genera in Z group were Paeniclostridium (23.82%), Lactobacillus (8.55%), Peptostreptococcus (7.78%), Clostridium_sensu_stricto_1 (7.17%), Actinomyces (3.99%), and Romboutsia (3.77%). Mean relative abundances of the 10 most abundant genera are listed in Table 4. Community heatmap analysis at the genus level of the 24 samples can be seen in Figure 2A.
Figure 2. Community heatmap analysis at genus level and α-diversities of gut microbiota among the two populations. (A) Community heatmap analysis on genus level. (B) Bacterial community richness (measured by Sobs index) in the Z and W groups. (C) Bacterial community diversity (measured by Shannon index) in the Z and W groups. p < 0.05 was considered statistically significant. ***P ≤ 0.001.
α-diversity, which refers to the diversity within a particular region, was calculated for two groups to examine differences between oriental white storks from Tianjin Zoo and Tianjin Qilihai Wetland. Community richness measured by OTUs (Sobs and Chao indices) showed that the index of the W group was higher than that of the Z group, indicating that richness of gut microbiota was greater in W group (Supplementary Figure 1). Differences between two groups were statistically significant (p < 0.05) (Figure 2B). Bacterial community diversity (Shannon index) was measured in the two groups, and the index of W group was higher than that of Z group (Supplementary Figure 1). There was a significant difference in community diversity between the W and Z groups (p < 0.05) (Figure 2C).
The relationship between the feces samples from two breeding conditions were represented by a dendrogram using Bray-Curtis distances (Figure 3A). This dendrogram indicated that the gut microbiota of the oriental white stork in Tianjin Zoo clustered together, while those in Tianjin Qilihai Wetland located on similar branches. To evaluate the difference in β-diversity of the 24 samples, PCoA was used to visualize similarities or dissimilarities between the Z and W groups (Figures 3B,C). Each symbol represents one gut microbiota on the PCoA plot, and most of the fecal samples in two groups were distinguishable by PCoA when considering the relative abundances of gut microbiomes. Congruent with the cluster analysis, bacterial communities of group Z clustered tightly and were separated from those of group W under both weighted and unweighted UniFrac metrics. The two groups tended to cluster separately from each other, indicating that each group had distinctive microbial populations. Using weighted UniFrac distance, bacterial communities of group Z were separated from those of group W along principal coordinate axis 1 (PC1) with the largest amount of variation (42.10%) (Figure 3B). Using unweighted UniFrac distance, bacterial communities of the 24 fecal samples explained the largest amount of variation (47.89%) along PC1 (Figure 3C). This clustering pattern was confirmed by an analysis of similarities (ANOSIM) and unweighted UniFrac showed a clearer separation of communities (ANOSIM, R = 0.71). Microbial composition between two groups at the phylum level (ANOSIM, R = 0.5621, p = 0.001) and genus level (ANOSIM, R = 0.6196, p = 0.001) were significantly different (Supplementary Figure 6).
Figure 3. Clustering analysis of the gut microbiotas and PCoA plots analysis. (A) Clustering analysis of the evolution of gut microbiotas in the Z and W groups. Gut microbiota trees were generated based on the Bray–Curtis distances generated by mothur. (B) PCoA plots based on weighted UniFrac distances of gut microbiome of oriental white storks. (C) PCoA plots based on unweighted UniFrac distances of gut microbiome of oriental white storks.
Unique and shared bacterial taxa of the gut microbiota from two groups were analyzed. A Venn diagram demonstrated that 1,299 OTUs from the 24 samples were shared as core bacterial OTUs and the unique OTUs in each group were 256 (Z group) and 839 (W group), respectively (Supplementary Figure 7).
There were some similarities in gut microbiota of oriental white storks from two groups. The top five abundant core phyla were Firmicutes, Proteobacteria, Actinobacteria, Chloroflexi, and Bacteroidetes, while the top five abundant core genera were Paeniclostridium, Lactobacillus, Peptostreptococcus, Clostridium_sensu_stricto_1, and Actinomyces. Next, LEfSe was used to identify OTUs differentially represented between oriental white storks from two groups (Figure 4A). LEfSe identified 10 and 28 taxa (LDA > 4.0) with discrepancies in relative abundance in the Z group and W group, respectively (Figure 4A).
