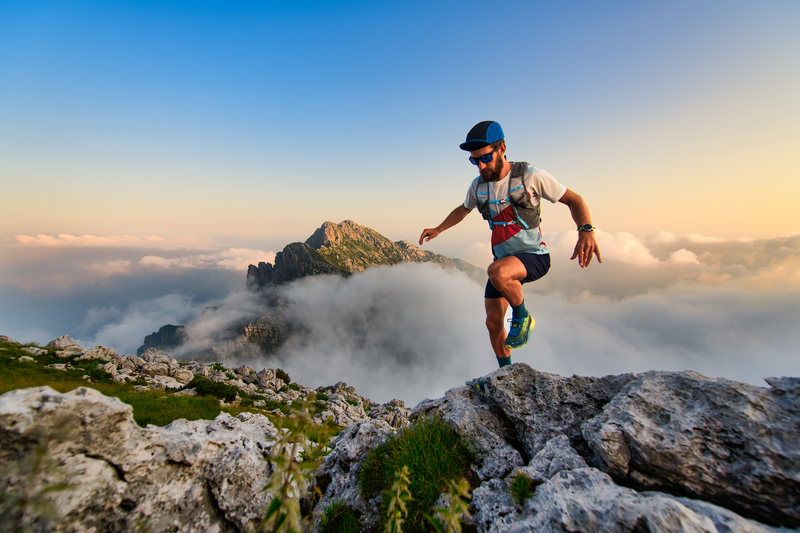
95% of researchers rate our articles as excellent or good
Learn more about the work of our research integrity team to safeguard the quality of each article we publish.
Find out more
ORIGINAL RESEARCH article
Front. Microbiol. , 31 May 2019
Sec. Food Microbiology
Volume 10 - 2019 | https://doi.org/10.3389/fmicb.2019.01223
Effective control of foodborne pathogens on produce requires science-based validation of interventions and control strategies, which typically involves challenge studies with a set of bacterial strains representing the target pathogens or appropriate surrogates. In order to facilitate these types of studies, a produce-relevant strain collection was assembled to represent strains from produce outbreaks or pre-harvest environments, including Listeria monocytogenes (n = 11), Salmonella enterica (n = 23), shiga-toxin producing Escherichia coli (STEC) (n = 13), and possible surrogate organisms (n = 8); all strains were characterized by whole genome sequencing (WGS). Strain diversity was assured by including the 10 most common S. enterica serotypes, L. monocytogenes lineages I–IV, and E. coli O157 as well as selected “non-O157” STEC serotypes. As it has previously been shown that strains and genetic lineages of a pathogen may differ in their ability to survive different stress conditions, a subset of representative strains for each “pathogen group” (e.g., Salmonella, STEC) was selected and assessed for survival of exposure to peroxyacetic acid (PAA) using strains pre-grown under different conditions including (i) low pH, (ii) high salt, (iii) reduced water activity, (iv) different growth phases, (v) minimal medium, and (vi) different temperatures (21°C, 37°C). The results showed that across the three pathogen groups pre-growth conditions had a larger effect on bacterial reduction after PAA exposure as compared to strain diversity. Interestingly, bacteria exposed to salt stress (4.5% NaCl) consistently showed the least reduction after exposure to PAA; however, for STEC, strains pre-grown at 21°C were as tolerant to PAA exposure as strains pre-grown under salt stress. Overall, our data suggests that challenge studies conducted with multi-strain cocktails (pre-grown under a single specific condition) may not necessarily reflect the relevant phenotypic range needed to appropriately assess different intervention strategies.
Control of foodborne pathogens along the supply chain relies on the development and implementation of validated interventions and control strategies, including validated heat treatment schemes or other pathogen reduction steps as well as validated sanitation procedures. While some industries have well established pathogen reduction steps supported by globally recognized validation studies (e.g., pasteurization of milk [Sarkar, 2015]), other food industries and commodities (e.g., baked goods, produce) have an urgent need for scientific validation of different control strategies. Validation is particularly challenging for commodities that represent considerable diversity of production and processing practices, such as it is the case for produce.
In addition, new regulations (such as the US Food Safety Modernization Act [FSMA]) place an increasing emphasis on science-based approaches and scientific validation of control strategies and interventions. Scientifically justifiable strain selection and growth conditions for validation and challenge studies are an essential part of a science-based food safety system. Typically, studies evaluating relevant pathogen interventions or growth and survival of pathogens are conducted using multiple strains, which may be used separately or in cocktails (mixture of multiple strains) (Scott et al., 2005). This approach is used to account for strain diversity and to assure that control strategies are designed to deliver the appropriate or required protection even with more tolerant strains. Pathogen strains used in these types of studies (and in “cocktails”) are typically selected to represent subtypes with a known association with a given product (such as “outbreak strains”), while also considering the phylogenetic diversity of a given target pathogen, which typically is addressed by including multiple distinct serotypes and/or phylogenetic groups. For example, for shiga-toxin producing Escherichia coli (STEC), representation of E. coli O157 as well as other STEC serotypes commonly associated with E. coli infections in the United States (e.g., O26, O45, O103, O111, O121, O145 [Gould et al., 2013]) is often desired; these additional serotypes will be referred to here as “non-O157 STEC” serotypes (in the United States this group is sometimes referred to as the “Big Six non-O157 STEC”).
Importantly, a number of studies have shown that different pathogen strains and genetic lineages can differ considerably in their ability to survive stress conditions; this has been well documented for key pathogens of concern to the produce industry including L. monocytogenes (De Jesus and Whiting, 2003; Bergholz et al., 2010; Cheng et al., 2015), S. enterica (Li et al., 2012; Andino and Hanning, 2015) and STEC (Oh et al., 2009; Carter et al., 2012; Lee et al., 2012; Wang et al., 2012).
While it has been well established that different strains and genetic lineages of a pathogen may differ in their ability to survive and grow under different stress conditions, the physiological state of bacterial cells and the conditions under which bacteria are pre-grown also have a considerable impact on the ability of foodborne pathogens to survive subsequent stress conditions, including produce-relevant interventions (such as chlorine washes) as specifically documented for L. monocytogenes (Bergholz et al., 2012, 2013; Poimenidou et al., 2016), S. enterica (Yang et al., 2001; Asakura et al., 2002; Gruzdev et al., 2011; Stackhouse et al., 2012; He et al., 2013) and STEC (Gawande and Griffiths, 2005; Shuai et al., 2017). One of the most well documented examples of the effect of environmental conditions on stress tolerance is the observation that S. enterica present in low water activity (aw) environments are considerably more tolerant to heat treatment than S. enterica present in high water activity environments (He et al., 2013; Peña-Meléndez et al., 2014). For example, S. enterica serotype Tennessee (“Salmonella Tennessee”) present in peanut butter with an aw of 0.2 showed less than a 3 log reduction after treatment at 90°C for 20 min, while reduction in peanut butter with an aw of 0.8 was around 5 log, a difference of at least 2 log reduction (He et al., 2013). These findings do suggest a need for further studies that evaluate and compare the effects of genetic diversity and growth conditions on subsequent stress tolerance and growth phenotypes. Challenge study guidance documents typically specify that “for either inactivation or growth studies, adaptation of cells should attempt to mimic the likely physiological state of the organism at the time it contaminates the food” (National Advisory Committee on Microbiological Criteria for Foods, 2010). However, there is limited specific guidance or data available that would help to determine which strains or growth conditions should be used for challenge or validation studies if no specific physiological state can easily be defined for contaminating pathogens, as likely in the produce industry where contamination of a single commodity can originate from very different environments.
The aim of this study was to (i) assemble a strain collection including S. enterica, L. monocytogenes and STEC, as well as possible surrogate organisms relevant for produce safety (with an initial bias toward United States relevant strains) and to (ii) use a diverse subset of strains from this collection to formally assess the effect of pre-growth conditions on subsequent survival of produce-relevant interventions, using exposure to peroxyacetic acid (PAA) as a model. Since PAA was patented in 1950 to treat fruits and vegetables to reduce spoilage its application for fresh produce has been well established (Greenspan and Margulies, 1950; Wisniewsky et al., 2000; Gonzalez et al., 2004; Hellstrom et al., 2006). PAA is also commonly used in wash water as well as for sanitation of food contact surfaces due to its activity over a broad temperature range and even under presence of organic matter (Lokkesmoe and Olson, 1995; Rodgers et al., 2004). Growth conditions used here were selected to focus on conditions that are either produce relevant (e.g., growth at 21°C) or that have previously been linked to increased stress tolerance, including low water activity (Goepfert et al., 1970; Mattick et al., 2000), acid stress (Lou and Yousef, 1997; Greenacre et al., 2006), salt stress (Begley et al., 2002; Bergholz et al., 2012, 2013), and minimal medium (Jenkins et al., 1988, 1990; Kenyon et al., 2002). The data from this study will help the produce industry to justify the use of (i) specific pathogen and surrogate strains and (ii) specific pre-growth or pre-adaptation conditions for validation studies. In addition, this study will provide access to a well characterized standard strain collection with all strains characterized by whole genome sequencing (WGS).
In order to assemble a produce-relevant strain collection, an initial draft collection was proposed that included strains linked to fresh produce outbreaks and pre-harvest environments, including S. enterica, STEC, and L. monocytogenes as well as select relevant surrogate strains. As a first step, pathogen strains isolated from human cases or food with key produce-associated outbreaks (e.g., the 2011 listeriosis outbreak linked to consumption of contaminated cantaloupe) were selected. This initial collection was supplemented with non-produce-associated strains as needed to represent the pathogen diversity for a given group. For example, produce-related STEC strains that were initially selected included E. coli O157 as well as two non-O157 STEC serotypes (i.e., O121, O26), these strains were supplemented with seven other STEC strains to represent the most common clinically associated serotypes in the United States (i.e., serotype O145, O111, O45 and O103 [Gould et al., 2013]). Surrogate organisms for inclusion in the initial draft strain collection were selected to include strains that had previously been used in produce-relevant validation or challenge studies, e.g., the rifampicin resistant E. coli strain TVS 353 (also designated W778), which has been used in a number of studies that assessed survival of E. coli on leafy greens (Tomás-Callejas et al., 2011; Weller et al., 2017a; Cai et al., 2018; Wright et al., 2018). The initial draft strain collection included 13 L. monocytogenes, 24 S. enterica, 10 STEC, as well as 10 surrogate organisms (5 additional E. coli, two Listeria innocua, one Listeria marthii, one Enterococcus faecalis, and one Enterococcus faecium).
An electronic survey was sent to 30 US-based experts from industry, academia, and government to solicit their input on the initial draft strain collection for its suitability to evaluate produce-relevant pathogens as well as surrogates for different phenotypic characteristics relevant to produce production and distribution (e.g., survival under selected stress conditions and in the presence of sanitizer); see Supplementary Table 1 for this survey, which includes a list of all strains in the draft collection. Experts were asked to rate each strain on a scale of 1 to 5 (1 – strain irrelevant, do not include, 2 – strain may be relevant, 3 – uncertain, strain may or may not be relevant, 4 – important, should be included, 5 – very important, must be included); information provided on the proposed strain collection included ID numbers, isolate origin (e.g., associated outbreak), and references that detailed the strain history or outbreak. Strains were selected for inclusion in the final strain set if at least 50% of respondents considered a strain as important (score of 4 or 5). Reviewers also had the option to suggest inclusion of additional strains; suggested strains were evaluated by the authors, who decided on their inclusion in the final strain set.
The final strain collection included (i) 11 L. monocytogenes, (ii) 23 S. enterica, (iii) 13 STEC, and (iv) 8 surrogate organisms (Table 1). All strains are stored in Brain Heart Infusion (BHI; Difco, Becton Dickinson, Sparks, MD) with 15% glycerol at -80°C.
Table 1. Final produce-relevant strains included in the collection L. monocytogenes, S. enterica, and E. coli, and surrogate organisms.
Isolates were streaked from glycerol stocks onto BHI agar plates and plates were incubated at 37°C for 24 h. An overnight culture was prepared by inoculating 5 mL BHI broth with a single colony, followed by incubation at 37°C for 12–14 h. Following manufacturer’s instructions for DNA extraction, 2 mL of the 12–14 h culture was pelleted and used for DNA extraction (DNeasy Blood and Tissue kit, Qiagen, Valencia, CA). Gram-positive bacteria were pre-treated in 200 μL lysis solution (20 mg/mL lysozyme, 20 mM Tris–HCl, 2 mM EDTA, 1.2% Triton X-100). DNA was eluted in 50 μL of 10 mM Tris–HCl at pH 7.5, followed by spectrophotometric assessment of DNA purity with a NanoDrop 2000 (Thermo Fisher Scientific, Waltham, MA) and DNA quantification with a fluorescent nucleic acid dye (Qubit dsDNA HS Assay Kit, Thermo Fisher Scientific) and a Qubit 2.0 fluorometer (Thermo Fisher Scientific). Libraries were prepared for sequencing with Nextera XT DNA sample preparation Kit and the associated Nextera XT Index Kit with 96 indices (Illumina, Inc., San Diego, CA). Library preparation was conducted according to the PulseNet standard operation procedure “Laboratory Standard Operating Procedure for Pulsenet Nextera XT Library Prep and Run Setup for the Illumina Miseq”1. Pooled samples were sequenced on an Illumina MiSeq platform with 2× 250 bp paired-end reads (Animal Health Diagnostic Center Cornell University) or HiSeq 2500 rapid run with 2× 100 bp paired-end reads (Genomics Facility of Cornell University).
