- 1Institute of Zoology, Zoological Society of London, London, United Kingdom
- 2Centre for Ecology and Conservation, University of Exeter, Penryn, United Kingdom
- 3MRC Centre for Global Infectious Disease Analysis, School of Public Health, Imperial College London, London, United Kingdom
- 4School of Science, Engineering and Environment, University of Salford, Salford, United Kingdom
Host-associated microbes form an important component of immunity that protect against infection by pathogens. Treating wild individuals with these protective microbes, known as probiotics, can reduce rates of infection and disease in both wild and captive settings. However, the utility of probiotics for tackling wildlife disease requires that they offer consistent protection across the broad genomic variation of the pathogen that hosts can encounter in natural settings. Here we develop multi-isolate probiotic consortia with the aim of effecting broad-spectrum inhibition of growth of the lethal amphibian pathogen Batrachochytrium dendrobatidis (Bd) when tested against nine Bd isolates from two distinct lineages. Though we achieved strong growth inhibition between 70 and 100% for seven Bd isolates, two isolates appeared consistently resistant to inhibition, irrespective of probiotic strategy employed. We found no evidence that genomic relatedness of the chytrid predicted similarity of inhibition scores, nor that increasing the genetic diversity of the bacterial consortia could offer stronger inhibition of pathogen growth, even for the two resistant isolates. Our findings have important consequences for the application of probiotics to mitigate wildlife diseases in the face of extensive pathogen genomic variation.
Introduction
Emerging infectious diseases pose a significant threat to human and wildlife health, and are responsible for driving declines in biodiversity around the globe (Jones et al., 2008; Tompkins et al., 2015; Scheele et al., 2019). The last few decades alone have witnessed the emergence and spread of multiple viral and fungal pathogens capable of infecting a broad range of host species, and developing strategies to mitigate the loss of wildlife species caused by disease is now a key priority. The use of probiotics, where hosts are exposed to microbes with known host-protective properties, represent a promising tool for tackling infectious disease (McKenzie et al., 2018). To date, probiotics have been applied in the fields of human medicine (Kerry et al., 2018), wildlife medicine (Kueneman et al., 2016), aquaculture (Tan et al., 2016) and both arable (Marcano et al., 2016) and livestock (Mingmongkolchai and Panbangred, 2018) agriculture, with varying success. Developing effective probiotics is complicated by the fact that distinct lineages of a pathogen exhibit markedly different susceptibilities to the same bacterial assemblages (Antwis et al., 2015; Antwis and Weldon, 2017; Muletz-Wolz et al., 2017; Antwis and Harrison, 2018). A bacterial probiotic that is inhibitory for one variant of a pathogen can promote growth of a different variant (e.g., Antwis and Harrison, 2018). Therefore, probiotics comprising single bacterial species appear unlikely to be provide a “silver bullet” capable of inhibition across the broad spectrum of pathogen variants observed in natural systems. This issue is exacerbated by the propensity of pathogen genotypes to spread rapidly around the globe, often as a result of human activity (O’Hanlon et al., 2018), exposing susceptible hosts to multiple and novel threats (Greenspan et al., 2018; Longo et al., 2019). Therefore, designing novel probiotic strategies that are effective against the full panorama of pathogen genotypes is necessary in order to effect meaningful change in the rates of infection caused by emerging infectious diseases.
Recent evidence suggests that the shortcomings of single species probiotics can be overcome by administering multiple bacterial isolates in a mixed community, referred to as a consortium (e.g., Piovia-Scott et al., 2017; Antwis and Harrison, 2018). However, quantitative estimates of the ability of multi-species consortia to elicit wide-spectrum inhibition of wildlife pathogens is scarce. Here, we test the ability of multi-isolate bacterial consortia to inhibit isolates of the chytrid fungus Batrachochytrium dendrobatidis (Bd), which causes the lethal disease chytridiomycosis in amphibians (Lips, 2016). Chytridiomycosis has resulted in the decline of over 500 amphibian species of the last 50 years (Scheele et al., 2019), and remains a major threat to amphibian biodiversity around the globe (Lips, 2016; O’Hanlon et al., 2018; Scheele et al., 2019). Application of bacterial probiotics can reduce the growth of Bd in vitro (Antwis et al., 2015; Muletz-Wolz et al., 2017; Piovia-Scott et al., 2017; Antwis and Harrison, 2018) and reduce Bd-associated mortality in vivo (Kueneman et al., 2016). Previous work has highlighted that constructing probiotic consortia that maximize the genetic distance among bacterial isolates elicits stronger inhibition against Bd than consortia containing the same number of more closely related isolates (Antwis and Harrison, 2018). These data suggest that the emergent functional properties of a probiotic consortium, in this case pathogen inhibition, may be a function of overall genetic diversity rather than species diversity (Piovia-Scott et al., 2017) and resonates with biodiversity-function relationships studies in macroecology (e.g., Brophy et al., 2017, see also Koskella et al., 2017). Maximizing intra-consortium genetic diversity may therefore increase the probability of obtaining broad-spectrum inhibition across a diverse suite of pathogen genotypes and minimize the impact of lethal wildlife diseases. Moreover, selecting bacteria that exhibit strong inhibitory capabilities individually may maximize the potential for broad-spectrum inhibition across pathogen genotypes when combined in a consortium.
Here we examine patterns of inhibition of both individual bacterial isolates and consortia tested against a panel of nine isolates of Bd (Table 1), comprising eight isolates of the highly virulent Global Panzootic Lineage (BdGPL) and one isolate of the more distantly related BdCAPE (see O’Hanlon et al., 2018). We seek to test the following predictions: (i) Bd isolates will vary in their susceptibility to inhibition by individual bacterial isolates, but using bacterial consortia will reduce the among-isolate variation in inhibition; (ii) probiotic consortia with higher mean genetic diversity will more likely elicit broad-spectrum inhibition of multiple pathogen variants; and (iii) Bd isolates that are more genetically similar will have more similar inhibition profiles across the panel of isolates tested.
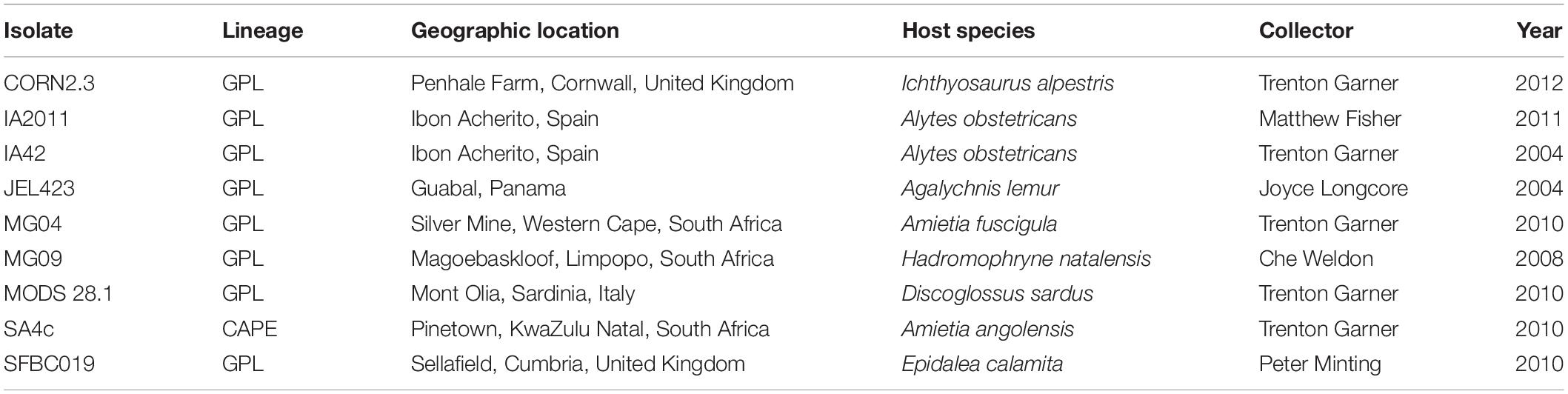
Table 1. Batrachochytrium dendrobatidis (Bd) strain information for nine isolates used in this study, comprising eight isolates of BdGPL, and one of BdCAPE.
