- 1Department of Economic Development, Jobs, Transport and Resources, Agriculture Victoria Research, AgriBio, Bundoora, VIC, Australia
- 2CSIRO Agriculture and Food, Glen Osmond, SA, Australia
- 3College of Medicine and Public Health, Flinders University, Bedford Park, SA, Australia
- 4School of Applied Systems Biology, La Trobe University, Melbourne, VIC, Australia
The soilborne fungus Rhizoctonia solani anastomosis group (AG) 8 is a major pathogen of grain crops resulting in substantial production losses. In the absence of resistant cultivars of wheat or barley, a sustainable and enduring method for disease control may lie in the enhancement of biological disease suppression. Evidence of effective biological control of R. solani AG8 through disease suppression has been well documented at our study site in Avon, South Australia. A comparative metatranscriptomic approach was applied to assess the taxonomic and functional characteristics of the rhizosphere microbiome of wheat plants grown in adjacent fields which are suppressive and non-suppressive to the plant pathogen R. solani AG8. Analysis of 12 rhizosphere metatranscriptomes (six per field) was undertaken using two bioinformatic approaches involving unassembled and assembled reads. Differential expression analysis showed the dominant taxa in the rhizosphere based on mRNA annotation were Arthrobacter spp. and Pseudomonas spp. for non-suppressive samples and Stenotrophomonas spp. and Buttiauxella spp. for the suppressive samples. The assembled metatranscriptome analysis identified more differentially expressed genes than the unassembled analysis in the comparison of suppressive and non-suppressive samples. Suppressive samples showed greater expression of a polyketide cyclase, a terpenoid biosynthesis backbone gene (dxs) and many cold shock proteins (csp). Non-suppressive samples were characterised by greater expression of antibiotic genes such as non-heme chloroperoxidase (cpo) which is involved in pyrrolnitrin synthesis, and phenazine biosynthesis family protein F (phzF) and its transcriptional activator protein (phzR). A large number of genes involved in detoxifying reactive oxygen species (ROS) and superoxide radicals (sod, cat, ahp, bcp, gpx1, trx) were also expressed in the non-suppressive rhizosphere samples most likely in response to the infection of wheat roots by R. solani AG8. Together these results provide new insight into microbial gene expression in the rhizosphere of wheat in soils suppressive and non-suppressive to R. solani AG8. The approach taken and the genes involved in these functions provide direction for future studies to determine more precisely the molecular interplay of plant-microbe-pathogen interactions with the ultimate goal of the development of management options that promote beneficial rhizosphere microflora to reduce R. solani AG8 infection of crops.
Introduction
Rhizoctonia root rot and bare patch disease, caused by the soilborne fungus Rhizoctonia solani anastomosis group (AG) 8, results in significant losses in cereal crops due to patches of stunted plants with reduced tillers and grain production (Hynes, 1933; Macnish, 1983; Rovira, 1986; Paulitz et al., 2010). In Australia yield losses are estimated to be $77 million per annum for wheat (Triticum aestivum) and barley (Hordeum vulgare) (Murray and Brennan, 2009, 2010). Rhizoctonia solani AG8 has a wide host range i.e., cereal crops, grasses, broadleaf crops, and weed species, but non-cereal crops in rotation have been shown to reduce pathogen inoculum levels (Gupta et al., 2012). However, in the absence of resistant cultivars of wheat or barley, current control measures are limited to management strategies such as cultivation to reduce R. solani AG8 hyphal networks, and herbicide application to remove inoculum carryover by weeds and volunteer plants (Roget, 1995; Roget et al., 1996). Fungicide seed dressings for pathogen control have previously proved ineffective or unreliable although a new product showing potential has been reported (Mckay et al., 2014; Almasudy et al., 2015). Therefore, a sustainable and enduring method for disease control is needed and may lie in the enhancement of biological disease suppression, where the resident microbial community counteracts the pathogen and/or restricts disease incidence (Cook et al., 1995; Wiseman et al., 1996; Roget et al., 1999).
In Australia and the Pacific Northwest of the USA, disease suppression has been demonstrated for R. solani AG8 and Gaeumannomyces graminis var. tritici (Ggt) (take-all disease) in cereal crops in farmer's fields and long-term trial sites, usually in association with continuous cereal cropping, stubble retention and no-till practices (Macnish, 1988; Roget, 1995; Pankhurst et al., 2002; Schillinger and Paulitz, 2006, 2014). Disease suppressive soils are defined as soils in which, because of their microbial composition and activity, a pathogen does not establish or persist, establishes but causes little or no disease, or establishes and causes disease for a while but thereafter the disease declines with successive crops of a susceptible host, even though the pathogen may persist in the soil (Cook and Baker, 1983; Schlatter et al., 2017). Soils with these characteristics have been identified globally for various soilborne plant pathogens including R. solani (Anees et al., 2010; Watanabe et al., 2011; Mavrodi et al., 2012), Ggt (Raaijmakers and Weller, 1998; McSpadden Gardener and Weller, 2001), Plasmodiophora spp. (Hjort et al., 2010), Streptomyces spp. (Sagova-Mareckova et al., 2015), Thielaviopsis basicola (Kyselková et al., 2009), Pythium ultimum (Löbmann et al., 2016), and Fusarium oxysporum (Alabouvette, 1986).
Various microbial mechanisms of disease suppression have been proposed. These include competition for sites and nutrients, particularly in the rhizosphere; antagonism via microbial production of volatiles, extracellular lytic enzymes and secondary metabolites such as iron-chelating siderophores and antibiotics; hyperparasitism; and elicitation of induced systemic resistance (ISR) by rhizobacteria (Weller et al., 2002; Raaijmakers et al., 2009; Pieterse et al., 2014; Schlatter et al., 2017). Antibiosis is the most studied mechanism of disease suppressive soils, where the growth and/or activity of one organism is inhibited by another organism via the production of specific or non-specific metabolites (Gómez Expósito et al., 2017). Antimicrobial producing bacteria, in particular Pseudomonas spp. capable of producing 2,4-diacetylphloroglucinol (DAPG), have received considerable attention because of their roles in suppression of black root rot of tobacco (Stutz et al., 1986; Ramette et al., 2006) and take-all of wheat (Raaijmakers and Weller, 1998; Cook, 2006). Latz et al. (2012) showed a correlation between disease suppression of R. solani (AG2–2IIIB) in sugarbeet seedlings and the abundance of two antibiotic genes phlD and prnD which produce the antifungal compounds DAPG and pyrrolnitrin respectively. Also being considered is the role of antimicrobial compounds at sub-inhibitory concentrations in modifying microbial community activity through intercellular signalling, motility, stress response, and biofilm formation (Romero et al., 2011; Philippot et al., 2013). Volatile compounds have been proposed to have a role in disease suppression as they may allow communication and competition between physically separated soil microorganisms, resulting in antagonistic interactions and changes in antibiotic and secondary metabolite production (Garbeva et al., 2014; Tyc et al., 2017). For most suppressive soils the mechanisms of suppression have not been fully defined (Schlatter et al., 2017). It has proven difficult to identify the mechanisms by which microorganisms suppress disease for many plant pathogens, possibly because of the multitude of interactive factors associated with crop type and cultivar, soil type, environment, and agronomic management practices, as well as the possibility of multiple organisms being involved. The microbial mechanism or mechanisms responsible for the suppression of R. solani AG8 are currently unknown.
The diversity of microorganisms involved in the suppression of some plant pathogens has been elucidated through the use of DNA-based community profiling techniques such as sequencing or microarrays (Kyselková et al., 2009, 2014; Sanguin et al., 2009; Mendes et al., 2011; Klein et al., 2013; Cha et al., 2015), including for R. solani AG8 suppressive soils (Yin et al., 2013; Donn et al., 2014; Penton et al., 2014). By contrast, the use of metagenomics to characterise the community genetic potential and metatranscriptomics to identify the expressed genes (mRNA) and active metabolic processes of microbial communities in suppressive soils remains novel and presents a significant opportunity to identify the complex microbial mechanisms. Two previous studies have examined disease suppression mechanisms using metagenomic or metatranscriptomic approaches. Hjort et al. (2010) focussed on the diversity of an antifungal chitinase gene in metagenomic DNA extracted from soil suppressive to club root disease of cabbage. In a metagenomic examination of the rhizosphere of sugar beet seedlings grown in pots, the introduction of R. solani AG2-2IIIB to a suppressive soil did not result in any change in bacterial composition, however metatranscriptomic analyses did reveal upregulation of stress related genes for oxidative stress response and guanosine-3,5-bispyrophosphate (ppGpp) metabolism in specific bacterial families (Chapelle et al., 2015). Based on these findings Chapelle et al. (2015) proposed a model for disease suppression whereby R. solani secretes oxalic and phenylacetic acid during colonization of the roots which exerts oxidative stress in the rhizobacterial community and the plant. This in turn may activate enhanced motility, biofilm formation and the production of yet unknown secondary metabolites by rhizosphere microbes to stop plant infection.