Figure 4. LEfSe analysis of gut microbiota and comparison of core gut microbiotas. (A) LEfSe analysis based on characterizing discriminative features of OTUs. (B) Top five abundant core phyla in two groups of oriental white storks. (C) Top five abundant core genera in two groups of oriental white storks.
The cladogram revealed that the core bacterial species in all 24 fecal samples were significantly different at all levels (Supplementary Figure 8). At the phylum level, the abundance of Firmicutes was significantly higher in the Z group than tin the W group, while the abundance of Bacteroidetes was significantly higher in the W group than in the Z group (p < 0.001) (Figure 4B). At the genus level, Paeniclostridium had a significantly higher abundance in the Z group than in the W group (p < 0.01) (Figure 4C).
A total of 296 KOs (KEGG Orthologs) and 42 KEGG pathways were mapped based on the high throughput sequencing, which were then classified into secondary KEGG pathways. The secondary KEGG pathways associated with the gut microbiotas included cell processes, metabolism, and genetic information processing, indicating that the differences in gut microbiota had important influences on the metabolism of C. boyciana (Figure 5).
This study characterized 24 fecal samples of oriental white storks living in two breeding condition, by sequencing of 16S rRNA gene amplicons using the Illumina MiSeq platform, analyzed the composition of the gut microbiota and compared the difference. The oriental white stork is one endangered waterbird species, and many conservation strategies have been brought up to protect C. boyciana, including establishment of suitable nature reserves (Zhou et al., 2013; Zheng et al., 2016; Tawa and Sagawa, 2020). However, there is limited knowledge of the gut microbiome of C. boyciana to efficiently support related conservation efforts. Therefore, to identify the gut microbiota associated with oriental white storks and differences in bacterial communities arising from captive and wild breeding groups, high-throughput 16S rRNA gene sequencing technology was employed to compare gut microbial compositions of oriental white storks from Tianjin Zoo and Tianjin Qilihai Wetland, respectively. This method allowed exploration of the microbiota composition and abundance without the need for cultivation (Caporaso et al., 2010; Gloor et al., 2010; Knutie and Gotanda, 2018).
Characterization of bacterial communities in the feces of oriental white storks provided evidence that the microbiota compositions were similar between the captivity and wild breeding groups, but abundances of the bacteria were significantly different between two groups. Gut microbiota of C. boyciana were predominantly composed of Firmicutes, Proteobacteria, and Actinobacteria at the phylum level, and Firmicutes was the most abundant phylum of Gram-positive bacteria in both Z group and W group. This microbiota composition was consistent with that of other wild bird species such as Canada goose (Lu et al., 2009), red-crowned cranes (Xie et al., 2016), Darwin’s finches (Michel et al., 2018), chinstrap penguins (Barbosa et al., 2016), northern bald ibis (Spergser et al., 2018), and kakapo (Waite et al., 2014), where members of the phylum Firmicutes dominated the microbiota of avian guts.
Members of the phylum Firmicutes play important roles in metabolism, digestion, and absorption of protein and other nutrients (Bernini et al., 2016; Berry, 2016). For example, Firmicutes are associated with breakdown of fatty acids, and Firmicutes produce more butyrate are which is considered a health-promoting molecule, since it can increase insulin sensitivity, regulate energy metabolism, and increase leptin gene expression. Bacteroidetes mainly produce acetate and propionate. Propionate could reduce the expression of enzymes involved in the de novo synthesis of fatty acids and Acetate could promote the secretion of insulin and ghrelin (Kong et al., 2013; Million et al., 2013; Lee et al., 2015). Although some studies speculated that the Firmicutes-to-Bacteroidetes (F/B) ratio may be related to obesity both in many animal models (Turnbaugh et al., 2006) and humans (Ley et al., 2006), the evidence suggesting an association between obesity and alterations of the Firmicutes/Bacteroidetes ratio is not convincing (Magne et al., 2020). In our study, the F/B ratio in Z group was higher than the ratio in W group, indicating that the gut microbiota of C. boyciana living in Tianjin Zoo could help the host use the nutrients more efficiently and collect calories from food since Firmicutes are more effective. It is difficult to obtain the physical indicators of oriental white storks living in Tianjin Qilihai Wetland, such as weight and health status, thus the relationship between the Firmicutes/Bacteroidetes ratio and obesity of two groups were still unknown.
