- 1Guangdong Provincial Engineering Research Center for Urban Water Recycling and Environmental Safety, Graduate School at Shenzhen, Tsinghua University, Shenzhen, China
- 2School of Environment and Energy, Shenzhen Graduate School, Peking University, Shenzhen, China
Ultramicrobacteria (UMB) are omnipresent and numerically dominate in freshwater, as microbes can present in drinking water systems, however, the UMB communities that occur and their removal behaviors remain poorly characterized in drinking water treatment plants (DWTPs). To gain insights into these issues, we profiled bacterial cell density, community structure and functions of UMB and their counterpart large bacteria (LB) using flow cytometry and filtration paired with 16S rRNA gene high-throughput sequencing in a full-scale DWTP. Contrary to the reduction of bacterial density and diversity, the proportion of UMB in the total bacteria community increased as the drinking water treatment process progressed, and biological activated carbon facilitated bacterial growth. Moreover, UMB were less diverse than LB, and their community structure and predicted functions were significantly different. In the DWTP, UMB indicator taxa were mainly affiliated with α/β/γ-Proteobacteria, Deinococcus–Thermus, Firmicutes, Acidobacteria, and Dependentiae. In particular, the exclusive clustering of UMB at the phylum level, e.g., Parcubacteria, Elusimicrobia, and Saccharibacteria, confirmed the fact that the ultra-small size of UMB is a naturally and evolutionarily conserved trait. Additionally, the streamlined genome could be connected to UMB, such as candidate phyla radiation (CPR) bacteria, following a symbiotic or parasitic lifestyle, which then leads to the observed high connectedness, i.e., non-random intra-taxa co-occurrence patterns within UMB. Functional prediction analysis revealed that environmental information processing and DNA replication and repair likely contribute to the higher resistance of UMB to drinking water treatment processes in comparison to LB. Overall, the study provides valuable insights into the occurrence and fate of UMB regarding community structure, phylogenetic characteristics and potential functions in a full-scale DWTP, and it is a useful reference for beneficial manipulation of the drinking water microbiome.
Introduction
Bacteria with an ultra-small cell size are omnipresent and numerically dominate in some natural aquatic ecosystems, including oceans, rivers, lakes, and groundwater (Cavicchioli and Ostrowski, 2003; Duda et al., 2012; Luef et al., 2015; Neuenschwander et al., 2015; Proctor et al., 2018). These small bacteria are known by a variety of terms such as “ultramicrobacteria (UMB),” “ultra-small bacteria,” “ultramicrocells,” “nanobacteria” or “low nucleic acid content (LNA) bacteria” (Cavicchioli and Ostrowski, 2003; Duda et al., 2012; Proctor et al., 2018). The UMB have small genomes (0.58–3.2 Mb) and an essentially constant cell volume of less than 0.1 μm3, independent of culture conditions, such as growth stages and nutrient concentrations (Cavicchioli and Ostrowski, 2003; Duda et al., 2012). UMB are found within phylogenetically distinct groups, including the Actinobacteria, Proteobacteria, Spirochaetes and the Cytophaga- Flavobacterium- Bacteroides branch (Hahn, 2004; Hahn et al., 2004; Miteva and Brenchley, 2005; Duda et al., 2012; Proctor et al., 2018).
The tiny cell size of UMB provides a larger surface-to-volume ratio, allowing more efficient uptake of nutrients from oligotrophic environments (Giovannoni et al., 2005; Duda et al., 2012) and protection against grazing pressure (Miteva and Brenchley, 2005; Williams et al., 2009). Furthermore, the streamlined genome of UMB optimizes and simplifies bacterial metabolism (Giovannoni et al., 2005; Williams et al., 2009). This minimizes maintenance requirements (e.g., phosphorus and nitrogen) for DNA synthesis and cell growth, permits efficient nutrient utilization and facilitates growth in nutrient-depleted conditions (Giovannoni et al., 2005, 2014; Williams et al., 2009). In addition, UMB can simultaneously assimilate a mixture of substrates, allowing relatively faster growth under carbon/energy-limited conditions (Egli, 2010). These versatile metabolic properties contribute to the adaptive growth of UMB at low concentrations of nutrients and explains their wide distribution from aquatic biotopes to terrestrial ecosystems (Cavicchioli and Ostrowski, 2003; Moran et al., 2015), even extreme habitats such as permafrost soils, ice sheet and thermal swamp moss (Loveland-Curtze et al., 2010; Suzina et al., 2015).
Biodiversity, population dynamics and functional activities of bacterial communities in drinking water treatment plants (DWTPs) are well documented (Ramseier et al., 2011; Besmer and Hammes, 2016; Zhang et al., 2017; Oh et al., 2018). The filtration process of DWTPs, including rapid sand filtration and biological granular activated carbon filtration (BAC) was designed to remove suspended particles, bacteria and dissolved organic matter through microbial degradation (Boon et al., 2011; Velten et al., 2011; Pinto et al., 2012; Basu et al., 2016). As a hygienic barrier, a disinfection step, e.g., ozonation, chlorination, chloramination, or ultraviolet (UV) treatment, was used to inactivate primary and opportunistic pathogenic bacteria to guarantee microbial quality in drinking water distribution systems (Gomez-Alvarez et al., 2012; Chiao et al., 2014; Inkinen et al., 2018). To date, it has been recognized that UMB are not simply dormant members of the microbial population, but play important functions in biogeochemical cycling of organic and inorganic matters (Cavicchioli and Ostrowski, 2003; Williams et al., 2009; Duda et al., 2012). As the heterotrophic and autotrophic bacteria are able to eliminate contaminants in DWTPs (Oh et al., 2018), UMB would stimulate the formation of a biofilm with a high metabolic biodiversity and thus can remediate polluted aquifers (Ross et al., 2001). It was also reported that UMB were capable of anaerobic dechlorination of polychlorinated biphenyls (May et al., 2008) and mineralizing herbicide at low concentration (Gözdereliler et al., 2013). However, bacterial growth in drinking water can lead to water quality decline and health risks, e.g., pathogen occurrence (Hou et al., 2018; Oh et al., 2018). Previous studies have shown that UMB possess extreme resistance mechanisms to cross-protect against various environmental stresses, such as high-intensity heat shocks, UV, viral infection, antibiotics and oxidative stress, e.g., treatments in H2O2 (Eguchi et al., 1996; Cavicchioli and Ostrowski, 2003). Ramseier et al. (2011) found that some UMB cell membranes exhibited lower damage reactivity than large bacteria (LB) during disinfection treatments using chlorine dioxide and permanganate. Furthermore, UMB can escape the 0.2-μm microfiltration trapping. Wang et al. (2008b) found that more than 10% of indigenous bacteria could pass through 0.22-μm pore size industrial-scale cartridge filtration units in groundwater aquifers. Recent studies revealed that UMB, termed LNA bacteria in flow cytometry (FCM), are more abundant than LB in drinking water (Liu et al., 2016, 2017b). Moreover, UMB may use a dormancy strategy for survival even under unfavorable growth conditions (Roesel and Grossart, 2012). It is therefore crucial to comprehensively investigate UMB community structure and their removal behaviors in DWTPs, and this could contribute to the beneficial manipulation of the drinking water microbiome.
In the current study, we aimed to test the hypothesis that UMB were more recalcitrant to removal than their counterpart LB in DWTPs. The specific objectives of the study were to: (1) profile the changes of bacterial cell density and community structure of UMB and LB in DWTPs via FCM and filtration paired with 16S rRNA gene sequencing; (2) identify indicator taxa, phylogenetic difference and fate of UMB and LB in DWTPs; and (3) predict and compare potential functional and metabolic capabilities of UMB and LB.
Materials and Methods
Water Sampling
Water samples were taken from a DWTP located in Guangdong province, China. The treatment processes of this DWTP comprised pre-ozonation, flocculation-sedimentation, rapid sand filtration, ozonation, biological granular activated carbon filtration and chlorination with a daily treatment capacity of 520,000 m3. The raw water (RW) and effluent of each process unit, i.e., flocculation-sedimentation effluent (FS), rapid sand filtration effluent (RSF), biological granular activated carbon filtration effluent (BAC), and chlorination water (CW), were sampled in 2017 on April 21 (DWTP1), May 6 (DWTP2), and May 24 (DWTP3), respectively. Volumes per sampling units ranged from 10 to 50 L depending on the pre-measured cell density of bacteria in the respective drinking water treatment units. Each filtration step was performed using 5–10 pieces of 47-mm filter, and filters in each step were pooled and then used to extract genomic DNA. It should be noted that the BAC tanks were in a state of maintenance on May 6. Therefore, we cannot collect the BAC effluent sample at that time. Residual chlorine was quenched using Na2S2O3 immediately during sampling. Water samples were kept in a 4°C ice box and then transported to the laboratory and processed for FCM within 3 h.
Separation of Large Bacteria and Ultramicrobacteria
A two-step filtration was used to obtain LB and UMB (Luef et al., 2015). The original water sample was initially filtered through 0.22-μm polyvinylidene fluoride membranes (47 mm, Millipore Cork, Ireland) using sterilized filtration units (Nalgene, Thermo Fisher Scientific, United States) mounted on sterilized glass bottles. Bacteria in the 0.22-μm filtrates were subsequently refiltered through 0.10-μm polycarbonate membranes (47 mm, Millipore). Bacterial cell density of the original water samples and 0.22/0.10-μm filtrates was measured immediately via FCM. Membranes collected from the 0.22-μm (LB, captured on 0.22-μm filter) and 0.10-μm (UMB, passing 0.22-μm filter and captured on 0.10-μm filter) filtration steps were stored at -80°C for later DNA extraction.
Bacterial cell density (cells/mL) was measured with FCM in the original water samples (CDoriginal) and 0.22-μm filtrates (CDUMB), where LB cell density (CDLB) was calculated as follows (Eq. 1):
The UMB proportion (UMB%) was expressed as follows (Eq. 2).
Flow Cytometry
Flow cytometric measurements were carried out as described previously (Liu et al., 2017a). One milliliter of water sample was stained with 10 μL/mL SYBR Green I (1:100 dilutions in dimethyl sulfoxide as the working solution; Invitrogen, United States), and incubated in the dark for 15 min at room temperature. FCM then was performed at a flow rate of 60 μL/min using a BD FACSCalibur system (BD Biosciences, San Jose, CA, United States) equipped with a 15-mW air-cooled argon laser, emitting at a fixed wavelength of 488 nm. All data were analyzed with the BD CellQuest Pro software (BD Biosciences). Green fluorescence was collected in the FL1 channel (520 ± 20 nm) and bacterial cell density was counted through the gating on a two-parameter dot-plot of green fluorescence against side scatter. Milli-Q cell-free water was used as the blank samples and sheath fluid as well in FCM. Positive signals of bacterial cell was separated from instrument noise or sample background by electronic gating with BD CellQuest Pro software (BD Biosciences). All samples were collected as logarithmic signals and were triggered on the green fluorescence channel. The coefficient of variation on FCM measurement for replicates should be below 2%, and all samples were measured in triplicate.