In this field-based experiment we examine the rhizosphere microbiome because it represents the frontline of defence for plant roots against attack by soilborne pathogens (Cook et al., 1995; Bowen and Rovira, 1999), and used a field site at Avon, South Australia that is historically well characterised for R. solani suppression (Roget, 1995; Roget et al., 1996; Barnett et al., 2006; Gupta et al., 2011; Donn et al., 2014; Penton et al., 2014). The objectives of this study were to use comparative metatranscriptomics (RNASeq) to characterise the functions and taxonomy of the rhizosphere microbiome in soils which are suppressive and non-suppressive for R. solani AG8, and identify differentially expressed microbial functional genes which may play a mechanistic role in disease suppression of R. solani AG8. Two different bioinformatic approaches were used to examine the rhizosphere samples based on unassembled and assembled analyses of the metatranscriptome libraries, and we report on differences in their annotation and differential expression analysis.
Materials and Methods
Site Details and Management Regimes
Two adjacent fields were studied on a farm in Avon, South Australia, in the southern wheat cropping region of Australia. The fields were suppressive (34°13′58.86″S, 138°18′35.16″E) and non-suppressive (34°14′23.70″S, 138°18′43.51″E) for the soilborne fungal pathogen R. solani AG8. The land at Avon has been utilised in field trials by the Commonwealth Scientific and Industrial Research Organisation (CSIRO) for over 25 years with the suppression of R. solani in cereal crops, resulting in reduced disease incidence, being well documented for more than 20 years (Roget, 1995; Roget et al., 1996; Barnett et al., 2006; Gupta et al., 2011; Donn et al., 2014; Penton et al., 2014).
The soil in both fields is classed as a Lithocalcic Calcarosol (Isbell, 2002). The climate is dominated by winter rainfall during the cereal cropping season and hot dry summers. Over the last 35 years the average total rainfall in the district was 338 mm per year, while the average growing season (April to October) rainfall was 253 mm (Bureau of Meteorology, Australia). For the 4 years prior to sampling, both fields were sown to cereals with wheat (T. aestivum cv. Gladius), barley (H. vulgare cv. Fleet), and oats (Avena sativa cv. Wallaroo) used in rotation. Both fields have been under continuous cereal cropping with stubble retention; though in the suppressive field this has occurred for more than 12 years compared to only 4 years in the non-suppressive field. Agronomic practices were similar for the two fields and comprised of direct drill sowing, fertiliser applications at sowing and 3 months after, and herbicide applications prior to sowing, during cropping and in the summer fallow period. In the year of sampling, both fields had been sown to the wheat variety Gladius on the same date (5 June 2012).
Soil Sampling
For each field, soil samples were collected 8 weeks post-sowing on 31 July 2012 when wheat was at tillering stage (GS20-29) and Rhizoctonia bare patches were visible in the non-suppressive field (Figure S1). There were four designated replicated 100 m2 plots in each field (GPS location described above) with two samples collected per plot, totalling eight soil samples per field. Each soil sample was made up of composited root+soil samples collected by digging up multiple wheat plants (~20) to a depth of 10 cm. All plants were collected randomly within the plot, hence samples included plants from both inside and outside of any disease patches. To collect soil samples, the root mass of each plant was gently shaken by hand. Soil that detached after gentle shaking was considered to be bulk soil, well homogenised for each sample, and eight samples were collected from each of the two fields for soil chemical and physical analyses (Table S1). Soil that was strongly adhered to the roots was considered as rhizosphere soil and detached by vigorous shaking of the root mass by hand. The rhizosphere soil was well homogenised for the samples and preserved in the field for RNA extractions. Eight rhizosphere samples each were collected from the disease suppressive and non-suppressive fields. Each rhizosphere sample for metatranscriptomics analysis was preserved for RNA extraction by adding 2 g of soil representing many different soil particles per sample to a tube containing 5 ml of Lifeguard (Mo-Bio, Carlsbad, CA, USA). The soil solution was shaken to make a suspension and then the tubes were kept at room temperature until our return to the laboratory where they were stored at −20°C. Bulk soil samples for chemical and physical analyses were stored at 4°C until they were shipped to a commercial laboratory (CSBP Lab, Bibra Lake, WA, Australia) for analyses according to National Association of Testing Authorities accredited standard procedures (http://www.nata.com.au).
Additional soil samples were also collected for the purpose of monitoring the abundance of R. solani AG8 inoculum in both fields. Samples were collected 1 week prior to sowing with a total of eight samples collected per field. Each sample was the product of 8 soil cores of 0–10 cm depth composited together, with two samples collected per replicate plot. Samples were also collected three times during the cropping season, using the rhizosphere sampling protocol described above, at 6 weeks post-sowing (plant disease incidence rating), 18 weeks post-sowing (physiological maturity), and 23 weeks post-sowing (harvest). The abundance of R. solani AG8 inoculum in both fields was determined by quantitative PCR (qPCR) as described in Penton et al. (2014). Briefly, DNA was extracted from each soil sample (~125 g) by the Root Disease Testing Service at SARDI (Adelaide, Australia) (Ophel-Keller et al., 2008). The qPCR assay was conducted using rDNA (TaqMan) probe sequences specific to R. solani AG8 (Ophel-Keller et al., 2008) and quantified against a standard curve of diluted R. solani AG8 genomic DNA (pg g−1 soil). Statistical significance of the difference in R. solani AG8 inoculum between suppressive and non-suppressive samples collected at the same time was tested using a paired t-test at P < 0.01.
Root Disease Assessment
Plant samples were collected from both fields at 6 weeks post-sowing for Rhizoctonia root rot disease assessment. Twenty plants were collected for each of the 8 replicates per field, with two samples collected from each of the four designated replicated 100 m2 plots described above. Roots were carefully washed to remove adhered soil, scored for the incidence and severity of Rhizoctonia root rot disease using a 0–5 rating scale, and average percent disease index scores calculated (Mcdonald and Rovira, 1985). Statistical significance of the difference in the disease index (%) between suppressive and non-suppressive samples was tested using a paired t-test at P < 0.01.
RNA Extraction and cDNA Sequencing
MoBio Lifeguard was removed from the thawed 2 g soil samples by centrifugation at 2,500 × g for 5 min. The solution was removed and the RNA extracted using an RNA PowerSoil Total RNA Isolation Kit (Mo-Bio). Extracted RNA was treated with DNase I (Turbo-DNA-free kit; Applied Biosystems, Foster City, CA, USA) to remove all traces of DNA. RNA quality and quantity was assessed using a NanoDrop 2000 spectrophometer (ThermoScientific) and gel electrophoresis.
Twelve samples (six suppressive and six non-suppressive) were used for mRNA enrichment using a Ribo-Zero Magnetic kit for Bacteria (specificity: 16S, 23S, and 5S rRNA from Gram-positive and Gram-negative bacterial total RNA) (Epicentre, Madison, WI, USA) according to manufacturer's instructions. A quantity of 1.65 μg of total RNA was used for each sample for mRNA enrichment. Ribosomal RNA-depleted RNA was cleaned by ethanol precipitation and the pellet resuspended in 18 μl of Elute, Prime, Fragment mix (TruSeq Stranded mRNA kit; Illumina, San Diego, CA, USA). Double-stranded cDNA was generated from the mRNA enriched RNA using Superscript II reverse transcriptase (Invitrogen, Carslbad, CA, USA) as per manufacturer's instructions. Library preparation using a TruSeq Stranded mRNA kit, processing and sequencing were performed in-house using the Illumina HiSeq 2000 with paired-end (PE) 100 bp sequencing of templates ~295 bp long. Metatranscriptomic libraries generated for all rhizosphere samples from the suppressive and non-suppressive fields (12 samples) are deposited in the NCBI Short Read Archive (study SRP126206; accession numbers SRR6349879–SRR6349890). The assembled metatranscriptome and Trinotate annotation file is available through Figshare (DOI:10.6084/m9.figshare.5657215).
Bioinformatic Analyses
The raw Illumina paired-end sequence reads were quality filtered with the adaptors and low quality bases removed using Trimmomatic (Bolger et al., 2014). Default settings were used with the exception that minimum sequence length was set at 50 bp. Quality control of the filtered sequencing reads was performed by visual inspection using FastQC (http://www.bioinformatics.babraham.ac.uk/projects/fastqc). Two bioinformatic approaches were used to characterise the metatranscriptomes (see Figure 1 for the work flow). The first approach utilised unassembled transcripts to compare metatranscriptome libraries, while the second approach used was based on metatranscriptome assembly and undertaken to improve both annotation of transcripts and statistical analyses of differential expression of transcripts between suppressive and non-suppressive samples.
