- 1Laboratoire de Santé Publique du Québec, Institut National de Santé Publique du Québec, Sainte-Anne-de-Bellevue, QC, Canada
- 2Department of Food Science and Agricultural Chemistry, McGill University, Sainte-Anne-de-Bellevue, QC, Canada
- 3National Microbiology Laboratory, Public Health Agency of Canada, Winnipeg, MB, Canada
- 4Département de Biochimie, de Microbiologie et de Bio-Informatique, Faculté des Sciences et de Génie, Université Laval, Quebec City, QC, Canada
- 5Département de Microbiologie, Infectiologie et Immunologie, Université de Montréal, Montreal, QC, Canada
Whole-genome sequencing (WGS) is the method of choice for bacterial subtyping and it is rapidly replacing the more traditional methods such as pulsed-field gel electrophoresis (PFGE). Here we used the high-resolution core genome single nucleotide variant (cgSNV) typing method to characterize clinical and food from Salmonella enterica serovar Heidelberg isolates in the context of source attribution. Additionally, clustered regularly interspaced short palindromic repeats (CRISPR) analysis was included to further support this method. Our results revealed that cgSNV was highly discriminatory and separated the outbreak isolates into distinct clusters (0–4 SNVs). CRISPR analysis was also able to distinguish outbreak strains from epidemiologically unrelated isolates. Specifically, our data clearly demonstrated the strength of these two methods to determine the probable source(s) of a 2012 epidemiologically characterized outbreak of S. Heidelberg. Using molecular cut-off of 0–10 SNVs, the cgSNV analysis of 246 clinical and food isolates of S. Heidelberg collected in Québec, in the same year of the outbreak event, revealed that retail and abattoir chicken isolates likely represent an important source of human infection to S. Heidelberg. Interestingly, the isolates genetically related by cgSNV also harbored the same CRISPR as outbreak isolates and clusters. This indicates that CRISPR profiles can be useful as a complementary approach to determine source attribution in foodborne outbreaks. Use of the genomic analysis also allowed to identify a large number of cases that were missed by PFGE, indicating that most outbreaks are probably underestimated. Although epidemiological information must still support WGS-based results, cgSNV method is a highly discriminatory method for the resolution of outbreak events and the attribution of these events to their respective sources. CRISPR typing can serve as a complimentary tool to this analysis during source tracking.
Introduction
Non-typhoidal Salmonella (NTS) enterica serovars are the most important causes of bacterial gastroenteritis (Acheson and Hohmann, 2001; Rabsch et al., 2001). Globally, it has been estimated that each year, approximately 93.8 million cases and 155,000 deaths are attributable to NTS (Majowicz et al., 2010). In Canada for example, NTS cause an estimated 88,000 gastrointestinal infections each year (Thomas et al., 2013). Among the NTS serovars, Salmonella enterica serovar Heidelberg is ranked amongst the top three serovars isolated from humans infected with Salmonella in Canada (National Enteric Surveillance Program [NESP], 2018). Outbreaks involving S. Heidelberg have been linked to the consumption of poultry and poultry products (Antunes et al., 2016). During epidemiological investigations, identifying the source(s) of foodborne outbreaks is important in order to implement corrective measures in the food chain that would prevent the reoccurrence of such outbreaks. Pulsed-field gel electrophoresis (PFGE) has been the Gold Standard method used by PulseNet Canada (PNC) since early the 2000s for the molecular typing of foodborne pathogens including Salmonella during outbreak investigations. However, a major drawback with the use of PFGE during outbreak investigations is its low resolution power that is further exacerbated when applied to S. Heidelberg typing owing to the extremely low genetic diversity of this serovar (Bekal et al., 2016; Vincent et al., 2018). This lack of adequate discriminatory power makes it difficult to track the source of a specific clone of S. Heidelberg implicated in foodborne outbreaks. Whole genome sequence (WGS) based methods, owing to their growing availability and high genomic resolution, are rapidly replacing traditional typing methods such as PFGE within major public health laboratories including PulseNet Canada (PNC) (Nadon et al., 2017). WGS-based methods include the high resolution core genome single nucleotide variant analysis typing method (cgSNV). The utility of this typing method in surveillance and outbreak detection has been already demonstrated in several Salmonella serovars in Canada, United States, and Australia (Hoffmann et al., 2014; Fu et al., 2017; Nadon et al., 2017). WGS data can also be mined for the presence of clustered regularly interspaced short palindromic repeats (CRISPR) arrays. CRISPR is part of an adaptive bacterial immunity system that precisely targets invading genetic elements such as phage genomes and plasmids (Garneau et al., 2010). Specifically, a CRISPR array is a genetic structure found in many bacterial genomes that consists of short repeat sequences spaced by short non-repetitive variable sequences named spacers. Variation in spacer content has been exploited for bacterial subtyping and epidemiological investigations in major Salmonella serovars (Shariat and Dudley, 2014).
Here, we assessed the effectiveness of the combination of cgSNV and CRISPR typing for the source tracking of an epidemiologically well-characterized foodborne outbreaks of S. Heidelberg that occurred in Québec in 2012 and non-documented cases. We also wanted to determine whether CRISPR evolution had any impact on the fitness of these isolates and also whether this evolution correlated with that of the cgSNV.
Materials and Methods
Bacterial Isolates Sources
A total of 246 S. Heidelberg isolates from Québec were included in the study. Identification and serotyping were confirmed by the standardized conventional agglutination and PFGE protocols following the PulseNet Canada (PNC) guidelines. One hundred ninety-three clinical isolates were also obtained from patients in Quebec hospitals as part of the active provincial surveillance program. Two food isolates (14-2571 and 14-2570) were obtained during the food poisoning incidents reported by the Ministère de l’Agriculture, des Pêcheries et de l’Alimentation du Québec (MAPAQ). Two outbreaks epidemiologically well documented were included: outbreak 2012-04-SH (n = 6 human isolates) and outbreak 2012-05-SH (n = 8 human isolates and n = 2 food isolates). In this study, non-documented cases (NDC) refer to isolates with incomplete epidemiological data.