16S rRNA Gene Sequencing With Illumina HiSeq 2500 Platform
Genomic DNA was extracted from LB and UMB, respectively, retained on sterile 0.22-μm and 0.10-μm membranes using a FastDNA SPIN Kit for Soil (MP Biomedicals, United States) and following the manual protocol. Additionally, three sterilized filter were used as the blank samples, i.e., the negative control in DNA extraction and PCR amplification. The 16S rRNA V4 region sequences (∼265 nucleotides) were amplified using primers F515 (5′-GTG CCA GCM GCC GCG GTA A-3′) and R806 (5′-GGA CTA CHV GGG TWT CTA AT-3′) (Ma et al., 2017) and PrimeSTAR HS DNA Polymerase (Takara Biomedical Technology, Kusatsu, Japan). The amplification program was as follows: 95°C for 5 min followed by 30 cycles of 95°C for 30 s, 60°C for 30 s and 72°C for 1 min, and a final extension at 72°C for 10 min. PCR assays were conducted in triplicate to minimize variation during amplification, then the PCR triplicates were pooled and sequenced as one sample. PCR products were purified with TaKara miniBEST DNA Fragment Purification Kit (Takara Biomedical Technology) and the concentration was measured by NanoDrop One (Thermo Scientific, United States). The PCR product concentration of the blank samples was below the detection limit. All purified PCR amplicons were mixed together to achieve equal mass concentration and were sequenced on an Illumina HiSeq 2500 PE250 (Novogene, Tianjin, China). Sequences were analyzed using Mothur software v. 1.39.5 as described in Kozich et al. (2013). Chimeras were checked with Chimera.uchime and sequencing depth was finally normalized to 17,000 sequences per sample. The 16S rRNA gene sequences were clustered into operational taxonomic units (OTUs) based on a similarity threshold of 97%. Singleton OTUs were removed and excluded for the following analysis. Taxonomic annotation of representative OTU sequences was conducted using BLASTn search against the SILVA v132 reference database (Quast et al., 2013).
Microbial Community and Co-occurrence Network Analysis
Rarified OTU tables were used to generate α- and β- diversity metrics. Species richness was the number of unique OTUs in the sample, and Shannon diversity index was calculated as the community α-diversity by R software using vegan package (Oksanen et al., 2018). Non-metric multidimensional scaling (NMDS) analysis was conducted to visualize community similarities using the Bray–Curtis dissimilarity as β-diversity. Adonis analysis was used to quantify the relative contribution of each factor (i.e., membrane pore size, treatment process units and each individual sampling) to the variation of community structure by vegan package. The linear discriminant analysis effect size (LEfSe) method was employed to discover specific indicator taxa for LB and UMB [threshold: logarithmic linear discriminant analysis (LDA) scores > 2 and Kruskal–Wallis test P-value < 0.05] (Segata et al., 2011). Those OTUs unassigned to indicator taxa were categorized into the “normal” taxa. To further visualize the differentiation of LB and UMB indicator taxa on the community composition, a taxonomic alike-tree was constructed at different classification levels using igraph package (Csardi and Nepusz, 2006). A phylogenetic tree was constructed using representative sequences of indicator taxa OTUs via the maximum likelihood method in MEGA 7, and then displayed via iTOL (Letunic and Bork, 2016).
A co-occurrence network analysis was performed for indicator taxa OTUs of LB and UMB associated with normal taxa OTUs according to the previous study (Ju and Zhang, 2015). Topological properties, including average weighted degree, network diameter, average path length, clustering coefficient and modularity, were calculated to describe the complex pattern of interrelationships among microbial taxa. The observed incidence (O%) and random incidence (R%) of co-occurrence patterns between taxa were statistically checked, and the degree of a lack of agreement between O% and R% (O/R ratio) was used as a benchmark of the non-randomness of observed co-occurrence patterns in bacterial communities.
Microbial Functional and Metabolic Prediction
The potential functional and metabolic capacities of OTUs were predicted based on the SILVA123 and Kyoto Encyclopedia for Genes and Genomes (KEGG) database using the Tax4fun package (Asshauer et al., 2015). The taxonomic profile of KEGG was obtained by the SILVA-based OTUs normalized by the 16S rRNA gene copy number. The Procrustes analysis was used to compare the difference of functional and metabolic capacities between LB and UMB (Forsberg et al., 2014). Student’s t-tests were performed using the “ggpubr” package in R, and the P-values were adjusted with the “BH” (or “fdr”) method of Benjamini–Hochberg to control the false discovery rate. Significantly differential enzyme-encoding genes were extracted and four categories of stress-resistant related functions in UMB were further classified based on the KEGG database (Supplementary Table S1).
Nucleotide Sequence Accession Numbers
Partial 16S rRNA gene sequences were deposited in the National Centre for Biotechnology Information (NCBI) Sequence Read Archive (SRA) under the accession number PRJNA481143.
Results
Changes of Cell Density and α-Diversity Along the Treatment Processes
Both LB and UMB cell densities were significantly reduced along the treatment process in the DWTP [P < 0.01, by analysis of variance (ANOVA)], except after the BAC unit (LB: 0.94–5.38 × 104 cells/mL; UMB: 1.22–9.68 × 102 cells/mL). However, the UMB proportion (UMB%) significantly increased along treatment units of the DWTP from 0.05–9.05% to 1.52–37.4% (P < 0.05, ANOVA, Figure 1A). The rarefaction curve of the assigned sequences per sample and Good’s Coverage ranging from 0.88 to 0.96 demonstrated that the sequencing depth was sufficient for each sample (Supplementary Figure S1). After removal of singleton OTUs, a total of 3,033 OTUs were obtained, including 2,826 LB OTUs and 2,523 UMB OTUs, with 2,316 OTUs shared by both LB and UMB (Supplementary Figure S2A). Overall, UMB community, as well as core UMB microbiome shared throughout all treatment units, were less diverse than LB community and core LB microbiome, respectively, among treatment units with exception of RSF (Figure 1B and Supplementary Figure S2). Meanwhile, variation in Shannon diversity index and richness of UMB was also less than that of LB among treatment units (P > 0.05, ANOVA). The LB α-diversity including Shannon index and richness decreased along the treatment units in DWTPs (P < 0.05, ANOVA), with the exception of the BAC. The BAC samples showed a complete reversal of the previous reduction in Shannon and richness index, indicating the highest diversities in the entire drinking water treatment units.
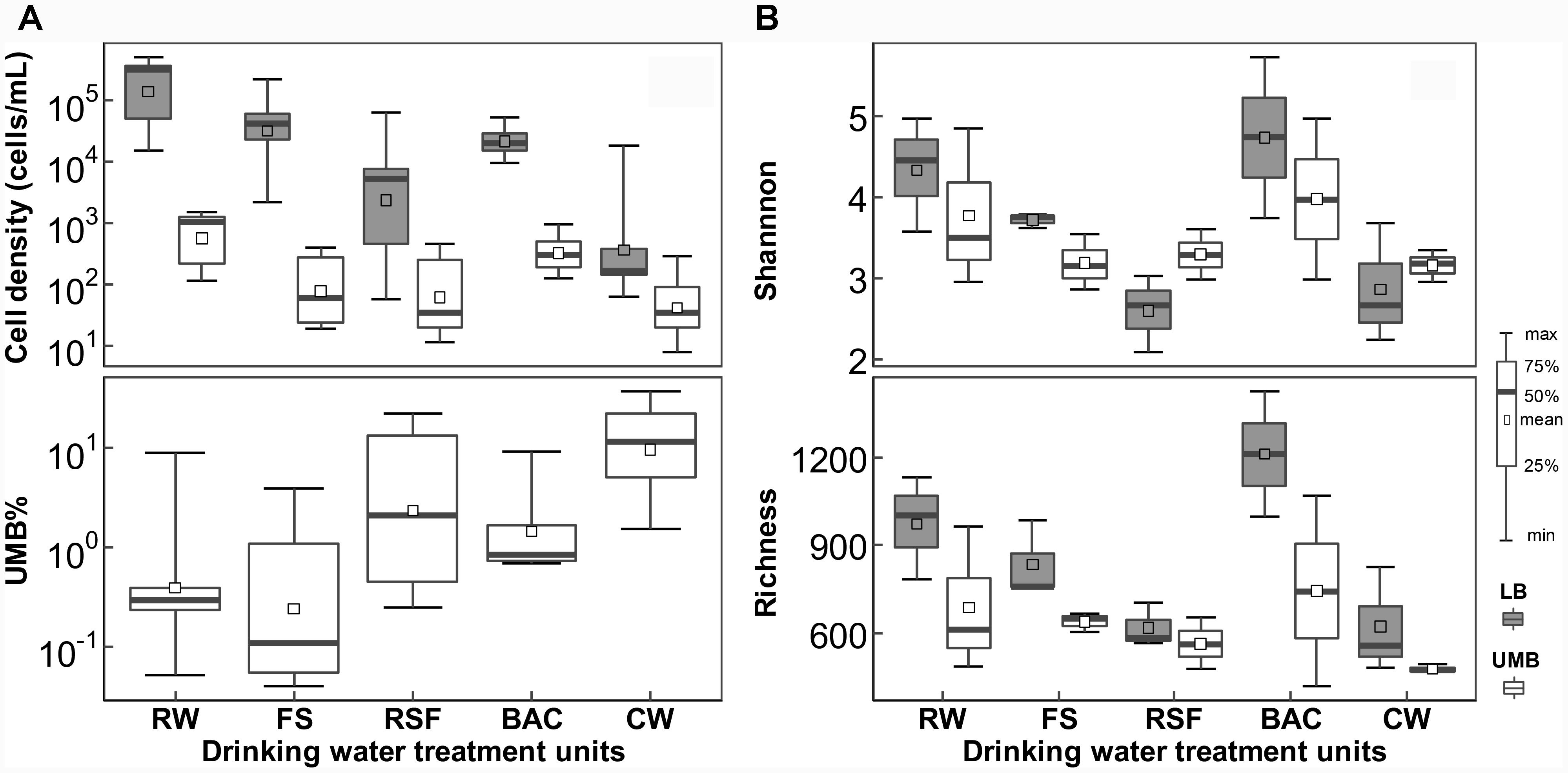
FIGURE 1. Changes in (A) cell density and (B) α-diversity for LB and UMB among treatment units of a DWTP. RW, raw water; FS, flocculation-sedimentation effluent; RSF, rapid sand filtration effluent; BAC, biological granular activated carbon filtration effluent; CW, chlorination water.