Materials and Methods
Isolation of Bacteria and Probiotic Design
We isolated bacteria from the skin of wild Agalychnis spp. frogs at Las Cuevas Research Station in the Maya Mountains of Belize by permission of the Belize Forestry Department (Research and Export Permit Number CD/60/3/12; Antwis et al., 2015). Briefly, we swabbed frogs and streaked these out on R2A agar. Bacteria were left to grow at ambient temperature for eight days, and we picked individual colonies using sterile swabs, which were stored in R2A media and shipped to the United Kingdom (DEFRA Authorization Number TARP/2012/224). We confirmed purity of bacteria by re-streaking on R2A and used colony PCR to amplify the 16S rRNA gene (with primers 27F and 1492R), which were sequenced at the University of Manchester. We aligned the forward and reverse sequences for each bacterium and blasted these against the NCBI database1 to identify bacteria. We maintained frozen stocks of pure bacteria stored in 30% glycerol and 70% tryptone solution at −80°C, which were used for subsequent in vitro challenge assays.
For in vitro challenge assays, we used three bacteria from each of four genera (Acinetobacter spp., Chryseobacterium spp., Serratia spp., and Stentrophomonas spp.) based on preliminary screening of inhibitory capabilities; medium to strong inhibitors pf Bd’s growth were selected to maximize the potential inhibitory capabilities of consortia (Supplementary Table S1). We added 50 μl of the 12 bacteria (three cultures per bacterial strain) to 15 ml of 1% tryptone and incubated these at 18°C for 36 h until turbid. We did not adjust cell density prior to inhibition to avoid altering the metabolite profiles already produced by cultures through the addition of media.
Challenge Assays
For each probiotic, we added bacteria to 12 ml of fresh 1% tryptone broth and left these to incubate for 4 h. A total of 3 ml of bacteria were used for each probiotic, split evenly between the number of bacteria added to each group; either one (“single probiotic”), two (“double probiotic”), or three (“triple probiotic”). Double probiotics were always constructed from two unique congeneric bacterial isolates. Triple probiotics were constructed from three bacteria either within the same genus (“inter-genera triple probiotic,” total of four mixes) or across three different genera (“intra-genus triple probiotic,” total of four mixes; Supplementary Table S1).
Nine Bd isolates were used for in vitro challenges, isolated from a range of host species from Europe, Africa and Central America (Table 1; see Bd Sequencing below for additional information). These were grown in 1% tryptone broth until maximum zoospore production was observed (∼3 days; ∼1 × 106 zoospores ml–1). Three flasks per Bd isolate were grown and combined for in vitro challenges. Bd zoospores were separated from sporangia by filtering through 20 μl sterile filters (Millipore, Ireland). Bacterial probiotics were filtered (0.22 μm sterile filter; Millipore, Ireland) to isolate bacterial products in the filtrate, which were kept on ice until Bd challenges were conducted.
To conduct the in vitro challenge assays, 50 μl of bacterial filtrate and 50 μl of Bd suspension were combined in 96-well plates. Each Bd -bacteria combination was run in triplicate. Positive controls were included using 50 μl 1% tryptone instead of bacterial filtrate. Negative controls were included using 50 μl sterile tryptone and 50 μl of heat-treated Bd for each isolate. The optical density (OD) was measured at 492 nm filter on a spectrophotometer on initial construction of the challenge assays, and every 24 h after for four subsequent days. For each measurement, data were transformed using the equation Ln[OD/(1-OD)], and a regression analysis was used to gain the slope values for each sample over time (Antwis and Harrison, 2018). The slopes of the three replicates for each Bd-bacteria combination were averaged, and the slope of the negative controls subtracted. Total Bd inhibition was calculated using the formula: Inhibition (%) = [1−(slope of sample/slope of control)] × 100 to give an “inhibition score.” A positive inhibition score represents inhibition of Bd growth and a negative score indicates enhanced growth of Bd. We did not use a nutrient depleted control in our experiments (Bell et al., 2013), which means Bd inhibition relative to the controls may be slightly under-estimated.
Bd Sequencing
Extracted high molecular weight DNA of six Bd isolates were sequenced on an Illumina HiSeqTM platform (Illumina, CA, United States), which produced 125 + 125 bp paired-end sequenced reads using the Illumina HiSeqTM high output V4 chemistry. Data analysis was done using a previously defined Bd specific alignment pipeline and stringent variant discovery conditions (O’Hanlon et al., 2018). A pairwise Bd relatedness matrix was generated in R by comparing genotype calls across all segregating sites in the isolate collection (185290 segregating sites).
Statistical Analysis
All statistical analyses, code and data files are provided as a supplementary R Markdown document to allow full reproducibility of results. All statistical analyses were conducted in the software R v.3.5.3 (R Core Team, 2019). We used the Bayesian mixed effects modeling package brms (Bürkner, 2017, 2018) to fit statistical models. We assessed convergence of models based on R-hat values, and visually inspected chains to ensure adequate mixing. We used an information criterion based on leave-one-out cross validation (LOO-IC; Vehtari et al., 2017), calculated by the loo package (Vehtari et al., 2019) called via brms to select among candidate models, and present mean parameter estimates of effects and 95% credible intervals as appropriate. LOO-IC values presented represent comparison to the null model.
Patterns of Inhibition Across Consortium Types and Bd Isolates: To examine the effects of probiotic type (single isolate, double isolate, etc.), Bd isolate and bacterial genus on inhibition scores, we fitted a linear mixed effects model (LMM) with inhibition as the response, probiotic type as a fixed effect, and Bd isolate and bacterial genus as random intercepts. As the dataset contained both positive and negative inhibition values, where a negative value represents facilitation of Bd growth, we used a Gaussian error structure to avoid having to remove the informative negative values. Ideally, we would have fitted a 2-stage Hurdle model to such data, simultaneously modeling the factors influencing the probability of Bd growth facilitation, and the magnitude of Bd inhibition | a positive inhibition value. However, these models were regrettably too complex for this dataset as the negative values were sparse and clustered within certain Bd isolates. Using a Gaussian model allowed us to capture these same patterns in a simpler model. We used the R package pheatmap (Kolde, 2019) version 1.0.12 to visualize differences in Bd inhibition across all probiotics tested.
Bd Relatedness and Inhibition
To assess whether more closely related Bd isolates had more similar inhibition profiles, we conducted a Mantel test on a matrix of pairwise relatedness values among the six Bd isolates for which we had genomic data, and a pairwise matrix where each cell value represented the correlation between the inhibition profiles for each pair of Bd isolates. To build a tree of Bd isolates, we used hierarchical clustering of genetic distance values using the “upgma” function in the phangorn package (Schliep, 2011; Schliep et al., 2017) in R.
Multi-Isolate Probiotics and Broad-Spectrum Bd Inhibition
To calculate genetic distance among bacterial isolates, we aligned the 16S rRNA gene sequences against the SILVA v132 references alignment (Quast et al., 2013; Yilmaz et al., 2014) using mothur 1.39.5 (Schloss et al., 2009). We used the seqinr package (Charif and Lobry, 2007) to read the alignment into R and calculate inter-sequence distance. To examine the effect of mean genetic distance among consortium members on Bd inhibition, we fitted a LMM with Bd inhibition as a response, both Bd isolate and probiotic genus as random intercepts, and genetic distance as a random slope given Bd isolate (i.e., Bd-specific slopes for the effect of probiotic genetic distance). As so many inhibition values were near the upper bound (100% inhibition), we logit transformed inhibition scores after converting % scores to the scale (0,1), and used a Gaussian error structure (Warton and Hui, 2011).