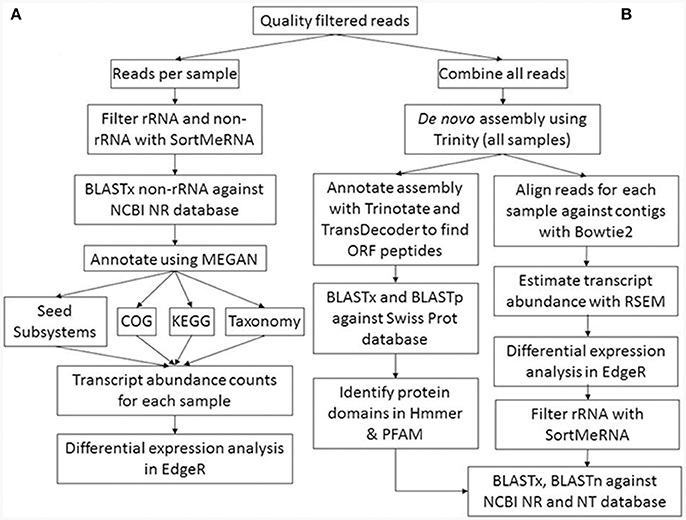
Figure 1. Metatranscriptome bioinformatic workflow. The two approaches used for metatranscriptomic analyses are shown: (A) direct annotation of short reads and differential expression analysis; (B) assembly of short reads into longer contigs, subsequent annotation, and differential expression analysis.
Direct Annotation of Unassembled Metatranscriptomic Sequences
Despite mRNA enrichment, the remaining rRNA sequences were removed prior to BLASTX analyses of the filtered sequence reads. Ribosomal RNA removal was performed using the SortMeRNA software with the eight default rRNA databases included in the software package, covering the small (16S/18S), large (23S/28S), and 5/5.8S ribosomal subunit (Kopylova et al., 2012). The non-rRNA reads and rRNA reads were differentiated using the software with the non-rRNA reads considered to represent mRNA, and paired reads subjected to BLASTX searches (E ≤ 0.02) against the National Centre for Biotechnology Information (NCBI) non-redundant (nr) protein database to obtain the top five BLAST matches per sequence.
The BLAST results were parsed using the lowest common ancestor (LCA) algorithm in MEGAN (version 5.10.2) (Huson et al., 2007) with the default parameters. A comparison file was generated in MEGAN for all 12 samples using absolute read counts. The number of assigned reads per taxa was extracted for different NCBI-based taxonomy levels. For functional annotation, the protein coding sequences were classified using the Clusters of Orthologous Groups of proteins (COGs) database (Tatusov et al., 2000), Kyoto Encyclopaedia of Genes and Genomes (KEGG) database (Kanehisa et al., 2014), and SEED Subsystem database (Overbeek et al., 2014) with the number of assigned reads per functional group extracted for each sample.
Metatranscriptome Assembly and Annotation
Trinity (version 2.2.0) (Haas et al., 2013) was used for de novo metatranscriptome assembly. The combined set of 348,722,194 quality filtered reads from all 12 rhizosphere libraries was combined into a single reference transcriptome assembly. Assembly and contig quality analysis was performed using both Trinity scripts and Transrate (Smith-Unna et al., 2016). The assembly was annotated using Trinotate (Bryant et al., 2017). Transcripts were subjected to a BLASTX search (E ≤ 1e−5) of the protein database Swiss-Prot (Boeckmann et al., 2005) downloaded from UniProt (http://www.uniprot.org/). The software Transdecoder (http://transdecoder.github.io) was used to predict likely coding regions within transcripts, and resulting protein products were subjected to a BLASTP search (E ≤ 1e−5) against the Swiss-Prot database. To identify conserved protein domains we used Hmmer software (http://hmmer.org/) and PFam (Finn et al., 2016). KEGG (Kanehisa et al., 2012), Gene Ontology (GO) (Ashburner et al., 2000), and Eggnog (Powell et al., 2012) annotations were retrieved from Swiss-Prot where transcripts could be assigned to these databases. All results for the reference assembly annotation were parsed by Trinotate, stored in a SQLite database and then reported as a tab-delimited summary file. The proportion of the assembly that represented rRNA reads was determined by aligning contigs to rRNA databases using Sort Me RNA as described above (Kopylova et al., 2012). Reads from each sample were individually mapped back onto the assembly using Bowtie (Langmead et al., 2009) and a count table of reads that align to each transcript produced using RSEM (RNA-Seq by Expectation-Maximization) (Li and Dewey, 2011) within the Trinity software package. Biological replicates were compared for the suppressive and non-suppressive metatranscriptome libraries using a Pearson correlation matrix computed in Trinity (Haas et al., 2013) based on transcript expression values.
Differential Expression Analysis
For the unassembled annotation four count tables were generated in MEGAN for the assigned species, COG, KEGG, and SEED functions (Table S2). For the assembled metatranscriptome analysis one count table of the estimated transcript (isoform) abundance was generated using Trinity (Datasheet 1). All count tables were analysed in edgeR version 3.16.5 (Robinson et al., 2010) to identify significantly differentially expressed (DE) taxa, functional groups or transcripts using a P-value of < 0.05, a false discovery rate (FDR) cut-off of 0.05 and minimum fold change (FC) of 2 for the unassembled analyses and 4 for the assembled analyses. Data from the unassembled annotation in MEGAN were filtered at a count-per-million (CPM) of >100 because unassembled sequences could be aligned to multiple reference genes attributed to a taxon, COG, KEGG, or SEED group, and a taxon or functional group was only retained if it was expressed in at least three of the six replicate samples. For the assembled metatranscriptome, transcript abundance was filtered at a CPM of 0.5 as a “gene” grouping may contain a cluster of multiple transcripts (isoforms), though expression was required in five of the six replicate samples. Normalisation to allow comparison between samples was performed for each count table in edgeR using TMM (trimmed mean of M-values). EdgeR settings included using the generalised linear model (GLM) likelihood ratio test with the contrast option (suppressive minus non-suppressive) (McCarthy et al., 2012). Differentially expressed transcripts from the Trinity assembly were also subject to BLASTX and BLASTN searches of Genbank (E ≤ 1e−5) for comparison to MEGAN analyses.
Results
Rhizoctonia Inoculum and Root Rot Assessment
Soils collected prior to sowing showed similar inoculum levels of the pathogen R. solani AG8 in both the suppressive and non-suppressive fields, as determined by quantitative PCR (pg DNA g−1 soil) (Figure 2A). These inoculum concentrations were considered a high disease risk condition. A build-up of inoculum was observed in both fields throughout the cropping season, in particular during the initial 8 weeks of crop growth. The build-up was greatest however, in the non-suppressive soils compared to that in the suppressive soils, resulting in significantly higher R. solani AG8 DNA in the non-suppressive soils (Figure 2A). These differences aligned with the data for the relative abundance of R. solani AG8 fungal transcripts expressed in the metatranscriptomic libraries from the rhizosphere samples collected at 8 weeks post-sowing (Figure S3).
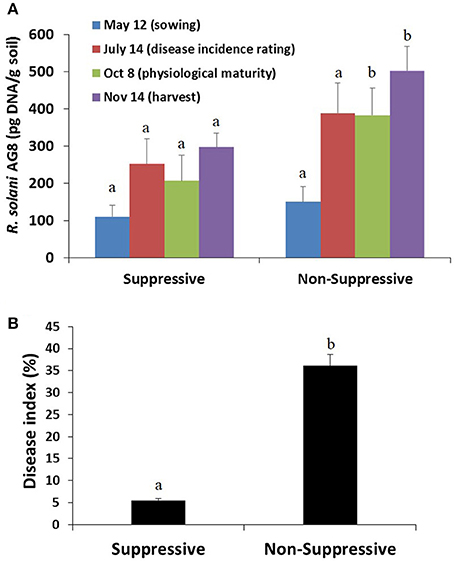
Figure 2. (A) Abundance of inoculum of the plant pathogen R. solani AG8 (pg DNA/g soil) as determined by quantitative PCR on soil samples collected prior to sowing and at different stages of the cropping season, and (B) root disease index (%) as assessed on plant roots sampled at 8 weeks post-sowing. Bars represent average values ± standard error. Values for suppressive and non-suppressive samples from the same sampling time that differed significantly (by paired t-test P < 0.01) are denoted with different letters.