Adapters were removed from sequences using Trimmomatic v 0.33 (Bolger et al., 2014) followed by quality assessment using FastQC v 0.11.42. Sequences were assembled de novo with SPAdes version 3.8.0 (Bankevich et al., 2012). Quality control of assemblies was performed with QUAST v 3.2 (Gurevich et al., 2013) and average coverage determined using SAMtools v 1.4.1 (Li et al., 2009). Contigs smaller than 200 bp were removed and the remaining contigs were searched against Kraken (Wood and Salzberg, 2014), using BLAST, to confirm strain identity. Additionally, serotypes were confirmed with Seqsero3 for S. enterica (Zhang et al., 2015) and SeroTypeFinder for E. coli (Joensen et al., 2015). A standard set of 21 sequenced Listeria genomes previously described by Liao et al. (2017) was used in a SNP-based phylogenetic analysis to confirm lineages for Listeria strains (Liao et al., 2017). S. enterica with acquired antibiotic resistance (e.g., rifampicin, naldixic acid resistance) were compared to their wildtype parent to identify high quality SNPs using the CFSAN SNP Pipeline (Davis et al., 2015). BLASTX was used to identify genes if SNPs were located in potential open reading frames (ORFs) (States and Gish, 1994).
The core SNP based analysis was performed using kSNP v 3, with estimated optimal kmer size 13 (determined using Kchooser), for each bacterial group including (i) Listeria, (ii) S. enterica, and (iii) E. coli (Gardner et al., 2015). A maximum-likelihood phylogeny based on core genome SNP was generated with 1000 bootstrap repetitions in RAxML for each bacterial group. Surrogate strain sequences were integrated into phylogenetic analysis with their associated bacterial group except for E. faecium (ATCC 8459) (Stamatakis, 2015). Phylogenetic trees were edited using FigTree v 1.3.44.
As an example, for additional screening for mutations, the selected strains were analyzed for mutations in key stress response regulator genes, including sigB (for Listeria) and rpoS (for S. enterica and E. coli). Reference genes used were sigB of EGD-e (259 aa; NCBI accession no. NC_003210.1:930671-931450), rpoS of S. enterica Typhi str. CT18 (330 aa, NCBI accession no. NC_003198.1:c2916069-2915077) and rpoS of E. coli K-12 (330 aa; NC_000913.3:c2867551-2866559); the K12 reference sequence was selected to represent a strain that was lacking any rpoS mutations that had been reported for some K12 strains, including the codon 33 amber mutation (Subbarayan and Sarkar, 2004). To identify non-synonymous mutations or premature stop codons, amino acid sequences were aligned using MUSCLE (Edgar, 2004).
Bacterial isolates were streaked from glycerol stocks onto Tryptic Soy Agar plates (TSA; Difco, Becton Dickinson, Sparks, MD) and incubated at 37°C for 24 h; following incubation plates were held at 4°C for a minimum of 24 h and a maximum of 7 days. Single colonies from these plates were inoculated into 5 mL of TSB, followed by incubation at 37°C with shaking for 12–14 h. These cultures were used to inoculate, at a 1:1000 dilution, pre-warmed side-arm flasks (Nephelo Flasks/C38 300 mL; Belco, Vineland, NJ) that contained either 30 or 100 mL of growth medium. Bacterial cultures were grown without shaking to early stationary phase at 37°C except when the pre-growth condition was defined as “mid-log phase” or “21°C.” Growth curves were generated for each strain in each condition to determine the OD for each growth phase using a spectrophotometer (20D+, Thermo Fisher Scientific) with a linear range from 0.2 to 0.7. Appropriate dilutions were made prior to reading if necessary, to stay within the linear range. For this study, seven different pre-growth conditions were selected, including (i) low pH (pH 5.0 for S. enterica and E. coli; pH 5.5 for Listeria), (ii) high salt (4.5% NaCl), (iii) reduced water activity (0.96 for S. enterica and E. coli; 0.95 for Listeria), (iv) two different growth phases (mid-log phase, stationary phase), (v) minimal medium including M9, prepared as previously described (Green and Sambrook, 2012) using 0.1% (w/v) casamino acids and either 0.4% glucose (w/v) (for S. enterica) or 0.3% fructose (w/v) (E. coli) and chemically defined minimal medium with 10 mM glucose for Listeria (Premaratne et al., 1991; Peña-Meléndez et al., 2014) and (vi) two different temperatures (37°C, 21°C). Water activity was adjusted using glycerol at 15.6% (v/v) and 13% (v/v) to achieve an aw of 0.95 (Listeria) and 0.96 (S. enterica and E. coli), respectively. The pH was adjusted using lactic acid and high salt environment was generated with additional 4% NaCl (w/v). The parameters for pre-growth were chosen based on preliminary experiments that identified the most stressful conditions (e.g., lowest pH) that would still allow for reproducible growth curves. The pre-growth conditions chosen for this project are only a selection of possible relevant stress conditions. For examples, pathogen contamination can occur both at the preharvest stages (e.g., from soil, wildlife feces) or post-harvest environments (e.g., dry or wet processing plant environments); hence pre-growth conditions may reflect a wide range of environmental conditions. Importantly, all stresses selected for this study have been previously shown to increase stress tolerance including low water activity (Goepfert et al., 1970; Mattick et al., 2000), acid stress (Lou and Yousef, 1997; Greenacre et al., 2006), salt stress (Begley et al., 2002; Bergholz et al., 2012, 2013), and minimal medium (Jenkins et al., 1988, 1990; Kenyon et al., 2002). Further details on all pre-growth conditions are reported in Supplementary Table 2 and Supplementary Figures 1–3.
Phylogenetic and SNP data were used to select four diverse wild type strains for L. monocytogenes and S. enterica as well as five diverse wild type strains for STEC for phenotypic characterization. These strains were supplemented with one surrogate for L. monocytogenes (L. innocua FSL C2-0008), two surrogates for S. enterica (the avirulent S. enterica MHM112 and E. faecium ATCC 8459), and one surrogate for STEC (E. coli TVS 353). Bacterial cultures pre-grown under different pre-growth conditions were exposed to PAA (Tsunami, Ecolab, St. Paul, MN) and PAA concentration was measured using Reflectoquant (RQflex 10, Millipore, Darmstadt, Germany). For each treatment, 1 mL of bacterial culture was added to 9 mL PAA (in a 15 mL Falcon tube) for a final concentration of either 60 ppm (Listeria), or 40 ppm (E. coli, S. enterica) followed by mixing through four inversions and incubation for 45 s (the 45 s time period included the time required for the four inversions). The sanitizer solution was inactivated by adding 100 μL 50% Na2S2O3 (w/v) immediately after the 45 s incubation, followed by four inversions of the tube to assure complete mixing; the four inversions took about 10 s and were completed after the 45 s exposure time. Control cultures were treated with phosphate-buffer saline solution (PBS) instead of PAA. Immediately after sanitizer inactivation, 50 μL of the appropriate dilutions were plated in duplicates on TSA plates using a spiral plater (Autoplate 4000, Advanced Instruments Inc., Norwood, MA). Plates were incubated at 37°C for 24 h (S. enterica, E. coli) or 48 h (Listeria). Colonies were enumerated using Color Q-Count (Model 530, Advanced Instruments Inc.). The PAA concentrations chosen here are below the maximum use level concentration of 80 ppm for wash water detailed in the US Code of Federal Regulations Title 21 (21CFR173.315); PAA concentrations were chosen to typically yield bacterial numbers above the detection limit of 100 CFU/mL after PAA treatment (in order to allow for quantification of die-off).
Statistical analyses were performed in R (version 3.1, R Core Team, Vienna, Austria). A generalized linear model was fitted to the binomial proportion of surviving cells with a loge link function using lme4 package (Bates et al., 2015); independent variables were the crossed random effects of strain and condition. The arithmetic mean of observed log reduction was reported when survival of surrogate organisms was compared to a particular strain set and limit of detection was substituted for values where post-sanitizer count was zero. All experiments were conducted in biological triplicate.
Trimmed raw reads and assembled genomes for all strains were submitted under the BioProject ID PRJNA395587 to NCBI’s Sequence Read Archive (SRA) and GenBank (Table 1). Information about the strain collection is also available at https://foodsafety.foodscience.cornell.edu/research-and-publications/-strain-collection. Data associated with each strain (e.g., source isolation, serotype, published papers associated with a given strain) are also available on Food Microbe Tracker5 (Vangay et al., 2013).
Strain requests within the United States or internationally can be directed to the original provider or the Food Safety Laboratory (FSL; Department of Food Science, Cornell University, Ithaca, NY; e-mail: bXcxNkBjb3JuZWxsLmVkdQ==). Strain requests for strains provided by the FDA, USDA, ATCC or the STEC Center have to be directed to these institutions (see Supplementary Table 3 for current contact information).
Among the 30 experts that were surveyed for their feedback on an initial draft collection that included a total of 57 strains, 19 provided responses including experts in academia (n = 6), government (n = 5), and industry (n = 8), all with at least 10 years of experience in food safety. More than 50% of experts who responded classified 33 of the 57 strains as “important” (ranking of 4 or 5); all of these strains were included in the final set. While 24 strains were classified as important by <50% of experts, seven of them were still included in the final set as their inclusion was necessary to assure strain diversity including the 10 most common S. enterica serotypes in the US-based on the incidence rate of Salmonella infections (Foodborne Diseases Active Surveillance and Network, 2017), all four L. monocytogenes lineages, and the most common non-O157 STEC serotypes linked to human illnesses in the United States (Gould et al., 2013). For example, the L. monocytogenes lineage IV strain FSL J1-158 (isolated from a goat) was classified as important by only 7/19 experts but was included to assure presence of at least one lineage IV strain in the final strain set. Expert reviewers also suggested 10 additional strains for inclusion. Of the suggested strains, four were added to the final strain collection. In addition, four parent strains were acquired along with antibiotic resistant derivatives of the parent strains, e.g., Salmonella Poona FSL R9-6569 is the rifampicin resistant derivative of FSL R9-6568. During the strain acquisition process and after completion of the survey, an additional seven strains were submitted to the strain collection by experts in academia and government e.g., the avirulent Salmonella Typhimurium strain MHM112 (FSL R9-6232) (de Moraes et al., 2016). The final collection includes a total of 55 strains, which are discussed in more detail below. While all strains were assigned Food Safety Lab (“FSL”) numbers, in this publication, the previous ID numbers will be used for previously reported surrogates to be consistent with previously published literature.
Strains for the collection were obtained from either (i) previously described collections (e.g., ILSI NA Listeria strain collection [Fugett et al., 2006], ATCC [Clark and Geary, 1974]), the Cornell Food Safety Lab (FSL) collection or (ii) various outside sources, e.g., Food and Drug Administration (FDA), Centers for Disease Control and Prevention (CDC), United States Department of Agriculture (USDA), Wisconsin State Laboratory of Hygiene, Texas Tech University, STEC Center Michigan State University, University of California Davis, and University of Florida (Table 1).
The L. monocytogenes strain set is comprised of 11 strains, 4 of them from listeriosis cases linked to produce, including cantaloupe (2011), packaged salad (2016) and sprouts (2014); one strain was obtained from soil collected in a spinach field (Weller et al., 2015). An additional six strains were included to ensure inclusion of lineages I–IV. The final strain set represented four Listeria serotypes, as well as six lineage I strains, three lineage II strains, and one strain for each lineage III and lineage IV. In addition to the 11 Listeria detailed above, the strain set includes L. innocua as a possible surrogate organism for L. monocytogenes (Girardin et al., 2005; Friedly et al., 2008; Omac et al., 2015); we specifically included L. innocua strain FSL C2-0008 (Table 1).
For three of the Listeria strains, WGS data were already available. WGS data for all other strains were generated (see Supplementary Table 4 for detailed WGS data). A core SNP maximum-likelihood tree showed that strains clustered by their lineages (Figure 1). Among all Listeria, the number of pairwise core SNP differences ranged from 1 to 6,014 SNPs. The maximum number of core SNP differences within lineage I strains was 359 SNPs as compared to 1,055 SNPs within lineage II. Lineage I isolates FSL J1-0107 and FSL J1-0108, showed no SNP differences based on the kSNP-based core SNPs, consistent with the fact that both isolates were obtained from the same outbreak. In contrast, high quality SNP analysis identified 14 SNP differences between these two isolates. Also, all strains were assessed for potential mutations in sigB, which encodes the alternative sigma factor σB; mutations in this gene may reduce stress tolerance (Raengpradub et al., 2008). Among the 12 strains, SigB was highly conserved with only four polymorphic amino acid sites (see Supplementary Figure 4 for details).
Figure 1. Listeria maximum-likelihood tree based on core SNP analysis using kSNP. The phylogeny for strains was inferred using RAxML and tree was rooted by midpoint. The strain FSL ID is followed by serotype and lineage. The node labels represent bootstrap values of 1000 repetitions. The bar indicates 0.2 substitutions per site. Strains marked with a star were selected as representative strains for phenotypic analyses (i.e., PAA experiments).