Variance of β-Diversity and Community Composition
The NMDS analysis showed that LB and UMB are clearly separated for each sample and individual sampling, while treatment process-induced changes are clear, but relatively minor in comparison (Figure 2A). The effect of 0.22-μm size-separation, individual DWTP sampling and their interactions could explain 34.6% of all community variations (10.9%, 13.1%, and 10.6%, respectively, Adonis, P < 0.001). Meanwhile, the treatment processes considerably affected bacterial communities, accounting for 23.4% of all community variations (Adonis, P < 0.001). For the community composition, the Proteobacteria dominated both LB (67.6–89.4%) and UMB (77.0–85.2%) in the DWTP, followed by Planctomycetes (3.6–11.5%) and Bacteroidetes (2.3–8.9%) for LB, and Deinococcus–Thermus (0.8–8.3%) and Firmicutes (1.5–5.4%) for UMB (Figure 2B). With respect to changes of community compositions of both LB and UMB in DWTP, compared with the RW, the Actinobacteria, Bacteroidetes, and Planctomycetes in both LB and UMB decreased along treatment units, with the exception that LB Bacteroidetes in CW was higher than that of RW (Figure 2B). In contrast, the relative abundances of Cyanobacteria, Deinococcus–Thermus, and Firmicutes in both LB and UMB increased along the treatment units. The phylum Proteobacteria increased from RW to CW in LB, and slightly increased in UMB, while there was a reduction from BAC to CW in UMB, and a reduction from RSF to BAC in LB (Figure 2B).
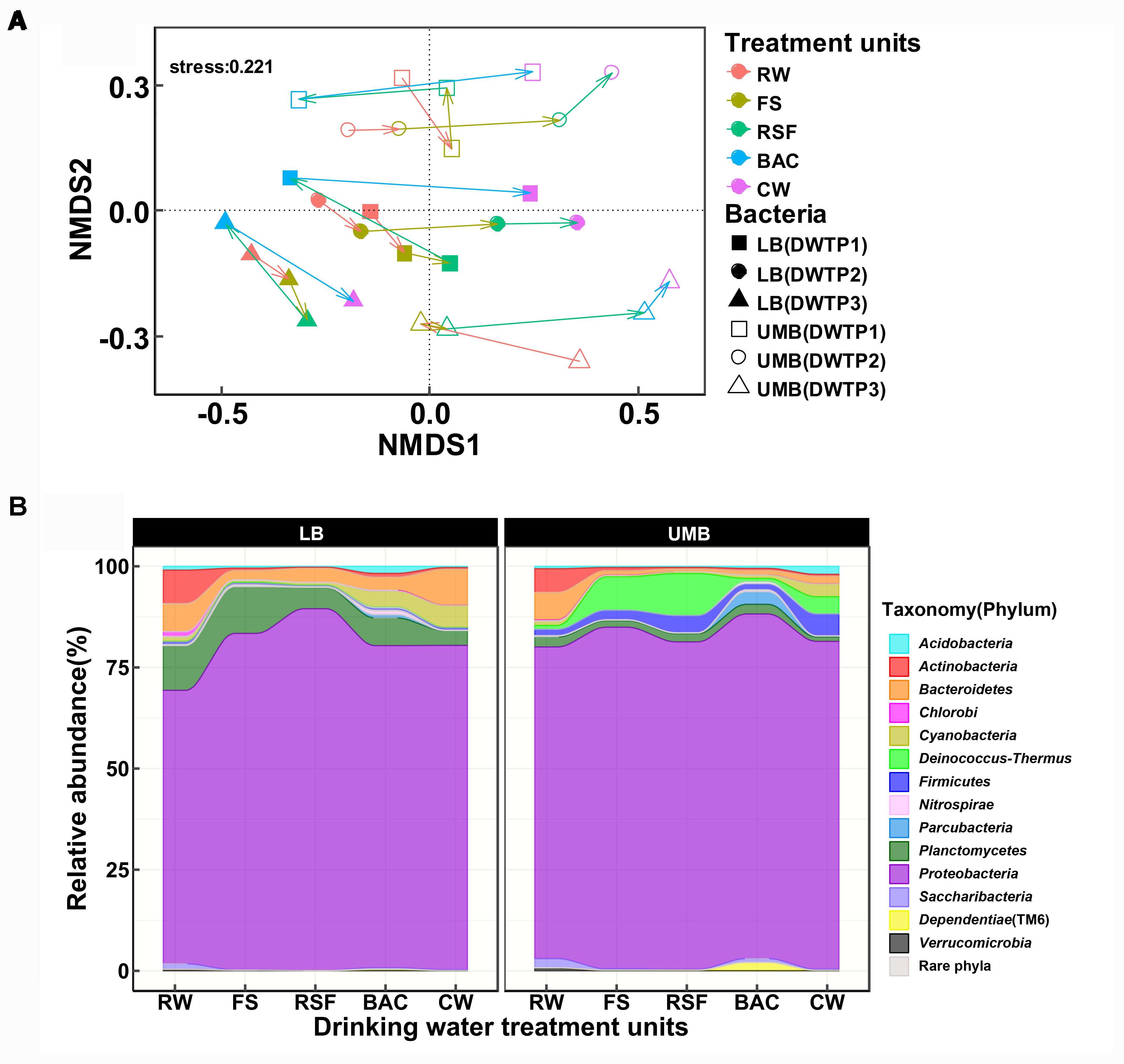
FIGURE 2. (A) Non-metric multidimensional scaling analysis (Bray–Curtis dissimilarity) for community structure of LB and UMB at the OTU level (97% similarity). (B) Variance of bacterial community composition of LB and UMB at the phylum level.
Occurrence of LB and UMB Indicator Taxa
The LEfSe algorithm can discover and explain differences in genomic features, including organisms, clades, OTUs, genes, or functions between two or more biological conditions in high-dimensional metagenomic data (Segata et al., 2011). Based on the differential OTUs abundance feature, 227 and 72 OTUs were, respectively, identified as the LB and UMB indicator taxa via the LEfSe algorithm (Supplementary Figure S3). LB indicator taxa accounted for 42.0% (ARA: the average of relative abundance of indicator taxa in all sample) of all LB communities (all 0.22-μm-filter-captured microbes) and UMB indicator taxa accounted for 37.4% of all UMB communities (all 0.1-μm-filter-captured microbes). These LB and UMB indicator taxa were phylogenetically located within 21 phyla (Figure 3). Both LB (ARA: 34.2%) and UMB (ARA: 27.9%) indicator taxa were mainly affiliated with phylum Proteobacteria, and several exclusive phyla were classified as UMB, i.e., Parcubacteria (OD1, ARA 0.25%), Elusimicrobia (ARA 0.10%) and Saccharibacteria (TM7, ARA 0.02%). The UMB indicator taxa also belonged to Deinococcus–Thermus, Firmicutes, Acidobacteria, and Dependentiae (TM6).
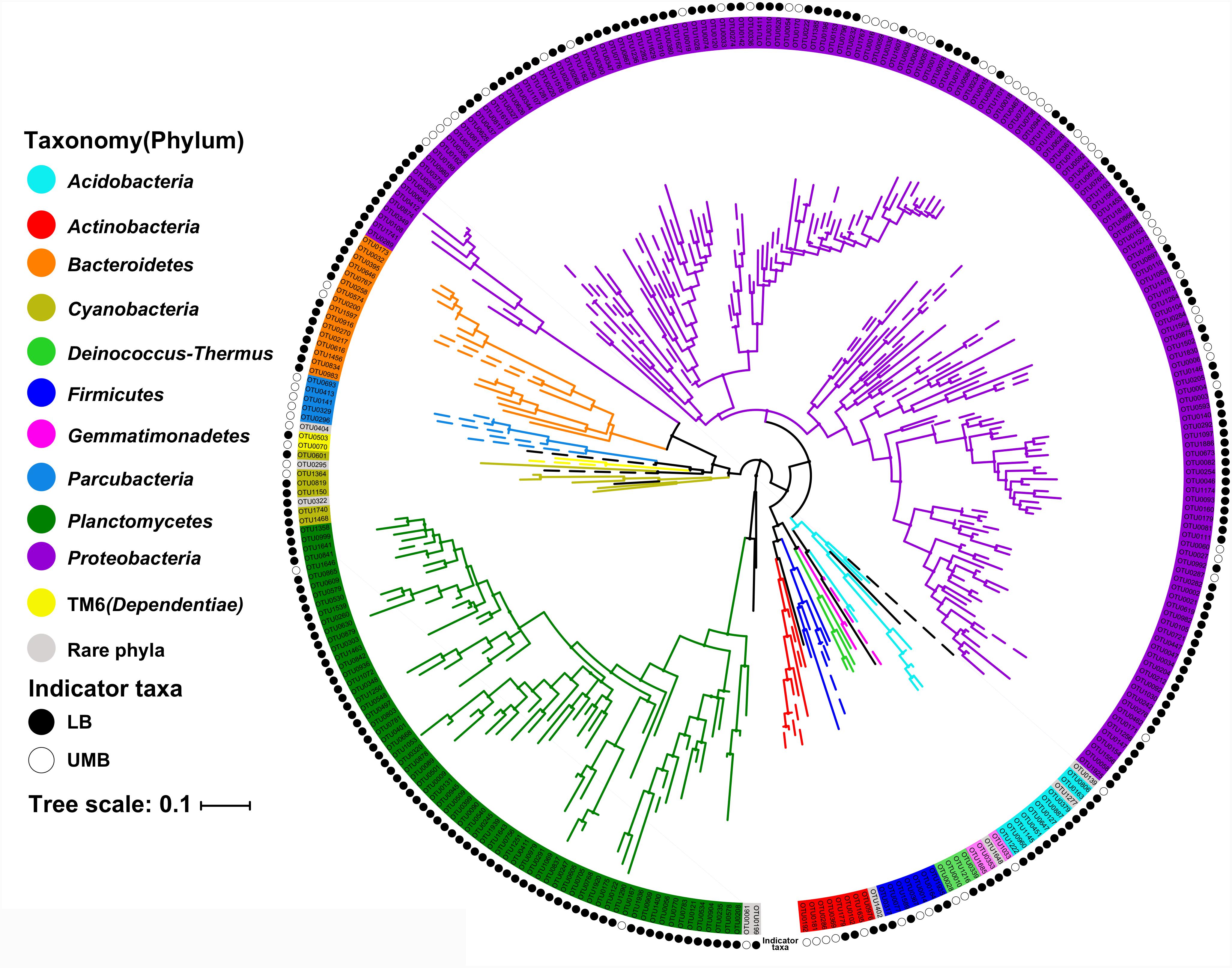
FIGURE 3. Maximum likelihood phylogenetic tree of LB and UMB indicator taxa. The branch color represents the taxonomy at the phylum level. Solid and open circles represent the LB and UMB indicator taxa, respectively. Bar, 10% sequence divergence.
Despite there being no exclusivity observed in Proteobacteria phylum clusters, when taxa were considered on the genus level some exclusive genera were detected in UMB indicator taxa, such as Kinneretia (ARA 2.4%), Ralstonia (ARA 2.1%), Dyella (ARA 2.1%), Ramlibacter (ARA 1.8%), Stenotrophomonas (ARA 1.1%), Burkholderia paraburkholderia (ARA 1.1%), Pandoraea (ARA 1.0%), Roseomonas (ARA 0.8%), and Vogesella (ARA 0.5%) as well as Anoxybacillus (ARA 2.5%) belonging to the phylum Firmicutes (Figure 4). These genera mainly aligned to the family Acetobacteraceae in the α-Proteobacteria, the families Neisseriaceae, Burkholderiaceae, and Comamonadaceae in the β-Proteobacteria, and the family Xanthomonadaceae in the γ-Proteobacteria. With a few exceptions, LB and UMB indicator taxa overlapped within several branches, e.g., Sphingomonas in the α-Proteobacteria class and Planctomyces in the phylum Planctomycetes.