An analysis of root samples from plants collected 8 weeks post-sowing showed very little Rhizoctonia disease incidence in the suppressive field (i.e., <5% infected roots with low level of infection), whereas the disease incidence and severity were significantly higher in plants from the non-suppressive field (i.e., >35% roots with higher level of infection) (Figure 2B). In the non-suppressive field disease incidence and infection of new roots, in particular crown roots, continued throughout the growing season until physiological maturity whereas there was no disease incidence on the crown roots of the crop in the suppressive field. The reduced vigour of Rhizoctonia infected plants, particularly in the non-suppressive field, would have contributed to the difference in grain yield between the two fields at harvest. The grain yield for the 2012 crop was 2.35 ± 0.08 t/ha for the suppressive field and 1.625 ± 0.04 t/ha for the non-suppressive field.
Basic Statistics of Metatranscriptomics Sequence Data
Across the 12 metatranscriptome libraries sequenced, the overall quality score of the raw sequence reads was very high with the majority of the reads averaging Phred scores of Q ≥ 30 (>77% for all samples). The metatranscriptomes generated a total of 200,313,684 and 148,408,510 quality filtered reads for the suppressive and non-suppressive samples respectively (Table 1).
Characterisation of Sequences in the Unassembled Metatranscriptomic Libraries
Reads that aligned to rRNA databases were identified and excluded from further analyses of the unassembled metatranscriptome libraries. The proportion of rRNA reads remaining in the total RNA after rRNA subtraction was greater in the suppressive samples (30–65% of reads) than the non-suppressive samples (22–34% of reads). After filtering to remove rRNA reads, 67,551,136 and 73,540,104 reads for the suppressive and non-suppressive samples respectively were considered as possible protein-coding transcripts (mRNA) and used for BLAST analyses (Table 2). Of the BLASTX processed transcripts, 16,789,984 (25%) from the suppressive samples and 26,693,540 (36%) from the non-suppressive samples could be assigned in MEGAN to a taxonomic rank, and functionally to KEGG, COG, and SEED categories. Rarefaction curves for absolute sequence numbers at species level indicated near-coverage saturation in the 12 samples with no difference based on whether samples originated from suppressive or non-suppressive fields (Figure S2).
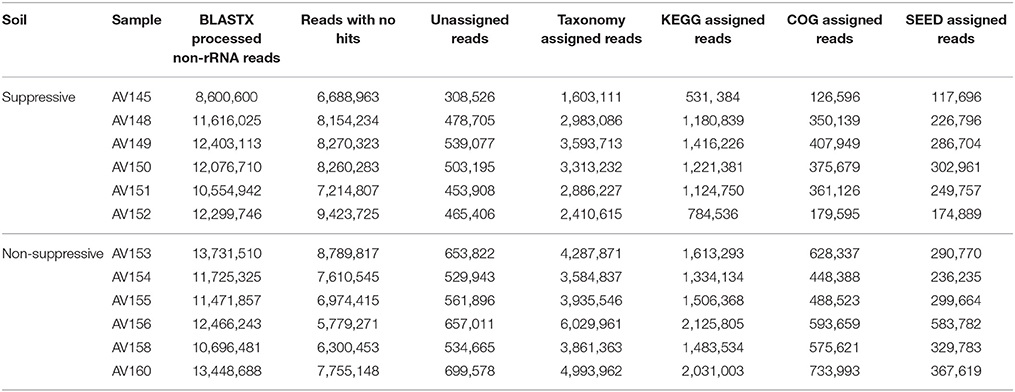
Table 2. Unassembled BLASTX processed non-rRNA reads for each of the 12 metatranscriptome libraries were assigned to taxonomic and functional databases using MEGAN.
Metatranscriptomic sequences were assigned to their closest taxonomic relative in the NCBI taxonomy based on the protein coding regions for bacteria, archaea, and eukaryotes (Table S2). Transcripts of R. solani AG8 were detected for each rhizosphere sample, confirming the presence and activity of the wheat pathogen in the root zone. The R. solani transcripts were most abundant in samples from the non-suppressive field and represented on average 6% of fungal sequences for the non-suppressive samples and 2% for the suppressive samples (Figure S3).
The taxonomic composition of the active rhizosphere community based on mRNA sequences was very similar between the metatranscriptome libraries of the suppressive and non-suppressive samples. The rhizosphere community was primarily bacterial, dominated by the classes Actinobacteria, Alphaproteobacteria, and Gammaproteobacteria. The families Micrococcaceae (Actinobacteria), Pseudomonadaceae, and Enterobacteriaceae (Gammaproteobacteria) accounted for a large proportion of the rhizosphere microbiome and varied in abundance between individual samples (Figure 3). Archaea present in the rhizosphere were predominantly from the family Nitrososphaeraceae (phylum Thaumarchaeota). The dominant fungal phylum in the rhizosphere was Ascomycota, representing on average 72% of all fungal transcripts. Other active fungal phyla were Basidiomycota (10%), which includes the genus Rhizoctonia, and Glomeromycota (4%) which form arbuscular mycorrhizae. All other fungal transcripts belonged to five phyla present at <1% relative abundance or could not be identified below the rank of Kingdom (14%). Fungal families were a much smaller proportion of the total microbial transcripts in the rhizosphere though 164 fungal families were identified (data not shown).
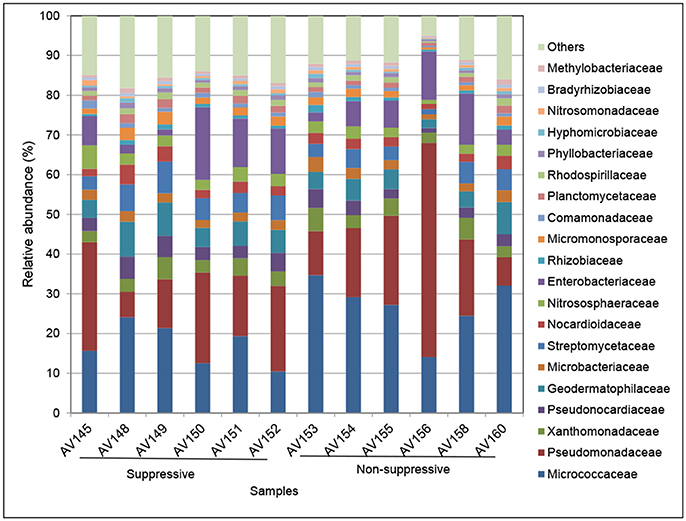
Figure 3. Relative abundance (%) of the major bacterial and archaeal families in metatranscriptomic libraries from rhizosphere samples collected from suppressive (AV145-AV152) and non-suppressive (AV153-AV160) soil. The taxonomic annotation is based on the Genbank non-redundant database and NCBI taxonomy. The category others represents families with a frequency of <1%, which included eukaryote transcripts.
Differential expression analysis at threshold of FC ≥ 2 showed that 65 bacterial species and one environmental archaeal strain differed significantly in their read counts (gene expression) between suppressive and non-suppressive fields (P < 0.05, FDR < 0.05; Figure 4, Table S3). Forty-two bacterial species from the Actinobacteria or Proteobacteria were more abundant (logFC = −1.044 to −5.491) in non-suppressive samples including 23 Arthrobacter species or strains (from NCBI taxonomy), three Pseudomonas spp., a Rhizobium spp., and a Nitrobacter spp. The 25 species more abundant (logFC = 1.050–6.669) in the suppressive samples were from a broad range of phyla including Actinobacteria, Proteobacteria, Firmicutes, Thaumarchaeota, Chloroflexi, and Bacteroidetes. The most abundant species in the suppressive samples based on fold change was Buttiauxella spp. (logFC = 6.669) while Streptomyces spp. were the most common with three species having significantly different expression (P < 0.05, FDR < 0.05, logFC = 1.195–2.249). Unlike the bacterial species, none of the fungal species detected in the metatranscriptome libraries showed significant differential expression between the suppressive and non-suppressive samples.