Paeniclostridium was the most common genus in Z group, while Lactobacillus was the most common genus in W group and was also a major component of the gut microbiota in Z group. The abundance of Lactobacillus was relatively high in both Z group and W group (8.55% and 8.33%, respectively). In previous reports, Lactobacillus were represented in gut microbiota communities that had relatively high β-xylosidase and β-glucosidase levels (Ruggiero et al., 2009; Cabre et al., 2012). The similarity in relative abundance of Lactobacillus in both groups in the current study might be due to the formulation of the diet. The diet of oriental white storks is relatively large, and although the dietary structure is different in two groups, the amounts of sugar consumed in the different environments might be similar. Thus, it would be interesting to study the relationship between dietary structure with habitation and explore the functions of these bacteria in the metabolism pathways of C. boyciana.
The UniFrac method that focused on the phylogenetic relationships was developed by Lozupone and Knight (2005). Both weighted and unweighted UniFrac metrics are very useful for analyzing the differences and associations of microbial communities (Lozupone and Knight, 2005; Campbell et al., 2015). In this study, both weighted and unweighted UniFrac metrics suggested that each fecal sample harbors different microbial communities, and the majority of gut microbiotas were conserved and clustered together among different breeding conditions of oriental white storks (Z group and W group).
PICRUSt was used to analyze the microbial functions of oriental white storks. Gut microbial taxa of C. boyciana were associated with functions such as cell processes and metabolism. These data facilitate understanding of the relationship between gut microbial taxa and metabolism, as well as the influence of gut microbes on host health (Sommer and Backhed, 2013). However, as in other avian studies on gut microbiota, one limitation of the current study is the acquirement of DNA from each individual bird, as this affects the study of gut microbiota in relation to host genetics and physiology at the individual level (Liu et al., 2020).
Analysis of the 24 fecal samples demonstrated that the gut microbiota of oriental white storks was similar to those of red-crowned cranes and black-necked cranes at the phylum level, while Firmicutes was the predominant phylum (Xie et al., 2016; Wang et al., 2020). Comparison of the relative abundance of gut microbiota in C. boyciana between Z group and W group revealed that community structure and abundance of gut microbiota were relatively stable at the phylum level, but were variable and complex at the genus level. There was a significant difference in the richness and diversity of microbial composition, and host intestinal microbiota may be related to diet (Turnbaugh et al., 2009), geography, and seasonal changes (Mueller et al., 2006). The differences between Z group and W group were living conditions and dietary conditions. Oriental white storks inhabiting Tianjin Qilihai Wetland required higher energy and had a more diverse diet, so W group had a greater abundance of gut microbiota than Z group. This study suggests that biogeographical and dietary factors may contribute to the community structure of the gut microbiota of C. boyciana since the metabolism and energy harvest needs were different between two groups. This is consistent with previous related avian findings that community structure and abundance of the gut microbiota are mainly affected by dietary conditions (Wang et al., 2020). The difference on diet patterns may contribute to the differences of fecal microbiota on two groups, and more work is needed to understand the functions of diet patterns and living condition on fecal microbiota. So next, whether living condition changes in the fecal microbiota will be investigated using a combination of metagenomics and metabolomics. Furthermore, since the different microbiota could have different effects on the digestion ability of carbohydrates, protein, and cellulose, the differences in feed composition will have important influences on the changes in host gut microbiota. This may affect nutrient utilization and could cause gastrointestinal diseases in oriental white storks. Hence, studies on the gut microbiota of C. boyciana will facilitate understanding of the specific functions of different bacteria related to digestion and absorption in the host, and could help improve conservation for threatened species both in artificial breeding groups and in wild groups.
This study characterized the gut microbiota of oriental white storks under different breeding conditions using 16S rRNA gene sequencing analysis. Microbiota compositions were similar between Z group and W group, but the abundances of bacteria differed. The genetic factors were the same, therefore it is hypothesized that the differences are mainly related to the environment and diet. Most gut microbiotas were clustered, respectively, between the different breeding conditions, and the gut microbiota were related to host metabolic pathways. This study provides insights into the composition and differences in gut microbiota of oriental white storks living in two breeding conditions. The findings will facilitate our further understanding of the relationship between biogeography, diet structure, and species diversity of the gut microbiota in oriental white storks, and thus help for the integrative conservation of this endangered species.