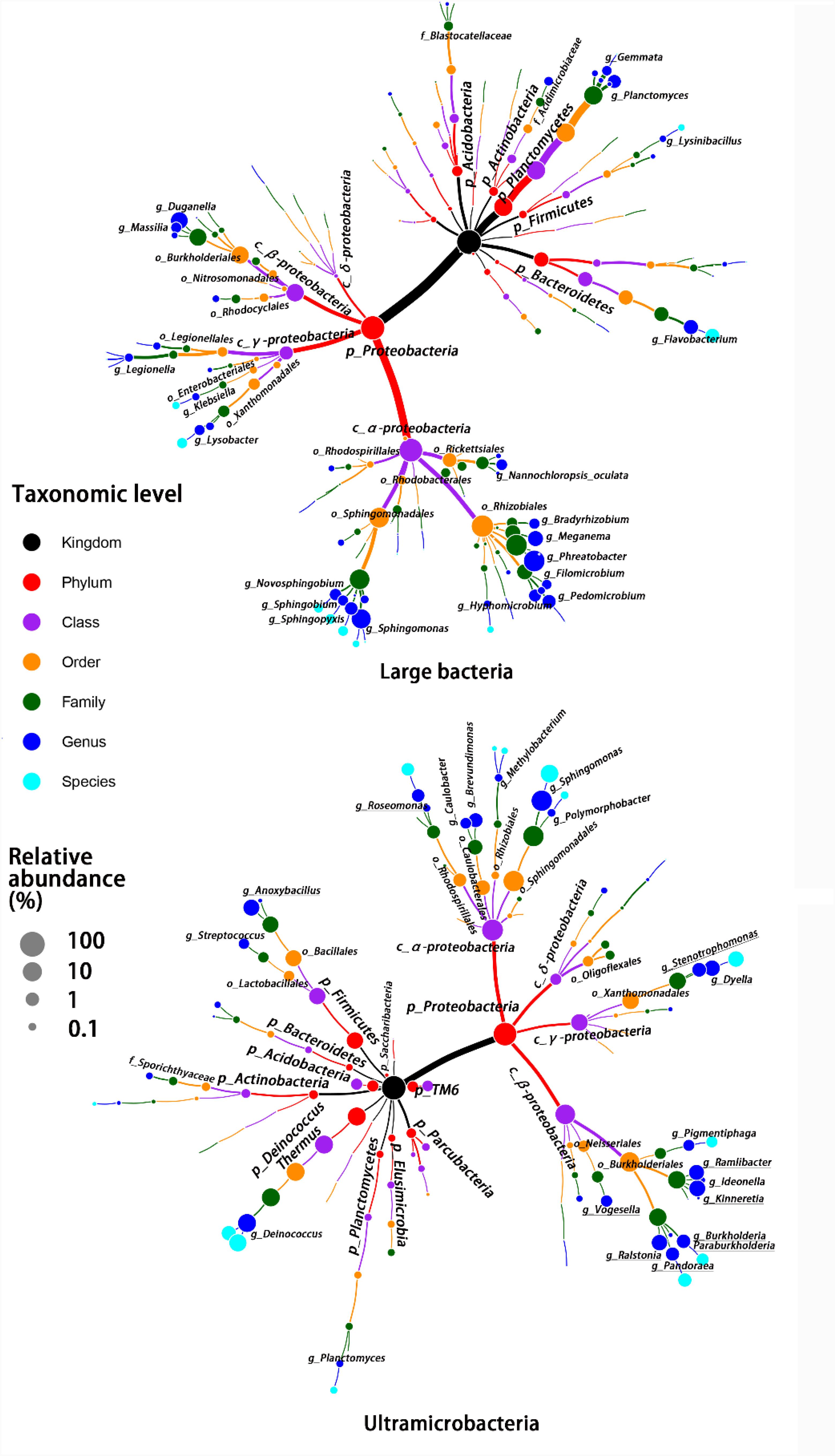
FIGURE 4. Taxonomic tree for LB and UMB indicator taxa composition at different taxon levels. Color and size of circles, respectively, represent the taxon level and average relative abundance of indicator taxa.
Co-occurrence patterns of LB/UMB indicator taxa and normal OTUs were explored using network inference based on strong (ρ > 0.8 and ρ < -0.8) and significant (P < 0.01) correlations (Figure 5). The co-occurrence patterns consisted of 109 nodes and 161 edges. The average weighted degree or node connectivity was 2.51. The average network distance between all pairs of nodes, i.e., average path length, was 4.51 edges with a network diameter of 12 edges. The clustering coefficient (that is, how nodes are embedded in their neighborhood and, thus, the degree to which they tend to cluster together) was 0.48 and the modularity index was 0.81. Structural and statistical analyses demonstrated that non-random co-occurrence patterns were evident within the intra indicators and the intra phylum (Figure 5). For the OTUs from the intra indicator taxa, LB–LB (55 OTUs), UMB–UMB (43 OTUs), and normal–normal (33 OTUs) tended to co-occur much more (81.4%) than expected under random association (33.9%), despite their different degrees of intra-type co-occurrence (as reflected by ‘O/R ratio,’ O/RLB-LB: 2.0, O/RUMB-UMB: 4.2, O/Rnormal-normal: 1.9). Among the phyla, Proteobacteria had the highest non-random (O% = 36.4%) and random (R% = 37.9%) co-occurrence incidences (O/R = 0.96). Actinobacteria, Bacteroidetes, and Planctomycetes showed higher possibilities of non-random co-occurrence than of random positive association (O/R = 11.0, 5.1, and 2.8, respectively). Moreover, higher non-random co-occurrence incidences were also observed for the OTUs from inter phyla (Figure 5). For example, higher incidences of co-occurrence than expected by chance were observed between OTUs from Proteobacteria and other phyla, including Acidobacteria, Deinococcus–Thermus, Elusimicrobia, Parcubacteria, and TM6 (Dependentiae) (O% > 1.0%, O/R > 1.5).
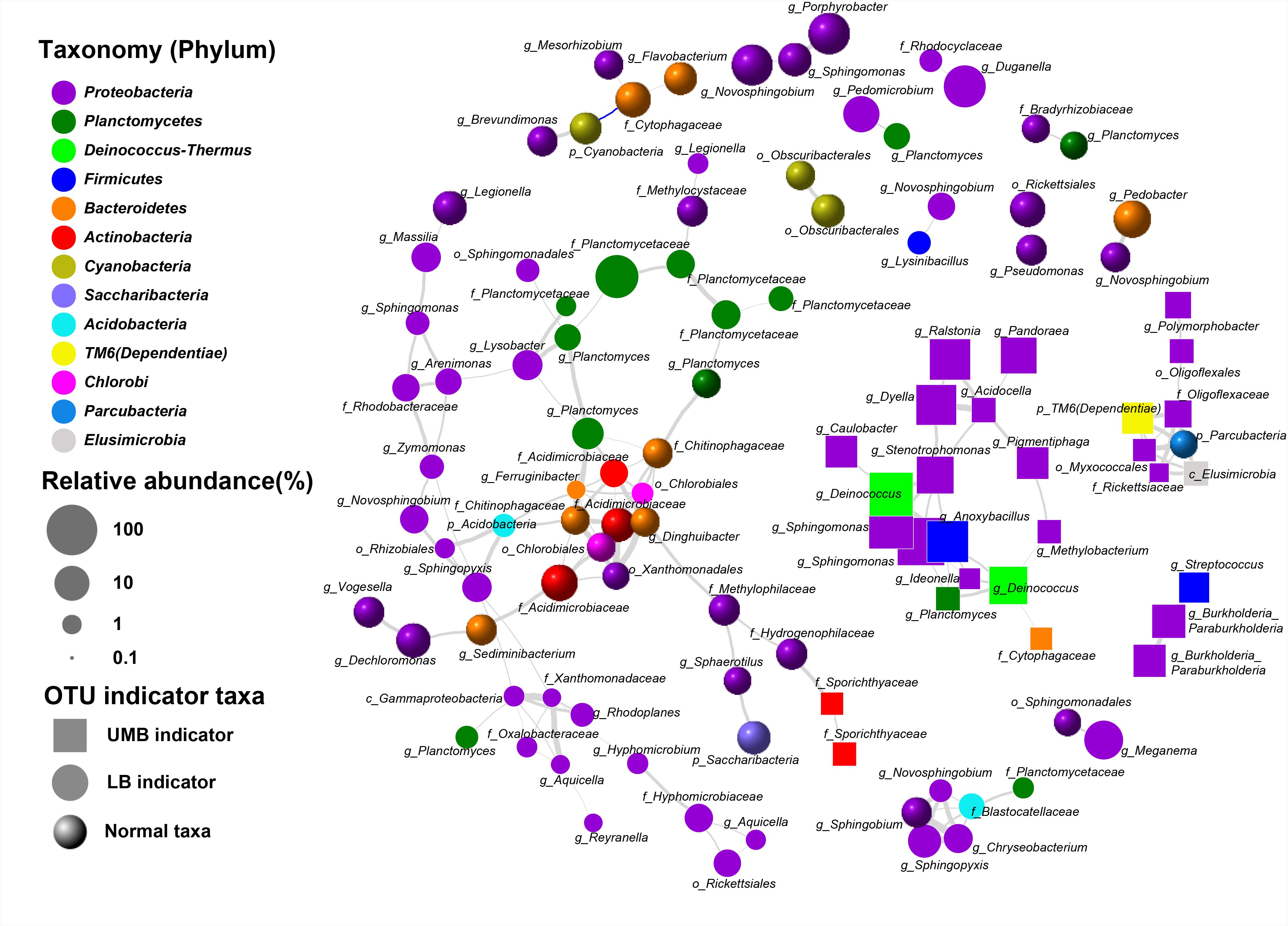
FIGURE 5. Co-occurrence of LB and UMB indicator taxa associated with normal taxa. Gray and blue curves represent significantly positive and negative correlation (P < 0.01). The thickness of the edges is proportional to the absolute value of Spearman’s correlation coefficient ranging from 0.8 to 1. The relative abundance cut-off values for the OTUs involved in network analysis were: indicator taxa OTUs > 0.02% and normal taxa OTUs > 0.1%. Each OTU was annotated into the lowest taxonomic rank.
The Fate of Individual Indicator Taxa in the DWTP
Compared with the RW, the total relative abundance of UMB indicator taxa in all UMB communities (almost all UMB indicator taxa OTUs) increased along the following treatment units (FS, RSF, and CW) with the exception of BAC, where the total relative abundance decreased to a level similar to RW, i.e., 13.0% (RW) – 47.4% (FS) – 38.0% (RSF) – 20.3% (BAC) – 62.6% (CW) (ANOVA, P < 0.05) (Figure 6 and Supplementary Figure S4). For LB indicator taxa, the changes of total relative abundance of LB indicator taxa ranged as follows: 31.8% (RW) – 46.9% (FS) – 61.7% (RSF) – 27.4% (BAC) – 37.5% (CW). In comparison to UMB, the variation of LB indicator taxa were mainly attributed to OTU0002 (Phreatobacter: α-Proteobacteria), OTU0011 (Duganella: β-Proteobacteria) and OTU0032 (Flavobacterium: Bacteroidetes), and the remaining major LB indicator taxa gradually reduced along the treatment process in the DWTP. Interestingly, the total relative abundance of both LB and UMB indicator taxa did not show an increase accompanying the increasing cell density and biodiversity in the BAC (Figure 1), but decreased to similar levels as those in the RW (Figure 6 and Supplementary Figure S4).