No food isolates were identified during the investigation of the outbreak 2012-04-SH. Amongst the 193 human isolates, 155 (80.3%) isolates exhibited pulsotype 2 (PNC designation SHXAI.0001/SHBNI.0001) which represented more than 50% of Quebec clinical isolates, in 2012. The other pulsotypes were used as external controls.
Fifty-one food and environmental isolates were collected as part of the Canadian Integrated Program for Antimicrobial Resistance Surveillance (CIPARS). Abattoir sampling was performed from cecal contents taken post-slaughter from broiler chickens. Routine food surveillance of Salmonella was performed on chicken and turkey and the samples were collected from chain stores and independent butchers.
These 51 S. Heidelberg isolates were subdivided as: chicken samples (n = 23), prepackaged chicken samples (n = 13), turkey samples (n = 7), and cecal chicken samples (n = 8). Epidemiological and genomic data of the isolates recovered from food and environmental samples were previously documented (Edirmanasinghe et al., 2017). Table 1 summarizes the metadata of the 246 S. Heidelberg isolates analyzed in this study.

Table 1. Epidemiologic and subtyping results of 246 S. Heidelberg human clinical and food isolates used in this study.
Whole Genome Sequencing
Isolates were cultured overnight at 37°C in brain heart infusion broth (BHIB). The genomic DNA was then extracted using the Metagenomic DNA isolation Kit for Water (Epicentre, Madison, WI, United States). Samples concentrations were measured with a Qubit fluorometer (Life Technologies, Carlsbad, CA, United States), standardized to 0.2 ng/μl and were stored at −20°C. Libraries were prepared using reagents provided in the Illumina Nextera XT DNA Library Preparation Kit (Illumina, Inc., San Diego, CA, United States) according to the manufacturer’s instructions. Paired-end sequencing was performed on the Illumina MiSeq system using 300 base read lengths. Whole-genome sequence contigs were de novo assembled using the SPAdes Genome Assembler integrated in IRIDA platform (Bankevich et al., 2012).
Core Genome SNV (cgSNV) Analysis
cgSNV analysis was performed using the SNVPhyl pipeline v.1.0 (Petkau et al., 2017) which is integrated as an individual pipeline component within the NML galaxy system (Afgan et al., 2018). Briefly, SMALT v.0.7.5 (The Sanger Institute) was used to align paired-end sequence reads against S. Heidelberg SL476 reference genome (GenBank accession number NC_011083.1). MUMmer v.3.23 (Kurtz et al., 2004) and PHAST (Arndt et al., 2016) were used to identify repeat and prophage regions in the reference genomes, respectively, which were excluded from the analyses. FreeBayes v.0.9.20 (Garrison and Marth, 2012) and SAMtools (Li et al., 2009)/BCFtools (Minevich et al., 2012) calling algorithms were used to identify variants. The SNV alignment was run through PhyML to construct a maximum likelihood tree (Guindon et al., 2010) and FigTree v1.4 was used to generate dendrograms (The Institute of Evolutionary Biology, United Kingdom). PHYLOViZ v2.0 was used to construct the minimum spanning trees based on the geoBURST algorithm (Francisco et al., 2012).
CRISPR Typing
A CRISPR type was defined by the unique spacer composition found in the two Salmonella CRISPR arrays, CRISPR1 and CRISPR2. The two Salmonella CRISPR loci, CRISPR1 and CRISPR2, were identified with the CRISPRFinder web service (Grissa et al., 2007). The direct repeat (29 nt) and spacer (32 nt) sequences were analyzed with Geneious and visualized with custom macros in Microsoft Excel. A CRISPR type of each isolate was defined as the CRISPR profile (CP) with a specific number reflecting its unique allelic type. The spacer sequence alignment was performed with Mega7 using Muscle. CRISPRTarget was used to identify protospacer matches. A match was defined as five or fewer SNPs between a spacer and a protospacer (Biswas et al., 2013; Shariat et al., 2015).
Results
Whole Genome Sequencing Results
We obtained an estimated average genome coverage of 99.4x (range, 30x–240.9x) for the set of 246 S. Heidelberg isolates. The number of SPAdes-assembled contigs (NrContigs) per isolate ranged from 17 to 256 but the majority (95.1%) of isolates assembled into fewer than 55 contigs (Supplementary Table S1).
Cluster Detection Based on the cgSNV Analysis
A total of 154 sequence types (STs) were identified for the 246 S. Heidelberg isolates. The ST defines the set of isolates displaying a genetic distance of 0 SNV. The genetic distance interpretation was based on the Public Health Agency of Canada (PHAC)/PulseNet Canada guidelines used to interpret the relatedness of the outbreak isolates (0–10 SNVs). Based on the maximum likelihood (Supplementary Figure S1) tree and the minimum spanning tree analysis (Figure 1), 16 different clusters (CL), with at least two isolates in each cluster, were identified including clinical and/or food isolates. The genetic distances among each cluster was determined using similarity matrix (data not shown). The outbreak isolates were closely related to other isolates from the same outbreak based on the cgSNV analysis. The documented outbreaks belonged to two distinct clusters (CL1 and CL8) and the genetic distances observed within each outbreak was; 0 and 0–4 SNVs for the outbreak 2012-04-SH and the outbreak 2012-05-SH, respectively (Table 2).