The datasets presented in this study can be found in online repositories. The names of the repository/repositories and accession number(s) can be found in the article/Supplementary Material.
D-PZ, HW, and Q-HZ conceived and designed the study and wrote the manuscript. F-TW collected the samples. HW and F-TW conducted the experiments. D-PZ, HW, and F-TW analyzed the data. All authors contributed to the article and approved the submitted version.
This study was supported by the Guangxi Key Laboratory of Rare and Endangered Animal Ecology, Guangxi Normal University and National Natural Science Foundation of China (grant numbers 31772468 and 31200293).
The authors declare that the research was conducted in the absence of any commercial or financial relationships that could be construed as a potential conflict of interest.
We thank the staff of Tianjin Zoo and Tianjin Qilihai Wetland Nature Reserve Management Committee for allowing us access to work at these places. We also thank the editors and reviewers at Frontiers in Microbiology for improving the manuscript with their professional suggestions.
The Supplementary Material for this article can be found online at: https://www.frontiersin.org/articles/10.3389/fmicb.2021.649466/full#supplementary-material
Amato, K. R., Yeoman, C. J., Kent, A., Righini, N., Carbonero, F., Estrada, A., et al. (2013). Habitat degradation impacts black howler monkey (Alouatta pigra) gastrointestinal microbiomes. ISME J. 7, 1344–1353. doi: 10.1038/ismej.2013.16
Barbosa, A., Balague, V., Valera, F., Martinez, A., Benzal, J., Motas, M., et al. (2016). Age-related differences in the gastrointestinal microbiota of chinstrap penguins (Pygoscelis antarctica). PLoS One 11:e0153215. doi: 10.1371/journal.pone.0153215
Bernini, L. J., Simao, A. N., Alfieri, D. F., Lozovoy, M. A., Mari, N. L., de Souza, C. H., et al. (2016). Beneficial effects of Bifidobacterium lactis on lipid profile and cytokines in patients with metabolic syndrome: a randomized trial. effects of probiotics on metabolic syndrome. Nutrition 32, 716–719. doi: 10.1016/j.nut.2015.11.001
Berry, D. (2016). The emerging view of firmicutes as key fibre degraders in the human gut. Environ. Microbiol. 18, 2081–2083. doi: 10.1111/1462-2920.13225
Cabre, E., Manosa, M., and Gassull, M. A. (2012). Omega-3 fatty acids and inflammatory bowel diseases-a systematic review. Br. J. Nutr. 107(Suppl. 2), S240–S252. doi: 10.1017/S0007114512001626
Campbell, A. M., Fleisher, J., Sinigalliano, C., White, J. R., and Lopez, J. V. (2015). Dynamics of marine bacterial community diversity of the coastal waters of the reefs, inlets, and wastewater outfalls of southeast Florida. Microbiologyopen 4, 390–408. doi: 10.1002/mbo3.245
Caporaso, J. G., Kuczynski, J., Stombaugh, J., Bittinger, K., Bushman, F. D., Costello, E. K., et al. (2010). QIIME allows analysis of high-throughput community sequencing data. Nat. Methods 7, 335–336. doi: 10.1038/nmeth.f.303
Caporaso, J. G., Lauber, C. L., Walters, W. A., Berg-Lyons, D., Huntley, J., Fierer, N., et al. (2012). Ultra-high-throughput microbial community analysis on the Illumina HiSeq and MiSeq platforms. ISME J. 6, 1621–1624. doi: 10.1038/ismej.2012.8
Cardoso, P., Stoev, P., Georgiev, T., Senderov, V., and Penev, L. (2016). Species conservation profiles compliant with the IUCN red list of threatened species. Biodivers. Data J. 4:e10356. doi: 10.3897/BDJ.4.e10356
Cheng, L., Zhou, L., Bao, Y., and Mahtab, N. (2020). Effect of conspecific neighbors on the foraging activity levels of the wintering oriental Storks (Ciconia boyciana): benefits of social information. Ecol. Evol. 10, 10384–10394. doi: 10.1002/ece3.6693
Cheng, L., Zhou, L., Wu, L., and Feng, G. (2019). Nest site selection and its implications for conservation of the endangered oriental stork Ciconia boyciana in yellow river delta. China. Bird Conser. Int. 30, 323–334. doi: 10.1017/s0959270919000303