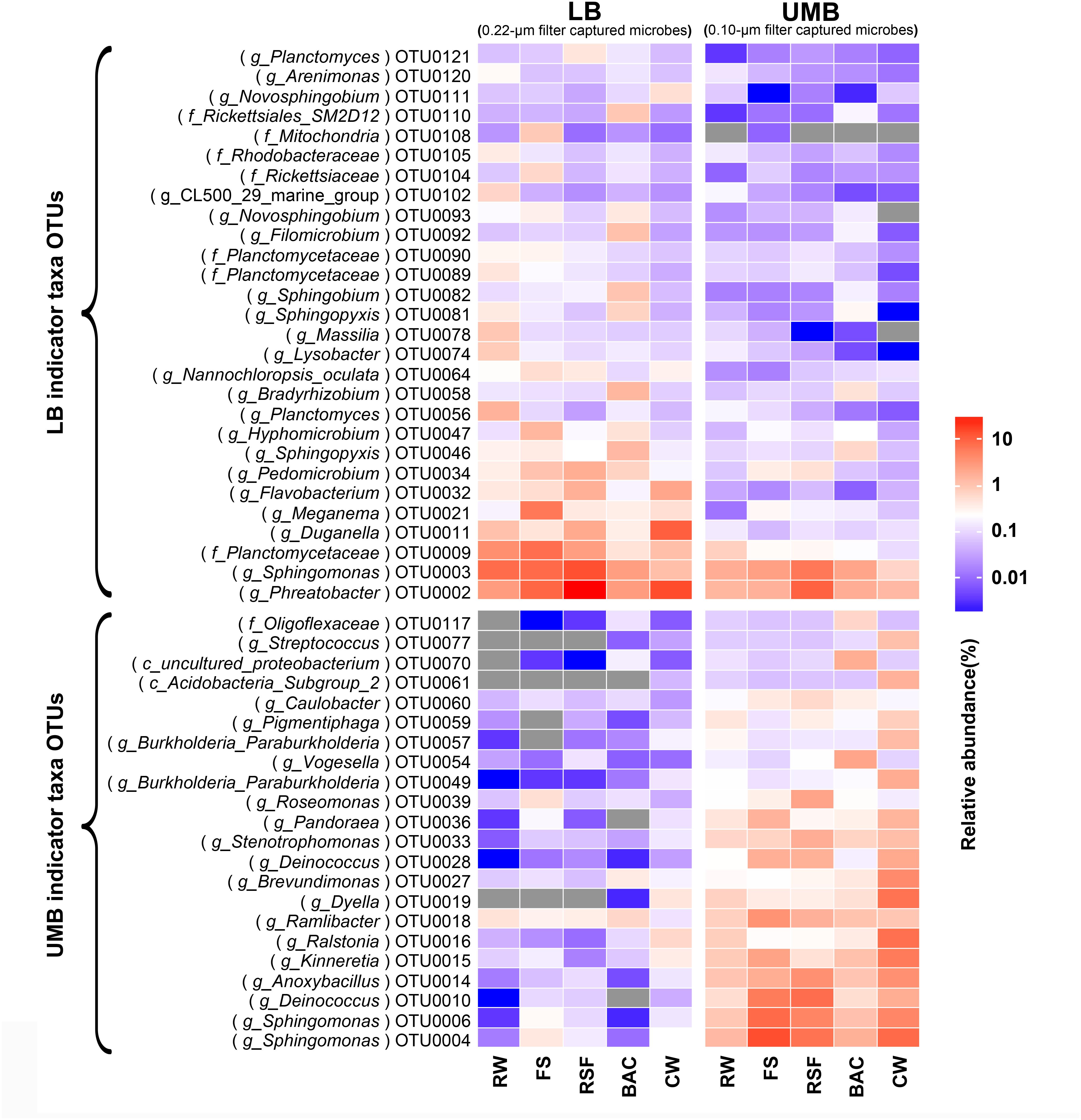
FIGURE 6. The fate of LB and UMB indicator taxa OTUs in the treatment units of a DWTP. Indicator taxa OTUs with a relative abundance more than 0.1% are shown, and each OTU is annotated into the lowest taxonomic rank.
Prediction of Functional and Metabolic Capacities
Procrustes analysis shows that functional and metabolic capacities predicted from 16S rRNA sequencing were significantly different between LB and UMB communities (M2 = 0.73, P < 0.05) (Supplementary Figure S5). These significantly different genes encoding key enzymes could be classified into the following functional/metabolic categories: energy/C/N-associated metabolism, environmental information processing, environmental stress resistance and DNA replication and repair. For detailed KEGG ortholog reference profiles see Supplementary Table S1. Overall, the relative abundances of such specific predicted genes were higher in UMB than in LB (ANOVA, P < 0.01) (Figure 7). In addition, the higher relative abundance of such enzyme-encoding genes in UMB increased along the treatment process in the DWTP.
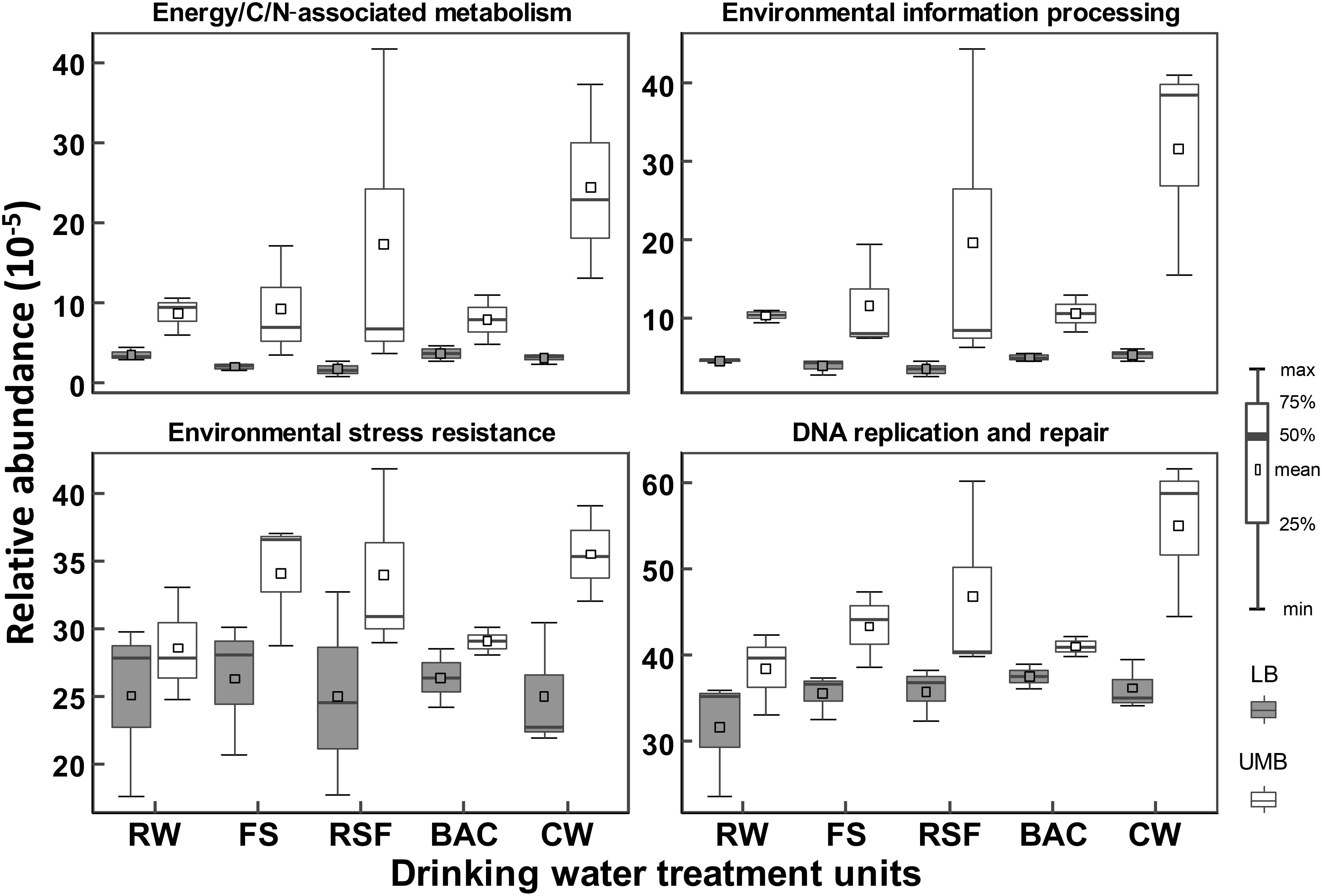
FIGURE 7. Relative abundances of inferred enzyme-encoding genes between LB and UMB in the treatment units of a DWTP. The inferred enzyme-encoding genes involved in the analysis are summarized in Supplementary Table S1.
Discussion
Variance of Bacterial Cell Density and Community
In this study, we profiled the bacterial density, community composition and structure of LB and UMB in a full-scale DWTP using FCM and 0.22/0.1-μm filtration paired with high-throughput sequencing approaches. Within each individual sampling batch, the LB and UMB communities were significantly different (Figures 1, 2). Size-separation (LB vs. UMB) had an important effect on the variation of bacterial community. Filtration via the 0.2-μm pore-size enables the selection of bacteria with a sufficiently small cell structure (Wang et al., 2008a,b) and small genome (Brown et al., 2015). Luef et al. (2015) found that the bacterial community composition of a post-0.2-μm filtrate was remarkably different to that retained on the 0.2-μm filter in ground water. Besides the 0.2-μm size-separation, in nature, such size-fractionated specific distributions of community composition were also evident in 0.4-μm (Proctor et al., 2018) and 1.6-μm (Ganesh et al., 2014) filtrates. UMB and LB were, respectively, termed as low nucleic acid (LNA) and high nucleic acid (HNA) content bacteria in FCM (Wang et al., 2009; Proctor et al., 2018), and LNA were proposed to broadly encompass the categories of UMB, ultra-small bacteria, ultramicrocells, nanobacteria, and candidate phyla radiation (CPR; Proctor et al., 2018). It was demonstrated that 0.45-μm filters can essentially separate HNA and LNA based on flow cytometric fingerprints (Wang et al., 2009). However, UMB are not necessarily equal to bacteria that can pass through a 0.45-μm filter, as LB with one small diameter can also pass through 0.45-μm filters (Wang et al., 2008a). Therefore, we chose the 0.2-μm filter to separate UMB and LB in this study.