Results
Patterns of Inhibition Across Consortium Types and Bd Isolates
There was strong variation in patterns of inhibition among both probiotic types (Figures 1A,B) and among Bd isolates within probiotic types (Figure 1B). When controlling for the effects of Bd isolate identity and bacterial genus in a LMM, single-isolate probiotics exhibited the lowest average inhibition scores (effect of probiotic type; ΔLOO = −14.3; Figures 1A,B). The model explained 33.1% (95% credible interval, 24.1% to 41.1%) of the variance in inhibition scores overall. Considering only the random effects, variance component analysis indicated that Bd isolate explained slightly more variance than bacterial genus [Bd mean (95% CI) = 28.4% (16.1–40.7); bacterial genus mean = 21.1% (7.9–36.3)].
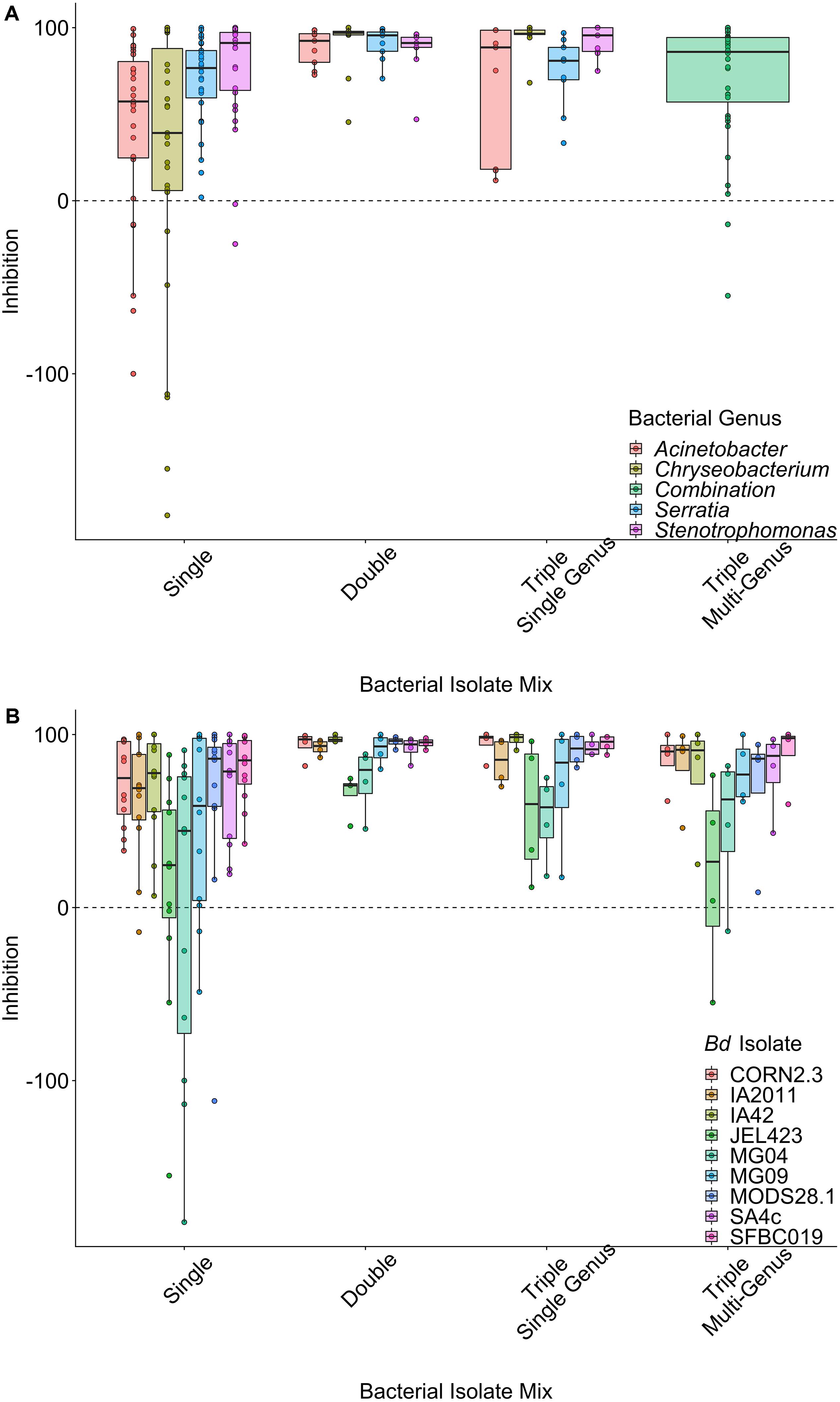
Figure 1. Raw data plots of inhibition scores split by probiotic type, grouped by (A) bacterial genus averaged over B. dendrobatidis (Bd) isolates and (B) Bd isolates averaged over bacterial genus. Data are displayed as boxplots with raw data points overlaid. Positive values (above the dashed line) indicate inhibition of Bd growth, whereas negative values indicate that Bd grows faster in the presence of the probiotic. There is clear variation in inhibition strength across Bd isolates and probiotic types.
We also detected substantially lower average inhibition for two of the nine Bd isolates (JEL423 and MG04) irrespective of the probiotic type applied (Figure 2 and Supplementary Figure S1). Hierarchical clustering also identified these two Bd isolates as having divergent inhibition profiles compared to other seven isolates (Figure 2). We found no evidence that more closely related Bd isolates had more similar inhibition profiles across the panel of probiotics tested (Mantel test p = 0.6; Figures 3A,B), although the two Bd isolates most resistant to inhibition were relatively genetically similar (both isolates were BdGPL JEL423/MG04 with a pairwise relatedness = 0.89; Figure 3A).