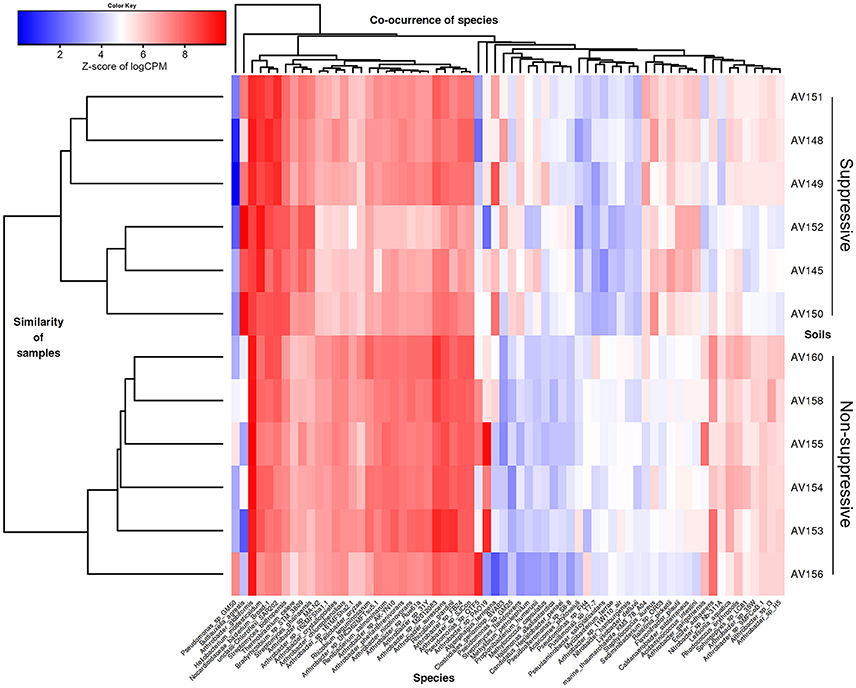
Figure 4. Heatmap showing microbial species with differential gene expression (FDR < 0.05, fold change > 2) for the unassembled metatranscriptomic libraries of suppressive (AV145-AV152) and non-suppressive (AV153-AV160) samples based on counts per million (CPM) sequence reads. Shown are species with the most extreme fold changes: logFC from −5.5 to −1 and 1 to 6.7.
Functional profiling of the unassembled suppressive and non-suppressive libraries was undertaken by comparing the tentative functions assigned in the KEGG, COG, and SEED databases in MEGAN (Table S2). For KEGG analysis, transcripts for suppressive and non-suppressive samples were assigned on average to the dominant pathways of Metabolism (32%), Unclassified KEGGs (31%), Genetic Information (22%), Environmental information Processing (6%), or other categories (9%). The number of unique KEGG orthology (KO) identifiers found for all 12 samples was 10,669.
Differential expression analysis of KEGG functions revealed eight significant KEGG identifiers (P-value and FDR < 0.05, FC ≥ 2; Table 3). Four KO identifiers were more abundant in non-suppressive samples including two ABC transporter proteins (K02077, K02074), putrescine oxidase (K03343) which is an enzyme in the urea cycle and metabolism of amino groups, and glutaredoxin (K06191), an essential component of the glutathione system that reductively detoxify substances such as arsenic and peroxides and are important in the synthesis of DNA. The four KO identifiers more abundant in suppressive samples represented large and small subunit hydrogenases (K06281 and K06282 respectively), a Type VI secretion system secreted protein Hcp (K11903), and a surface adhesion protein (K12549).
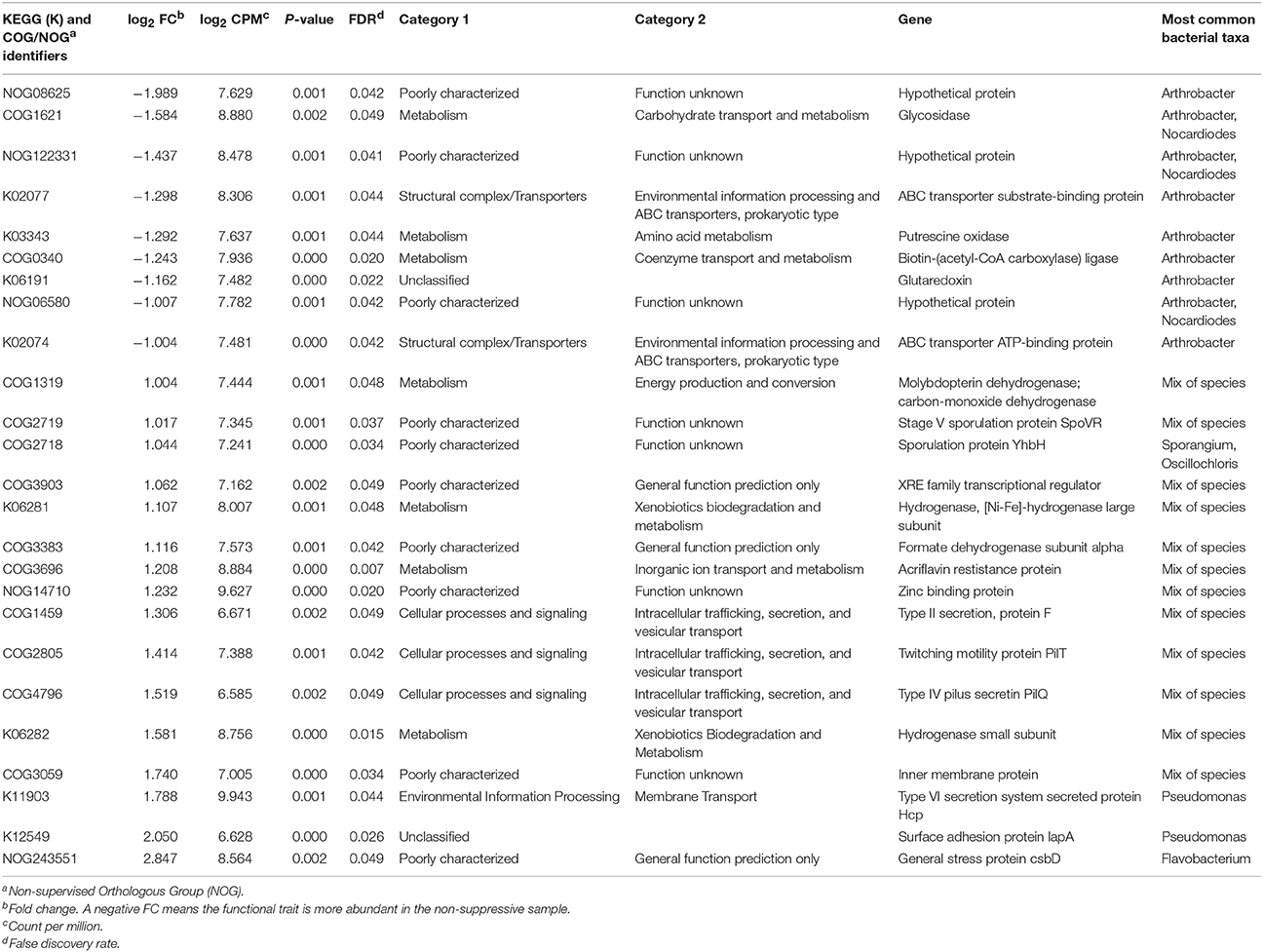
Table 3. Identification of differentially expressed functional traits from the KEGG and COG databases with a fold change >1 in unassembled metatranscriptome libraries of suppressive samples compared to non-suppressive samples.
For COG analysis, transcripts for suppressive and non-suppressive samples were assigned on average to the categories of Metabolism (35%), Information, Storage and Processing (28%), Cellular Processes (17%), and Poorly Categorised COGs (20%). The number of orthologous groups identified from the COG database for all 12 samples was 9,950. Seventeen orthologous groups showed differential expression (P < 0.05, FRD < 0.05, FC ≥ 2) (Table 3). The five COGs with greater transcript counts in the non-suppressive samples were glycosidase (COG1621) which is associated with carbohydrate metabolism, a biotin metabolism gene (COG0340) and three hypothetical proteins (NOG08625, NOG06580, NOG122331). The 12 COGs with greater transcripts counts in the suppressive samples had a variety of functions including sporulation protein SpoVR (COG2719), sporulation protein YhbH (COG2718), Type II secretion protein (COG1459), Type IV pilus secretin PilQ (COG4796), and twitching motility protein PilT (COG2805). Transcripts were assigned to 29 SEED pathways in MEGAN, though none of the SEED identified genes differed significantly in their expression between the suppressive and non-suppressive samples.
Metatranscriptome Assembly, Annotation, and Differential Gene Expression
We co-assembled libraries from 12 samples totalling 174,361,097 100 base paired-end reads. The assembly generated contained 2,092,555 transcript contigs clustered into 1,747,231 “gene” groupings totalling 620.7 Mb. The average transcript length was 296 bases, while 10% (N10) of contigs were 654 bases and 50% (N50) were 278 bases. The median contig length was 248 bases and the longest was 16,460 bases. The E90N50 contig length was 253 bases. Further assembly and contig quality statistics are provided in Table S4. The total number of original reads which mapped back to the assembly was 89% with almost all mapping as properly paired reads (87%). A comparison of biological replicates for the suppressive and non-suppressive libraries showed greater similarity between replicates from the same field than between fields (average Pearson correlation between replicates: 0.5 for suppressive and 0.43 for non-suppressive) and no outliers were identified in a Principal Component Analysis (PCA) (Figure S4). The number of contigs identified as rRNA subunits using Sort Me RNA was 106,746 (5.1%) while 1,985,809 contigs (94.89%) were identified as non-rRNA.