With respect to the engineered DWTP system, treatment processes have a considerable effect on the variation of bacterial communities. The cell density of both UMB and LB reduced as the treatment process progressed except in the BAC unit (Figure 1). This pattern agrees with previous studies concerning improvements of water quality in DWTPs (Zhang et al., 2017; Oh et al., 2018). As shown in Figures 2B, 6, the variation in relative abundance of different bacteria in the DWTP suggested that the drinking water treatment processes could impact bacterial communities in different ways. For example, flocculation-sedimentation and sand filtration can physically remove a considerable amount of microorganisms (Li C. et al., 2017; Hou et al., 2018); meanwhile, microorganisms that can preferentially attach to filter media surfaces would not be released with effluents (Pinto et al., 2012). The disinfection process has differential selection pressure for resistant bacteria (Ramseier et al., 2011; Gomez-Alvarez et al., 2012; Chiao et al., 2014; Inkinen et al., 2018). It was reported that the kinetics of cytomembrane damage of LB was much faster than that of UMB during chlorine dioxide and permanganate treatments (Ramseier et al., 2011). Similarly, previous studies also found that the UMB genera Sphingomonas, Methylobacterium, and Legionella, which occurred in the present study, were more abundant in the free chlorine and monochloramine disinfection effluents (Gomez-Alvarez et al., 2012; Wang et al., 2013).
Contrary to the reduction in previous treatment units, bacterial cell density and diversity in the BAC effluent increased to levels comparable to those in the RW. An explanation might be that ozonation placed before the BAC treatment unit could oxidize natural organic matter into low-molecular-weight dissolved organic carbon (DOC). This process results in very little reduction of DOC under low dose ozonation but a remarkable increase of the biodegradable DOC, namely microbial assimilable organic carbon (Velten et al., 2011; Basu et al., 2016; Besmer and Hammes, 2016). Furthermore, owing to the high specific surface area, adsorption capacity and bioavailable substrates, BAC could harbor a more dense and diverse microbiome than sand filter media (Basu et al., 2016). Consequently, with biomass accumulation and shear forces exerted by hydraulic shocks, biofilms would detach from and pass through the BAC filter media, thus increasing cell density in the BAC effluent (Boon et al., 2011; Besmer and Hammes, 2016). Nevertheless, a diverse microbial biofilm community that colonizes in BAC can contribute to improving water treatment efficiency through the metabolism of nutrients and organics (Li D. et al., 2017).
In strong contrast to the reduction of cell density and diversity, the proportion of UMB in total bacteria community increased as the treatment process progressed (Figure 1). This suggested that UMB were more persistent than LB against the treatment processes in DWTPs. The reasons for this might be that UMB could penetrate the filter media more easily than LB due to the ultra-small cell size. Moreover, the multi-stress resistance mechanisms possessed by UMB, e.g., DNA repair mechanisms, superoxide dismutase, peroxide stress response regulator and drug efflux transporter pump (Figure 7 and Supplementary Table S1), could protect UMB against the disinfection treatment process (Eguchi et al., 1996; Cavicchioli and Ostrowski, 2003; Ramseier et al., 2011).
Occurrence of LB and UMB Indicator Taxa
Caution should be exercised when using filter-size separation of UMB since a fraction of UMB can be captured above the surface of membrane filters due to matrix effects at the beginning of filtration (Wang et al., 2008a). In addition, LB with a small diameter in one dimension can also pass through the 0.22-μm filter pore (Wang et al., 2008a; Proctor et al., 2018). Therefore, LB and UMB were not exclusively present on 0.22-μm and 0.1-μm filters, respectively, and there might be overlapping OTUs present in both groups of a filter pair (Figure 2B). To overcome such imperfections caused by filtration and to compare microbial community differentiation, the LEfSe algorithm was employed to determine specific indicator taxa for UMB and LB.
Phylogenetic classification of indicator taxa confirmed previous studies that, the LB and UMB clustering was not entirely consistent and exclusive at the phyla level (Proctor et al., 2018), and that bacterial cell size had considerable variability within a phylum (Stepanauskas et al., 2017). In spite of this, in the present study, some UMB were still exclusively assigned to CPR and symbiont phyla such as Parcubacteria (OD1), Saccharibacteria (TM7), and Elusimicrobia (Figures 3, 4), consistent with several prior studies showing that most CPR bacteria had ultra-small cells (Brown et al., 2015; Luef et al., 2015; Proctor et al., 2018). In addition, the data further proved the prior knowledge that the ultra-small size of UMB is an evolutionarily conserved characteristic, rather than a temporary physiological state, e.g., a starved or inactive state (Luef et al., 2015; Proctor et al., 2018).
At the genera level, only some UMB genera identified in this study, such as Anoxybacillus and Stenotrophomonas, have been previously reported as UMB. The genus Anoxybacillus has the smallest genomes (2.6–2.9 Mb) in the family Bacillaceae, and the presence of adaptive genes enable Anoxybacillus to survive under conditions of heat, UV irradiation, and extreme pH (Goh et al., 2014). The UMB genus Stenotrophomonas has been detected in natural waterborne environments (Sundaram et al., 2001) and potable drinking water (Silbaq, 2009), and is regarded as an epibiont with Bacillus subtilis (Shorokhova et al., 2017). Other genera, including Kinneretia, Ralstonia, Dyella, Ramlibacter, Burkholderia paraburkholderia, Pandoraea, Roseomonas, and Vogesella, have been identified as UMB for the first time in this study. It is noteworthy that the genus Sphingomonas is dominant in both UMB (12.1%) and LB (7.9%) indicator taxa despite numerous Sphingomonas sp. strains being characterized as UMB, e.g., Sphingopyxis alaskensis (formerly Sphingomonas alaskensis) (Eguchi et al., 1996; Cavicchioli et al., 2003). This phenomenon could be due to the intrinsically remarkable variability in cell size of Sphingomonas. Therefore, further characterization is required to explain why such different physical characteristics affiliated with a phylogenetically akin lineage (Proctor et al., 2018). The genus Brevundimonas also overlapped between LB and UMB indicator taxa, in which rod-shaped Brevundimonas diminuta was used as a standard bacterium for 0.2-μm filter-testing.
Significant positive correlation indicated higher intra-taxa co-occurrence within UMB as well as LB. As shown in Figure 5, the positive correlation between Planctomycetes and Methylocystaceae, consistent with a previous study that members of Planctomycetes were involved in methanogenesis and methane oxidation processes (Chistoserdova et al., 2004), might suggest cooperative relations between Planctomycetes and Methylobacterium/Methylocystaceae. Interestingly, the CPR bacteria, including Parcubacteria, Dependentiae and Elusimicrobia, co-occurred strongly and significantly. These non-random co-occurrence patterns revealed that phylogenetically distant UMB bacteria can share similar ecological niches, or possess cooperative relations, and such high connectedness most likely resulted from symbiotic interactions, such as mutualism, commensalism, or parasitism (Nelson and Stegen, 2015; Yeoh et al., 2016).
Natural selective pressures are likely to minimize cell complexity through optimizing bacteria metabolic burden and concomitant loss of involved genes (Giovannoni et al., 2014). The simplicity of the adaptive genome pares down the unusual growth nutrient requirement for DNA synthesis and cell growth, and provides a selective advantage under resource-limited environments (Giovannoni et al., 2005). However, such reductive evolution in streamlined metabolism also leads to the absence of some common regulatory mechanisms (Giovannoni et al., 2014). Consequently, the streamlined UMB lack numerous biosynthetic pathways for producing all essential cellular building blocks, e.g., amino acids and nucleotides, and instead depend on metabolic resources from other microorganisms, thereby living a symbiotic or parasitic lifestyle (McLean et al., 2013; Brown et al., 2015; Luef et al., 2015). For example, Parcubacteria (OD1) could pass through a 0.2-μm filter (Luef et al., 2015) and have a very small genome size (<1 Mb), with severely reduced metabolic functions, e.g., ATP, cofactors, amino acids, nucleotides, and fatty acids synthase (Nelson and Stegen, 2015; Suzuki et al., 2017). Dependentiae (TM6) was globally distributed parasitism and endosymbiosis, was associated with small genomes (1.0–1.5 Mb), and usually lacked complete biosynthesis pathways for most amino acids, lipids, and nucleotides (McLean et al., 2013; Yeoh et al., 2016). The novel phylum Elusimicrobia, with a diameter range of 0.15–0.30 μm, was also reported to be an intracellular symbiont (Geissinger et al., 2009; Zheng et al., 2016). In short, the streamlined genome is connected to UMB following a symbiotic or parasitic lifestyle, which then leads to the observed high connectedness as inferred above, i.e., non-random intra-taxa co-occurrence pattern within UMB.
Predicted Functional Traits of UMB
The higher degree of specific physiological functions might contribute to adaptation of UMB to a multitude of changes in the environments, such as nutrient limitation, reactive oxygen and nitrogen species, membrane damage, elevated temperature, ribosome disruption, symbiotic interactions, and antibiotic pressure (Groisman, 2016; Zhang et al., 2017). As shown in Figure 7, the higher relative abundance of environmental information processing pathways, e.g., two-component regulatory system (Supplementary Table S1), enable UMB to respond quickly to stress conditions (Giovannoni et al., 2005; Groisman, 2016), and environmental stress resistance mechanisms (e.g., SOD1, Fur and Uve1 gene Supplementary Table S1) can protect UMB against UV and hydrogen peroxide stress (Yonemasu et al., 1997; Gomez-Alvarez et al., 2012). It was reported that Deinococcus radiodurans, from the phylum Deinococcus–Thermus, was capable of robust DNA replication and repair processes (Slade et al., 2009), and could strongly resist extreme stresses including γ-ray/UV radiation, oxidation, desiccation and high temperature (Tian and Hua, 2010). Furthermore, consistent with previous studies that the LD12 UMB lineage in α-Proteobacteria exhibited a higher uptake of glutamine and glutamate (Salcher et al., 2011) and UMB could efficiently utilize scarce nutrients (Giovannoni et al., 2005, 2014; Williams et al., 2009; Zhang et al., 2017), the prediction data indicated that genus with functions related to energy, carbohydrate, nucleotide and amino acid metabolism were more abundant in UMB compared with those in LB (Figure 7 and Supplementary Table S1). Altogether, these specific physiological functions likely resulted in the higher resistance of UMB to drinking water treatment processes in comparison to LB.
In summary, the current study profiled bacterial cell density, community structure and potential functions of UMB and LB in a full-scale DWTP. The data confirmed the hypothesis that UMB are more recalcitrant to drinking water treatment processes than LB despite being less diverse, and that the ultra-small structure of UMB is a naturally conserved phylogenetic trait as shown by their exclusivity at a high taxonomic level (i.e., phylum). Furthermore, the results indicated that biological activated carbon could facilitate bacterial growth, and community structure and predicted functions between UMB and LB were significantly different in DWTP. The UMB indicator taxa mainly belonged to α/β/γ-Proteobacteria, Deinococcus–Thermus, Firmicutes, Acidobacteria, and Dependentiae. In addition, the streamlined genomes is likely connected to UMB, such as CPR bacteria, following a symbiotic or parasitic lifestyle, which then results in the non-random intra-taxa co-occurrence patterns. Finally, the higher specific physiological functions, including environmental information processing and DNA replication and repair, could explain the higher resistance of UMB to drinking water treatment processes in comparison to LB.