The S. enterica strain set is comprised of 23 strains; 13 of these strains are from outbreaks linked to produce, including cantaloupe (two outbreaks, 2000–2002 and 2008), tomatoes (two outbreaks, 2002 and 2005), orange juice (2005), jalapeno peppers (2008), cucumbers (2015), alfalfa sprouts (2016), and bean sprouts (2014). Three strains were associated with outbreaks linked to almonds (2000–2001) and peanut butter (two outbreaks, 2006–2007 and 2008–2009). An additional four strains were included to ensure representation of the 10 most common serotypes associated with human illnesses in the United States (Foodborne Diseases Active Surveillance and Network, 2017); the final set represents 16 S. enterica serotypes. Finally, the strain set includes two S. enterica strains that have previously been used in produce-relevant validation studies (e.g., Salmonella Senftenberg FSL R9-5274). In addition to the 23 S. enterica detailed above, the strain set also includes three surrogate organisms relevant for S. enterica, specifically E. faecium ATCC 8459 (FSL R9-5275) and the two avirulent S. Typhimurium MHM112 and MHM108 (FSL R9-6232 and FSL R9-6231, respectively).
For one of the 23 S. enterica strains (as well as for E. faecium ATCC 8459 and the avirulent Salmonella Typhimurium MHM112), WGS data were already available; WGS data were generated for all other strains (Table 1). Initial analysis of the WGS data identified two S. enterica isolates where the serotype predicted based on WGS did not match the reported serotype (i.e., the serotype reported for the two outbreaks these isolates were associated with). For example, a Salmonella Poona isolate (received as representing an isolate from the cantaloupe outbreak in 2000–2002) was identified as serotype Agona based on WGS data, indicating that the wrong isolate was sequenced. These two isolates were subsequently acquired from another source; WGS of these new isolates confirmed that they represented the correct serotype.
Whole genome sequencing data for all S. enterica strains yielded genome sizes from 4.6 to 5.3 Mbp (see Supplementary Table 4 for detailed WGS data). Among all S. enterica strains (excluding antibiotic resistant strains derived from a given parent strain), the number of pairwise core SNP differences ranged from 51 to 13,436 SNPs. A core SNP maximum-likelihood tree showed that strains clustered by serotypes except for (i) the one serotype 4,[5],12:i:- strain, which, as expected, clustered closely within Typhimurium and (ii) one Newport strain, which clustered closely with serotype Litchfield, consistent with previous data that Newport is polyphyletic and represents multiple lineages (Timme et al., 2013; Toboldt et al., 2013; Zheng et al., 2017) (Figure 2).
Figure 2. Salmonella maximum-likelihood tree based on core SNP analysis using kSNP. The phylogeny for strains was inferred using RAxML and tree was rooted by midpoint. The strain FSL ID is followed by serotype; R∗ and N∗ indicate rifampicin and nalidixic acid resistant strains, respectively. The node labels represent bootstrap values of 1000 repetitions. The bar indicates 0.04 substitutions per site. Strains marked with a star were selected as representative strains for phenotypic analyses (i.e., PAA experiments).
Further WGS analyses were performed to identify specific mutations in (i) the two S. enterica strains that had been selected for resistance to rifampicin (FSL R9-6567, FSL R9-5251) and (ii) one S. enterica strain that had been selected for resistance to nalidixic acid (FSL R9-5220). High quality SNP analysis identified a single point mutation in the RNA polymerase subunit B gene (rpoB) in the rifampicin resistant strain FSL R9-6567, as compared to its parent strain (FSL R9-6568). The rifampicin resistant strain FSL R9-6567 also had a non-synonymous mutation in flhE, which is part of the flhBAE operon and has a potential role as a chaperone and contributes to flagellar biosynthesis (Lee et al., 2015). The rifampicin resistant strain FSL R9-5251 showed 9 non-synonymous mutations relative to its parent strain, including two in rpoC, which encodes the RNA polymerase β′ subunit, as well as one each in fadJ (fatty acid oxidation complex subunit alpha) (Iram and Cronan, 2006), fadR (the transcriptional regulator for fatty acid degradation), hemL (the glutamate-a-semialdehyde aminotransferase), glpR (transcriptional repressor of sugar metabolism), and yhdA (encoding the regulatory protein CsrD, which controls degradation of CsrB and CsrC RNA) (Suzuki et al., 2006). The nalidixic resistant strain FSL R9-5220 showed one non-synonymous mutation in gyrA, which encodes the DNA gyrase subunit A and plays an essential role in DNA replication (Piddock et al., 1998) (see Supplementary Table 5 for details).
All strains were also assessed for potential mutations in rpoS, which encodes the alternative sigma factor (RpoS); mutations in this gene have previously been shown to accumulate during lab passages and may reduce stress tolerance (Sutton et al., 2000). Among the 22 strains, RpoS was highly conserved with only three polymorphic amino acid sites; strain FSL R9-5505 carried a point mutation, which leads to a frameshift and premature stop codon (see Supplementary Figure 5 for details). The surrogate organism (the avirulent S. Typhimurium, MHM112) was excluded from the analysis due to low coverage.
The STEC set is comprised of 13 strains; eight of these strains are from E. coli outbreaks linked to produce, including baby spinach (2006), shredded romaine lettuce (2010), sprouts (two outbreaks, 2011 and 2012), clover sprouts (two outbreaks, 2012 and 2014), cabbage salad (2014), and alfalfa sprouts (2016). An additional two strains collected in the Republic of Georgia (2009) and one strain from an outbreak linked to apple cider were also included. In order to ensure inclusion of the non-O157 STEC serotypes, an additional two strains were included representing serotypes O45 and O103. The strain set also includes four surrogate organisms relevant for E. coli, including three generic E. coli isolated from the environment (FSL R9-4077, FSL R9-4078, FSL R9-4079), as well as a naturally occurring non-pathogenic E. coli O157:H7 (FSL R9-3467), all of which have been used previously as surrogates (Tomás-Callejas et al., 2011; Atwill et al., 2015; Weller et al., 2017a,b; Cai et al., 2018; Wright et al., 2018) (Table 1).
For two of the 13 STEC strains (as well as the non-pathogenic E. coli O157:H7), WGS data was already available; WGS data were generated for all the other strains and yielded genome sizes from 5.2 to 5.6 Mbp (see Supplementary Table 4 for detailed WGS data). A core SNP maximum likelihood tree showed that strains clustered by serotype (Figure 3). Among all E. coli strains, the number of pairwise core SNP differences ranged from 22 to 1,174 SNPs. All strains were also assessed for potential mutations in rpoS, which encodes the alternative sigma factor (RpoS); polymorphism in this gene as well as accumulation of mutations in lab strains which may alter stress response have been previously described (Snyder et al., 2012). Among the 17 strains, RpoS was highly conserved with only two polymorphic amino acid sites detected in FSL R9-5257 (D118N), and FSL R9-5513 (G309D). In addition, a single point mutation in strain FSL R9-3467 lead to a frameshift and premature stop codon. For strain FSL R9-5512, the last eight amino acids in the sequence differ from the consensus sequence, due to a 11 bp deletion (nucleotides 966 to 976) at the 3′ end of rpoS (see Supplementary Figure 6 for details).
Figure 3. Escherichia coli maximum-likelihood tree based on core SNP analysis using kSNP. The phylogeny for strains was inferred using RAxML and tree was rooted by midpoint. The strain FSL ID is followed by serotype. The node labels represent bootstrap values of 1000 repetitions. The bar indicates 0.05 substitutions per site. Strains marked with a star were selected as representative strains for phenotypic analyses (i.e., PAA experiments).
As an initial application, our strain collection was used to characterize the die-off of different bacterial strains after short exposure to a produce industry relevant sanitizer (i.e., PAA). For these experiments, a subset of strains was conveniently selected for each bacterial group, including (i) four L. monocytogenes and one L. innocua (this species represents a possible surrogate for L. monocytogenes); (ii) four S. enterica and two potential S. enterica surrogates (the avirulent Salmonella Typhimurium MHM112 and E. faecium ATCC 8459) and (iii) five STEC and one potential surrogate (i.e., E. coli FSL R9-4077). Pathogen strains were selected to represent phylogenetically distinct strains (based on the core SNP phylogenies, see Figures 1–3) and preference was given to select strains from produce-associated outbreaks. Each strain was pre-grown under each of the seven different conditions to assess the relative impact of strain diversity and growth condition on PAA survival.
To assess the variance of responses due to strain versus the variance of responses due to pre-growth condition, data for each of the three pathogen groups (i.e., L. monocytogenes, S. enterica, STEC) were assessed using a crossed random effects model. This model showed that for each pathogen group, the “condition” variance component was larger than the “strain” variance component and the “strain-condition interaction” variance component (Table 2). A larger variance component for condition means that condition contributes the most to the overall response variance. For L. monocytogenes, the variance component for “condition” was 64.2 (95% CI = 21.2, 245.2), while the variance components for “strain” and “strain-condition interaction” were 7.4 (95% CI = 0.0, 72.6) and 1.6 (95% CI = 0.0, 28.0), respectively. For S. enterica, the variance component for “condition” was 53.0 (95% CI = 0.0, 122.2), while the variance components for “strain” and “strain-condition interaction” were 15.9 (95% CI = 0.0, 51.0) and 26.5 (95% CI = 0.0, 107.4), respectively. For STEC, the variance component for “condition” was 126.7 (95% CI = 13.6, 274.9); while the variance components for “strain” and “strain-condition interaction” were 0.0 (95% CI = 0.0, 9.0) and 0.0 (95% CI = 0.0, 28.0), respectively. The reported variance components for L. monocytogenes, S. enterica and STEC indicate that pre-growth conditions have a larger effect on variation in responses than strain diversity.
Table 2. Variance components of crossed random effects model for L. monocytogenes, S. enterica, and STEC.
As four L. monocytogenes strains, each pre-grown under seven different conditions, were evaluated for survival of exposure to 60 ppm PAA for 45 s, die-off data for a total of 28 “strain-condition” combinations were created. Among these “strain-condition” combinations, die-off ranged from a low of 0.5 log (FSL J1-0031, growth under high salt) to a high of 6.4 log (FSL R9-5411, growth in defined minimal medium). The mean die-off rates for different conditions ranged from a low of 1.0 log (for pre-growth under salt stress) to a high of 5.6 log (for pre-growth in minimal media). The range of die-off values observed with different strains pre-grown under a single condition ranged from 0.5 to 1.8 log (1.3 log range) for pre-growth under salt stress to 2.5 to 4.6 log (2.1 log range) for pre-growth at 21°C.
The mean die-off rates for different strains ranged from a low of 2.1 log (for FSL J1-031, lineage III, 4a) to a high of 3.3 log (FSL R9-0506, lineage II, 1/2a). The range of die-off values observed for a given strain pre-grown under different conditions ranged from 0.5 to 4.5 log (4.0 log range) for strain FSL J1-031 to 1.0 to 6.4 log (5.4 log range) for strain FSL R9-5411. Consistent with the model data detailed in the previous section, the ranges of responses observed within a strain pre-grown under different conditions were considerable larger than the ranges of responses observed within a given condition. While this observation could be due to the fact that more conditions than strains were evaluated, the model results detailed above are not affected by the difference in number of strains (n = 4) and conditions assessed (n = 7).
The average response for the L. innocua strain (FSL C2-0008) lies within the range of response of the other strains for five of the conditions (i.e., pre-growth in defined minimal medium, at 21°C, at pH 5.5, to mid-log phase and high salt). When pre-grown to stationary phase L. innocua showed a 2.9 log reduction as compared to the average log reduction for the four L. monocytogenes strains, which ranged from 0.9 to 2.8 log. When pre-grown in reduced water activity, L. innocua showed numerically higher tolerance (1.5 log reduction) as compared to the range observed among the four L. monocytogenes strains (log reduction ranged from 2.0 to 3.8 log) (Figure 4; see Supplementary Table 6 for detailed log reduction data).
Figure 4. Average log reduction of four Listeria strains and one surrogate (L. innocua, FSL C2-0008) when pre-grown under different conditions prior to exposure to 60 ppm PAA for 45 s. Pre-growth conditions are shown on the X-axis and include pre-growth (i) at 21°C (21C), (ii) in defined minimal medium (DM), (iii) under reduced water activity (Glycerol), (iv) to mid-log phase (Midlog), (v) in 4.5% additional NaCl (NaCl), (vi) at pH 5.5 (pH5.5) and (vii) to stationary phase (Stationary). When calculating log reduction, values with count zero post-sanitizer treatment were substituted with the detection limit (e.g., 100 CFU/mL). Data represent averages from three trials; the standard deviation of the three trials and number of trials with values below detection limit after PAA exposure are listed in Supplementary Table 6.
As four S. enterica strains, each pre-grown under seven different conditions, were evaluated for survival of exposure to 40 ppm PAA for 45 s, die-off data for a total of 28 “strain-condition” combinations were created. Among these “strain-condition” combinations, die-off ranged from a low of 2.6 log (FSL R9-5344, growth under salt stress) to a high of 7.1 log (FSL R9-5272, growth in minimal medium). The mean die-off rates for different conditions ranged from a low of 3.1 log (for pre-growth under salt stress) to a high of 6.0 log (for pre-growth to mid-log phase). The range of die-off values observed with different strains pre-grown under a single condition ranged from 4.9 to 6.4 log (1.5 log range) for pre-growth at low pH to 4.8 to 7.1 log (2.3 log range) for pre-growth in minimal media.
The mean die-off rates for different strains ranged from a low of 4.6 log (for FSL R9-5502, Salmonella Poona) to a high of 6.2 log (FSL R9-5272, Salmonella Enteritidis). The range of die-off values observed for a given strain pre-grown under different conditions ranged from 4.3 to 7.1 log (2.8 log range) for strain FSL R9-5272 to 2.6 to 6.2 log (3.6 log range) for strain FSL R9-5344. Consistent with the model data detailed in the previous section, the ranges of responses observed within a strain pre-grown under different conditions are considerable larger than the ranges of responses observed within a given condition.