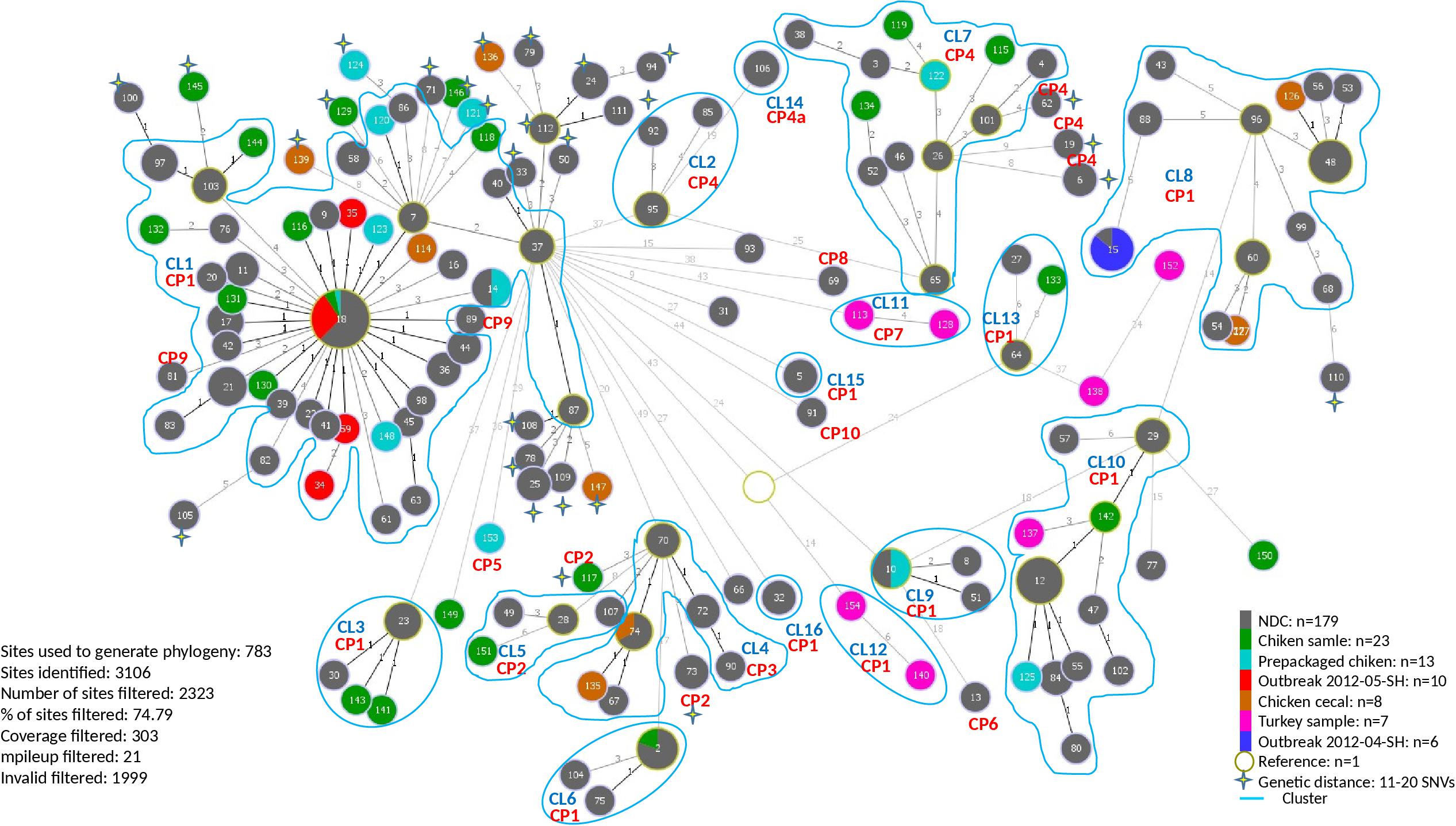
Figure 1. Minimum spanning phylogenetic tree based on the cgSNV analysis of 246 sequenced human and food Salmonella enterica serovar Heidelberg strains isolated in 2012 from the Province of Québec. The size of each node is proportional to the number of isolates and isolates in the same node have 0 SNV difference. The numbers in the circle represent the sequence types and the numbers on the branches connecting the circles represent the number of SNVs differences. CRISPR profiles (CP) are stated for each cluster (CL). The rest of the cgSNV sequence types displayed CP1. Non-documented cases (NDC) represent the isolates with incomplete epidemiological data.
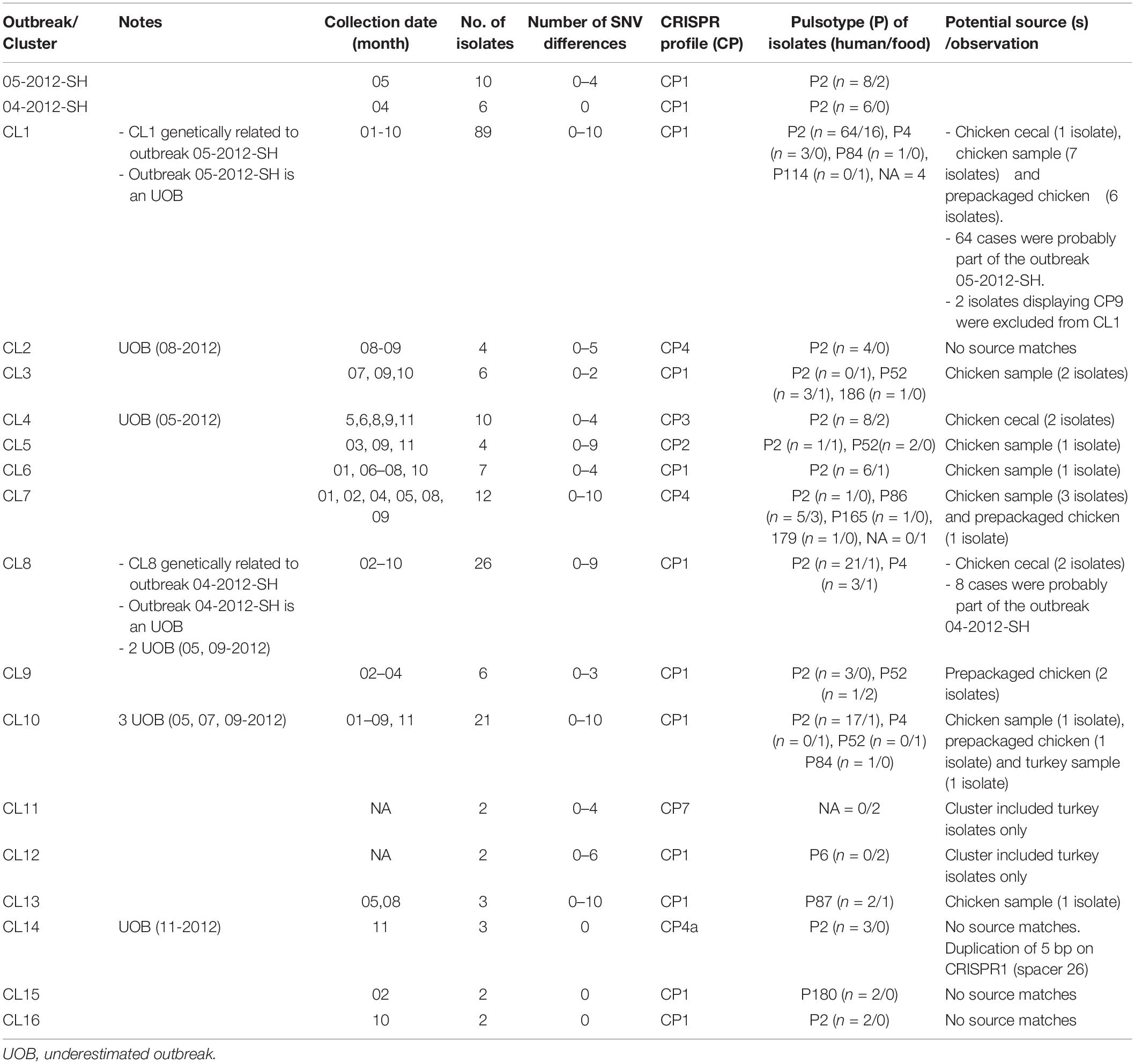
Table 2. Genetic distances between isolates and potential sources of outbreaks and clusters using cgSNV/CRISPR analysis of the 246 Salmonella enterica serovar Heidleberg isolates (2012).
In our study the PFGE patterns identified based on the guidelines described by Tenover et al. (1995) were confirmed to be genetically related by cgSNV as these isolates clustered together. Based on the epidemiological data, the categorization of isolates by cgSNV as outbreak-related was mostly concordant with the results obtained from the PFGE typing method. However, several isolates which were previously excluded from the outbreak investigation due to lack of epidemiological data were clustered with isolates of the two outbreaks as they differed by less than 10 SNVs, suggesting they may have been outbreak-related. Moreover, several human and food isolates displaying 11–20 SNVs were also probably related to the different clusters. Likewise, 10 putative distinct S. Heidelberg outbreaks, occurred in 2012, which were likely underestimated using PFGE analysis were categorized in separate