We applied Trinotate to the 2.1 million transcript contigs in our assembly, finding 886,783 transcripts matched 102,982 unique Swiss-Prot proteins, and 12,105 Trinity transcripts matched more than 80% of their length with the best matched protein sequence. The number of annotated transcripts was 960,807 (46%) when the additional databases of EGGNOG, KEGG, PFAM, and GO were considered as well as the Swiss-Prot BLAST annotations. Trinotate reported 9,055 unique KEGG identifiers, and 7,197 unique COG identifiers for the assembly. As with the unassembled MEGAN sequence analysis, transcripts of Rhizoctonia spp. (designated as Thanatephorus spp.) were detected in the metatranscriptome assembly, in this case as eight contigs representing the lignin-degrading enzymes laccase-1 and laccase-3.
For differential expression analysis, unassembled reads were aligned for each sample to the contigs to estimate the expression profiles of all contigs across the suppressive and non-suppressive samples (Data sheet 1). Analysis of the assembly revealed 7217 contigs with differential expression for suppressive and non-suppressive samples (P < 0.05, FRD < 0.05, FC ≥ 4). Of these differentially expressed contigs, 2,676 (37%) were rRNA subunits as they aligned with rRNA databases using SortMeRNA and BLASTN of NCBI nt (E ≤ 1e−5). Based on the BLASTN annotation, 63% of these rRNA contigs were bacterial, 34% were eukaryotic and <1% were archaeal. The majority of the rRNA contigs (2,360; 88%) were more abundant in the suppressive libraries while only 316 contigs (12%) were more abundant in non-suppressive libraries. After the removal of rRNA, 4541 differentially expressed contigs remained of which 2,652 were more abundant in non-suppressive libraries and 1,889 were more abundant in suppressive libraries.
A large number of the differentially expressed contigs could not be functionally annotated with the numerous functional databases used (BLASTX of protein databases NCBI nr and Swiss-Prot, BLASTP of Swiss-Prot, EggNog, KEGG, PFam, and GO) and were either hypothetical proteins or had no hits. Notably the number of these non-functionally annotated contigs was far greater for the suppressive (1,662; 88%) than non-suppressive samples (1,024; 39%). Of the DE contigs that could be functionally annotated 1,628 were more abundant in the non-suppressive libraries and 227 in the suppressive libraries (Table S5).
The taxonomic annotation of the DE contigs was examined using the BLASTX annotation of NCBI nr (as for the unassembled reads in MEGAN). The majority of contigs were bacterial (1499/1855 contigs; 81%) while archaea and eukaryotes represented <1% each (14 contigs) (Table S6). Contigs with greater expression in the non-suppressive libraries were from 76 genera with the most common species or strains being Pseudomonas spp. (593 contigs; 37%), Arthrobacter spp. (419 contigs; 26%), and Stenotrophomonas spp. (83 contigs; 5%) (Figure 5). Contigs with greater expression in the suppressive libraries represented only 30 genera though the frequency of the most common species or strains was much lower than in non-suppressive samples with Stenotrophomonas spp. the most common with 43 contigs (19%), followed by Buttiauxella spp. (33 contigs; 15%) and Pseudomonas spp. (10 contigs; 4%) (Figure 5). Based on BLASTP of Swiss-Prot 3% (62/1,855) of all annotated differentially expressed contigs were found to be viral (Table S5).
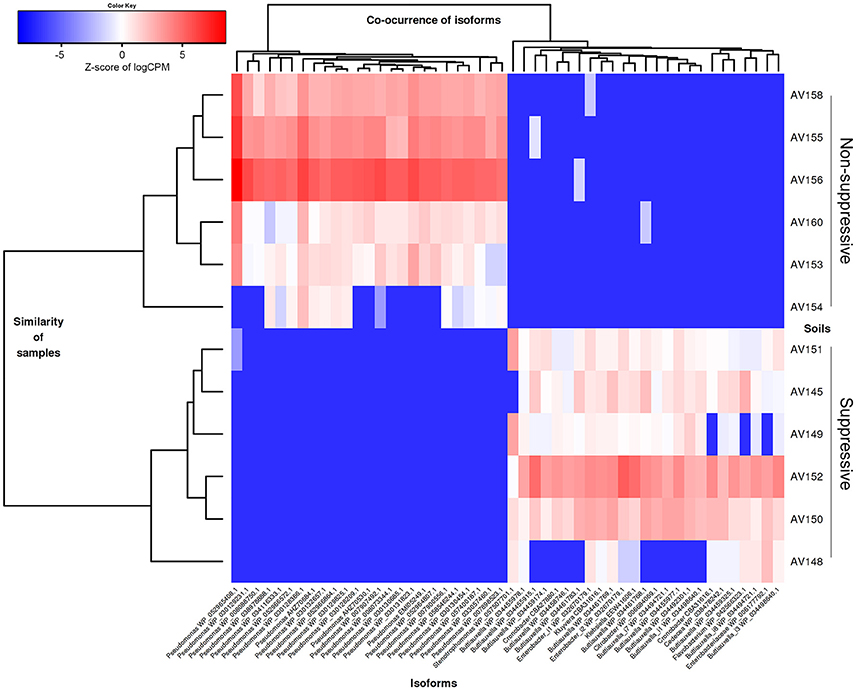
Figure 5. Heatmap showing the abundance of contigs (isoforms) based on their taxonomic annotation with differential gene expression (FDR < 0.05, fold change ≥ 4) for the metatranscriptomic libraries of suppressive (AV145-AV152) and non-suppressive (AV153-AV160) samples, based on counts per million (CPM) sequence reads. Contigs shown have the 25 highest and lowest fold changes (logFC from −15 to −11 and from +11 to +16 and FDR < 1e-08) and were able to be annotated at genus using the NCBI nr data with their Genbank identification number shown.
Functional characterisation of the DE contigs was considered using all database assignments. Of the 227 annotated contigs that were more abundant in the suppressive libraries (Table S5), a large number of contigs were involved in the functions of regulation of DNA transcription and ribosome translation. Genes outside of these functions that were expressed more in the suppressive samples included a polyketide cyclase, a terpenoid biosynthesis backbone gene 1-deoxy-D-xylulose-5-phosphate synthase (dxs), and many stress and adaptation genes with 11 contigs representing cold shock proteins (csp, scoF, capB) (Figure 6). Osmotic stress genes (osmY) were also expressed with two DE contigs being more abundant in suppressive samples and two in non-suppressive samples.
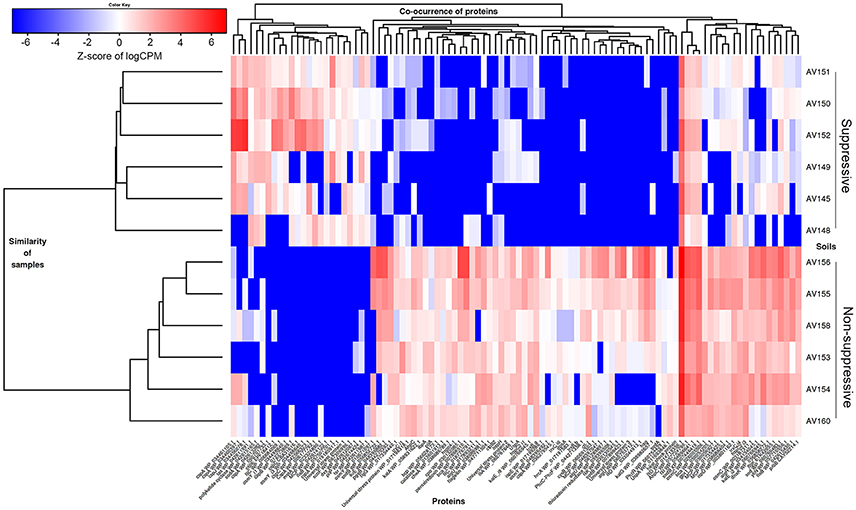
Figure 6. Heatmap showing differential gene expression (FDR < 0.05, fold change ≥ 4) for genes from the metatranscriptomic libraries of suppressive (AV145-AV152) and non-suppressive (AV153-AV160) samples, based on counts per million (CPM) sequence reads. A selection of contigs (isoforms) with rhizosphere related functions is shown annotated by gene name and the NCBI protein reference sequence number.