The average response for the avirulent S. enterica strain MHM112 lies within the range of response of the other strains for four of the conditions (i.e., pre-growth in reduced water activity, M9 minimal medium, to mid-log phase and stationary phase). When pre-grown under salt stress, at 21°C and pH 5.0, the avirulent S. enterica showed a numerically larger reduction after PAA exposure (5.4 log for salt stress, 4.0 log for 21°C and 4.4 log for pH 5.0) as compared to the average log reduction for the four S. enterica strains, which ranged from 2.6 to 4.3 log for salt stress, 4.8 to 6.8 log for 21°C and 4.9 to 6.4 for pH 5.0) (Figure 5 and see Supplementary Table 6 for detailed log reduction data). The average log reduction of surrogate organism E. faecium (ATCC 8459) for S. enterica was numerically lower across all conditions ranging from 0.0 to 0.08 log when the lowest log reduction for all other S. enterica ranged from 2.6 to 4.6 log, not including the 3.9 log reduction for E. faecium cells grown to mid-log phase which was also numerically lower than compared to 6.0 log reduction for all other S. enterica strains (Figure 5 and Supplementary Table 6). Log reduction data for E. faecium in minimal medium was not available due to the lack of growth in the medium.
Figure 5. Average log reduction of four S. enterica strains and two surrogate strains (avirulent Salmonella, MHM112 and E. faecium, ATCC8459) when pre-grown under different conditions prior to exposure to 40 ppm PAA for 45 s. Pre-growth conditions are shown on the X-axis and include pre-growth (i) at 21°C (21C), (ii) under reduced water activity (Glycerol), (iii) in minimal medium (M9), (iv) to mid-log phase (Midlog), (v) in 4.5% additional NaCl (NaCl), (vi) at pH 5.0 (pH5) and (vii) to stationary phase (Stationary). When calculating log reduction, values with count zero post-sanitizer treatment were substituted with the detection limit (e.g., 100 CFU/mL). Data represent averages from three trials; the standard deviation of the three trials and number of trials with values below detection limit after PAA exposure are listed in Supplementary Table 6.
As five STEC strains, each pre-grown under seven different conditions, were evaluated for survival of exposure to 40 ppm PAA for 45 s, die-off data for a total of 35 “strain-condition” combinations were created. Among these “strain-condition” combinations, die-off ranged from a low of 1.5 log (FSL R9-5271, growth under salt stress) to a high of 6.5 log (FSL R9-5516, FSL R9-5271, growth in minimal medium). The mean die-off rates for different conditions ranged from a low of 2.9 log (for pre-growth under salt stress and at 21°C) to a high of 6.5 log (for pre-growth in minimal media). The range of die-off values observed with different strains pre-grown under a single condition ranged from 5.3 to 6.1 log (0.8 log range) for pre-growth to mid-log phase to 1.5 to 4.2 log (2.7 log range) for pre-growth under salt stress.
The mean die-off rates for different strains ranged from a low of 4.2 log (for FSL R9-5516, O145) to a high of 4.6 log (FSL R9-5517, O103). The range of die-off values observed for a given strain pre-grown under different conditions ranged from 2.9 to 6.1 log (3.2 log range) for strain FSL R9-5258 to 1.5 to 6.5 log (5.0 log range) for strain FSL R9-5271. Consistent with the model data detailed in the previous section, the ranges of responses observed within a strain pre-grown under different conditions are considerable larger than the ranges of responses observed within a given condition.
The average response for the STEC surrogate E. coli TVS 353 lies within the range of response of the other strains for six conditions (i.e., pre-grown in reduced water activity, high salt, M9 minimal medium, pH 5.0, to mid-log phase and stationary phase). When pre-grown at 21°C, E. coli TVS 353 showed a 5.2 log reduction as compared to the average log reduction for the five STEC strains, which ranged from 2.0 to 3.9 log (Figure 6 and see Supplementary Table 6 for detailed log reduction data).
Figure 6. Average log reduction of five STEC strains and one surrogate (E. coli strain, TVS 353) when pre-grown under different conditions prior to exposure to 40 ppm PAA for 45 s. Pre-growth conditions are shown on the X-axis and include pre-growth (i) at 21°C (21C), (ii) under reduced water activity (Glycerol), (iii) in minimal medium (M9), (iv) to mid-log phase (Midlog), (v) in 4.5% additional NaCl (NaCl), (vi) at pH 5.0 (pH5) and (vii) to stationary phase (Stationary). When calculating log reduction, values with count zero post-sanitizer treatment were substituted with the detection limit (e.g., 100 CFU/mL). Data represent averages from three trials; the standard deviation of the three trials and number of trials with values below detection limit after PAA exposure are listed in Supplementary Table 6.
A modeling-based approach was used to assess the range of bacterial reductions expected when different combinations of strains and growth conditions were tested for PAA survival. Specifically, the 95% prediction intervals (PIs) for log reduction were estimated with a cross-random effects model where either (i) no effect was fixed (random combinations of strain and growth condition), (ii) the effect of a single strain was fixed (single strain with random selection of growth condition) or (iii) the effect of a single pre-growth condition was fixed (single condition with random selection of strains). For example, for L. monocytogenes when all strains and conditions were chosen at random and none of the effects were fixed, the PI covered a 8.7 log range. By comparison, the experimentally observed responses for L. monocytogenes covered a 5.9 log range (from a 0.5 log reduction observed for FSL J1-031 pre-grown under high salt to a 6.4 log-reduction observed for FSL R9-5411 pre-grown in minimal medium). The larger range for the model-based approach is due to the fact that the possible log reduction parameters cover a larger range than the observed log reductions (which represents the arithmetic mean of 3 replicates). The L. monocytogenes PIs for single strains pre-grown under different conditions ranged from 8.4 to 8.6 log (depending on strain), while PIs for single pre-growth conditions (each with multiple strains) ranged from a 6.3 to a 6.4 log reduction (depending on growth condition) (Figure 7A). For S. enterica, when all strains and conditions were chosen at random and none of the effects were fixed, the PI covered a 9.7 log range, for single strains pre-grown under different conditions PIs ranged from 9.7 to 9.9 log, while PIs for single pre-growth conditions ranged from 8.6 to 8.8 log (Figure 7B). For STEC, when all strains and conditions were chosen at random and none of the effects were fixed, the PI covered a 11.3 log range, for single strains pre-grown under different conditions PIs ranged from 11.1 to 11.3 log, while PIs for single pre-growth conditions ranged from 7.4 to 7.7 log (Figure 7C). Overall, the model predictions further support that assessment of a single strain pre-grown under different conditions captures a larger range of responses as compared to assessment of multiple strains pre-grown under a single condition.
Figure 7. Estimated ranges of log reduction after PAA exposure for different combinations of strains and pre-growth conditions. 95% prediction intervals for log reduction were estimated based on a crossed-random effects model where no effect was fixed (left panel, labeled “None”), the effect of strain was fixed, and conditions were random effects (middle panel, labeled “Strain”), and the effect of condition was fixed and strains were random effects (right panel, labeled “Condition”). The error bars of the 95% prediction intervals show the range of responses in log reduction (y-axis) for a given effect after exposure to PAA of (A) L. monocytogenes, (B) S. enterica, and (C) STEC.
With increasing requirements for science-based food safety strategies, industry needs scientifically sound approaches for validation studies, including selection of pathogen or surrogate strains used and the use of specific protocols for bacterial growth prior to validation studies. There is a particular need for pathogen and surrogate strain collections, as well as growth protocols for the produce industry as validation of intervention and control strategies is particularly important for this industry, which often lacks well established and validated pathogen control steps. Therefore, we assembled a produce-relevant bacterial strain collection that includes key pathogens as well as possible surrogate organisms, followed by WGS to characterize and validate the strains in this collection. In a proof of concept experiment, a subset of strains was further assessed, after pre-growth under different conditions, for survival of PAA exposure. These experiments provided clear evidence that growth conditions prior to challenge studies have a larger effect on phenotypic outcomes than strain diversity, suggesting that the use of a cocktail that contains strains pre-grown under different conditions may provide a valuable alternative to currently more typically used multi-strain cocktails pre-grown under a single condition.
The produce-relevant strain collection assembled includes 11 L. monocytogenes, 23 S. enterica, and 13 STEC, as well as 8 possible surrogate organisms. Selection of appropriate surrogate organisms can be controversial since it is necessary to determine whether the chosen organism emulates the target for each different use condition. While additional surrogates may hence need to be added, the current collection should represent an appropriate starting point. For example, L. innocua has been well established as a surrogate for L. monocytogenes including through studies where representatives of these two species showed similar (i) growth on baby spinach leaves (Omac et al., 2015), (ii) survival rates in soil (Girardin et al., 2005), and (iii) D and z-values for heat treatment at various temperatures (Friedly et al., 2008). While microbial strain collections for a variety of pathogens have been established [e.g., ILSI NA L. monocytogenes Strain Collection (Fugett et al., 2006); standard reference collection of STEC strains (Manning, 2011)], the collection here represents the first strain collection tailored to produce safety research. Broad use of this standard strain collection will also allow for better comparison of data across different studies. This will be facilitated by the fact that some of the strains in this collection have already been used, including studies of S. enterica survival on tomatoes (López-Velasco et al., 2012, 2013), antimicrobial treatments of broccoli and radish seeds (Landry et al., 2015), penetration of S. enterica into fruit (Danyluk et al., 2010), E. coli survival on lettuce (Bezanson et al., 2012; Weller et al., 2017b), and persistence on tomatoes, cantaloupe and spinach (de Moraes et al., 2016). In addition, initial characterization of selected surrogates in this collection for PAA sensitivity already provides some valuable information. For example, while a comparison of the avirulent Salmonella surrogate strain MHM112 with the other Salmonella for strains suggests the suitability of this strain as a surrogate organism for PAA treatment, use of E. faecium in challenge studies, at least with PAA, would typically underestimate the efficacy of PAA for Salmonella as this surrogate strain showed considerably higher PAA resistance as compared to Salmonella.
Acquisition of pathogen strains, particularly from international sources, is however, not trivial and hence this collection is biased towards strains from the United States, even though some strains from international sources are included, such as an E. coli O104:H4 (FSL R9-5256) from the sprout-associated outbreak in Germany 2011 (Ahmed et al., 2012). Lack of a broad geographical representation could be a particular challenge for S. enterica, where it has been established that some serotypes show strong geographical associations; for example, serotype Stanley is predominantly found in Thailand (Hendriksen et al., 2009) and was responsible for an outbreak linked to alfalfa sprouts (Werner et al., 2007). One could also consider including additional L. monocytogenes strains that represent the most prevalent clonal complexes (CC) worldwide for each lineage e.g., CC1, CC2 and CC3 for lineage I, as well as CC7 and CC9 for lineage II (Chenal-Francisque et al., 2011). Moreover, the collection could be extended to include spoilage organisms such as Pseudomonas strains to assess the effectiveness of sanitizer against (i) spoilage organisms or (ii) foodborne pathogens present in mixed biofilms that include Pseudomonas. While the strain collection described here provides a valuable starting point, it is anticipated that in the future other strains (e.g., from new outbreaks, international sources) will be added and that individual users may supplement the collection with specific strains of interest (e.g., strains previously linked to a facility or recalls of interest).
All strains included in the collection were characterized by WGS using KRAKEN and kSNP to confirm strain identity and to classify strains based on their genetic relationships, respectively. WGS data will also allow for future unambiguous identification of strains and strain verification, which is crucial when strains are distributed to other researchers with the associated risk of cross-contamination or mislabeling. This challenge was confirmed here as two isolates received did not match the expected serotype and needed to be re-acquired from a third party. Similarly, previous studies (Goto, 1992; Stacey, 2000; Madisch and Heim, 2007) have shown for bacterial isolates and viruses that mislabeling can occur at culture collections for type strains. Availability of a strain collection with associated publicly available WGS data will allow users to validate every strain upon receipt and/or over time, as well as after completion of experiments. For example, after PAA exposure surviving strains could have been characterized by WGS to identify if the sanitizer is selecting for strains with spontaneous mutations that confer an advantage. WGS data could also be used to develop strain specific primers, which would be valuable if confirmation of recovered challenge strains is needed (e.g., in field studies) (Tomás-Callejas et al., 2011; Naganandhini et al., 2015). Not unexpected, some genome sequence data represented a considerable number of contigs (>100), including all E. coli strains, which is consistent with previous observations that assembly of E. coli genomes from short read sequencing data is challenging, presumably due to the presence of a larger number of repeat regions (Higgins et al., 1982; Gilson et al., 1984; Brem, 2000; Williams et al., 2013). Future users of this collection may hence select to re-sequence some of the strains in this collection with long-read sequencing methods, e.g., PACBIO.
Whole genome sequencing data for strains included in a collection also provides an opportunity to screen strains for unusual mutations that may lead to phenotypes that are not representative of a specific species or serotype. Initial WGS-based screen and quality checks could include (i) identification of genes with premature stop codons, (ii) identification of non-synonymous changes in functional regions of key genes (e.g., stress response genes), and (iii) partial or full deletions of key genes. Identification of mutations that affect key phenotypes is particularly important if strains will be used for challenge studies, where mutations that affect stress response mechanisms may lead to overestimation of the effectiveness of an intervention. Bioinformatic evaluation of strains for mutations in key stress response (and virulence genes) is also important as many strains may have been passaged for a number of generations in rich media and hence are more likely to have undergone adaptation to a laboratory environment (Eydallin et al., 2014; Liu et al., 2017). Importantly, we identified premature stops codons in rpoS, which encodes a global stress regulator, in one Salmonella and one E. coli strain; mutations in this gene have previously been shown to lead to reduced stress resistance (Leenanon and Drake, 2001; Kabir et al., 2004). A previous study of human, spinach, and environmental E. coli O157:H7 isolates associated with the 2006 spinach outbreak in the United States, also reported that three human isolates as well as two spinach isolates tested carried rpoS mutations and showed considerably reduced acid tolerance as compared to environmental and spinach isolates without rpoS mutations (Parker et al., 2012).