clusters by our analysis. Additionally, cgSNV identified several clinical isolates (ST: 13, 77, 91, 31, 93, 69) as potential sporadic cases not related to the outbreaks and clusters (Figure 1). These isolates differed by >?20 SNVs from outbreak isolates and clusters which were not discriminated using PFGE method.
CRISPR Profiles Distribution
All the CRISPR1 and CRISPR2 arrays identified in this study are shown in Figure 2A. Based on the diversity of their spacer content, only 11 CRISPR profiles (CP) were identified. Putative last common ancestor (LCA), defined as an array containing a full complement of spacers (Shariat et al., 2015), harbored 29 unique spacers for CRISPR1 and 18 unique spacers for CRISPR2. None of the isolates displayed a LCA on the CRISPR1 as the number of spacers ranged from 11 to 27 and displayed eight different allelic types. On the other hand, the number of spacers in CRISPR2 ranged from 6 to 18 and exhibited four different allelic types. Duplication of spacers was not observed in any of the 246 S. Heidelberg isolates. This was concordant with the previous findings of Shariat et al. (2015) for the S. Heidelberg serovar.
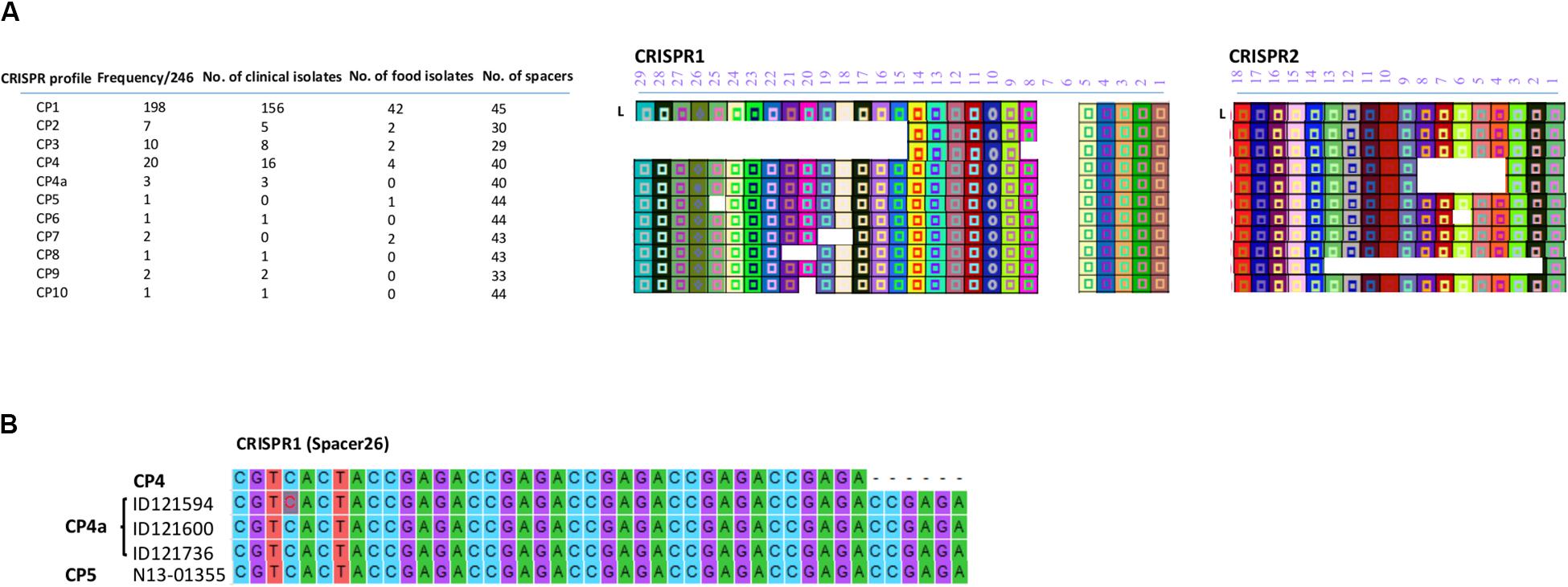
Figure 2. (A) CRISPR patterns and organization of spacer content of CRISPR alleles identified in the 246 human and food S. Heidelberg isolates. Each unique spacer is represented by a colored box and a symbol. The shape of the symbol indicates the length of the spacer. A change in the shape of symbol in CP4a within spacer 26 of CRISPR1 is due to repeated elements and signifies a change in the length of the spacer. The direct repeat sequences located between the spacers are not displayed. L is the position of the leader sequence. (B) Sequence alignment showing the repetition of 6 bp in spacer 26 located in the CRISPR1 array.