Author Contributions
JL, XL, and BL conceived and designed the experiments and wrote the paper. JL and RZ performed the experiments. JL, JZ, GZ, and KY analyzed the data.
Conflict of Interest Statement
The authors declare that the research was conducted in the absence of any commercial or financial relationships that could be construed as a potential conflict of interest.
Acknowledgments
We are grateful for financial support from the China Postdoctoral Science Foundation (2016M591190), ShenZhen Knowledge Innovation Program – Basic Research Project (JCYJ20150831192847649 and JCYJ20170412171918012), and the Development and Reform Commission of Shenzhen Municipality (Urban Water Recycling and Environment Safety Program).
Supplementary Material
The Supplementary Material for this article can be found online at: https://www.frontiersin.org/articles/10.3389/fmicb.2018.02922/full#supplementary-material
References
Asshauer, K. P., Wemheuer, B., Daniel, R., and Meinicke, P. (2015). Tax4Fun: predicting functional profiles from metagenomic 16S rRNA data. Bioinformatics 31, 2882–2884. doi: 10.1093/bioinformatics/btv287
Basu, O. D., Dhawan, S., and Black, K. (2016). Applications of biofiltration in drinking water treatment - a review. J. Chem. Technol. Biotechnol. 91, 585–595. doi: 10.1016/j.watres.2017.07.080
Besmer, M. D., and Hammes, F. (2016). Short-term microbial dynamics in a drinking water plant treating groundwater with occasional high microbial loads. Water Res. 107, 11–18. doi: 10.1016/j.watres.2016.10.041
Boon, N., Pycke, B. F. G., Marzorati, M., and Hammes, F. (2011). Nutrient gradients in a granular activated carbon biofilter drives bacterial community organization and dynamics. Water Res. 45, 6355–6361. doi: 10.1016/j.watres.2011.09.016
Brown, C. T., Hug, L. A., Thomas, B. C., Sharon, I., Castelle, C. J., Singh, A., et al. (2015). Unusual biology across a group comprising more than 15% of domain Bacteria. Nature 523, 208–211. doi: 10.1038/nature14486
Cavicchioli, R., and Ostrowski, M. (2003). Ultramicrobacteria. London: Macmillan Publishers Ltd. doi: 10.1038/npg.els.0000309
Cavicchioli, R., Ostrowski, M., Fegatella, F., Goodchild, A., and Guixa-Boixereu, N. (2003). Life under nutrient limitation in oligotrophic marine environments: an eco/physiological perspective of Sphingopyxis alaskensis (formerly Sphingomonas alaskensis). Microb. Ecol. 45, 203–217. doi: 10.1007/s00248-002-3008-6
Chiao, T.-H., Clancy, T. M., Pinto, A., Xi, C., and Raskin, L. (2014). Differential resistance of drinking water bacterial populations to monochloramine disinfection. Environ. Sci. Technol. 48, 4038–4047. doi: 10.1021/es4055725
Chistoserdova, L., Jenkins, C., Kalyuzhnaya, M. G., Marx, C. J., Lapidus, A., Vorholt, J. A., et al. (2004). The enigmatic Planctomycetes may hold a key to the origins of methanogenesis and methylotrophy. Mol. Biol. Evol. 21, 1234–1241. doi: 10.1093/molbev/msh113
Csardi, G., and Nepusz, T. (2006). The igraph software package for complex network research. InterJ. Complex Syst. 1695, 1–9.
Duda, V. I., Suzina, N. E., Polivtseva, V. N., and Boronin, A. M. (2012). Ultramicrobacteria: formation of the concept and contribution of ultramicrobacteria to biology. Microbiology 81, 379–390. doi: 10.1134/S0026261712040054
Egli, T. (2010). How to live at very low substrate concentration. Water Res. 44, 4826–4837. doi: 10.1016/j.watres.2010.07.023
Eguchi, M., Nishikawa, T., Macdonald, K., Cavicchioli, R., Gottschal, J. C., and Kjelleberg, S. (1996). Responses to stress and nutrient availability by the marine ultramicrobacterium Sphingomonas sp. strain RB2256. Appl. Environ. Microbiol. 62, 1287–1294.
Forsberg, K. J., Patel, S., Gibson, M. K., Lauber, C. L., Knight, R., Fierer, N., et al. (2014). Bacterial phylogeny structures soil resistomes across habitats. Nature 509, 612–616. doi: 10.1038/nature13377
Ganesh, S., Parris, D. J., De Long, E. F., and Stewart, F. J. (2014). Metagenomic analysis of size-fractionated picoplankton in a marine oxygen minimum zone. ISME J. 8, 187–211. doi: 10.1038/ismej.2013.144
Geissinger, O., Herlemann, D. P. R., Morschel, E., Maier, U. G., and Brune, A. (2009). The ultramicrobacterium ”Elusimicrobium minutum” gen. nov., sp nov., the first cultivated representative of the termite group 1 phylum. Appl. Environ. Microbiol. 75, 2831–2840. doi: 10.1128/AEM.02697-08
Giovannoni, S. J., Thrash, J. C., and Temperton, B. (2014). Implications of streamlining theory for microbial ecology. ISME J. 8, 1553–1565. doi: 10.1038/ismej.2014.60
Giovannoni, S. J., Tripp, H. J., Givan, S., Podar, M., Vergin, K. L., Baptista, D., et al. (2005). Genome streamlining in a cosmopolitan oceanic bacterium. Science 309, 1242–1245. doi: 10.1126/science.1114057
Goh, K. M., Gan, H. M., Chan, K. G., Chan, G. F., Shahar, S., Chong, C. S., et al. (2014). Analysis of anoxybacillus genomes from the aspects of lifestyle adaptations, prophage diversity, and carbohydrate metabolism. PLoS One 9:e90549. doi: 10.1371/journal.pone.0090549
Gomez-Alvarez, V., Revetta, R. P., and Santo Domingo, J. W. (2012). Metagenomic analyses of drinking water receiving different disinfection treatments. Appl. Environ. Microbiol. 78, 6095–6102. doi: 10.1128/AEM.01018-12
Gözdereliler, E., Boon, N., Aamand, J., De Roy, K., Granitsiotis, M. S., Albrechtsen, H. J., et al. (2013). Comparing metabolic functionalities, community structures, and dynamics of herbicide-degrading communities cultivated with different substrate concentrations. Appl. Environ. Microbiol. 79, 367–375. doi: 10.1128/AEM.02536-12
Groisman, E. A. (2016). “Feedback control of two-component regulatory systems,” in Annual Review of Microbiology, Vol. 70, ed. S. Gottesman (Palo Alto: Annual Reviews), 103–124.
Hahn, M. W. (2004). Broad diversity of viable bacteria in ’sterile’ (0.2 μm) filtered water. Res. Microbiol. 155, 688–691. doi: 10.1016/j.resmic.2004.05.003
Hahn, M. W., Stadler, P., Wu, Q. L., and Pöckl, M. (2004). The filtration–acclimatization method for isolation of an important fraction of the not readily cultivable bacteria. J. Microbiol. Methods 57, 379–390. doi: 10.1016/j.mimet.2004.02.004
Hou, L., Zhou, Q., Wu, Q., Gu, Q., Sun, M., and Zhang, J. (2018). Spatiotemporal changes in bacterial community and microbial activity in a full-scale drinking water treatment plant. Sci. Total Environ. 625, 449–459. doi: 10.1016/j.scitotenv.2017.12.301
Inkinen, J., Jayaprakash, B., Ahonen, M., Pitkanen, T., Makinen, R., Pursiainen, A., et al. (2018). Bacterial community changes in copper and PEX drinking water pipeline biofilms under extra disinfection and magnetic water treatment. J. Appl. Microbiol. 124, 611–624. doi: 10.1111/jam.13662
Ju, F., and Zhang, T. (2015). Bacterial assembly and temporal dynamics in activated sludge of a full-scale municipal wastewater treatment plant. ISME J. 9, 683–695. doi: 10.1038/ismej.2014.162
Kozich, J. J., Westcott, S. L., Baxter, N. T., Highlander, S. K., and Schloss, P. D. (2013). Development of a dual-index sequencing strategy and curation pipeline for analyzing amplicon sequence data on the MiSeq Illumina sequencing platform. Appl. Environ. Microbiol. 79, 5112–5120. doi: 10.1128/AEM.01043-13
Letunic, I., and Bork, P. (2016). Interactive tree of life (iTOL) v3: an online tool for the display and annotation of phylogenetic and other trees. Nucleic Acids Res. 44, W242–W245. doi: 10.1093/nar/gkw290
Li, C., Ling, F., Zhang, M., Liu, W.-T., Li, Y., and Liu, W. (2017). Characterization of bacterial community dynamics in a full-scale drinking water treatment plant. J. Environ. Sci. 51, 21–30. doi: 10.1016/j.jes.2016.05.042
Li, D., Stanford, B., Dickenson, E., Khunjar, W. O., Homme, C. L., Rosenfeldt, E. J., et al. (2017). Effect of advanced oxidation on N-nitrosodimethylamine (NDMA) formation and microbial ecology during pilot-scale biological activated carbon filtration. Water Res. 113, 160–170. doi: 10.1016/j.watres.2017.02.004
Liu, J., Ma, D., Ma, L., Song, Y., Gao, G., and Wang, Y. (2017a). Geographic distribution pattern of low and high nucleic acid content bacteria on a river-catchment scale. Mar. Freshw. Res. 68, 1618–1625. doi: 10.1071/MF16068
Liu, J., Zhao, Z., Chen, C., Cao, P., and Wang, Y. (2017b). In-situ features of LNA and HNA bacteria in branch ends of drinking water distribution systems. J. Water Supply Res. Technol. Aqua 66, 300–307. doi: 10.2166/aqua.2017.108
Liu, T., Kong, W., Chen, N., Zhu, J., Wang, J., He, X., et al. (2016). Bacterial characterization of Beijing drinking water by flow cytometry and MiSeq sequencing of the 16S rRNA gene. Ecol. Evol. 6, 923–934. doi: 10.1002/ece3.1955
Loveland-Curtze, J., Miteva, V., and Brenchley, J. (2010). Novel ultramicrobacterial isolates from a deep Greenland ice core represent a proposed new species, Chryseobacterium greenlandense sp nov. Extremophiles 14, 61–69. doi: 10.1007/s00792-009-0287-6
Luef, B., Frischkorn, K. R., Wrighton, K. C., Holman, H. Y. N., Birarda, G., Thomas, B. C., et al. (2015). Diverse uncultivated ultra-small bacterial cells in groundwater. Nat. Commun. 6:6372. doi: 10.1038/ncomms7372
Ma, L., Li, B., Jiang, X.-T., Wang, Y.-L., Xia, Y., Li, A.-D., et al. (2017). Catalogue of antibiotic resistome and host-tracking in drinking water deciphered by a large scale survey. Microbiome 5:154. doi: 10.1186/s40168-017-0369-0
May, H. D., Miller, G. S., Kjellerup, B. V., and Sowers, K. R. (2008). Dehalorespiration with Polychlorinated Biphenyls by an anaerobic ultramicrobacterium. Appl. Environ. Microbiol. 74, 2089–2094. doi: 10.1128/AEM.01450-07
McLean, J. S., Lombardo, M. J., Badger, J. H., Edlund, A., Novotny, M., Yee-Greenbaum, J., et al. (2013). Candidate phylum TM6 genome recovered from a hospital sink biofilm provides genomic insights into this uncultivated phylum. Proc. Natl. Acad. Sci. U.S.A. 110, E2390–E2399. doi: 10.1073/pnas.1219809110
Miteva, V. I., and Brenchley, J. E. (2005). Detection and isolation of ultrasmall microorganisms from a 120,000-year-old Greenland glacier ice core. Appl. Environ. Microbiol. 71, 7806–7818. doi: 10.1128/AEM.71.12.7806-7818.2005
Moran, X. A., Alonso-Saez, L., Nogueira, E., Ducklow, H. W., Gonzalez, N., Lopez-Urrutia, A., et al. (2015). More, smaller bacteria in response to ocean’s warming? Proc. R. Soc. B Biol. Sci. 282:20150371. doi: 10.1098/rspb.2015.0371
Nelson, W. C., and Stegen, J. C. (2015). The reduced genomes of Parcubacteria (OD1) contain signatures of a symbiotic lifestyle. Front. Microbiol. 6:713. doi: 10.3389/fmicb.2015.00713
Neuenschwander, S. M., Salcher, M. M., and Pernthaler, J. (2015). Fluorescence in situ hybridization and sequential catalyzed reporter deposition (2C-FISH) for the flow cytometric sorting of freshwater ultramicrobacteria. Front. Microbiol. 6:247. doi: 10.3389/fmicb.2015.00247
Oh, S., Hammes, F., and Liu, W.-T. (2018). Metagenomic characterization of biofilter microbial communities in a full-scale drinking water treatment plant. Water Res. 128, 278–285. doi: 10.1016/j.watres.2017.10.054
Oksanen, J., Blanchet, F. G., Friendly, M., Kindt, R., Legendre, P., Mcglinn, D., et al. (2018). vegan: Community Ecology Package. R package version 2.5-1. Available at: https://CRAN.R-project.org/package=vegan.