Strains can also be modified to ease the recovery of a specific strain from a mixed-sample e.g., selection for antibiotic resistance or incorporation of antibiotic resistance genes into the chromosome. Three strains in the collection (FSL R9-5251, FSL R9-5220, FSL R9-6567) had been selected for resistance to nalidixic acid or rifampicin; for these antibiotics, a single point mutation is sufficient to confer resistance. However, antibiotic resistance can lead to pleiotropic effects resulting in dramatic changes in the phenotype which has to be considered when drawing conclusions from challenge or validation studies using the antibiotic resistant strain instead of the wildtype. Also, multiple additional mutations may be present in strains selected for antibiotic resistance, including compensatory mutations that occurred subsequently to the mutation that conferred antibiotic resistance (Robinson et al., 2015; Collery et al., 2017). Indeed, additional non-synonymous mutations were identified in two out of three strains. For example, in strain FSL R9-6567, a non-synonymous change was found in flhE, which is a periplasmatic protein that regulates flagellar biosynthesis. As deletion of flhE in S. enterica has previously been shown to cause a proton leak and changes in the outer membrane (Lee et al., 2015), it may be necessary to assess the consequences of this (or other) non-synonymous mutations before including strains in experimental studies.
Currently, challenge and validation studies are typically conducted with multiple strains, which may be used separately or as a mixture of multiple strains (so called “cocktails”) (Scott et al., 2005). This approach allows to account for strain diversity and to assure that control strategies are designed to deliver the appropriate or required protection even with more tolerant strains. Strains are often selected to represent outbreaks or food sources relevant to a given challenge study (e.g., studies on lettuce would use strains from lettuce or lettuce-associated outbreaks) and strains typically are pre-grown under a single condition. In this study, it was shown that pre-growth conditions have a larger effect on the range of phenotypic responses than strain diversity. Inclusion of different pre-growth conditions in challenge and validation studies is particularly important if it is not possible to define the specific physiological status of bacteria in the natural environments and contamination events relevant for a given challenge study, which may often be the case in the produce industry.
However, the importance of including strain diversity in challenge studies cannot be neglected. In this study, the importance of strain diversity was particularly evident for S. enterica, where even though the variance component for “condition” was very large, the variance component for “strain-condition interaction” was larger than for the other two pathogen groups, indicating that the interaction between strain diversity and pre-growth conditions had an impact on PAA survival. These findings for S. enterica could at least be partially due to the fact that the S. enterica strains included in the collection appear to represent larger genomic diversity (as, for example, supported by larger variation in genome size, suggesting a larger accessory genome) than the STEC and L. monocytogenes strains. While a number of previous studies have also shown strain variation with regard to bacterial survival of stress conditions e.g., salt or acid stress (Lianou et al., 2006; Hingston et al., 2017), in many cases this is driven by a few strains that showed extremely high sensitivity to a given stress, which often could be tracked to mutations in key genes (e.g., stress response genes) (Parker et al., 2012; Kovacevic et al., 2013). In other cases, strains do clearly differ in their tolerance to food relevant stress conditions, even though the magnitude of variation is often comparatively small and therefore may be of limited practical relevance. For example, among 101 L. monocytogenes isolates the minimum inhibitory concentration (MIC) to benzalkonium chloride, a quaternary ammonium compound, ranged from 5 to 13 ppm for isolates containing the resistance genes qacH or bcrABC, while isolates without these genes showed MICs ≤ 5 ppm (Møretrø et al., 2017). While this suggests that strains with and without these resistance genes should have a low tolerance to quaternary ammonium at the typical use concentrations, which are at least 200 ppm, one could argue that reduced sensitivity to low quaternary ammonium concentrations could still be relevant as pathogens in processing plants may sometimes only be reached by diluted sanitizer.
There are, however, also a few clear examples of foodborne pathogen strains that show considerably enhanced tolerance to specific food-associated stress conditions, such as S. enterica and E. coli strains that encode a heat resistance islet (LHR), which appears to considerably enhance heat resistance; up to 3 log decreased survival of heat stress was reported in E. coli when one of the three genes yfdX1, yfdX2, and hdeD on LHR1 were deleted (Mercer et al., 2017). Even though the contribution of strain diversity to phenotypic variation are on average smaller than the contributions of growth conditions, inclusion of highly tolerant strains in challenge studies as well as inclusion of strain diversity thus remains important. This leads to the proposal that future challenge studies should consider including multiple strains, but with each pre-grown under a different condition. If conditions that organisms are typically exposed to are known and well defined (e.g., dry inoculation for nuts [Blessington et al., 2013]), these conditions should be used for or included among the pre-growth conditions. Similarly, if a specific sanitizer is repeatedly used in a processing facility, validation studies should assess if pathogens are adapting to low level concentrations of the sanitizer over time. For experiments on sanitizer survival, it may also be appropriate to include pre-growth under exposure to sub-lethal sanitizer levels. Overall, if “typical” pre-growth conditions are unknown or cannot easily be defined, pre-growth under different conditions, focusing on those that have shown to increase stress tolerance, may be appropriate, which is consistent with prior recommendations to grow the inoculum to stationary phase, which typically leads to more stress tolerant bacterial cells (Beuchat et al., 2001).
Interestingly, in this study strains consistently showed highest PAA tolerance when pre-grown under high salt (mean die-off was 1.0 log for L. monocytogenes, 3.1 log for S. enterica and 2.9 log for STEC) and least tolerance when pre-grown in minimal medium (mean die-off 5.6 log for L. monocytogenes and 6.0 log for STEC) or mid-log phase (mean die-off 6.0 log for S. enterica). These findings are consistent with a number of studies that have shown cross-protection of bacteria exposed to one stress (e.g., salt stress) to subsequent exposure to another stress (e.g., oxidative stress). For example, L. monocytogenes pre-adapted in 6% NaCl became more tolerant when exposed to 50 mM H2O2 than compared to control cultures (Bergholz et al., 2012). In another study, pre-growth of L. monocytogenes under osmotic stress lead to increased heat resistance (Jørgensen et al., 1995), as well as nisin and bile salt tolerance (Begley et al., 2002; Bergholz et al., 2013). Examples of cross-protection, by other stresses, against additional food relevant stress conditions include increased heat resistance after acid adaptation in S. enterica and E. coli (Leyer and Johnson, 1993; Haberbeck et al., 2017), increased survival of filamentous S. enterica in low pH and during desiccation, when pre-grown in reduced water activity (Stackhouse et al., 2012). However, exposure to one stress, does not always provide enhanced tolerance to another stress; for example, it has been shown for S. enterica that adaptation to acid stress leads to increased sensitivity to subsequent oxidative stress (Greenacre et al., 2006) due to the downregulation of the transcription factor OxyR, which is crucial in oxidative stress response (Christman et al., 1985). Overall, this supports the importance of pre-growth under multiple conditions rather than focusing on pre-growth in a single condition (e.g., salt), as different pre-growth conditions may enhance tolerance to different given interventions. Our data indicate though that certain pre-growth conditions (e.g., mid-log phase, minimal media) may generally lead to less stress tolerant bacterial cells and hence should potentially be excluded as an appropriate pre-growth condition. This is consistent with data that show reduced stress tolerance of log phase cells during osmotic stress as compared to stationary phase cells (Jenkins et al., 1990). Similarly, reduced stress tolerance to high osmolarity had previously been shown for L. monocytogenes that was pre-grown in defined minimal medium as compared to pre-growth in BHI (Maria-Rosario et al., 1995). This is likely due to accumulation of compatible solutes, such as glycine betaine and carnitine, that are present in full media (e.g., BHI); accumulation of these compounds helps stabilize enzymes and proteins, thus ensuring their continuous function in adverse conditions (Mendum and Smith, 2002).
Overall, our data indicate that conditions used to grow bacterial strains prior to challenge studies have, on average, a larger effect on challenge study outcomes and survival as compared to strain diversity. Strain diversity parameters that may affect stress tolerance and survival of interventions can often be linked to mutations in stress response genes that can be easily identified with appropriate bioinformatic approaches as long as genome sequence data are available, as is the case for all strains included in the collection described here. However, strains that show “hyper-tolerance” to certain stress conditions do exist and their inclusion in challenge sets is important, particularly if challenge studies are intended to identify “worst case scenarios” (i.e., highest resistance to be expected among naturally occurring pathogen strains). Based on the data available to date, we suggest that challenge studies may want to utilize a 5-strain cocktail with (i) each strain confirmed to not have known mutations in key relevant stress response and other genes and where (ii) each strain is pre-grown under a different condition (possibly excluding conditions that are well established to yield hyper-tolerant strains, e.g., log phase); under some circumstances, random number generators could be used to select the 5 conditions to be used from a larger set of possible and valid pre-growth conditions. In addition, where existence of strains that are hyper-tolerant has been established, these should be included when appropriate for the stress condition or intervention evaluated. However, consistent with prior recommendations, if a given challenge study targets a product pathogen combination where pathogens are expected to be in very specific and well defined physiological state (e.g., low water activity in dry foods), pre-adaptation of all strains in cocktail to these conditions would typically still be warranted.
The datasets and code for analyses for this study can be found in the CPS-strain-collection repository on github (https://github.com/FiaHa/CPS-strain-collection).
AH, VG-O, and MW designed the study. AH conducted the experiments. AH, JK, LC, and DK performed the bioinformatical and statistical analyses. AH and MW wrote the manuscript.
This research was supported by the Center for Produce Safety, Award no. 2016CPS10 (awarded to MW) and by the U.S. Department of Agriculture’s (USDA) Agricultural Marketing Service through Grant 15-SCBGP-CA-0046. Its contents are solely the responsibility of the authors and do not necessarily represent the official views of the USDA.
The authors declare that the research was conducted in the absence of any commercial or financial relationships that could be construed as a potential conflict of interest.
We appreciate the contributions of the reviewers of our strain collection drafts and contributors to the strain collection P. Gerner-Smidt, M. Danyluk, L. Harris, R. Worobo, K. Nightingale, M. de Moraes, M. Allard, P. Fratamico, T. Monson, K. Musser, P. Griffin, M. Parish, S. Assar, M. Sharma, E. Brown, R. Mandrell, B. Sauders, L. Gorski, E. Berry, R. Boyer, L. Beuchat, T. Suslow, J. Farber, F. Yiannas, J. Brennan, B. Whitaker, D. McDonald, K. Refsnider, T. Stoltenberg, G. Keller, and J. Reed. We are grateful for the technical assistance of Maureen Gunderson, Jeff Tokman, and Jordan Skeens. We are also thankful for the support from the Cornell Statistical Consultant Unit.
The Supplementary Material for this article can be found online at: https://www.frontiersin.org/articles/10.3389/fmicb.2019.01223/full#supplementary-material
Ahmed, S. A., Awosika, J., Baldwin, C., Bishop-Lilly, K. A., Biswas, B., Broomall, S., et al. (2012). Genomic comparison of Escherichia coli O104:H4 isolates from 2009 and 2011 reveals plasmid, and prophage heterogeneity, including shiga toxin encoding phage stx2. PLoS One 7:e48228. doi: 10.1371/journal.pone.0048228
Andino, A., and Hanning, I. (2015). Salmonella enterica: survival, colonization, and virulence differences among serovars. Sci. World J. 2015:520179.
Angelo, K. M., Conrad, A. R., Saupe, A., Dragoo, H., West, N., Sorenson, A., et al. (2017). Multistate outbreak of Listeria monocytogenes infections linked to whole apples used in commercially produced, prepackaged caramel apples: United States, 2014–2015. Epidemiol. Infect. 145, 848–856. doi: 10.1017/s0950268816003083
Asakura, H., Makino, S., Takagi, T., Kuri, A., Kurazono, T., Watarai, M., et al. (2002). Passage in mice causes a change in the ability of Salmonella enterica serovar Oranienburg to survive NaCl osmotic stress: resuscitation from the viable but non-culturable state. FEMS Microbiol. Lett. 212, 87–93. doi: 10.1016/s0378-1097(02)00711-5
Atwill, E. R., Chase, J. A., Oryang, D., Bond, R. F., Koike, S. T., Cahn, M. D., et al. (2015). Transfer of Escherichia coli O157:H7 from simulated wildlife scat onto romaine lettuce during foliar irrigation. J. Food Prot. 78, 240–247. doi: 10.4315/0362-028X.JFP-14-277
Bankevich, A., Nurk, S., Antipov, D., Gurevich, A. A., Dvorkin, M., Kulikov, A. S., et al. (2012). SPAdes: a new genome assembly algorithm and its applications to single-cell sequencing. J. Comput. Biol. 19, 455–477. doi: 10.1089/cmb.2012.0021
Bates, D., Mächler, M., Bolker, B., and Walker, S. (2015). Fitting linear mixed-effects models using lme4. J. Stat. Softw. 67:48.