Of note, two SNVs occurred in spacer 2 of CRISPR2 in the isolates classified in CP1, A-G and A-T of ID121948 and ID117342, respectively (Supplementary Table S2). All these isolates also belonged to CL10. However, these spacer SNVs were not taken into consideration to distinguish these isolates. Furthermore, we found a repetition of 6 bp (ccgaga) in spacer 26 located on CRISPR1 of the three isolates belonging to the CL14 (ID121594, ID121600, and ID121736) exhibiting CP4a and one food isolate (N13-01355) belonging to the CP5 (Figure 2B). This indicates that the spacer analysis may have some usability to help distinguish some clusters although this hypothesis requires a more-in depth investigation.
We also tried to determine whether any of the analyzed spacers matches phage or plasmid sequences (protospacers) using CRISPRTarget. Among the 396 arrays analyzed from the 246 isolates, only 6 spacers for which 14 putative protospacers were found; 5 spacers on CRISPR1 and 1 spacer on CRISPR2. Interestingly, only two protospacers were found in phage sequences (Salmonella phage SEN34 and Enterobacterial phage mEp39) while 12 protospacers were found in plasmid sequences (Supplementary Table S3).
Almost 80.5% (n = 198) of the isolates exhibited CRISPR profile 1 (CP1), containing 27 and 18 spacers in CRISPR1 and CRISPR2 arrays, respectively. Interestingly, this profile was the most observed in both clinical (156/193 isolates: 80.8%) and food isolates (42/53 isolates: 79.25%). CP1 was shared by both outbreaks (2012-05-SH, 2012-04-SH) and was also found in the majority of the CL1 and CL8 isolates, which are genetically related to the outbreaks. Our analysis revealed two NDCs [ID119099 (ST81) and ID119764 (ST89)], differentiated from CL1 based on CRISPR profile, exhibiting CP9 which lost 12 spacers in the CRISPR2 locus compared to CP1. The arrangement and microevolution of CRISPR spacers allows typing and subtyping. The high-resolution of CRISPR-based typing methods could constitute a practical means for rapid typing and source tracking. The CP4 was the second most frequent CRISPR profile at 8.3% (16/193) of clinical isolates and 7.6% (4/53) of food isolates. This profile lost 5 spacers (4–8) from the CRISPR2 locus and was found in two clusters (CL2 and CL7) and four other cases displaying ST62, 19 and 6 with 9–15 SNVs probably related to CL7. Cluster 4 and cluster 5, which were genetically close (2–11 SNVs differences), were also distinguished based on their CRISPR profiles. They displayed CP3 and CP2 for CL4 and CL5, respectively. In particular, CP5 and CP7 were only found in one prepackaged chicken (N13-01355) and in CL11 containing two retail turkey isolates (N13-01313 and N13-01290), respectively. Whereas, CP6, CP8, and CP10 were identified in sporadic clinical cases (12-2552, ID118298 and ID119947) genetically unrelated to any of the identified clusters.
Source Tracking Using Combined cgSNV and CRISPR Analysis
Our analysis revealed that cgSNV typing linked outbreaks and different cluster isolates to their potential contaminating food source (s). The two isolates obtained during the food poisoning incidents which were obtained from food leftovers recovered from a marriage banquet were perfectly clustered with 2012-05-SH outbreak and several (64 isolates) other NDCs (0–10 SNVs differences). Interestingly, eight retail samples, six prepackaged and one abattoir chicken isolates were seen to have potential genetic linkages with 2012-05-SH outbreak and several cases which were part of the largest cgSNV cluster (CL1) in this study. Moreover, using WGS data, we also observed that the isolates genomically related belonged to the same CRISPR profile (CP1) as the outbreak isolates except for the two NDC displaying CP9 which were excluded from this cluster (Figure 1). Two chicken cecal isolates clustered with CL8, which is genetically related to 2012-04-SH outbreak displaying CP1. Two other chicken cecal isolates were also clustered with CL4 and displayed the same CRISPR profiles (CP3) as the clinical isolates. Among the clusters sharing CP4, only CL7 genetically linked to food isolates (one prepackaged chicken and three retail chicken sample isolates.) Retail chicken was the only possible source of CL3, CL5, and CL6. The prepackaged chicken was also the only potential source of the CL9. Interestingly, CL10 was associated to retail chicken and retail turkey food which indicates that the food contamination source could be due to multiple animal sources. Our analysis suggests poultry products and their environment as one potential source of S. Heidelberg infections.