Functions of the 1,628 annotated DE contigs which were more abundant in the non-suppressive libraries (Table S5) included transcription, translation, carbohydrate metabolism, energy metabolism, lipid metabolism, and amino acid metabolism. Genes that were more abundant in the non-suppressive samples (Figure 6) included genes for the biosynthesis and regulation of antibiotics such as phenazine e.g., phenazine biosynthesis family protein (phzf) and its transcriptional activator protein (phzR), and pyrrolnitrin with non-heme chloroperoxidase (cpo). Also more abundant in the non-suppressive samples were five contigs for superoxide dismutase (sod) genes and 22 contigs representing genes for protection from reactive oxygen species (ROS) and superoxide radicals such as catalase (kat, bca, osmC), peroxiredoxins (ahpC, bcp), glutathione peroxidases (gpx1, proB), and thioredoxin system genes (trx). A large number of DE motility genes were more abundant in the non-suppressive samples than suppressive samples with 13 compared to three contigs for the genes flagellin (fliC, fliA, fliK), flagellar hook proteins (flgK, fliD), flagellar basal-body rod proteins and a fimbrial protein (pilA). Additionally, one contig for alginate biosynthesis genes (alg), which results in swarming motility, and two contigs for biofilm synthesis and regulation genes (pgaB, bssS) were expressed more in non-suppressive samples while the expression of chemotaxis genes (mcp, motA, cheA, tas, tsr) was similar for both suppressive and non-suppressive samples with four DE contigs each. While suppressive samples were characterised by greater abundance of contigs for cold-shock proteins, the non-suppressive samples displayed differential expression for stress response and adaptation with nine heat shock proteins (ibpA, hsp) and associated chaperones (dnaK, clpB), and a nutrient stress gene (dspA). Two contigs for genes in the metabolism of terpenoid backbone synthesis (ispD, ispE) were also expressed more in non-suppressive samples, forming a different part of the MEP/DOXP pathway to the gene expressed more in the suppressive sample (dxs). Interestingly, we also found a plant defence protein, chalcone synthase (chs), a type III polyketide synthase that was more abundant in the non-suppressive samples.
Soil Physico-Chemical Properties
Soil physico-chemical properties were measured for 16 bulk soil samples collected in the two fields (Table S1). The two fields were characterised by very similar soil textures with 7–11% clay, 8–12% silt, and 76–83% sand. Both soils were pH (water) 8.6. The suppressive soil had nearly twice the organic carbon (1.63%), total nitrogen (0.1%), and total P (0.04%) content of the non-suppressive field (0.94, 0.06, and 0.02% respectively).
Discussion
Disease suppression of the cereal crop pathogen R. solani AG8 is regulated by resident soil microorganisms and may assist in the development of a complementary strategy for minimising damage to wheat crops. Central to this biocontrol strategy is the need to identify the mechanisms of suppression and microbes that mediate these functions. This study builds on the knowledge of disease suppression of R. solani AG8 at a long-term cereal cropping site at Avon, South Australia (Roget, 1995; Roget et al., 1996; Barnett et al., 2006; Gupta et al., 2011; Donn et al., 2014; Penton et al., 2014) using an approach that identifies the active functions of the suppressive and non-suppressive rhizosphere microbiome. The detection of DNA (by qPCR) and transcripts of R. solani in the rhizosphere of healthy wheat roots of the suppressive field at Avon supports the concept of disease suppression where the pathogen may be present but does not cause significant disease (Baker and Cook, 1974). Differential expression analyses revealed that suppressive rhizosphere soils contained more polyketide and cold-shock stress genes while non-suppressive rhizosphere soils expressed many different oxidative stress genes such as superoxide dismutase and peroxidases, as well as flagella and antibiotic biosynthesis genes for phenazine and pyrrolnitrin. Our study of a suppressive soil is the first to use the metatranscriptomic approach in a field crop.
Many of the active taxa in the metatranscriptomes were common to both the suppressive and non-suppressive rhizosphere soils (Figure 3). Of the taxa with differential expression, there were differences in the dominant genera and diversity of taxa between the two soils, based on the taxonomic affiliation with the NCBI nr database (Figures 4, 5). The active rhizosphere of non-suppressive soil was dominated by Pseudomonas spp. (Gamma-Proteobacteria) and Arthrobacter spp. (Actinobacteria). Pseudomonas spp. are highly prevalent rhizosphere bacteria (Doornbos et al., 2011) and are associated with plant growth promotion and disease suppression (Stutz et al., 1986; Weller et al., 2002, 2007; Raaijmakers et al., 2009). Our observation that Pseudomonas spp. transcripts are more abundant in the rhizosphere of non-suppressive soils agrees with reports of higher prevalence of Pseudomonas spp. in soils with greater take-all disease severity compared to suppressive soil (Sanguin et al., 2009), in soils within R. solani AG8 disease patches compared to outside patches (Donn et al., 2014), and on the surface of necrotic wheat roots infected with Ggt or R. solani compared to healthy roots (Chapon et al., 2002; De Souza et al., 2003). The increased the abundance of active Pseudomonas spp. observed in the non-suppressive field at Avon may be associated with the premature senescence of R. solani diseased roots in that field and the consequent nutrient release in the rhizosphere.
Arthrobacter spp. transcripts were also co-dominant in the rhizosphere of the non-suppressive soil compared to the suppressive soil. This genus is known for its plant growth promoting traits (Cacciari et al., 1971; Banerjee et al., 2010; Fernández-González et al., 2017), including antagonistic activity against plant pathogens such as Fusarium spp., Pythium spp., and Verticillium dahliae (Mohamed et al., 2017). Arthrobacter are a common soil bacterium with both oligotrophic and copiotrophic lifestyles (Fierer et al., 2007; Ho et al., 2017) and are known to occur in the rhizosphere of wheat (Landa et al., 2003; Lenc et al., 2015). The greater abundance of Arthrobacter spp. in the non-suppressive rhizosphere may reflect their ability to degrade plant cell wall components including cellulose, pectin and xylan (Fernández-González et al., 2017) as well as metabolising glucose and mannose (Fernández-González et al., 2017) which are common wheat root exudates (Baetz and Martinoia, 2014). The increased abundance of carbohydrate and amino acid metabolism genes in the non-suppressive samples supports the theory that Arthrobacter spp. may be utilising recalcitrant carbon substances from R. solani damaged roots, and along with Pseudomonas spp. be acting saprophytically to assimilate exudates from diseased roots.
To identify biological functional patterns that differed between the suppressive and non-suppressive soils and may be related to disease suppression we used differential gene expression analysis. Unique to this study were the large number of genes expressed in the non-suppressive samples in defence against oxidative stress (Figure 6). Expressed mostly by Pseudomonas spp. and Arthrobacter spp., were genes encoding superoxide dismutase which detoxifies superoxides, catalases and peroxidases which detoxify hydrogen peroxide (Levy et al., 1992) and thioredoxin system genes which donate electrons to peroxiredoxins to remove ROS and nitrogen species with a fast reaction rate (Lu and Holmgren, 2014; Figure 6). ROS have multiple roles in plant-pathogen interactions e.g., they may function in defence in a host plant through their direct toxicity to pathogens or the activation of various metabolic pathways, while necrotrophic pathogens such as R. solani can use oxidative processes during their attack and invasion of plant tissues (Waśkiewicz et al., 2014). The production of ROS in wheat roots infected by R. solani AG8 was reported by Foley et al. (2016), though they were unable to distinguish in the interaction whether the ROS originated from the pathogen or the host as both can produce and detoxify ROS. Interestingly, our study showed an overwhelming induction of ROS detoxifying genes (sod, trx, kat, gpx, bca, and bcp) occurred in the rhizosphere bacteria of the non-suppressive samples. Bacteria in nature are continuously challenged by toxic oxygen metabolites. Pseudomonas and Arthrobacter spp. may dominate in the non-suppressive rhizosphere under conditions of R. solani infection because they can survive and function by producing protective ROS detoxifying enzymes (Levy et al., 1992).
One of the most well characterised mechanisms of disease suppression is antagonism by the production of antibiotics (Raaijmakers and Weller, 1998; Weller et al., 2002; Ramette et al., 2006; Raaijmakers et al., 2009; Kinkel et al., 2012). Given this, we expected that any antibiotic genes detected in our metatranscriptome analyses would be associated with the suppressive samples. Instead we found three antibiotic genes from Pseudomonas spp. expressed more in the non-suppressive samples (Figure 6). These genes were for the production and regulation of phenazine (phzf, phzR) and pyrrolnitrin (cpo) and were 17–1,220 fold greater in the non-suppressive samples (Table S5). As we only sampled once in the cropping cycle for our metatranscriptome analysis we cannot exclude these antibiotics as having a role in R. solani suppression. The expression of phenazine and pyrrolnitrin genes by Pseudomonas spp. in the non-suppressive rhizosphere in this study could be part of a defence strategy to mediate competition between the highly abundant Pseudomonas and Arthrobacter species rather than as a defence against R. solani (Kinkel et al., 2012). The expression of antibiotic genes and oxidative stress may also be interlinked in Pseudomonas spp. as the mode of action of phenazine is the accumulation of hydrogen peroxide and superoxide (Mousa and Raizada, 2015), thus oxidative stress genes might be expressed endogenously.