Pinto, A. J., Xi, C., and Raskin, L. (2012). Bacterial community structure in the drinking water microbiome is governed by filtration processes. Environ. Sci. Technol. 46, 8851–8859. doi: 10.1021/es302042t
Proctor, C. R., Besmer, M. D., Langenegger, T., Beck, K., Walser, J.-C., Ackermann, M., et al. (2018). Phylogenetic clustering of small low nucleic acid-content bacteria across diverse freshwater ecosystems. ISME J. 12, 1344–1359. doi: 10.1038/s41396-018-0070-8
Quast, C., Pruesse, E., Yilmaz, P., Gerken, J., Schweer, T., Yarza, P., et al. (2013). The SILVA ribosomal RNA gene database project: improved data processing and web-based tools. Nucleic Acids Res. 41, D590–D596. doi: 10.1093/nar/gks1219
Ramseier, M. K., Von Gunten, U., Freihofer, P., and Hammes, F. (2011). Kinetics of membrane damage to high (HNA) and low (LNA) nucleic acid bacterial clusters in drinking water by ozone, chlorine, chlorine dioxide, monochloramine, ferrate(VI), and permanganate. Water Res. 45, 1490–1500. doi: 10.1016/j.watres.2010.11.016
Roesel, S., and Grossart, H.-P. (2012). Contrasting dynamics in activity and community composition of free-living and particle-associated bacteria in spring. Aquat. Microb. Ecol. 66, 169–181. doi: 10.3354/ame01568
Ross, N., Villemur, R., Marcandella, E., and Deschenes, L. (2001). Assessment of changes in biodiversity when a community of ultramicrobacteria isolated from groundwater is stimulated to form a biofilm. Microb. Ecol. 42, 56–68.
Salcher, M. M., Pernthaler, J., and Posch, T. (2011). Seasonal bloom dynamics and ecophysiology of the freshwater sister clade of SAR11 bacteria ’that rule the waves’ (LD12). ISME J. 5, 1242–1252. doi: 10.1038/ismej.2011.8
Segata, N., Izard, J., Waldron, L., Gevers, D., Miropolsky, L., Garrett, W. S., et al. (2011). Metagenomic biomarker discovery and explanation. Genome Biol. 12:R60. doi: 10.1186/gb-2011-12-6-r60
Shorokhova, A. P., Suzina, N. E., Polivtseva, V. N., Esikova, T. Z., Ross, D. V., Kholodenko, V. P., et al. (2017). Sporulation of Bacillus subtilis in binary cultures with ultramicrobacteria. Microbiology 86, 42–48. doi: 10.1134/S002626171701012X
Silbaq, F. S. (2009). Viable ultramicrocells in drinking water. J. Appl. Microbiol. 106, 106–117. doi: 10.1111/j.1365-2672.2008.03981.x
Slade, D., Lindner, A. B., Paul, G., and Radman, M. (2009). Recombination and replication in DNA repair of heavily irradiated Deinococcus radiodurans. Cell 136, 1044–1055. doi: 10.1016/j.cell.2009.01.018
Stepanauskas, R., Fergusson, E. A., Brown, J., Poulton, N. J., Tupper, B., Labonte, J. M., et al. (2017). Improved genome recovery and integrated cell-size analyses of individual uncultured microbial cells and viral particles. Nat. Commun. 8:84. doi: 10.1038/s41467-017-00128-z
Sundaram, S., Mallick, S., Eisenhuth, J., Howard, G., and Brandwein, H. (2001). Retention of water-borne bacteria by membrane filters - Part II: Scanning electron microscopy (SEM) and fatty acid methyl ester (FAME) characterization of bacterial species recovered downstream of 0.2/0.22 micron rated filters. PDA J. Pharm. Sci. Technol. 55, 87–113.
Suzina, N. E., Esikova, T. Z., Oleinikov, R. R., Gafarov, A. B., Shorokhova, A. P., Polivtseva, V. N., et al. (2015). Comparative characteristics of free-living ultramicroscopical bacteria obtained from natural biotopes. Appl. Biochem. Microbiol. 51, 159–168. doi: 10.1134/S0003683815020192
Suzuki, S., Ishii, S. I., Hoshino, T., Rietze, A., Tenney, A., Morrill, P. L., et al. (2017). Unusual metabolic diversity of hyperalkaliphilic microbial communities associated with subterranean serpentinization at The Cedars. ISME J. 11, 2584–2598. doi: 10.1038/ismej.2017.111
Tian, B., and Hua, Y. J. (2010). Carotenoid biosynthesis in extremophilic Deinococcus-Thermus bacteria. Trends Microbiol. 18, 512–520. doi: 10.1016/j.tim.2010.07.007
Velten, S., Boller, M., Koester, O., Helbing, J., Weilenmann, H.-U., and Hammes, F. (2011). Development of biomass in a drinking water granular active carbon (GAC) filter. Water Res. 45, 6347–6354. doi: 10.1016/j.watres.2011.09.017
Wang, H., Pryor, M. A., Edwards, M. A., Falkinham, J. O., and Pruden, A. (2013). Effect of GAC pre-treatment and disinfectant on microbial community structure and opportunistic pathogen occurrence. Water Res. 47, 5760–5772. doi: 10.1016/j.watres.2013.06.052
Wang, Y. Y., Hammes, F., Boon, N., Chami, M., and Egli, T. (2009). Isolation and characterization of low nucleic acid (LNA)-content bacteria. ISME J. 3, 889–902. doi: 10.1038/ismej.2009.46
Wang, Y. Y., Hammes, F., DüGgelin, M., and Egli, T. (2008a). Influence of size, shape, and flexibility on bacterial passage through micropore membrane filters. Environ. Sci. Technol. 42, 6749–6754.
Wang, Y. Y., Hammes, F., and Egli, T. (2008b). The impact of industrial-scale cartridge filtration on the native microbial communities from groundwater. Water Res. 42, 4319–4326. doi: 10.1016/j.watres.2008.07.027
Williams, T. J., Ertan, H., Ting, L., and Cavicchioli, R. (2009). Carbon and nitrogen substrate utilization in the marine bacterium Sphingopyxis alaskensis strain RB2256. ISME J. 3, 1036–1052. doi: 10.1038/ismej.2009.52
Yeoh, Y. K., Sekiguchi, Y., Parks, D. H., and Hugenholtz, P. (2016). Comparative genomics of candidate phylum TM6 suggests that parasitism is widespread and ancestral in this lineage. Mol. Biol. Evol. 33, 915–927. doi: 10.1093/molbev/msv281
Yonemasu, R., Mccready, S. J., Murray, J. M., Osman, F., Takao, M., Yamamoto, K., et al. (1997). Characterization of the alternative excision repair pathway of UV-damaged DNA in Schizosaccharomyces pombe. Nucleic Acids Res. 25, 1553–1558. doi: 10.1093/nar/25.8.1553
Zhang, Y., Oh, S., and Liu, W.-T. (2017). Impact of drinking water treatment and distribution on the microbiome continuum: an ecological disturbance’s perspective. Environ. Microbiol. 19, 3163–3174. doi: 10.1111/1462-2920.13800
Zheng, H., Dietrich, C., Radek, R., and Brune, A. (2016). Endomicrobium proavitum, the first isolate of Endomicrobia class. nov (phylum Elusimicrobia) – an ultramicrobacterium with an unusual cell cycle that fixes nitrogen with a Group IV nitrogenase. Environ. Microbiol. 18, 191–204. doi: 10.1111/1462-2920.12960
Keywords: ultramicrobacteria, drinking water treatment, microbial community, high-throughput sequencing, flow cytometry, 0.22-μm filtration, metabolic functions, genome streamlining
Citation: Liu J, Zhao R, Zhang J, Zhang G, Yu K, Li X and Li B (2018) Occurrence and Fate of Ultramicrobacteria in a Full-Scale Drinking Water Treatment Plant. Front. Microbiol. 9:2922. doi: 10.3389/fmicb.2018.02922
Received: 16 July 2018; Accepted: 14 November 2018;
Published: 05 December 2018.
Edited by:
Frederik Hammes, Swiss Federal Institute of Aquatic Science and Technology, SwitzerlandReviewed by:
Helmut Bürgmann, Eawag: Swiss Federal Institute of Aquatic Science and Technology, SwitzerlandVarun N. Srinivasan, Northeastern University, United States
Copyright © 2018 Liu, Zhao, Zhang, Zhang, Yu, Li and Li. This is an open-access article distributed under the terms of the Creative Commons Attribution License (CC BY). The use, distribution or reproduction in other forums is permitted, provided the original author(s) and the copyright owner(s) are credited and that the original publication in this journal is cited, in accordance with accepted academic practice. No use, distribution or reproduction is permitted which does not comply with these terms.
*Correspondence: Xiaoyan Li, lixiaoyan@sz.tsinghua.edu.cn Bing Li, bingli@sz.tsinghua.edu.cn