Clemente, J. C., Ursell, L. K., Parfrey, L. W., and Knight, R. (2012). The impact of the gut microbiota on human health: an integrative view. Cell 148, 1258–1270. doi: 10.1016/j.cell.2012.01.035
Cole, J. R., Wang, Q., Cardenas, E., Fish, J., Chai, B., Farris, R. J., et al. (2009). The Ribosomal database project: improved alignments and new tools for rRNA analysis. Nucleic Acids Res. 37, D141–D145. doi: 10.1093/nar/gkn879
Colston, T. J., and Jackson, C. R. (2016). Microbiome evolution along divergent branches of the vertebrate tree of life: what is known and unknown. Mol. Ecol. 25, 3776–3800. doi: 10.1111/mec.13730
Cui, Y. F., Wang, F. J., Yu, L., Ye, H. H., and Yang, G. B. (2019). Metagenomic comparison of the rectal microbiota between rhesus macaques (Macaca mulatta) and cynomolgus macaques (Macaca fascicularis). Zool. Res. 40, 89–93. doi: 10.24272/j.issn.2095-8137.2018.061
Edgar, R. C. (2013). UPARSE: highly accurate OTU sequences from microbial amplicon reads. Nat. Methods 10, 996–998. doi: 10.1038/nmeth.2604
Fan, S., Zhao, Q., Li, H., Zhu, B., Dong, S., Xie, Y., et al. (2020). Cyclical helping hands: seasonal tailwinds differentially affect migrating oriental storks (Ciconia boyciana) travel speed. Avian Res. 11:10
Gloor, G. B., Hummelen, R., Macklaim, J. M., Dickson, R. J., Fernandes, A. D., MacPhee, R., et al. (2010). Microbiome profiling by illumina sequencing of combinatorial sequence-tagged PCR products. PLoS One 5:e15406. doi: 10.1371/journal.pone.0015406
Han, J. I., Jang, H. J., and Na, K. J. (2016). Hematologic and serum biochemical reference intervals of the oriental white stork (Ciconia boyciana) and the application of an automatic hematologic analyzer. J. Vet. Sci. 17, 399–405. doi: 10.4142/jvs.2016.17.3.399
Hird, S. M., Sanchez, C., Carstens, B. C., and Brumfield, R. T. (2015). Comparative gut microbiota of 59 neotropical bird species. Front. Microbiol. 6:1403. doi: 10.3389/fmicb.2015.01403
Hosomi, K., Ohno, H., Murakami, H., Natsume-Kitatani, Y., Tanisawa, K., Hirata, S., et al. (2017). Method for preparing DNA from feces in guanidine thiocyanate solution affects 16s rRNA-based profiling of human microbiota diversity. Sci. Rep. 7:4339.
Itoh, Y., Ogawa, A., Murata, K., Hosoda, T., and Mizuno, S. (1997). Identification of the sex of oriental white stork, Ciconia boyciana, by the polymerase chain reaction based on its sex chromosome-specific DNA sequences. Genes Genet. Syst. 72, 51–56. doi: 10.1266/ggs.72.51
Kachiprath, B., Jayanath, G., Solomon, S., and Sarasan, M. (2018). CTAB influenced differential elution of metagenomic DNA from saltpan and marine sediments. 3 Biotech 8:44. doi: 10.1007/s13205-017-1078-x
Knutie, S. A., and Gotanda, K. M. (2018). A non-invasive method to collect fecal samples from wild birds for microbiome studies. Microb. Ecol. 76, 851–855. doi: 10.1007/s00248-018-1182-4
Kohl, K. D. (2012). Diversity and function of the avian gut microbiota. J. Comp. Physiol B. 182, 591–602. doi: 10.1007/s00360-012-0645-z
Kong, L. C., Holmes, B. A., Cotillard, A., Habi-Rachedi, F., Brazeilles, R., Gougis, S., et al. (2014). Dietary patterns differently associate with inflammation and gut microbiota in overweight and obese subjects. PLoS One 9:e109434. doi: 10.1371/journal.pone.0109434
Kong, L. C., Tap, J., Aron-Wisnewsky, J., Pelloux, V., Basdevant, A., Bouillot, J. L., et al. (2013). Gut microbiota after gastric bypass in human obesity: increased richness and associations of bacterial genera with adipose tissue genes. Am. J. Clin. Nutr. 98, 16–24. doi: 10.3945/ajcn.113.058743
Lee, S. M., Han, H. W., and Yim, S. Y. (2015). Beneficial effects of soy milk and fiber on high cholesterol diet-induced alteration of gut microbiota and inflammatory gene expression in rats. Food Funct. 6, 492–500. doi: 10.1039/c4fo00731j
Lee, W. J., and Hase, K. (2014). Gut microbiota-generated metabolites in animal health and disease. Nat. Chem. Biol. 10, 416–424. doi: 10.1038/nchembio.1535
Ley, R. E., Turnbaugh, P. J., Klein, S., and Gordon, J. I. (2006). Microbial ecology: human gut microbes associated with obesity. Nature 444, 1022–1023.