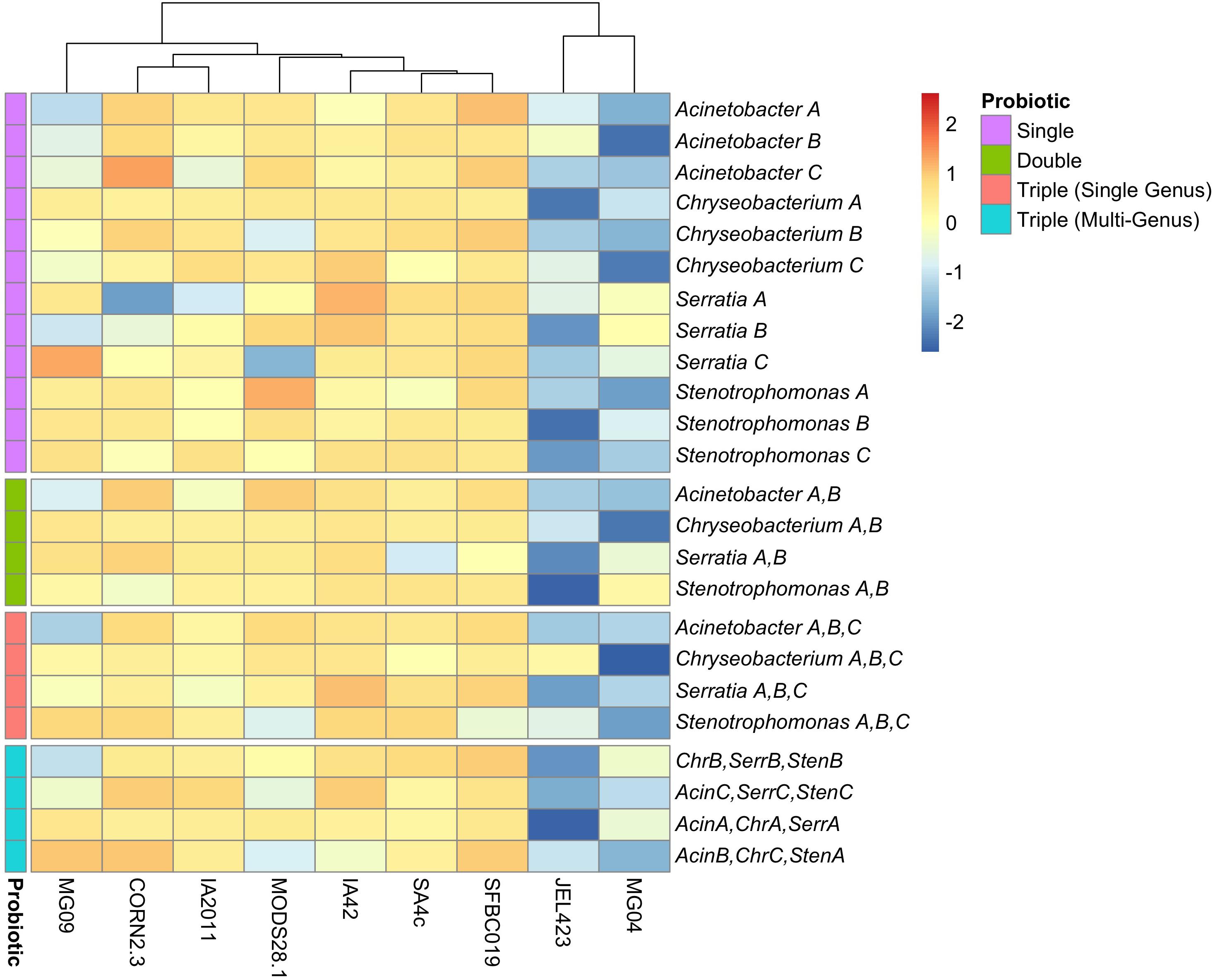
Figure 2. Heatmap of inhibition scores across nine Bd isolates for all probiotic types. Rows are ordered based on increasing probiotic complexity, from single bacterial isolate to multi-genus triple-isolate probiotics. Probiotics are color-coded according to complexity (see “Probiotic” legend). Columns have been clustered according to similarity of inhibition profiles among Bd isolates. Data are scaled by row to permit comparison of inhibition strength across Bd isolates for each probiotic. Multi-isolate probiotic abbreviations: Chr, Chryseobacterium; Acin, Acinetobacter; Serr, Serratia; Sten, Stenotrophomonas.
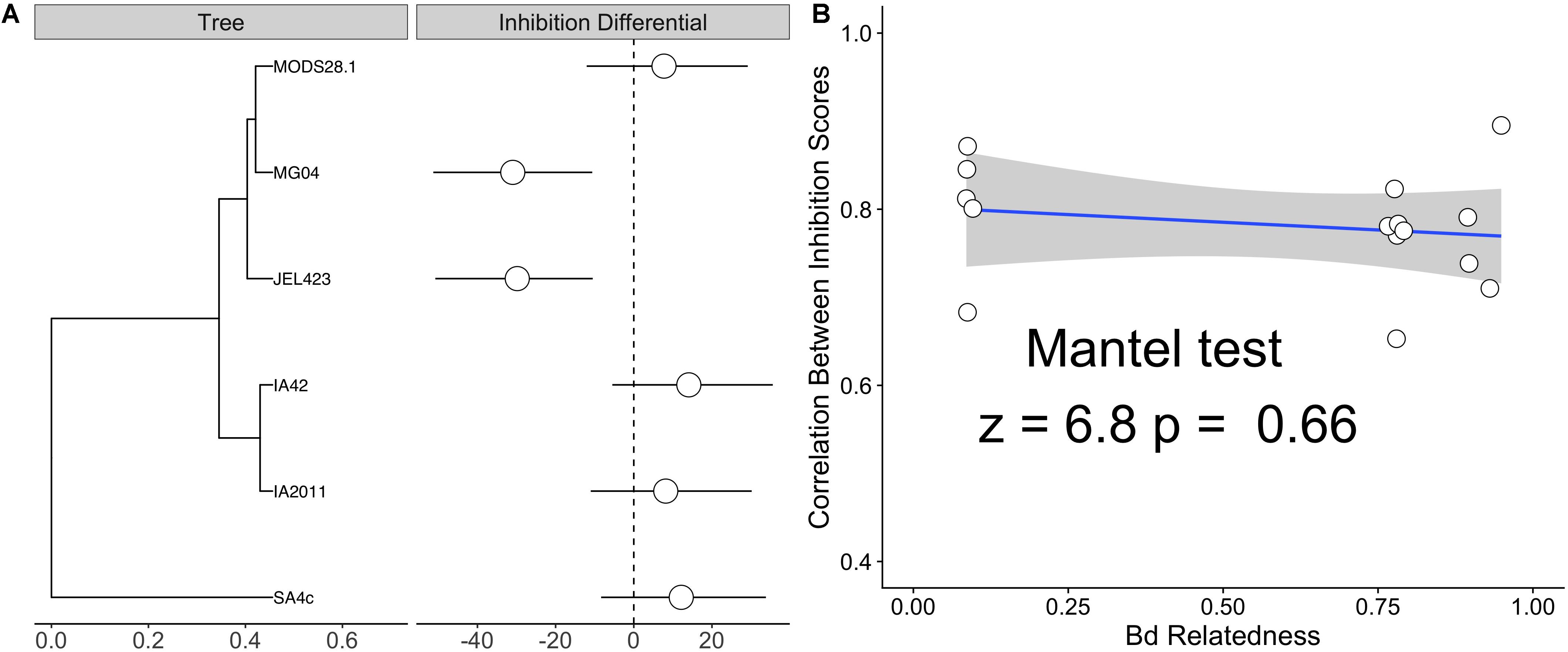
Figure 3. (A) Phylogenetic tree of six sequenced Bd isolates alongside isolate-specific inhibition differential – defined as the departure from the global mean inhibition value from a Bayesian linear mixed effects model (LMM) with a Bd random effect. Estimates are marginalized with respect to bacterial genus of probiotic isolates. Both JEL423 and MG04 exhibit 95% credible intervals not crossing zero, indicative of lower average inhibition potential compared to other isolates. (B) Correlation plot between the average pairwise relatedness between Bd isolates and the correlation between their inhibition scores for the panel of tested probiotics (listed in Figure 2). A Mantel test indicated no obvious relationship between inter-isolate genetic distance and similarity of inhibition profiles (p = 0.6).
Multi-Isolate Probiotics and Broad-Spectrum Bd Inhibition
We found that consortia containing isolates from three different bacterial genera had higher average between-isolate distance than those containing two or three bacterial isolates from the same genus (Supplementary Figure S2). However, there was no evidence that higher average genetic distance among isolates in a probiotic resulted in stronger inhibition when controlling for Bd isolate and bacterial genus [effect of genetic distance mean (95% CI) = −2.77 (−10.99,5.09); ΔLOO effect of genetic distance = −0.2; Figure 4].