Discussion
The overarching goal of this research was to demonstrate how an integrative approach between stakeholders led to the identification of the potential source of the S. Heidelberg outbreaks that occurred in 2012 in Québec, Canada. Unlike PFGE method, cgSNV typing was highly discriminatory for Salmonella surveillance and outbreak support (Bekal et al., 2016; Vincent et al., 2018). Moreover, CRISPR typing could reveal genetic relatedness between strains or serovars and could be used for source tracking of Salmonella outbreaks (Deng et al., 2015; Xie et al., 2017). In this study, we analyzed clinical and food isolates, including chicken and turkey products, collected in Quebec in the same year as the outbreaks. The outbreaks and the majority of the human isolates analyzed exhibited pulsovar 2 based on PFGE analysis, while they were well separated into different unrelated clusters using cgSNV and CRISPR analysis. S. Heidelberg isolates within the outbreaks exhibited 0–4 SNVs differences between each other while the nearest NDCs to these outbreak groups differed by 0–10 SNVs. Until recently, it was difficult to link all the outbreaks cases using PFGE. Our findings suggest that the cgSNV analysis found NDCs that can probably be part of these outbreaks. Additionally, the analysis of different clusters showed that number of outbreaks were probably underestimated when using traditional typing methods because the epidemiological evidence to link these isolates was not available.
Investigation of spacer diversity revealed 11 different CRISPR profiles. The majority of the identified cgSNV clusters displayed CP1 and the remaining clusters exhibited different CRISPR profiles except for those sharing CP4. Our findings revealed that CP1 and CP4 might represent the predominant CRISPR type circulating among human and poultry S. Heidelberg isolates in 2012. It is tempting to suggest that these CRISPR types may serve as a guide for future prevention and surveillance programs. Furthermore, the identification of CP1, CP2, CP3, and CP4 in both poultry products and human isolates demonstrates the probable transmission of strains carrying these CRISPR types from poultry to human. No significant association between food type and CRISPR profile was observed, likely due to the fact that the analyzed isolates provided came from one source type (poultry). Previous study on Salmonella enterica serovar Enteritidis showed that different CRISPR profiles may circulate between food from different animal sources (duck and pig) (Li et al., 2018). Further studies including different animal sources of Salmonella serovars are needed to elucidate this aspect.
We previously demonstrated that CRISPR typing alone was less discriminatory compared to cgSNV (Vincent et al., 2018). The presence of identical CRISPR profiles among food, outbreak, non-outbreak isolates and unrelated clusters confirms the limitation of CRISPR subtyping in the investigation of outbreaks and food source tracking. However, some NDCs, food isolates and clusters genetically related by cgSNV could be separated based on the CRISPR typing. Therefore, CRISPR analysis can be used as a complementary approach for not only Salmonella foodborne outbreaks subtyping, but also for food source tracking.
Rapidly linking clinical isolates and possible food sources, during epidemiological investigation of outbreaks, is critical to eradicate the source(s) of the outbreaks and thereby limit its impact (Barco et al., 2013; Pires et al., 2014). In the current study, the food history of the patients was not available to suggest any food sources for sampling and testing. Nonetheless, we have been able to trace the potential source of a 2012 epidemiologically well-characterized foodborne outbreak and NDCs involving S. Heidelberg in Quebec. The combined cgSNV/CRISPR approaches were able to match and exclude food isolates from different clinical isolates clusters. The clustering of isolates from humans and food sources implicated poultry products as source for human infections. Our analysis is consistent with the results obtained by CIPARS where the chicken sources accounted for the majority (81%) of S. Heidelberg isolates, and of these, 76% were from retail chicken meat (Canadian Integrated Program for Antimicrobial Resistance Surveillance [CIPARS], 2015). However, more studies on the food survey data are needed to confirm our speculation.
The transmission of S. Heidelberg isolates from an environmental source to a food product vehicle and ultimately to humans is possible as several abattoir isolates were genetically related (0–10 SNVs) to food and human isolates based on both cgSNV and CRISPR typing. This confirms that the poor food-handling can be an important factor of transmission and cross contamination. Most often, public health stakeholders attribute foodborne outbreaks to one animal source during outbreaks investigations. Our analysis, however, showed that cross contamination due to multiple animal sources may also occur during food poisoning incidents, this is the case of the turkey and chicken isolates identified in the CL10. This finding may also indicate a potential risk of infection from inadequately handled poultry products (Jain et al., 2008; Griffith, 2013). Our analysis also revealed that the same strain from the same cluster could be found in different poultry product types in a given year and may be recovered at varying time intervals. These findings not only suggest a high environmental stability of some S. Heidelberg isolates but also that common contamination sources along the food production chain may favor the circulation of any given isolate for a long period.
Conclusion
The cgSNV method is a highly discriminatory method for the resolution of clusters and outbreak events and the attribution of these events to their respective contaminating sources. The faster CRISPR typing can be useful for source tracking as well as serve as a complimentary tool to the cgSNV analysis during source attribution. This multi-disciplinary and multi-jurisdictional approach underscores the importance of using an integrated surveillance for outbreak investigations and source attribution. Although our findings are based on a limited number of food sources, our study, however, provides a potential tool to help identify sources of foodborne S. Heidelberg outbreaks especially if they are correlated with epidemiological data.
Data Availability Statement
The datasets generated for this study can be found in the PRJNA541551.
Ethics Statement
This study uses strains isolated from humans and obtained in the context of provincial surveillance program. Laboratoire de Santé Publique du Québec did not require the study to be reviewed or approved by an ethics committee because strains are obtained routinely for surveillance purpose and their secondary use do not require ethical study.
Author Contributions
SB, KY, and VU conceptualized and designed the work. KY and VU wrote the manuscript, conducted the data analysis, interpretation and explored the core of the topic. MM, DT, and SM contributed to the methodology of the work by providing PFGE and CRISPR data, respectively. EF provided bioinformatics support. SB, CB, FD-B, LG, and CN supervised the project and provided the administrative resources. All authors critically revised and approved the final version of the manuscript.
Funding
This study was supported by a grant from Genome Canada under Award Number #8505. The funders had no role in study design, data collection and analysis, decision to publish, or preparation of the manuscript. SM holds the Canada Research Chair in Bacteriophages.
Conflict of Interest
The authors declare that the research was conducted in the absence of any commercial or financial relationships that could be construed as a potential conflict of interest.