Begley, M., Gahan, C. G. M., and Hill, C. (2002). Bile stress response in Listeria monocytogenes LO28: adaptation, cross-protection, and identification of genetic loci involved in bile resistance. Appl. Environ. Microbiol. 68, 6005–6012. doi: 10.1128/aem.68.12.6005-6012.2002
Bergholz, T. M., Bowen, B., Wiedmann, M., and Boor, K. J. (2012). Listeria monocytogenes shows temperature-dependent and -independent responses to salt stress, including responses that induce cross-protection against other stresses. Appl. Environ. Microbiol. 78, 2602–2612. doi: 10.1128/AEM.07658-11
Bergholz, T. M., Den Bakker, H. C., Fortes, E. D., Boor, K. J., and Wiedmann, M. (2010). Salt stress phenotypes in Listeria monocytogenes vary by genetic lineage and temperature. Foodborne Pathog. Dis. 7, 1537–1549. doi: 10.1089/fpd.2010.0624
Bergholz, T. M., Tang, S., Wiedmann, M., and Boor, K. J. (2013). Nisin resistance of Listeria monocytogenes is increased by exposure to salt stress and is mediated via LiaR. Appl. Environ. Microbiol. 79, 5682–5688. doi: 10.1128/AEM.01797-13
Beuchat, L. R., Farber, J. M., Garrett, E. H., Harris, L. J., Parish, M. E., Suslow, T. V., et al. (2001). Standardization of a method to determine the efficacy of sanitizers in inactivating human pathogenic microorganisms on raw fruits and vegetables. J. Food Prot. 64, 1079–1084. doi: 10.4315/0362-028x-64.7.1079
Bezanson, G., Delaquis, P., Bach, S., Mckellar, R., Topp, E., Gill, A., et al. (2012). Comparative examination of Escherichia coli O157:H7 survival on romaine lettuce and in soil at two independent experimental sites. J. Food Prot. 75, 480–487. doi: 10.4315/0362-028X.JFP-11-306
Blessington, T., Theofel, C. G., and Harris, L. J. (2013). A dry-inoculation method for nut kernels. Food Microbiol. 33, 292–297. doi: 10.1016/j.fm.2012.09.009
Bolger, A. M., Lohse, M., and Usadel, B. (2014). Trimmomatic: a flexible trimmer for illumina sequence data. Bioinformatics 30, 2114–2120. doi: 10.1093/bioinformatics/btu170
Burall, L. S., Grim, C. J., and Datta, A. R. (2017). A clade of Listeria monocytogenes serotype 4b variant strains linked to recent listeriosis outbreaks associated with produce from a defined geographic region in the US. PLoS One 12:e0176912. doi: 10.1371/journal.pone.0176912
Cai, S., Worobo, R. W., and Snyder, A. B. (2018). Outgraded produce variably retains surface inoculated Escherichia coli through washing. Int. J. Food Microbiol. 269, 27–35. doi: 10.1016/j.ijfoodmicro.2018.01.012
Carter, M. Q., Parker, C. T., Louie, J. W., Huynh, S., Fagerquist, C. K., and Mandrell, R. E. (2012). RcsB contributes to the distinct stress fitness among Escherichia coli O157:H7 curli variants of the 1993 hamburger-associated outbreak strains. Appl. Environ. Microbiol. 78, 7706–7719. doi: 10.1128/AEM.02157-12
Centers for Disease Control and Prevention [CDC] (1989). Listeriosis associated with consumption of turkey franks. MMWR 38, 267–268.
Centers for Disease Control and Prevention [CDC] (2002a). Multistate outbreaks of Salmonella serotype poona infections associated with eating cantaloupe from Mexico–United States and Canada, 2000-2002. MMWR 51, 1044–1047.
Centers for Disease Control and Prevention [CDC] (2002b). Outbreak of multidrug-resistant Salmonella newport–United States, January-April 2002. MMWR 51, 545–548.
Centers for Disease Control and Prevention [CDC] (2006). Ongoing multistate outbreak of Escherichia coli serotype O157:H7 infections associated with consumption of fresh spinach–United States, September 2006. MMWR 55, 1045–1046.
Centers for Disease Control and Prevention [CDC] (2007). Multistate outbreak of Salmonella serotype Tennessee infections associated with peanut butter–United States, 2006-2007. MMWR 56, 521–524.
Centers for Disease Control and Prevention [CDC] (2008a). Multistate outbreak of Salmonella Litchfield infections linked to cantaloupes (Final Update). Available at: https://www.cdc.gov/salmonella/2008/cantaloupes-4-2-2008.html (accessed July 27, 2018).
Centers for Disease Control and Prevention [CDC] (2008b). Outbreak of Salmonella serotype Saintpaul infections associated with multiple raw produce items–United States, 2008. MMWR 57, 929–934.
Centers for Disease Control and Prevention [CDC] (2009). Multistate outbreak of Salmonella infections associated with peanut butter and peanut butter-containing products–United States, 2008-2009. MMWR 58, 85–90.
Centers for Disease Control and Prevention [CDC] (2010). Multistate outbreak of human E. coli O145 infections linked to shredded romaine lettuce from a single processing facility (Final Update). Available at: https://www.cdc.gov/ecoli/2010/shredded-romaine-5-21-10.html (accessed July 29, 2018).
Centers for Disease Control and Prevention [CDC] (2011a). Multistate outbreak of human Salmonella I 4,[5],12:i:- infections linked to alfalfa sprouts (Final Update). Available at: https://www.cdc.gov/salmonella/2010/alfalfa-sprouts-2-10-11.html (accessed July 27, 2018).
Centers for Disease Control and Prevention [CDC] (2011b). Multistate outbreak of listeriosis associated with Jensen Farms cantaloupe–United States, August-September 2011. MMWR 60, 1357–1358.
Centers for Disease Control and Prevention [CDC] (2012a). Multistate outbreak of shiga toxin-producing Escherichia coli O26 infections linked to raw clover sprouts at Jimmy John’s restaurants (Final Update). Available at: https://www.cdc.gov/ecoli/2012/o26-02-12/index.html (accessed July 29, 2018).
Centers for Disease Control and Prevention [CDC] (2012b). Notes from the field: human Salmonella Infantis infections linked to dry dog food–United States and Canada, 2012. MMWR 61:436.
Centers for Disease Control and Prevention [CDC] (2014). Multistate outbreak of shiga toxin-producing Escherichia coli O121 infections linked to raw clover sprouts (Final Update). Available at: https://www.cdc.gov/ecoli/2014/o121-05-14/index.html (accessed July 29, 2018).
Centers for Disease Control and Prevention [CDC] (2015a). Multistate outbreak of Salmonella Enteritidis infections linked to bean sprouts (Final Update). Available at: https://www.cdc.gov/salmonella/enteritidis-11-14/index.html (accessed July 29, 2018).
Centers for Disease Control and Prevention [CDC] (2015b). Multistate Outbreak of Salmonella Poona Infections Linked to Imported Cucumbers (Final Update). Available at: https://www.cdc.gov/salmonella/poona-09-15/index.html (accessed July 27, 2018).
Centers for Disease Control and Prevention [CDC] (2015c). Wholesome soy products, Inc. Sprouts and investigation of human listeriosis cases (Final Update). Available at: https://www.cdc.gov/listeria/outbreaks/bean-sprouts-11-14/index.html (accessed July 29,2018).
Centers for Disease Control and Prevention [CDC] (2016a). Multistate outbreak of Salmonella infections linked to alfalfa sprouts from one contaminated seed lot (Final Update). Available at: http://www.cdc.gov/salmonella/muenchen-02-16/ (accessed July 27, 2018).
Centers for Disease Control and Prevention [CDC] (2016b). Multistate outbreak of shiga toxin-producing Escherichia coli O157 infections linked to alfalfa sprouts produced by Jack & The Green Sprouts (Final Update). Available at: https://www.cdc.gov/ecoli/2016/o157-02-16/index.html (accessed July 29, 2018).
Chenal-Francisque, V., Lopez, J., Cantinelli, T., Caro, V., Tran, C., Leclercq, A., et al. (2011). Worldwide distribution of major clones of Listeria monocytogenes. Emerg. Infect. Dis. 17, 1110–1112. doi: 10.3201/eid/1706.101778
Cheng, C., Yang, Y., Dong, Z., Wang, X., Fang, C., Yang, M., et al. (2015). Listeria monocytogenes varies among strains to maintain intracellular pH homeostasis under stresses by different acids as analyzed by a high-throughput microplate-based fluorometry. Front. Microbiol. 6:15. doi: 10.3389/fmicb.2015.00015
Christman, M. F., Morgan, R. W., Jacobson, F. S., and Ames, B. N. (1985). Positive control of a regulon for defenses against oxidative stress and some heat-shock proteins in Salmonella Typhimurium. Cell 41, 753–762. doi: 10.1016/s0092-8674(85)80056-8
Clark, W. A., and Geary, D. H. (1974). The story of the American type culture collection–its history and development (1899-1973). Adv. Appl. Microbiol. 17, 295–309. doi: 10.1016/s0065-2164(08)70562-8
Collery, M. M., Kuehne, S. A., Mcbride, S. M., Kelly, M. L., Monot, M., Cockayne, A., et al. (2017). What’s a SNP between friends: The influence of single nucleotide polymorphisms on virulence and phenotypes of Clostridium difficile strain 630 and derivatives. Virulence 8, 767–781. doi: 10.1080/21505594.2016.1237333
Danyluk, M. D., Interiano Villeda, L. O., Friedrich, L. M., Schneider, K. R., and Etxeberria, E. (2010). Natural-light labeling of tomatoes does not facilitate growth or penetration of Salmonella into the fruit. J. Food Prot. 73, 2276–2280. doi: 10.4315/0362-028x-73.12.2276
Danyluk, M. D., Jones, T. M., Abd, S. J., Schlitt-Dittrich, F., Jacobs, M., and Harris, L. J. (2007). Prevalence and amounts of Salmonella found on raw California almonds. J. Food Prot. 70, 820–827. doi: 10.4315/0362-028x-70.4.820
Davis, S., Pettengill, J. B., Luo, Y., Payne, J., Shpuntoff, A., Rand, H., et al. (2015). CFSAN SNP Pipeline: an automated method for constructing SNP matrices from next-generation sequence data. PeerJ. Comput. Sci. 1:e20. doi: 10.7717/peerj-cs.20
De Jesus, A. J., and Whiting, R. C. (2003). Thermal inactivation, growth, and survival studies of Listeria monocytogenes strains belonging to three distinct genotypic lineages. J. Food Prot. 66, 1611–1617. doi: 10.4315/0362-028x-66.9.1611
de Moraes, M. H., Chapin, T. K., Ginn, A., Wright, A. C., Parker, K., Hoffman, C., et al. (2016). Development of an avirulent Salmonella surrogate for modeling pathogen behavior in pre- and postharvest environments. Appl. Environ. Microbiol. 82, 4100–4111. doi: 10.1128/AEM.00898-16
Edgar, R. C. (2004). MUSCLE: a multiple sequence alignment method with reduced time and space complexity. BMC Bioinform. 5:113. doi: 10.1186/1471-2105-5-113
Eydallin, G., Ryall, B., Maharjan, R., and Ferenci, T. (2014). The nature of laboratory domestication changes in freshly isolated Escherichia coli strains. Environ. Microbiol. 16, 813–828. doi: 10.1111/1462-2920.12208
Foodborne Diseases Active Surveillance and Network (2017). FoodNet 2015 Surveillance Report (Final Data). Atlanta: CDC.
Friedly, E. C., Crandall, P. G., Ricke, S., O’bryan, C. A., Martin, E. M., and Boyd, L. M. (2008). Identification of Listeria innocua surrogates for Listeria monocytogenes in hamburger patties. J Food Sci 73, M174–M178. doi: 10.1111/j.1750-3841.2008.00719.x
Fugett, E., Fortes, E., Nnoka, C., and Wiedmann, M. (2006). International life sciences institute North America Listeria monocytogenes strain collection: development of standard Listeria monocytogenes strain sets for research and validation studies. J. Food Prot. 69, 2929–2938. doi: 10.4315/0362-028x-69.12.2929
Gardner, S. N., Slezak, T., and Hall, B. G. (2015). kSNP3.0: SNP detection and phylogenetic analysis of genomes without genome alignment or reference genome. Bioinformatics 31, 2877–2878. doi: 10.1093/bioinformatics/btv271
Gawande, P. V., and Griffiths, M. W. (2005). Effects of environmental stresses on the activities of the uspA, grpE and rpoS promoters of Escherichia coli O157:H7. Int. J. Food Microbiol. 99, 91–98. doi: 10.1016/j.ijfoodmicro.2004.06.012
Gilson, E., Clement, J. M., Brutlag, D., and Hofnung, M. (1984). A family of dispersed repetitive extragenic palindromic DNA sequences in E. coli. EMBO J. 3, 1417–1421. doi: 10.1002/j.1460-2075.1984.tb01986.x
Girardin, H., Morris, C. E., Albagnac, C., Dreux, N., Glaux, C., and Nguyen-The, C. (2005). Behaviour of the pathogen surrogates Listeria innocua and Clostridium sporogenes during production of parsley in fields fertilized with contaminated amendments. FEMS Microbiol. Ecol. 54, 287–295. doi: 10.1016/j.femsec.2005.04.003
Goepfert, J. M., Iskander, I. K., and Amundson, C. H. (1970). Relation of the heat resistance of Salmonella to the water activity of the environment. Appl. Microbiol. 19, 429–433.