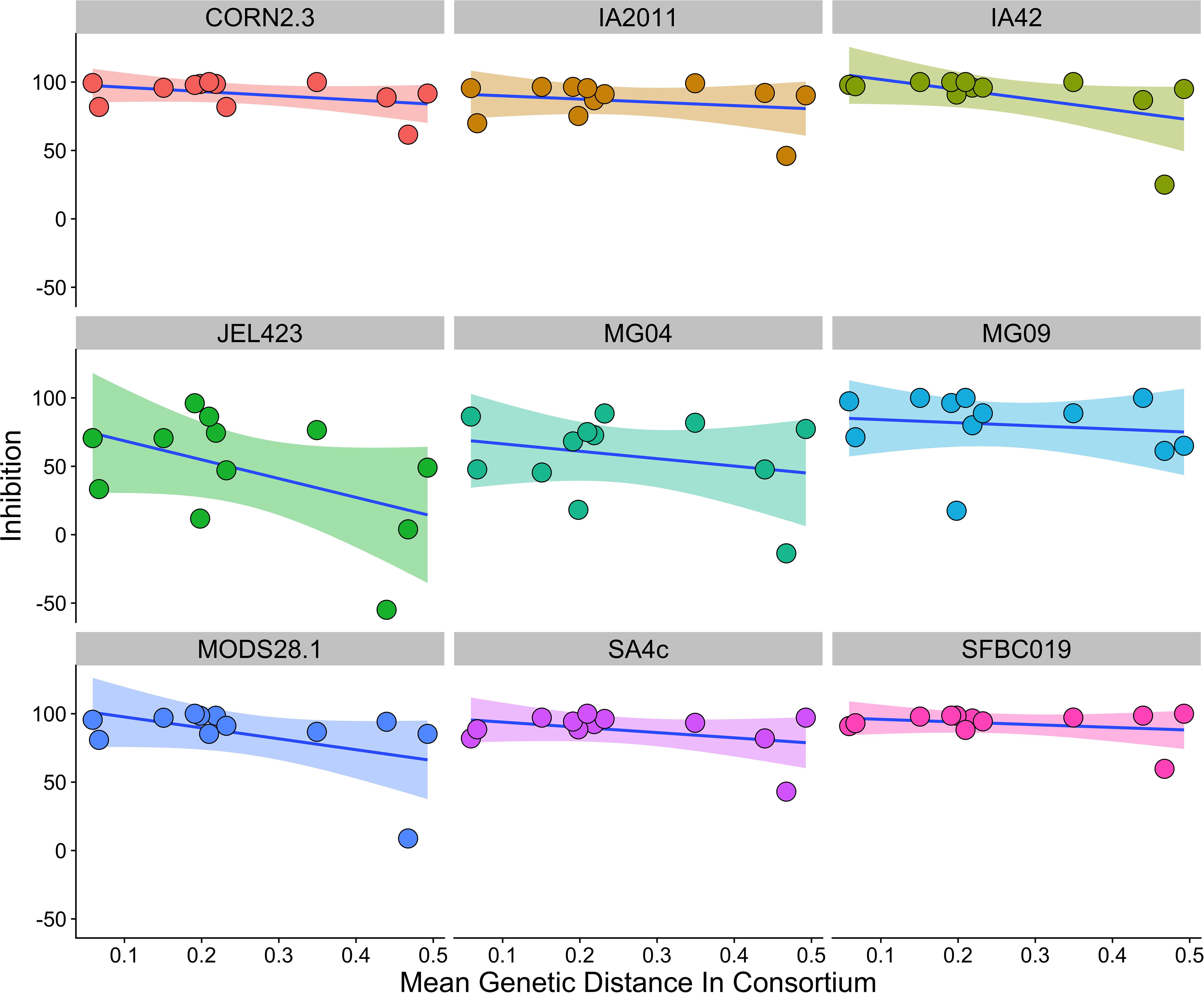
Figure 4. Relationship between average genetic distance of members of a multi-isolate probiotic (2 isolates or more) and inhibition of Bd, split by Bd isolate identity. There was no evidence of a relationship between genetic distance and inhibition when controlling for probiotic ID, bacterial genus and Bd isolate identity. A linear trend line is provided for illustration.
Considering only consortia constructed using bacterial isolates from a single genus, we detected no consistent effect of increasing probiotic complexity from one to three isolates on overall inhibition score [effect of number of isolates, mean (95% CI) = 3.66 (−0.86; 8.06); Figure 5A]. Conversely, variance component analysis revealed strong variation among both bacterial genera and Bd isolates in determining overall inhibition [percentage variance explained (95% CI): Bd = 34.96 (19.3–51.3); bacterial genus = 21.68 (2.73–44.6)]. The high variance among Bd isolates is evidenced by three Bd isolates (JEL423, MG04, and MG09) displaying highly unstable inhibition dynamics as probiotic complexity increased (Figure 5A). Whereas for most Bd isolates, increasing probiotic complexity yielded higher or stable inhibition scores, for JEL423, MG04, MG09 the addition of bacterial isolates appeared to cause decreases in average inhibition for two (JEL423 and MG09) and three (MG04) of the bacterial consortia, respectively. Consistent with this, there was a negative correlation across all Bd isolates between the mean inhibition and variance in inhibition across genera for three-isolate probiotics (Spearman’s rho = −0.93, p < 0.001; Figure 5A).
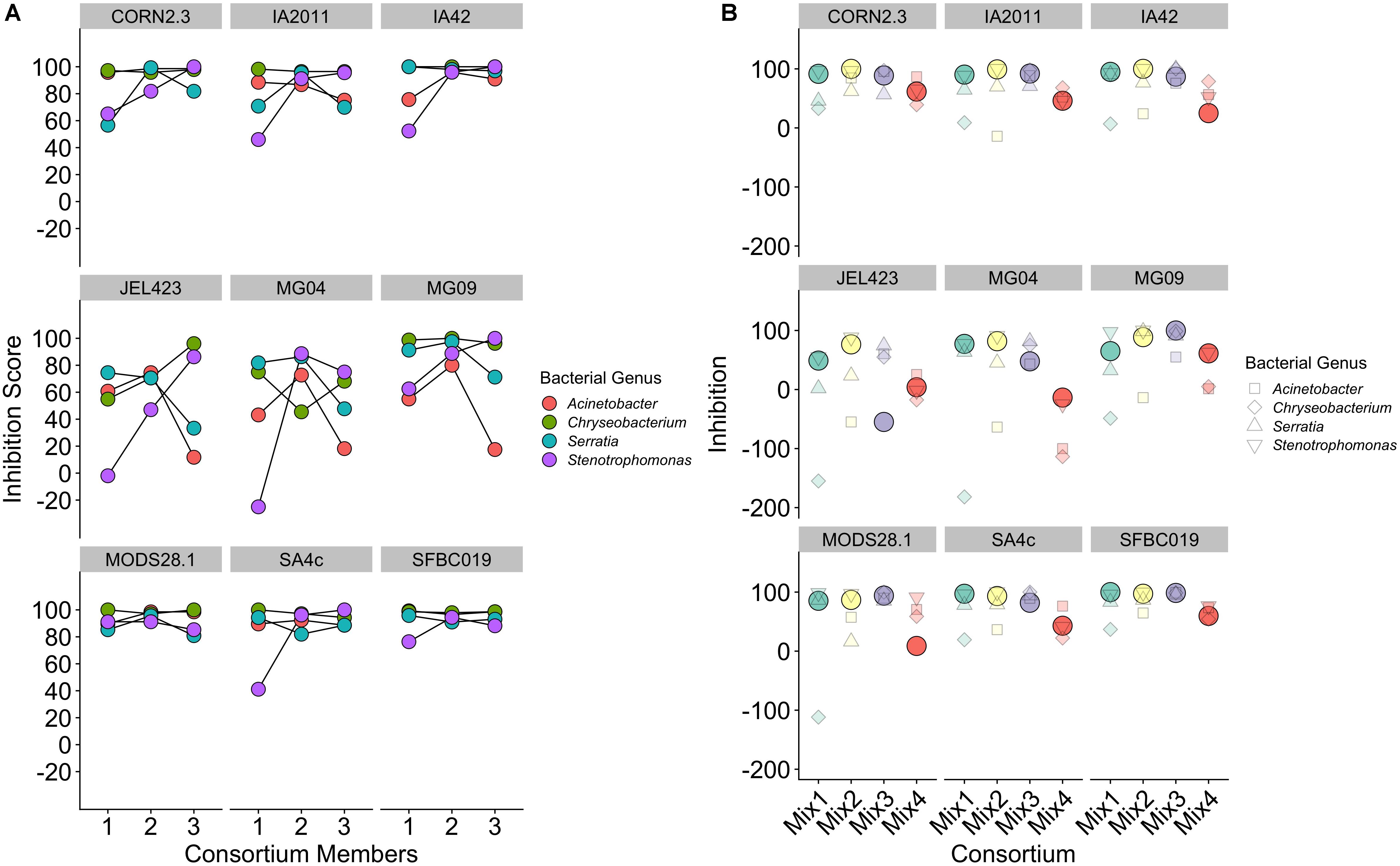
Figure 5. (A) Inhibition scores for single-genus probiotics of increasing complexity from single to three-isolate consortia, split by Bd isolate. (B) Inhibition scores for three-member probiotic consortia containing isolates from three different bacteria genera. Solid circles indicate the inhibition score for a consortium for each Bd. Smaller shapes show the individual inhibition scores of the three single isolates that comprise each consortium, with shape corresponding to bacterial isolate genus.