Acknowledgments
We thank the Bacteriology Unit staff at the Laboratoire de Santé Publique du Québec and members of National Microbiology Laboratory’s Enteric Diseases Reference Services Unit for sequencing. We also thank Dr. Philippe Horvath for access to the DuPont CRISPR tools.
Supplementary Material
The Supplementary Material for this article can be found online at: https://www.frontiersin.org/articles/10.3389/fmicb.2020.01317/full#supplementary-material
References
Acheson, D., and Hohmann, E. L. (2001). Nontyphoidal salmonellosis. Clin. Infect. Dis 32, 263–269. doi: 10.1086/318457
Afgan, E., Baker, D., Batut, B., Van Den Beek, M., Bouvier, D., Cech, M., et al. (2018). The Galaxy platform for accessible, reproducible and collaborative biomedical analyses: 2018 update. Nucleic Acids Res. 46, W537–W544. doi: 10.1093/nar/gky379
Antunes, P., Mourao, J., Campos, J., and Peixe, L. (2016). Salmonellosis: the role of poultry meat. Clin. Microbiol. Infect. 22, 110–121. doi: 10.1016/j.cmi.2015.12.004
Arndt, D., Grant, J. R., Marcu, A., Sajed, T., Pon, A., Liang, Y., et al. (2016). PHASTER: a better, faster version of the PHAST phage search tool. Nucleic Acids Res. 44, W16–W21. doi: 10.1093/nar/gkw387
Bankevich, A., Nurk, S., Antipov, D., Gurevich, A. A., Dvorkin, M., Kulikov, A. S., et al. (2012). SPAdes: a new genome assembly algorithm and its applications to single-cell sequencing. J. Comput. Biol. 19, 455–477. doi: 10.1089/cmb.2012.0021
Barco, L., Barrucci, F., Olsen, J. E., and Ricci, A. (2013). Salmonella source attribution based on microbial subtyping. Int. J. Food Microbiol. 163, 193–203. doi: 10.1016/j.ijfoodmicro.2013.03.005
Bekal, S., Berry, C., Reimer, A., Van Domselaar, G., Beaudry, G., Fournier, E., et al. (2016). Usefulness of high-quality core genome single-nucleotide variant analysis for subtyping the highly clonal and the most prevalent Salmonella enterica serovar Heidelberg clone in the context of outbreak investigations. J. Clin. Microbiol. 54, 289–295. doi: 10.1128/JCM.02200-15
Biswas, A., Gagnon, J. N., Brouns, S. J., Fineran, P. C., and Brown, C. M. (2013). CRISPRTarget: bioinformatic prediction and analysis of crRNA targets. RNA Biol. 10, 817–827. doi: 10.4161/rna.24046
Canadian Integrated Program for Antimicrobial Resistance Surveillance [CIPARS] (2015). Canadian Integrated Program for Antimicrobial Resistance Surveillance (CIPARS) report 2012. Annual Report, Chapter 4-Integrated Findings, and Discussion. Guelph, ON: Government of Canada. Public Health Agency of Canada.
Deng, X., Shariat, N., Driebe, E. M., Roe, C. C., Tolar, B., Trees, E., et al. (2015). Comparative analysis of subtyping methods against a whole-genome-sequencing standard for Salmonella enterica serotype Enteritidis. J. Clin. Microbiol. 53, 212–218. doi: 10.1128/JCM.02332-14
Edirmanasinghe, R., Finley, R., Parmley, E. J., Avery, B. P., Carson, C., Bekal, S., et al. (2017). A whole-genome sequencing approach to study cefoxitin-resistant Salmonella enterica serovar Heidelberg isolates from various sources. Antimicrob. Agents Chemother. 61:e001919-16. doi: 10.1128/AAC.01919-16
Francisco, A. P., Vaz, C., Monteiro, P. T., Melo-Cristino, J., Ramirez, M., and Carrico, J. A. (2012). PHYLOViZ: phylogenetic inference and data visualization for sequence based typing methods. BMC Bioinformatics 13:87. doi: 10.1186/1471-2105-13-87
Fu, S., Hiley, L., Octavia, S., Tanaka, M. M., Sintchenko, V., and Lan, R. (2017). Comparative genomics of Australian and international isolates of Salmonella Typhimurium: correlation of core genome evolution with CRISPR and prophage profiles. Sci. Rep. 7:9733. doi: 10.1038/s41598-017-06079-1
Garneau, J. E., Dupuis, M. E., Villion, M., Romero, D. A., Barrangou, R., Boyaval, P., et al. (2010). The CRISPR/Cas bacterial immune system cleaves bacteriophage and plasmid DNA. Nature 468, 67–71. doi: 10.1038/nature09523
Garrison, E., and Marth, G. (2012). Haplotype-based variant detection from short-read sequencing. arXiv [Preprint] Available online at: https://arxiv.org/abs/1207.3907 (accessed July, 2012).