Li, H. Z., Li, N., Wang, J. J., Li, H., Huang, X., Guo, L., et al. (2020). Dysbiosis of gut microbiome affecting small intestine morphology and immune balance: a rhesus macaque model. Zool. Res. 41, 20–31. doi: 10.24272/j.issn.2095-8137.2020.004
Liu, H., Li, Z., Si, H., Zhong, W., Fan, Z., and Li, G. (2020). Comparative analysis of the gut microbiota of the blue fox (Alopex lagopus) and raccoon dog (Nyctereutes procyonoides). Arch. Microbiol. 202, 135–142. doi: 10.1007/s00203-019-01721-0
Liu, H.-Y., Lin, Z.-S., and Liu, H.-Y. (2008). Response of oriental white storks Ciconia boyciana to the accumulative impact of anthropogenic habitat destruction and possible Allee effect. Bird Conser. Int. 18, 292–300. doi: 10.1017/s0959270908000440
Lozupone, C., and Knight, R. (2005). UniFrac: a new phylogenetic method for comparing microbial communities. Appl. Environ. Microbiol. 71, 8228–8235. doi: 10.1128/aem.71.12.8228-8235.2005
Lu, J., Santo Domingo, J. W., Hill, S., and Edge, T. A. (2009). Microbial diversity and host-specific sequences of Canada goose feces. Appl. Environ. Microbiol. 75, 5919–5926. doi: 10.1128/aem.00462-09
Magne, F., Gotteland, M., Gauthier, L., Zazueta, A., Pesoa, S., Navarrete, P., et al. (2020). The Firmicutes/Bacteroidetes ratio: a relevant marker of gut dysbiosis in obese patients? Nutrients 12:1474. doi: 10.3390/nu12051474
Michel, A. J., Ward, L. M., Goffredi, S. K., Dawson, K. S., Baldassarre, D. T., Brenner, A., et al. (2018). The gut of the finch: uniqueness of the gut microbiome of the Galapagos vampire finch. Microbiome 6:167.
Million, M., Lagier, J. C., Yahav, D., and Paul, M. (2013). Gut bacterial microbiota and obesity. Clin. Microbiol. Infect 19, 305–313.
Mueller, S., Saunier, K., Hanisch, C., Norin, E., Alm, L., Midtvedt, T., et al. (2006). Differences in fecal microbiota in different European study populations in relation to age, gender, and country: a cross-sectional study. Appl. Environ. Microbiol. 72, 1027–1033. doi: 10.1128/aem.72.2.1027-1033.2006
Quast, C., Pruesse, E., Yilmaz, P., Gerken, J., Schweer, T., Yarza, P., et al. (2013). The SILVA ribosomal RNA gene database project: improved data processing and web-based tools. Nucleic Acids Res. 41, D590–D596.