Gonzalez, R. J., Luo, Y., Ruiz-Cruz, S., and Mcevoy, J. L. (2004). Efficacy of sanitizers to inactivate Escherichia coli O157:H7 on fresh-cut carrot shreds under simulated process water conditions. J. Food Prot. 67, 2375–2380. doi: 10.4315/0362-028x-67.11.2375
Goto, M. (1992). Taxonomic confusion of Pseudomonas cissicola originated from mislabeling by international type culture collections. Int. J. Syst. Bacteriol. 42, 503–505. doi: 10.1099/00207713-42-3-503
Gould, L. H., Mody, R. K., Ong, K. L., Clogher, P., Cronquist, A. B., Garman, K. N., et al. (2013). Increased recognition of non-O157 shiga toxin–producing Escherichia coli infections in the United States during 2000–2010: epidemiologic features and comparison with E. coli O157 infections. Foodborne Pathog. Dis. 10, 453–460. doi: 10.1089/fpd.2012.1401
Green, M. R., and Sambrook, J. (2012). Molecular cloning : A laboratory Manual, 4th Edn. New York, NY: Cold Spring Harb Lab Press.
Greenacre, E. J., Lucchini, S., Hinton, J. C. D., and Brocklehurst, T. F. (2006). The lactic acid-induced acid tolerance response in Salmonella enterica serovar Typhimurium induces sensitivity to hydrogen peroxide. Appl. Environ. Microbiol. 72, 5623–5625. doi: 10.1128/aem.00538-06
Greene, S. K., Daly, E. R., Talbot, E. A., Demma, L. J., Holzbauer, S., Patel, N. J., et al. (2008). Recurrent multistate outbreak of Salmonella Newport associated with tomatoes from contaminated fields, 2005. Epidemiol. Infect. 136, 157–165. doi: 10.1017/s095026880700859x
Greenspan, F. P., and Margulies, P. H. (1950). Peroxy acid treatment to control pathogenic organisms on growing plants. US patent 2,512,640. Buffalo Electro-Chemical Co.,
Gruzdev, N., Pinto, R., and Sela, S. (2011). Effect of desiccation on tolerance of Salmonella enterica to multiple stresses. Appl. Environ. Microbiol. 77, 1667–1673. doi: 10.1128/AEM.02156-10
Gurevich, A., Saveliev, V., Vyahhi, N., and Tesler, G. (2013). QUAST: quality assessment tool for genome assemblies. Bioinformatics 29, 1072–1075. doi: 10.1093/bioinformatics/btt086
Haberbeck, L. U., Wang, X., Michiels, C., Devlieghere, F., Uyttendaele, M., and Geeraerd, A. H. (2017). Cross-protection between controlled acid-adaptation and thermal inactivation for 48 Escherichia coli strains. Int. J. Food Microbiol. 241, 206–214. doi: 10.1016/j.ijfoodmicro.2016.10.006
He, Y., Li, Y., Salazar, J. K., Yang, J., Tortorello, M. L., and Zhang, W. (2013). Increased water activity reduces the thermal resistance of Salmonella enterica in peanut butter. Appl. Environ. Microbiol. 79, 4763–4767. doi: 10.1128/AEM.01028-13
Hellstrom, S., Kervinen, R., Lyly, M., Ahvenainen-Rantala, R., and Korkeala, H. (2006). Efficacy of disinfectants to reduce Listeria monocytogenes on precut iceberg lettuce. J. Food Prot. 69, 1565–1570. doi: 10.4315/0362-028x-69.7.1565
Hendriksen, R. S., Bangtrakulnonth, A., Pulsrikarn, C., Pornruangwong, S., Noppornphan, G., Emborg, H. D., et al. (2009). Risk factors and epidemiology of the ten most common Salmonella serovars from patients in Thailand: 2002-2007. Foodborne Pathog. Dis. 6, 1009–1019. doi: 10.1089/fpd.2008.0245
Higgins, C. F., Ames, G. F., Barnes, W. M., Clement, J. M., and Hofnung, M. (1982). A novel intercistronic regulatory element of prokaryotic operons. Nature 298, 760–762. doi: 10.1038/298760a0
Hingston, P., Chen, J., Dhillon, B. K., Laing, C., Bertelli, C., Gannon, V., et al. (2017). Genotypes associated with Listeria monocytogenes isolates displaying impaired or enhanced tolerances to cold, salt, acid, or desiccation stress. Front. Microbiol. 8:369. doi: 10.3389/fmicb.2017.00369
Iram, S. H., and Cronan, J. E. (2006). The beta-oxidation systems of Escherichia coli and Salmonella enterica are not functionally equivalent. J. Bact. 188, 599–608. doi: 10.1128/jb.188.2.599-608.2006
Isaacs, S., Aramini, J., Ciebin, B., Farrar, J. A., Ahmed, R., Middleton, D., et al. (2005). An international outbreak of salmonellosis associated with raw almonds contaminated with a rare phage type of Salmonella Enteritidis. J. Food Prot. 68, 191–198. doi: 10.4315/0362-028x-68.1.191
Jain, S., Bidol, S. A., Austin, J. L., Berl, E., Elson, F., Lemaile-Williams, M., et al. (2009). Multistate outbreak of Salmonella Typhimurium and Saintpaul infections associated with unpasteurized orange juice–United States, 2005. Clin. Infect. Dis. 48, 1065–1071. doi: 10.1086/597397
Jenkins, D. E., Chaisson, S. A., and Matin, A. (1990). Starvation-induced cross protection against osmotic challenge in Escherichia coli. J. Bacteriol. 172, 2779–2781. doi: 10.1128/jb.172.5.2779-2781.1990
Jenkins, D. E., Schultz, J. E., and Matin, A. (1988). Starvation-induced cross protection against heat or H2O2 challenge in Escherichia coli. J. Bacteriol. 170, 3910–3914. doi: 10.1128/jb.170.9.3910-3914.1988
Joensen, K. G., Tetzschner, A. M., Iguchi, A., Aarestrup, F. M., and Scheutz, F. (2015). Rapid and easy in silico serotyping of Escherichia coli isolates by use of whole-genome sequencing data. J. Clin. Microbiol. 53, 2410–2426. doi: 10.1128/JCM.00008-15
Jørgensen, F. J., Stephens, P. J., and Knöchel, S. (1995). The effect of osmotic shock and subsequent adaption on the thermotolerance and cell morphology of Listeria monocytogenes. J. Appl. Bact. 79, 274–281. doi: 10.1111/j.1365-2672.1995.tb03137.x
Kabir, M. S., Sagara, T., Oshima, T., Kawagoe, Y., Mori, H., Tsunedomi, R., et al. (2004). Effects of mutations in the rpoS gene on cell viability and global gene expression under nitrogen starvation in Escherichia coli. Microbiology 150, 2543–2553. doi: 10.1099/mic.0.27012-0
Kenyon, W. J., Sayers, D. G., Humphreys, S., Roberts, M., and Spector, M. P. (2002). The starvation-stress response of Salmonella enterica serovar Typhimurium requires sigma(E)-, but not CpxR-regulated extracytoplasmic functions. Microbiology 148, 113–122. doi: 10.1099/00221287-148-1-113
Kopit, L. M., Kim, E. B., Siezen, R. J., Harris, L. J., and Marco, M. L. (2014). Safety of the surrogate microorganism Enterococcus faecium NRRL B-2354 for use in thermal process validation. Appl. Environ. Microbiol. 80, 1899–1909. doi: 10.1128/AEM.03859-13
Kovacevic, J., Arguedas-Villa, C., Wozniak, A., Tasara, T., and Allen, K. J. (2013). Examination of food chain-derived Listeria monocytogenes strains of different serotypes reveals considerable diversity in inlA genotypes, mutability, and adaptation to cold temperatures. Appl. Environ. Microbiol. 79, 1915–1922. doi: 10.1128/AEM.03341-12
Landry, K. S., Micheli, S., Mcclements, D. J., and Mclandsborough, L. (2015). Effectiveness of a spontaneous carvacrol nanoemulsion against Salmonella enterica Enteritidis and Escherichia coli O157:H7 on contaminated broccoli and radish seeds. Food Microbiol. 51, 10–17. doi: 10.1016/j.fm.2015.04.006
Lee, J., Monzingo, A. F., Keatinge-Clay, A. T., and Harshey, R. M. (2015). Structure of Salmonella FlhE, conserved member of a flagellar Type III secretion operon. J. Mol. Biol. 427, 1254–1262. doi: 10.1016/j.jmb.2014.11.022
Lee, K., French, N. P., Jones, G., Hara-Kudo, Y., Iyoda, S., Kobayashi, H., et al. (2012). Variation in stress resistance patterns among stx genotypes and genetic lineages of shiga toxin-producing Escherichia coli O157. Appl. Environ. Microbiol. 78, 3361–3368. doi: 10.1128/AEM.06646-11
Leenanon, B., and Drake, M. A. (2001). Acid stress, starvation, and cold stress affect poststress behavior of Escherichia coli O157:H7 and nonpathogenic Escherichia coli. J. Food Prot. 64, 970–974. doi: 10.4315/0362-028x-64.7.970
Leyer, G. J., and Johnson, E. A. (1993). Acid adaptation induces cross-protection against environmental stresses in Salmonella Typhimurium. Appl. Environ. Microbiol. 59, 1842–1847.
Li, H., Bhaskara, A., Megalis, C., and Tortorello, M. L. (2012). Transcriptomic analysis of Salmonella desiccation resistance. Foodborne Pathog. Dis. 9, 1143–1151. doi: 10.1089/fpd.2012.1254
Li, H., Handsaker, B., Wysoker, A., Fennell, T., Ruan, J., Homer, N., et al. (2009). The sequence alignment/map format and SAMtools. Bioinformatics 25, 2078–2079. doi: 10.1093/bioinformatics/btp352
Lianou, A., Stopforth, J. D., Yoon, Y., Wiedmann, M., and Sofos, J. N. (2006). Growth and stress resistance variation in culture broth among Listeria monocytogenes strains of various serotypes and origins. J. Food Prot. 69, 2640–2647. doi: 10.4315/0362-028x-69.11.2640
Liao, J., Wiedmann, M., and Kovac, J. (2017). Genetic stability and evolution of the sigB allele, used for Listeria sensu stricto subtyping and phylogenetic inference. Appl. Environ. Microbiol. 83, e00306–e00317. doi: 10.1128/AEM.00306-17
Liu, B., Eydallin, G., Maharjan, R. P., Feng, L., Wang, L., and Ferenci, T. (2017). Natural Escherichia coli isolates rapidly acquire genetic changes upon laboratory domestication. Microbiology 163, 22–30. doi: 10.1099/mic.0.000405
Lokkesmoe, K., and Olson, K. (1995). Process for inhibition of microbial growth in aqueous transport streams. US patent 5,409,713A. Saint Paul, MN: Ecolab Inc.
López-Velasco, G., Tomás-Callejas, A., Diribsa, D., Wei, P., and Suslow, T. V. (2013). Growth of Salmonella enterica in foliar pesticide solutions and its survival during field production and postharvest handling of fresh market tomato. J. Appl. Microbiol. 114, 1547–1558. doi: 10.1111/jam.12150
López-Velasco, G., Tomás-Callejas, A., Sbodio, A., Artés-Hernández, F., and Suslow, T. V. (2012). Chlorine dioxide dose, water quality and temperature affect the oxidative status of tomato processing water and its ability to inactivate Salmonella. Food Control 26, 28–35. doi: 10.1016/j.foodcont.2011.12.016
Lou, Y., and Yousef, A. E. (1997). Adaptation to sublethal environmental stresses protects Listeria monocytogenes against lethal preservation factors. Appl. Environ. Microbiol. 63, 1252–1255.
Madisch, I., and Heim, A. (2007). Extent of circulation of incorrectly labeled adenovirus 50 and 51 prototype preparations. J Clin Microbiol 45, 2092. doi: 10.1128/jcm.00251-07
Manning, S. D. (2011). A Reference Center to Facilitate the Study of Shiga Toxin-Producing Escherichia coli. Available at: http://www.shigatox.net/new/ (accessed July 26, 2018).