Griffith, C. (2013). “Advances in understanding the impact of personal hygiene and human behaviour on food safety,” in Advances in Microbial Food Safety, Ed. J. N. Sofos (Amsterdam: Elsevier), 401–416. doi: 10.1533/9780857098740.5.401
Grissa, I., Vergnaud, G., and Pourcel, C. (2007). CRISPRFinder: a web tool to identify clustered regularly interspaced short palindromic repeats. Nucleic Acids Res. 35, W52–W57. doi: 10.1093/nar/gkm360
Guindon, S., Dufayard, J. F., Lefort, V., Anisimova, M., Hordijk, W., and Gascuel, O. (2010). New algorithms and methods to estimate maximum-likelihood phylogenies: assessing the performance of PhyML 3.0. Syst. Biol. 59, 307–321. doi: 10.1093/sysbio/syq010
Hoffmann, M., Zhao, S., Pettengill, J., Luo, Y., Monday, S. R., Abbott, J., et al. (2014). Comparative genomic analysis and virulence differences in closely related Salmonella enterica serotype heidelberg isolates from humans, retail meats, and animals. Genome Biol. Evol. 6, 1046–1068. doi: 10.1093/gbe/evu079
Jain, S., Chen, L., Dechet, A., Hertz, A. T., Brus, D. L., Hanley, K., et al. (2008). An outbreak of enterotoxigenic Escherichia coli associated with sushi restaurants in Nevada, 2004. Clin. Infect. Dis. 47, 1–7. doi: 10.1086/588666
Kurtz, S., Phillippy, A., Delcher, A. L., Smoot, M., Shumway, M., Antonescu, C., et al. (2004). Versatile and open software for comparing large genomes. Genome Biol. 5:R12. doi: 10.1186/gb-2004-5-2-r12
Li, H., Handsaker, B., Wysoker, A., Fennell, T., Ruan, J., Homer, N., et al. (2009). The Sequence Alignment/Map format and SAMtools. Bioinformatics 25, 2078–2079. doi: 10.1093/bioinformatics/btp352
Li, Q., Wang, X., Yin, K., Hu, Y., Xu, H., Xie, X., et al. (2018). Genetic analysis and CRISPR typing of Salmonella enterica serovar Enteritidis from different sources revealed potential transmission from poultry and pig to human. Int. J. Food Microbiol. 266, 119–125. doi: 10.1016/j.ijfoodmicro.2017.11.025
Majowicz, S. E., Musto, J., Scallan, E., Angulo, F. J., Kirk, M., O’brien, S. J., et al. (2010). The global burden of nontyphoidal Salmonella gastroenteritis. Clin. Infect. Dis. 50, 882–889. doi: 10.1086/650733
Minevich, G., Park, D. S., Blankenberg, D., Poole, R. J., and Hobert, O. (2012). CloudMap: a cloud-based pipeline for analysis of mutant genome sequences. Genetics 192, 1249–1269. doi: 10.1534/genetics.112.144204
Nadon, C., Van Walle, I., Gerner-Smidt, P., Campos, J., Chinen, I., Concepcion-Acevedo, J., et al. (2017). PulseNet International: vision for the implementation of whole genome sequencing (WGS) for global food-borne disease surveillance. Euro. Surveill. 22:30544.
National Enteric Surveillance Program [NESP] (2018). National Enteric Surveillance Program Annual Summary (Nesp) 2017. Guelph: Government of Canada. Public Health Agency of Canada.
Petkau, A., Mabon, P., Sieffert, C., Knox, N. C., Cabral, J., Iskander, M., et al. (2017). SNVPhyl: a single nucleotide variant phylogenomics pipeline for microbial genomic epidemiology. Microb Genom. 3:e000116. doi: 10.1099/mgen.0.000116
Pires, S. M., Vieira, A. R., Hald, T., and Cole, D. (2014). Source attribution of human salmonellosis: an overview of methods and estimates. Foodborne Pathog. Dis. 11, 667–676. doi: 10.1089/fpd.2014.1744
Rabsch, W., Tschape, H., and Baumler, A. J. (2001). Non-typhoidal salmonellosis: emerging problems. Microbes Infect. 3, 237–247. doi: 10.1016/S1286-4579(01)01375-2
Shariat, N., and Dudley, E. G. (2014). CRISPRs: molecular signatures used for pathogen subtyping. Appl. Environ. Microbiol. 80, 430–439. doi: 10.1128/AEM.02790-13
Shariat, N., Timme, R. E., Pettengill, J. B., Barrangou, R., and Dudley, E. G. (2015). Characterization and evolution of Salmonella CRISPR-Cas systems. Microbiology 161, 374–386. doi: 10.1099/mic.0.000005
Tenover, F. C., Arbeit, R. D., Goering, R. V., Mickelsen, P. A., Murray, B. E., Persing, D. H., et al. (1995). Interpreting chromosomal DNA restriction patterns produced by pulsed-field gel electrophoresis: criteria for bacterial strain typing. J. Clin. Microbiol. 33, 2233–2239. doi: 10.1128/jcm.33.9.2233-2239.1995
Thomas, M. K., Murray, R., Flockhart, L., Pintar, K., Pollari, F., Fazil, A., et al. (2013). Estimates of the burden of foodborne illness in Canada for 30 specified pathogens and unspecified agents, circa 2006. Foodborne Pathog. Dis. 10, 639–648. doi: 10.1089/fpd.2012.1389
Vincent, C., Usongo, V., Berry, C., Tremblay, D. M., Moineau, S., Yousfi, K., et al. (2018). Comparison of advanced whole genome sequence-based methods to distinguish strains of Salmonella enterica serovar Heidelberg involved in foodborne outbreaks in Quebec. Food Microbiol. 73, 99–110. doi: 10.1016/j.fm.2018.01.004
Keywords: source attribution, Salmonella enterica serovar Heidelberg, core genome SNV, CRISPR, PFGE, foodborne outbreaks, genomic typing
Citation: Yousfi K, Usongo V, Berry C, Khan RH, Tremblay DM, Moineau S, Mulvey MR, Doualla-Bell F, Fournier E, Nadon C, Goodridge L and Bekal S (2020) Source Tracking Based on Core Genome SNV and CRISPR Typing of Salmonella enterica Serovar Heidelberg Isolates Involved in Foodborne Outbreaks in Québec, 2012. Front. Microbiol. 11:1317. doi: 10.3389/fmicb.2020.01317
Received: 18 February 2020; Accepted: 25 May 2020;
Published: 17 June 2020.
Edited by:
Arun K. Bhunia, Purdue University, United StatesReviewed by:
Edward G. Dudley, Pennsylvania State University, United StatesJie Zheng, United States Food and Drug Administration, United States
Copyright © 2020 Yousfi, Usongo, Berry, Khan, Tremblay, Moineau, Mulvey, Doualla-Bell, Fournier, Nadon, Goodridge and Bekal. This is an open-access article distributed under the terms of the Creative Commons Attribution License (CC BY). The use, distribution or reproduction in other forums is permitted, provided the original author(s) and the copyright owner(s) are credited and that the original publication in this journal is cited, in accordance with accepted academic practice. No use, distribution or reproduction is permitted which does not comply with these terms.
*Correspondence: Sadjia Bekal, sadjia.bekal@inspq.qc.ca
†These authors share first authorship