Considering only probiotics constructed using bacterial isolates from multiple genera, in 72% of cases (26/36) the probiotic exhibited an inhibition score that was higher than the mean of the single-isolate inhibition scores for the isolates that probiotic contained (Figure 5B; Wilcoxon signed rank test, V = 514, p = 0.004). Only in 19% of cases (7/36) did the inhibition score exceed the score of the isolate with strongest inhibition in the probiotic (Wilcoxon signed rank rest V = 66.5, p = 1; Figure 5B). As for single-genus triple-isolate probiotics, we observed a negative correlation between the among-consortium variance in inhibition and mean inhibition across the nine Bd isolates (Spearman’s rho = −0.86, p < 0.005; Figure 5B).
Discussion
Our findings have revealed consistent variation among Bd isolates in their susceptibility to inhibition by potential bacterial probiotics. Whilst the growth of most isolates could be suppressed by between 70 and 100%, two of the isolates (JEL423 and MG04) appeared to resist inhibition irrespective of the probiotic type being employed. Consistent with previous research, treatments comprising just a single bacterial isolate performed worse on average when tested across a range of Bd isolates (Antwis et al., 2015; Muletz-Wolz et al., 2017; Antwis and Harrison, 2018). Employing multi-isolate treatments increased average inhibition, but contrary to expectation, increasing the genetic diversity of the consortium did not lead to increased inhibition values. These data have important ramifications for our ability to design probiotics that are consistently effective against the diverse genetic variants of Bd encountered in nature (Becker et al., 2017; O’Hanlon et al., 2018).
There is now growing evidence that multi-strain probiotic consortia offer both increased inhibition potential for individual Bd isolates (Piovia-Scott et al., 2017), and a higher probability of effecting broad-spectrum inhibition across multiple genomic variants of Bd, compared to probiotics comprising only a single bacterial isolate (Antwis and Harrison, 2018; this study). Using probiotics containing two or more isolates impeded Bd growth more strongly on average than single isolates. However, unlike a previous study (Antwis and Harrison, 2018), we found no support for our prediction that more genetically diverse probiotic consortia were more effective at constraining Bd growth. One explanation for this pattern is that the effectiveness of two-isolate consortia was already very high because consortia were constructed from isolates known to offer strong inhibition against one of more Bd isolates. It follows, therefore, that when inhibition by single bacterial isolates is weak, co-culturing genetically diverse bacteria can elicit much stronger inhibition (Antwis and Harrison, 2018). However, when variance in inhibition is compressed because average inhibition is already high, the genetic distance among consortium members is less important in determining inhibition strength. The corollary of this is that there will undoubtedly be variants of Bd even more resistant to treatment with probiotics not tested in this dataset, and in such cases where mean growth inhibition is lower (i.e., yielding more variance in the trait) we might expect the diversity-function relationship to re-emerge.
Though we observed an increase in mean inhibition when using two more bacterial isolates in a consortium, the dynamics of change in inhibition with increasing probiotic complexity were not uniform across isolates of Bd. For three isolates in particular (JEL423, MG04, and MG09), increasing the species-richness of the probiotic destabilized inhibition dynamics and increased variance in inhibition among bacterial genera. It is noteworthy that two of these isolates were those that were most resistant to inhibition; we observed the same negative mean-variance relationship for consortia comprising isolates from three bacterial genera as we did for single-genus consortia. Similarly, it was rare to observe inhibition values for triple consortia that exceeded that of the strongest single isolate inhibitor in the community, indicating no evidence for strong synergistic effects when co-culturing. Taken together, these results suggest that the emergent functional properties of these microbial communities, specifically the efficacy of the repertoire of anti-fungal metabolites they produce when co-cultured, vary in concert with the genotype of the pathogen. These patterns highlight the difficulties associated with predicting microbial community dynamics and function purely from species composition (Widder et al., 2016; Momeni et al., 2017; see also Koskella et al., 2017). Previous work using consortia to mitigate Bd has observed strong dominance effects in probiotic communities, where the species attaining highest relative abundance following co-culture is determined by the initial species composition (Piovia-Scott et al., 2017). Though we did not track relative abundance of individual community members, variation across the consortia we tested relative to their individual isolate inhibition scores likely reflects competition effects driving different members of each consortium to dominance (see Friedman et al., 2017). Clearly, increasing probiotic complexity can modify emergent function in either a beneficial or a detrimental way, depending on the Bd isolate used in the challenge. Therefore, the outcome of the competition changes with total community complexity (e.g., Piovia-Scott et al., 2017), which in turn modifies the utility of the consortium to depress Bd growth. Elucidating these complex relationships between microbial community diversity-function and pathogen genotype forms a critical component of our understanding of how to develop probiotics with maximum effectiveness in the face of enormous pathogen genomic variation. More broadly, it highlights the utility of the bacteria-Bd system for answering general questions about how community function scales with complexity in microbial assemblages.
We found no evidence that genetic similarity of Bd isolates predicted similarity of inhibition scores across the six isolates for which there were genomic data, comprising five BdGPL isolates and one BdCAPE. Muletz-Wolz et al. (2017) found that the inhibition profiles of eight Bd isolates clustered by phylogenetic relatedness, but tested a much larger phylogenetic breath of isolates including B. salimandrivorans (Bsal) and B. dendrobatidis-Brazil, which clustered together and separately from six BdGPL isolates. We detected no such distinction in our panel of Bds, where we might have expected the BdCAPE lineage to cluster independently of isolates belonging to BdGPL. Whilst our data do not support a linear trend between relatedness and inhibition, we cannot discount the possibility that there may be clusters of resistant Bd genotypes throughout the broader Bd phylogeny. Bd can rapidly evolve resistance to inhibition by antimicrobial peptides through changes in genomic traits such as chromosomal copy number (Farrer et al., 2013), and the presence of two inhibition-resistant isolates in our dataset may reflect prior selection against constraint of their growth by bacterial metabolites. Future work should seek to quantify susceptibility to inhibition over a much broader repertoire of Bd lineages, including Bsal, to test this possibility. It would be particularly interesting to investigate whether clusters of inhibition-resistant genotypes cluster spatially and/or align with particular environmental conditions. Recent in vitro work has highlighted that abiotic conditions such as temperature can both affect the efficacy of bacterial probiotics against Bd (Muletz-Wolz et al., 2017) and virulence traits of the pathogen such as growth rate of zoosporangia (Muletz-Wolz et al., 2019). These studies suggest that although we have achieved relatively consistent inhibition across multiple pathogen genomic variants in this study under controlled laboratory conditions, the true emergent properties of probiotics in nature will instead be function of pathogen genome, probiotic genome(s), and environment interactions (GxGxE), which makes predicting inhibition strength of these probiotics for novel Bd genotypes even more challenging.