Ruggiero, C., Lattanzio, F., Lauretani, F., Gasperini, B., Andres-Lacueva, C., and Cherubini, A. (2009). Omega-3 polyunsaturated fatty acids and immune-mediated diseases: inflammatory bowel disease and rheumatoid arthritis. Curr. Pharm. Des. 15, 4135–4148. doi: 10.2174/138161209789909746
Salter, S. J., Cox, M. J., Turek, E. M., Calus, S. T., Cookson, W. O., Moffatt, M. F., et al. (2014). Reagent and laboratory contamination can critically impact sequence-based microbiome analyses. BMC Biol. 12:87.
Segata, N., Izard, J., Waldron, L., Gevers, D., Miropolsky, L., Garrett, W. S., et al. (2011). Metagenomic biomarker discovery and explanation. Genome Biol. 12:R60. doi: 10.1186/gb-2011-12-6-r60
Sommer, F., and Backhed, F. (2013). The gut microbiota–masters of host development and physiology. Nat. Rev. Microbiol. 11, 227–238. doi: 10.1038/nrmicro2974
Spergser, J., Loncaric, I., Tichy, A., Fritz, J., and Scope, A. (2018). The cultivable autochthonous microbiota of the critically endangered Northern bald ibis (Geronticus eremita). PLoS One 13:e0195255. doi: 10.1371/journal.pone.0195255
Stanley, D., Geier, M. S., Chen, H., Hughes, R. J., and Moore, R. J. (2015). Comparison of fecal and cecal microbiotas reveals qualitative similarities but quantitative differences. BMC Microbiol. 15:51.
Tawa, K., and Sagawa, S. (2020). Stable isotopic analysis of stuffed specimens revealed the feeding habits of oriental storks Ciconia boyciana in Japan before their extinction in the wild. J. Ornithol. 162, 193–206. doi: 10.1007/s10336-020-01806-4
Turnbaugh, P. J., Hamady, M., Yatsunenko, T., Cantarel, B. L., Duncan, A., Ley, R. E., et al. (2009). A core gut microbiome in obese and lean twins. Nature 457, 480–484. doi: 10.1038/nature07540
Turnbaugh, P. J., Ley, R. E., Mahowald, M. A., Magrini, V., Mardis, E. R., and Gordon, J. I. (2006). An obesity-associated gut microbiome with increased capacity for energy harvest. Nature 444, 1027–1031.
van Dongen, W. F., White, J., Brandl, H. B., Moodley, Y., Merkling, T., Leclaire, S., et al. (2013). Age-related differences in the cloacal microbiota of a wild bird species. BMC Ecol. 13:11. doi: 10.1186/1472-6785-13-11
Waite, D. W., and Taylor, M. W. (2014). Characterizing the avian gut microbiota: membership, driving influences, and potential function. Front. Microbiol. 5:223.
Waite, D. W., and Taylor, M. W. (2015). Exploring the avian gut microbiota: current trends and future directions. Front. Microbiol. 6:673.
Waite, D. W., Eason, D. K., and Taylor, M. W. (2014). Influence of hand rearing and bird age on the fecal microbiota of the critically endangered kakapo. Appl. Environ. Microbiol. 80, 4650–4658. doi: 10.1128/aem.00975-14
Wang, Q., Garrity, G. M., Tiedje, J. M., and Cole, J. R. (2007). Naive Bayesian classifier for rapid assignment of rRNA sequences into the new bacterial taxonomy. Appl. Environ. Microbiol. 73, 5261–5267. doi: 10.1128/aem.00062-07
Wang, W., Wang, F., Li, L., Wang, A., Sharshov, K., Druzyaka, A., et al. (2020). Characterization of the gut microbiome of black-necked cranes (Grus nigricollis) in six wintering areas in China. Arch. Microbiol. 202, 983–993. doi: 10.1007/s00203-019-01802-0
Wang, X., Su, X., Cui, X., and Ning, K. (2015). MetaBoot: a machine learning framework of taxonomical biomarker discovery for different microbial communities based on metagenomic data. PeerJ 3:e993. doi: 10.7717/peerj.993
Wei, Z. H., Li, Y. K., Xu, P., Qian, F. W., Shan, J. H., and Tu, X. B. (2016). Patterns of change in the population and spatial distribution of oriental white storks (Ciconia boyciana) wintering in Poyang Lake. Zool. Res. 37, 338–346.