Maria-Rosario, A., Davidson, I., Debra, M., Verheul, A., Abee, T., and Booth, I. R. (1995). The role of peptide metabolism in the growth of Listeria monocytogenes ATCC 23074 at high osmolarity. Microbiology 141, 41–49. doi: 10.1099/00221287-141-1-41
Mattick, K. L., Jorgensen, F., Legan, J. D., Lappin-Scott, H. M., and Humphrey, T. J. (2000). Habituation of Salmonella spp. at reduced water activity and its effect on heat tolerance. Appl. Environ. Microbiol. 66, 4921–4925. doi: 10.1128/aem.66.11.4921-4925.2000
Mendum, M. L., and Smith, L. T. (2002). Characterization of glycine betaine porter I from Listeria monocytogenes and its roles in salt and chill tolerance. Appl. Environ. Microbiol. 68, 813–819. doi: 10.1128/aem.68.2.813-819.2002
Mercer, R., Nguyen, O., Ou, Q., Mcmullen, L., and Ganzle, M. G. (2017). Functional analysis of genes comprising the locus of heat resistance in Escherichia coli. Appl. Environ. Microbiol. 83, e01400–e014017. doi: 10.1128/AEM.01400-17
Møretrø, T., Schirmer, B. C. T., Heir, E., Fagerlund, A., Hjemli, P., and Langsrud, S. (2017). Tolerance to quaternary ammonium compound disinfectants may enhance growth of Listeria monocytogenes in the food industry. Int. J. Food Microbiol. 241, 215–224. doi: 10.1016/j.ijfoodmicro.2016.10.025
Naganandhini, S., Kennedy, Z. J., Uyttendaele, M., and Balachandar, D. (2015). Persistence of pathogenic and non-pathogenic Escherichia coli strains in various tropical agricultural soils of India. PLoS One 10:e0130038. doi: 10.1371/journal.pone.0130038
National Advisory Committee on Microbiological Criteria for Foods (2010). Parameters for determining inoculated pack/challenge study protocols. J. Food Prot. 73, 140–202. doi: 10.4315/0362-028x-73.1.140
Nightingale, K., Bovell, L., Grajczyk, A., and Wiedmann, M. (2007). Combined sigB allelic typing and multiplex PCR provide improved discriminatory power and reliability for Listeria monocytogenes molecular serotyping. J. Microbiol. Methods 68, 52–59. doi: 10.1016/j.mimet.2006.06.005
Oh, D. H., Pan, Y., Berry, E., Cooley, M., Mandrell, R., Breidt, F. Jr., et al. (2009). Escherichia coli O157:H7 strains isolated from environmental sources differ significantly in acetic acid resistance compared with human outbreak strains. J. Food Prot. 72, 503–509. doi: 10.4315/0362-028x-72.3.503
Omac, B., Moreira, R. G., Castillo, A., and Castell-Perez, E. (2015). Growth of Listeria monocytogenes and Listeria innocua on fresh baby spinach leaves: Effect of storage temperature and natural microflora. Postharvest. Biol. Technol. 100, 41–51. doi: 10.1016/j.postharvbio.2014.09.007
Parker, C. T., Kyle, J. L., Huynh, S., Carter, M. Q., Brandl, M. T., and Mandrell, R. E. (2012). Distinct transcriptional profiles and phenotypes exhibited by Escherichia coli O157:H7 isolates related to the 2006 spinach-associated outbreak. Appl. Environ. Microbiol. 78, 455–463. doi: 10.1128/AEM.06251-11
Peña-Meléndez, M., Perry, J. J., and Yousef, A. E. (2014). Changes in thermal resistance of three Salmonella serovars in response to osmotic shock and adaptation at water activities reduced by different humectants. J. Food Prot. 77, 914–918. doi: 10.4315/0362-028X.JFP-13-201
Piddock, L. J., Ricci, V., Mclaren, I., and Griggs, D. J. (1998). Role of mutation in the gyrA and parC genes of nalidixic-acid-resistant Salmonella serotypes isolated from animals in the United Kingdom. J. Antimicrob. Chemother. 41, 635–641. doi: 10.1093/jac/41.6.635
Poimenidou, S. V., Chatzithoma, D.-N., Nychas, G.-J., and Skandamis, P. N. (2016). Adaptive response of Listeria monocytogenes to heat, salinity and low pH, after habituation on cherry tomatoes and lettuce leaves. PLoS One 11:e0165746. doi: 10.1371/journal.pone.0165746
Premaratne, R. J., Lin, W. J., and Johnson, E. A. (1991). Development of an improved chemically defined minimal medium for Listeria monocytogenes. Appl. Environ. Microbiol. 57, 3046–3048.
Raengpradub, S., Wiedmann, M., and Boor, K. J. (2008). Comparative analysis of the sigma B-dependent stress responses in Listeria monocytogenes and Listeria innocua strains exposed to selected stress conditions. Appl. Environ. Microbiol. 74, 158–171. doi: 10.1128/aem.00951-07
Robinson, L. J., Cameron, A. D. S., and Stavrinides, J. (2015). Spontaneous and on point: do spontaneous mutations used for laboratory experiments cause pleiotropic effects that might confound bacterial infection and evolution assays? FEMS Microbiol. Lett. 362:fnv177. doi: 10.1093/femsle/fnv177
Rodgers, S. L., Cash, J. N., Siddiq, M., and Ryser, E. T. (2004). A comparison of different chemical sanitizers for inactivating Escherichia coli O157:H7 and Listeria monocytogenes in solution and on apples, lettuce, strawberries, and cantaloupe. J. Food Prot. 67, 721–731. doi: 10.4315/0362-028x-67.4.721
Sarkar, S. (2015). Microbiological considerations: pasteurized milk. Int. J. Dairy Sci. 10, 206–218. doi: 10.3923/ijds.2015.206.218
Scott, V. N., Swanson, K. M. J., Freier, T. A., Pruett, W., Sveum, W. H., Hall, P. A., et al. (2005). Guidelines for conducting Listeria monocytogenes challenge testing of foods. Food Prot. Trends 25, 818–825. doi: 10.1016/j.foodres.2015.06.004
Shuai, Z., Banting, G. S., Ruecker, N. J., and Neumann, N. F. (2017). Stress resistance in naturalised waste water E. coli strains. J. Environ. Eng. Sci. 12, 42–50. doi: 10.1680/jenes.16.00021
Snyder, E., Gordon, D. M., and Stoebel, D. M. (2012). Escherichia coli lacking RpoS are rare in natural populations of non-pathogens. G3 2, 1341–1344. doi: 10.1534/g3.112.003855
Srikantiah, P., Bodager, D., Toth, B., Kass-Hout, T., Hammond, R., Stenzel, S., et al. (2005). Web-based investigation of multistate salmonellosis outbreak. Emerg. Infect. Dis. 11, 610–612. doi: 10.3201/eid1104.040997
Stacey, G. N. (2000). Cell contamination leads to inaccurate data: we must take action now. Nature 403:356. doi: 10.1038/35000394
Stackhouse, R. R., Faith, N. G., Kaspar, C. W., Czuprynski, C. J., and Wong, A. C. (2012). Survival and virulence of Salmonella enterica serovar enteritidis filaments induced by reduced water activity. Appl. Environ. Microbiol. 78, 2213–2220. doi: 10.1128/AEM.06774-11
Stamatakis, A. (2015). Using RAxML to infer phylogenies. Curr. Protoc. Bioinform. 51, 6.14.11–16.14.14.
States, D. J., and Gish, W. (1994). Combined use of sequence similarity and codon bias for coding region identification. J. Comput. Biol. 1, 39–50. doi: 10.1089/cmb.1994.1.39
Subbarayan, P. R., and Sarkar, M. (2004). A comparative study of variation in codon 33 of the rpoS gene in Escherichia coli K12 stocks: implications for the synthesis of sigma(s). Mol. Genet. Genomics 270, 533–538. doi: 10.1007/s00438-003-0944-x
Sutton, A., Buencamino, R., and Eisenstark, A. (2000). rpoS mutants in archival cultures of Salmonella enterica serovar Typhimurium. J. Bact. 182, 4375–4379. doi: 10.1128/jb.182.16.4375-4379.2000
Suzuki, K., Babitzke, P., Kushner, S. R., and Romeo, T. (2006). Identification of a novel regulatory protein (CsrD) that targets the global regulatory RNAs CsrB and CsrC for degradation by RNase E. Genes Dev. 20, 2605–2617. doi: 10.1101/gad.1461606
Timme, R. E., Pettengill, J. B., Allard, M. W., Strain, E., Barrangou, R., Wehnes, C., et al. (2013). Phylogenetic diversity of the enteric pathogen Salmonella enterica subsp. enterica inferred from genome-wide reference-free SNP characters. Genome Biol. Evol. 5, 2109–2123. doi: 10.1093/gbe/evt159
Toboldt, A., Tietze, E., Helmuth, R., Junker, E., Fruth, A., and Malorny, B. (2013). Population structure of Salmonella enterica serovar 4,[5],12:b:- strains and likely sources of human infection. Appl. Environ. Microbiol. 79, 5121–5129. doi: 10.1128/aem.01735-13
Tomás-Callejas, A., López-Velasco, G., Camacho, A. B., Artés, F., Artés-Hernández, F., and Suslow, T. V. (2011). Survival and distribution of Escherichia coli on diverse fresh-cut baby leafy greens under preharvest through postharvest conditions. Int. J. Food Microbiol. 151, 216–222. doi: 10.1016/j.ijfoodmicro.2011.08.027
Vangay, P., Fugett, E. B., Sun, Q. I., and Wiedmann, M. (2013). Food microbe tracker: a web-based tool for storage and comparison of food-associated microbes. J. Food Prot. 76, 283–294. doi: 10.4315/0362-028X.JFP-12-276
Wang, R., Bono, J. L., Kalchayanand, N., Shackelford, S., and Harhay, D. M. (2012). Biofilm formation by shiga toxin-producing Escherichia coli O157:H7 and non-O157 strains and their tolerance to sanitizers commonly used in the food processing environment. J. Food Prot. 75, 1418–1428. doi: 10.4315/0362-028X.JFP-11-427
Weller, D., Wiedmann, M., and Strawn, L. K. (2015). Spatial and temporal factors associated with an increased prevalence of Listeria monocytogenes in spinach fields in New York State. Appl. Environ. Microbiol. 81, 6059–6069. doi: 10.1128/AEM.01286-15
Weller, D. L., Kovac, J., Kent, D. J., Roof, S., Tokman, J. I., Mudrak, E., et al. (2017a). Escherichia coli transfer from simulated wildlife feces to lettuce during foliar irrigation: a field study in the Northeastern United States. Food Microbiol. 68, 24–33. doi: 10.1016/j.fm.2017.06.009
Weller, D. L., Kovac, J., Roof, S., Kent, D. J., Tokman, J. I., Kowalcyk, B., et al. (2017b). Survival of Escherichia coli on lettuce under field conditions encountered in the Northeastern United States. J. Food Prot. 80, 1214–1221. doi: 10.4315/0362-028X.JFP-16-419
Werner, S., Boman, K., Einemo, I., Erntell, M., De Jong, B., Lindqvist, A., et al. (2007). Outbreak of Salmonella Stanley in Sweden associated with alfalfa sprouts, July-August 2007. Euro. Surveill. 12:E071018.071012.
Williams, D., Trimble, W. L., Shilts, M., Meyer, F., and Ochman, H. (2013). Rapid quantification of sequence repeats to resolve the size, structure and contents of bacterial genomes. BMC Genomics 14:537. doi: 10.1186/1471-2164-14-537
Winter, A. R., Stewart, G. F., Mcfarlane, V. H., and Solowey, M. (1946). Pasteurization of liquid egg products: III. Destruction of Salmonella in liquid whole egg. Am. J. Public Health Nations Health 36, 451–460. doi: 10.2105/ajph.36.5.451
Wisniewsky, M. A., Glatz, B. A., Gleason, M. L., and Reitmeier, C. A. (2000). Reduction of Escherichia coli O157:H7 counts on whole fresh apples by treatment with sanitizers. J. Food Prot. 63, 703–708. doi: 10.4315/0362-028x-63.6.703
Wood, D. E., and Salzberg, S. L. (2014). Kraken: ultrafast metagenomic sequence classification using exact alignments. Genome Biol. 15:R46. doi: 10.1186/gb-2014-15-3-r46
Wright, D., Feibert, E., Reitz, S., Shock, C., and Waite-Cusic, J. (2018). Field evidence supporting conventional onion curing practices as a strategy to mitigate Escherichia coli contamination from irrigation water. J. Food Prot. 81, 369–376. doi: 10.4315/0362-028X.JFP-17-231
Yang, H., Li, Y., and Johnson, M. G. (2001). Survival and death of Salmonella Typhimurium and Campylobacter jejuni in processing water and on chicken skin during poultry scalding and chilling. J. Food Prot. 64, 770–776. doi: 10.4315/0362-028x-64.6.770
Zhang, S., Yin, Y., Jones, M. B., Zhang, Z., Deatherage Kaiser, B. L., Dinsmore, B. A., et al. (2015). Salmonella serotype determination utilizing high-throughput genome sequencing data. J. Clin. Microbiol. 53, 1685–1692. doi: 10.1128/JCM.00323-15
Keywords: whole genome sequencing, produce safety, Listeria, Salmonella, E. coli, peroxyacetic acid
Citation: Harrand AS, Kovac J, Carroll LM, Guariglia-Oropeza V, Kent DJ and Wiedmann M (2019) Assembly and Characterization of a Pathogen Strain Collection for Produce Safety Applications: Pre-growth Conditions Have a Larger Effect on Peroxyacetic Acid Tolerance Than Strain Diversity. Front. Microbiol. 10:1223. doi: 10.3389/fmicb.2019.01223
Received: 02 March 2019; Accepted: 16 May 2019;
Published: 31 May 2019.
Edited by:
Paula Teixeira, Catholic University of Portugal, PortugalReviewed by:
Alexandre Leclercq, Institut Pasteur, FranceCopyright © 2019 Harrand, Kovac, Carroll, Guariglia-Oropeza, Kent and Wiedmann. This is an open-access article distributed under the terms of the Creative Commons Attribution License (CC BY). The use, distribution or reproduction in other forums is permitted, provided the original author(s) and the copyright owner(s) are credited and that the original publication in this journal is cited, in accordance with accepted academic practice. No use, distribution or reproduction is permitted which does not comply with these terms.
*Correspondence: Martin Wiedmann, bXcxNkBjb3JuZWxsLmVkdQ==
Disclaimer: All claims expressed in this article are solely those of the authors and do not necessarily represent those of their affiliated organizations, or those of the publisher, the editors and the reviewers. Any product that may be evaluated in this article or claim that may be made by its manufacturer is not guaranteed or endorsed by the publisher.
Research integrity at Frontiers
Learn more about the work of our research integrity team to safeguard the quality of each article we publish.