In our study, we filtered metabolites derived from bacterial co-culture to be used in inhibition challenges against Bd. Though challenges of this nature provide compelling evidence of the ability of certain bacterially derived metabolites to prevent Bd growth, they are not necessarily accurate reflections of natural interactions among host-associated microbes, or between microbes and the pathogen. For example, the phenotypic traits of bacteria grown in planktonic form, as we have cultured them, can differ vastly from those same bacteria grown in biofilms (e.g., San-Wong et al., 2015). Likewise, our assays prevent us from quantifying how the strength of bacteria-Bd interactions may be modified by presence of physical contact between cells of the resident bacteria and the pathogen. One way to overcome these limitations for future work would be to use a biolfilm model of infection, which is ideally suited to studying both polymicrobial dynamics and pathogen interactions (Gabrilska and Rumbaugh, 2015). Such assays would provide more accurate estimates of competition and growth processes within bacterial communities, and permit physical interaction between bacterial biofilms and Bd. Concordant with our results, Piovia-Scott et al. (2017) used a biofilm model to demonstrate that the strength of inhibition of Bd is a function of increased bacterial community complexity (see also Antwis and Harrison, 2018). Future work should seek to quantify the metabolic phenotype of bacteria grown in biofilm conditions to identify the functional mechanism by which host-associated microbes can mitigate the proliferation of the pathogen.
In summary, developing effective probiotic strategies to mitigate wildlife disease is clearly complicated by the ability of the pathogen to evolve resistance to potential probiotics (Farrer et al., 2013), the modulation of pathogen virulence and probiotic effectiveness by abiotic conditions (Muletz-Wolz et al., 2017, 2019), and the ability of the pathogen to generate novel, potentially more virulent genotypes through hybridization (Greenspan et al., 2018). Understanding the interplay between pathogen genomics and local environment in determining pathogen virulence, and the ability to dampen virulence traits with probiotics, remains a major research priority.
Data Availability Statement
All code and data to reproduce the results in this manuscript are available on Figshare at DOI https://doi.org/10.6084/m9.figshare.c.4666922.
Ethics Statement
The animal study was reviewed and approved by the University of Salford Ethical Approval Committee.
Author Contributions
RA and XH conceived the study and wrote the manuscript with input from all authors. RA collected the bacterial inhibition data. TS and MF sequenced the Bd isolates and calculated Bd relatedness data. XH analyzed the data.
Funding
This study was funded by the University of Salford Research Pump Priming Fund awarded to RA. XH was funded by an Institute of Zoology Research Fellowship. MF and TS were supported by NERC grant NE/S000844/1.
Conflict of Interest
The authors declare that the research was conducted in the absence of any commercial or financial relationships that could be construed as a potential conflict of interest.
Acknowledgments
The authors would like to thank Prof. Ché Weldon and Prof. Trenton Garner for access to Batrachochytrium dendrobatidis isolates used in this study. MF is a fellow on the CIFAR ‘Fungal Kingdom’ program.
Supplementary Material
The Supplementary Material for this article can be found online at: https://www.frontiersin.org/articles/10.3389/fmicb.2019.03134/full#supplementary-material
Footnotes
References
Antwis, R. E., and Harrison, X. A. (2018). Probiotic consortia are not uniformly effective against different amphibian chytrid pathogen isolates. Mol. Ecol. 27, 577–589. doi: 10.1111/mec.14456
Antwis, R. E., Preziosi, R. F., Harrison, X. A., and Garner, T. W. (2015). Amphibian symbiotic bacteria do not show a universal ability to inhibit growth of the global panzootic lineage of Batrachochytrium dendrobatidis. Appl. Environ. Microbiol. 81, 3706–3711. doi: 10.1128/aem.00010-15
Antwis, R. E., and Weldon, C. (2017). Amphibian skin defences show variation in ability to inhibit growth of Batrachochytrium dendrobatidis isolates from the Global Panzootic Lineage. Microbiology 163, 1835–1838. doi: 10.1099/mic.0.000570
Becker, C. G., Greenspan, S. E., Tracy, K. E., Dash, J. A., Lambertini, C., Jenkinson, T. S., et al. (2017). Variation in phenotype and virulence among enzootic and panzootic amphibian chytrid lineages. Fungal Ecol. 26, 45–50. doi: 10.1016/j.funeco.2016.11.007
Bell, S. C., Alford, R. A., Garland, S., Padilla, G., and Thomas, A. D. (2013). Screening bacterial metabolites for inhibitory effects against Batrachochytrium dendrobatidis using a spectrophotometric assay. Dis. Aquat. Organ. 103, 77–85. doi: 10.3354/dao02560
Brophy, C., Dooley, Á, Kirwan, L., Finn, J. A., McDonnell, J., Bell, T., et al. (2017). Biodiversity and ecosystem function: making sense of numerous species interactions in multi-species communities. Ecology 98, 1771–1778. doi: 10.1002/ecy.1872
Bürkner, P. (2018). Advanced bayesian multilevel modeling with the R package brms. R J. 10, 395–411. doi: 10.32614/RJ-2018-2017
Bürkner, P. C. (2017). brms: an R package for bayesian multilevel models using stan. J. Stat. Softw. 80, 1–28. doi: 10.18637/jss.v080.i01
Charif, D., and Lobry, J. R. (2007). “SeqinR 1.0-2: a contributed package to the R project for statistical computing devoted to biological sequences retrieval and analysis,” in Structural Approaches to Sequence Evolution, eds E. Roman, and U. Bastolla, (Berlin: Springer), 207–232. doi: 10.1007/978-3-540-35306-5_10
Farrer, R. A., Henk, D. A., Garner, T. W., Balloux, F., Woodhams, D. C., and Fisher, M. C. (2013). Chromosomal copy number variation, selection and uneven rates of recombination reveal cryptic genome diversity linked to pathogenicity. PLoS Genet. 9:e1003703. doi: 10.1371/journal.pgen.1003703
Friedman, J., Higgins, L. M., and Gore, J. (2017). Community structure follows simple assembly rules in microbial microcosms. Nat. Ecol. Evol. 1:0109. doi: 10.1038/s41559-017-0109
Gabrilska, R. A., and Rumbaugh, K. P. (2015). Biofilm models of polymicrobial infection. Future Microbiol. 10, 1997–2015. doi: 10.2217/fmb.15.109
Greenspan, S. E., Lambertini, C., Carvalho, T., James, T. Y., Toledo, L. F., Haddad, C. F., et al. (2018). Hybrids of amphibian chytrid show high virulence in native hosts. Sci. Rep. 8:9600. doi: 10.1038/s41598-018-27828-w
Jones, K. E., Patel, N. G., Levy, M. A., Storeygard, A., Balk, D., Gittleman, J. L., et al. (2008). Global trends in emerging infectious diseases. Nature 451:990. doi: 10.1038/nature06536
Kerry, R. G., Patra, J. K., Gouda, S., Park, Y., Shin, H. S., and Das, G. (2018). Benefaction of probiotics for human health: a review. J. Food Drug Anal. 26, 927–939. doi: 10.1016/j.jfda.2018.01.002
Kolde, R. (2019). pheatmap: Pretty Heatmaps. R Package Version 1.0.12. Available at: https://CRAN.R-project.org/package=pheatmap (accessed May 2019).