White, J. R., Nagarajan, N., and Pop, M. (2009). Statistical methods for detecting differentially abundant features in clinical metagenomic samples. PLoS. Comput. Biol. 5:e1000352. doi: 10.1371/journal.pcbi.1000352
Wienemann, T., Schmitt-Wagner, D., Meuser, K., Segelbacher, G., Schink, B., Brune, A., et al. (2011). The bacterial microbiota in the ceca of Capercaillie (Tetrao urogallus) differs between wild and captive birds. Syst. Appl. Microbiol. 34, 542–551. doi: 10.1016/j.syapm.2011.06.003
Wu, X., Zhang, H., Chen, J., Shang, S., Wei, Q., Yan, J., et al. (2016). Comparison of the fecal microbiota of dholes high-throughput Illumina sequencing of the V3-V4 region of the 16S rRNA gene. Appl. Microbiol. Biotechnol. 100, 3577–3586. doi: 10.1007/s00253-015-7257-y
Xenoulis, P. G., Gray, P. L., Brightsmith, D., Palculict, B., Hoppes, S., Steiner, J. M., et al. (2010). Molecular characterization of the cloacal microbiota of wild and captive parrots. Vet. Microbiol. 146, 320–325. doi: 10.1016/j.vetmic.2010.05.024
Xie, Y., Xia, P., Wang, H., Yu, H., Giesy, J. P., Zhang, Y., et al. (2016). Effects of captivity and artificial breeding on microbiota in feces of the red-crowned crane (Grus japonensis). Sci. Rep. 6:33350.
Xiong, J. B., Nie, L., and Chen, J. (2019). Current understanding on the roles of gut microbiota in fish disease and immunity. Zool. Res. 40, 70–76.
Yamada, Y., Itagawa, S., Yoshida, T., Fukushima, M., Ishii, J., Nishigaki, M., et al. (2019). Predicting the distribution of released Oriental white stork (Ciconia boyciana) in central Japan. Ecol. Res. 34, 277–285. doi: 10.1111/1440-1703.1063
Zan, S., Zhou, L., Jiang, H., Zhang, B., Wu, Z., and Hou, Y. (2008). Genetic structure of the oriental white stork (Ciconia boyciana): implications for a breeding colony in a non-breeding area. Integr. Zool. 3, 235–244. doi: 10.1111/j.1749-4877.2008.00096.x
Zheng, H., Shen, G., Shang, L., Lv, X., Wang, Q., McLaughlin, N., et al. (2016). Efficacy of conservation strategies for endangered oriental white storks (Ciconia boyciana) under climate change in Northeast China. Biol. Conser. 204, 367–377. doi: 10.1016/j.biocon.2016.11.004
Keywords: gut microbiota, oriental white storks, microbial diversity, high-throughput sequencing, conservation
Citation: Wu H, Wu F-T, Zhou Q-H and Zhao D-P (2021) Comparative Analysis of Gut Microbiota in Captive and Wild Oriental White Storks: Implications for Conservation Biology. Front. Microbiol. 12:649466. doi: 10.3389/fmicb.2021.649466
Received: 04 January 2021; Accepted: 03 March 2021;
Published: 25 March 2021.
Edited by:
Bin-Bin Xie, Shandong University, ChinaReviewed by:
Ursula Höfle, University of Castilla-La Mancha, SpainCopyright © 2021 Wu, Wu, Zhou and Zhao. This is an open-access article distributed under the terms of the Creative Commons Attribution License (CC BY). The use, distribution or reproduction in other forums is permitted, provided the original author(s) and the copyright owner(s) are credited and that the original publication in this journal is cited, in accordance with accepted academic practice. No use, distribution or reproduction is permitted which does not comply with these terms.
*Correspondence: Da-Peng Zhao, c2t5emRwQHRqbnUuZWR1LmNu; emRwXzA2MDlAMTYzLmNvbQ==; Qi-Hai Zhou, emhvdXFoQGlvei5hYy5jbg==
†These authors have contributed equally to this work
Disclaimer: All claims expressed in this article are solely those of the authors and do not necessarily represent those of their affiliated organizations, or those of the publisher, the editors and the reviewers. Any product that may be evaluated in this article or claim that may be made by its manufacturer is not guaranteed or endorsed by the publisher.
Research integrity at Frontiers
Learn more about the work of our research integrity team to safeguard the quality of each article we publish.