Koskella, B., Hall, L. J., and Metcalf, C. J. (2017). The microbiome beyond the horizon of ecological and evolutionary theory. Nat. Ecol. Evol. 1:1606. doi: 10.1038/s41559-017-0340-2
Kueneman, J. G., Woodhams, D. C., Harris, R., Archer, H. M., Knight, R., and McKenzie, V. J. (2016). Probiotic treatment restores protection against lethal fungal infection lost during amphibian captivity. Proc. R. Soc. B 283:20161553. doi: 10.1098/rspb.2016.1553
Lips, K. R. (2016). Overview of chytrid emergence and impacts on amphibians. Philos. Trans. R. Soc. B 371:20150465. doi: 10.1098/rstb.2015.0465
Longo, A. V., Fleischer, A. C., and Lips, K. R. (2019). Double trouble: co-infections of chytrid fungi will severely impact widely distributed newts. Biol. Invas. 21, 2233–2245. doi: 10.1007/s10530-019-01973-3
Marcano, I. E., Díaz-Alcántara, C. A., Urbano, B., and González-Andrés, F. (2016). Assessment of bacterial populations associated with banana tree roots and development of successful plant probiotics for banana crop. Soil Biol. Biochem. 99, 1–20. doi: 10.1016/j.soilbio.2016.04.013
McKenzie, V. J., Kueneman, J. G., and Harris, R. N. (2018). Probiotics as a tool for disease mitigation in wildlife: insights from food production and medicine. Ann. N. Y. Acad. Sci. 1429, 18–30. doi: 10.1111/nyas.13617
Mingmongkolchai, S., and Panbangred, W. (2018). Bacillus probiotics: an alternative to antibiotics for livestock production. J. Appl. Microbiol. 124, 1334–1346. doi: 10.1111/jam.13690
Momeni, B., Xie, L., and Shou, W. (2017). Lotka-Volterra pairwise modeling fails to capture diverse pairwise microbial interactions. eLife 6:e25051. doi: 10.7554/eLife.25051
Muletz-Wolz, C. R., Almario, J. G., Barnett, S. E., DiRenzo, G. V., Martel, A., Pasmans, F., et al. (2017). Inhibition of fungal pathogens across genotypes and temperatures by amphibian skin bacteria. Front. Microbiol. 8:1551. doi: 10.3389/fmicb.2017.01551
Muletz-Wolz, C. R., Barnett, S. E., DiRenzo, G. V., Zamudio, K. R., Toledo, L. F., James, T. Y., et al. (2019). Diverse genotypes of the amphibian-killing fungus produce distinct phenotypes through plastic responses to temperature. J. Evol. Biol. 32, 287–298. doi: 10.1111/jeb.13413
O’Hanlon, S. J., Rieux, A., Farrer, R. A., Rosa, G. M., Waldman, B., Bataille, A., et al. (2018). Recent Asian origin of chytrid fungi causing global amphibian declines. Science 360, 621–627. doi: 10.1126/science.aar1965
Piovia-Scott, J., Rejmanek, D., Woodhams, D. C., Worth, S. J., Kenny, H., McKenzie, V., et al. (2017). Greater species richness of bacterial skin symbionts better suppresses the amphibian fungal pathogen Batrachochytrium dendrobatidis. Microb. Ecol. 74, 217–226. doi: 10.1007/s00248-016-0916-4
Quast, C., Pruesse, E., Yilmaz, P., Gerken, J., Schweer, T., Yarza, P., et al. (2013). The SILVA ribosomal RNA gene database project: improved data processing and web-based tools. Opens external link in new window. Nucleic Acids Res. 41, D590–D596. doi: 10.1093/nar/gks1219
R Core Team (2019). R: A Language and Environment for Statistical Computing. Vienna: R Foundation for Statistical Computing.
San-Wong, H., Maker, G. L., Trengove, R. D., and O’Handley, R. M. (2015). GCMS-based metabolite profiling of Salmonella typhimurium differentiates between biofilm and planktonic phenotypes. Appl. Environ. Microbiol. 81, 2660–2666. doi: 10.1128/AEM.03658-14
Scheele, B. C., Pasmans, F., Skerratt, L. F., Berger, L., Martel, A., Beukema, W., et al. (2019). Amphibian fungal panzootic causes catastrophic and ongoing loss of biodiversity. Science 363, 1459–1463. doi: 10.1126/science.aav0379
Schliep, K., Potts, A. J., Morrison, D. A., and Grimm, G. W. (2017). Intertwining phylogenetic trees and networks. Methods Ecol. Evol. 8, 1212–1220. doi: 10.1111/2041-210x.12760
Schliep, K. P. (2011). phangorn: phylogenetic analysis in R. Bioinformatics 27, 592–593. doi: 10.1093/bioinformatics/btq706
Schloss, P. D., Westcott, S. L., Ryabin, T., Hall, J. R., Hartmann, M., Hollister, E. B., et al. (2009). Introducing mothur: open-source, platform-independent, community-supported software for describing and comparing microbial communities. Appl. Environ. Microbiol. 75, 7537–7541. doi: 10.1128/AEM.01541-09
Tan, L. T., Chan, K. G., Lee, L. H., and Goh, B. H. (2016). Streptomyces bacteria as potential probiotics in aquaculture. Front. Microbiol. 7:79. doi: 10.3389/fmicb.2016.00079
Tompkins, D. M., Carver, S., Jones, M. E., Krkošek, M., and Skerratt, L. F. (2015). Emerging infectious diseases of wildlife: a critical perspective. Trends Parasitol. 31, 149–159. doi: 10.1016/j.pt.2015.01.007
Vehtari, A., Gabry, J., Yao, Y., and Gelman, A. (2019). loo: Efficient Leave-One-Out Cross-Validation and WAIC for Bayesian Models. R Package Version 2.1.0. Available at: https://CRAN.R-project.org/package=loo (accessed May 2019).
Vehtari, A., Gelman, A., and Gabry, J. (2017). Practical Bayesian model evaluation using leave-one-out cross-validation and WAIC. Stat. Comput. 27, 1413–1432. doi: 10.1007/s11222-016-9696-4
Warton, D. I., and Hui, F. K. (2011). The arcsine is asinine: the analysis of proportions in ecology. Ecology 92, 3–10. doi: 10.1890/10-0340.1
Widder, S., Allen, R. J., Pfeiffer, T., Curtis, T. P., Wiuf, C., Sloan, W. T., et al. (2016). Challenges in microbial ecology: building predictive understanding of community function and dynamics. ISME J. 10:2557. doi: 10.1038/ismej.2016.45
Keywords: chytrid fungus, Batrachochytrium dendrobatidis, wildlife disease, amphibian decline, amphibian disease
Citation: Harrison XA, Sewell T, Fisher M and Antwis RE (2020) Designing Probiotic Therapies With Broad-Spectrum Activity Against a Wildlife Pathogen. Front. Microbiol. 10:3134. doi: 10.3389/fmicb.2019.03134
Received: 24 September 2019; Accepted: 26 December 2019;
Published: 22 January 2020.
Edited by:
Christoph Vorburger, Swiss Federal Institute of Aquatic Science and Technology, SwitzerlandReviewed by:
Sergio Enrique Pasteris, Universidad Nacional de Tucumán, ArgentinaKristin M. Burkholder, University of New England, United States
Copyright © 2020 Harrison, Sewell, Fisher and Antwis. This is an open-access article distributed under the terms of the Creative Commons Attribution License (CC BY). The use, distribution or reproduction in other forums is permitted, provided the original author(s) and the copyright owner(s) are credited and that the original publication in this journal is cited, in accordance with accepted academic practice. No use, distribution or reproduction is permitted which does not comply with these terms.
*Correspondence: Xavier A. Harrison, x.harrison@exeter.ac.uk; xav.harrison@gmail.com; Rachael E. Antwis, r.e.antwis@salford.ac.uk