Motility transcripts were another functional group with differential expression between the two rhizosphere soils, with 13 transcripts for flagella genes and an alginate biosynthesis gene expressed more in the non-suppressive samples compared to three flagella genes in the suppressive samples. The majority of the flagella genes were expressed by Pseudomonas spp. in the non-suppressive soil, and thus probably involved in colonisation of the rhizosphere. This process requires flagella-dependent motility for migration into the root zone, followed by bacterial biofilm formation (Mauchline et al., 2015).
In the suppressive soil the dominant differentially expressed taxa were Stenotrophomonas spp. (for assembled analyses only) and Buttiauxella spp, both Gamma-Proteobacteria, as well as diverse taxa including Pseudomonas and Streptomyces spp. (Actinobacteria) (Figures 4, 5). Stenotrophomonas spp. transcripts were the most abundant representing 19 and 5% of the contigs with greater differential expression in the suppressive and non-suppressive samples respectively. Stenotrophomonas spp. are of great interest because of their plant growth promotion traits and use as biocontrol agents (Berg et al., 2010; Schmidt et al., 2012). Stenotrophomonas maltophilia is commonly found in the rhizosphere of many plants (including wheat) or as endophytes (Berg et al., 1999) and has been shown in vitro to produce antifungal xanthobaccins and the macrocyclic lactam antibiotic maltophilin (Berg et al., 1996; Ryan et al., 2009). Furthermore S. maltophilia has also been shown to inhibit P. ultimum, both in vitro and in soil microcosms, by producing chitinase and protease (Dunne et al., 1997). Both S. maltophilia and Stenotrophomonas rhizophila can also produce volatile organic compounds (VOCs) which inhibit mycelial growth of R. solani on cabbage (Kai et al., 2007). S. maltophilia was found to be more abundant in the rhizosphere of soil suppressive of R. solani on sugar beet (Mendes et al., 2013), which aligns with our finding of greater expression of Stenotrophomonas spp. in soils suppressive of R. solani AG8. The Stenotrophomonas spp. contigs that were significantly more abundant in suppressive rhizosphere soil were identified as genes for chemotaxis, flagellin, fimbrial protein, polyketide cyclase, superoxide dismutase, and outer membrane proteins.
Buttiauxella was another dominant genus with greater differential expression in the suppressive rhizosphere (4 and 15% of suppressive soil DE genes for unassembled and assembled analyses respectively). Expressed genes of this genus were characterised by fold changes of up to 100 and 650 in the unassembled and assembled analyses respectively. Buttiauxella spp. have been isolated from soil and fresh water (Kämpfer, 2015) though little is known of their role in soil. Antimicrobial activity has been demonstrated for a glycolipid extracted and identified from a soil Buttiauxella sp, however the biosynthetic pathway of the glycolipid is currently unknown (Marzban et al., 2016). The dominance of Buttiauxella spp. in the suppressive rhizosphere may also be related to carbon substrate preferences. Goldfarb et al. (2011) showed that Buttiauxella warmboldiae str. DSM 9404 preferentially used the amino acid glycine as a labile carbon source for growth in soil microcosms and glycine is exuded by healthy wheat roots (Warren et al., 2016). The substantial difference in expression of genes of Buttiauxella spp between suppressive and non-suppressive rhizosphere soil requires further investigation.
Streptomyces spp. comprised a small proportion of the transcripts with greater abundance in suppressive samples (12% for unassembled analyses). This genus has been well documented as agents of disease suppression, biocontrol, plant growth promotion, and broad-spectrum antibiotic production (Liu et al., 1996; Weller et al., 2002; Bakker et al., 2010; Kinkel et al., 2012; Govindasamy et al., 2013; Schlatter et al., 2017). A small number of transcripts from Pseudomonas spp. were also differentially expressed more in the suppressive samples (4% for assembled and unassembled analyses), though these represented contigs from different gene families to those expressed in the non-suppressive samples.
To date unassembled read-based analyses are more frequently used for soil or rhizosphere functional metatranscriptome studies (Stewart et al., 2011; Damon et al., 2012; de Menezes et al., 2012; Nacke et al., 2014; Tveit et al., 2014; Yergeau et al., 2014; Chapelle et al., 2015; Hultman et al., 2015; Kim and Liesack, 2015; Malik et al., 2016; Newman et al., 2016; Masuda et al., 2017) than metatranscriptome assembly (Holmes et al., 2017), possibly because they are simpler to use. Our study identified that for the metatranscriptomes of suppressive samples the interpretation of differentially expressed genes was very different depending on whether the analyses were undertaken on unassembled or assembled reads. For the unassembled analyses, genes that were more abundant in the suppressive samples compared to the non-suppressive samples included the surface adhesion protein lapA, twitching motility protein pilT, and type II, IV and VI secretion proteins (secretion protein F, pilQ, Hcp, respectively) (Table 3). Type II secretion systems are involved in surface adhesion, colonisation, biofilm formation, genetic material uptake and virulence in mammalian and bacterial plant pathogens such as Ralstonia solanacearum, Xylella fastidiosa, and Xanthomonas spp. (Burdman et al., 2011) while Type VI secretion proteins have been shown to contribute to pathogenicity in many bacteria (Zhou et al., 2012). A potential association between secretion systems and disease suppression has been demonstrated for Pseudomonas fluorescens Pf29Arp and take-all disease (Marchi et al., 2013). The bacterium reduced the severity of take-all disease, though genome analysis revealed it lacked antibiotic synthesis gene clusters and expressed different patterns for type III and type VI secretion systems on healthy and Ggt diseased wheat roots (Marchi et al., 2013).
In the assembled analyses, differentially expressed genes that were more abundant in suppressive samples included a polyketide cyclase gene, a terpenoid biosynthesis gene (dxs), as well as cold shock (csp, scoF, capB), universal (Usp), and osmotic (osmY) stress proteins (Figure 6), however only 12% of these DE genes could be annotated, indicating there is still much we don't know about the active functions of the suppressive soil microbiome. Suppressive samples expressed more genes involved in the regulation of DNA transcription and ribosome translation than non-suppressive samples, as well as cold shock genes which have been implicated as transcription and translation factors (Pearson et al., 2015). Terpenoid transcripts were differentially expressed in the two rhizosphere soils though they represented different parts of the KEGG terpenoid backbone biosynthetic pathway (data not shown). Polyketides and terpenoids are both antimicrobial secondary metabolites (Mousa and Raizada, 2015), with the antibiotic polyketides DAPG and pyoluteorin known to be associated with disease suppression (Mousa and Raizada, 2015). Given the ability of Stenotrophomonas spp. to produce antibiotics and secondary metabolites such as VOC, both the genus and the polyketide cyclase gene expressed in our study provide good targets for further investigation of R. solani AG8 suppression mechanisms.
Garoutte et al. (2016) described an in-depth comparison of bioinformatic analysis approaches for a soil metatranscriptome using assembled and unassembled protocols though they primarily focussed on annotation and did not have replicates to test how annotation methods affect differential gene expression. An evaluation of the two bioinformatic approaches used in this study to characterise and compare the rhizosphere metatranscriptomes of suppressive and non-suppressive soils revealed that each approach had different advantages or disadvantages regarding annotation and differential expression analysis. The first approach of using unassembled reads in MEGAN was most beneficial in providing a visual and numerical comparison of the metatranscriptome annotations of individual samples, though functional annotations were limited to the built-in databases in the software. A disadvantage of the unassembled analysis was the computationally intense and time consuming BLAST processing required for sample annotation, though this issue has been addressed by the development of the software DIAMOND (Buchfink et al., 2015). Gene annotation for the unassembled analysis was possible for only 25 and 36% of transcripts for the suppressive and non-suppressive samples respectively. In comparison, our second approach of co-assembling the 12 metatranscriptomes into a single assembly allowed more databases to be used in annotation and resulted in 46% of contigs being annotated.
The assembly approach proved to be most advantageous when it came to discriminating differentially expressed genes in the suppressive and non-suppressive samples. The assembly-based analysis is contig-centric and resulted in 7217 DE contigs (FC ≥ 4), while the unassembled read-based approach is gene-centric and resulted in only 25 DE KEGG and COG identifiers at lower stringency (FC ≥ 2). Two explanations exist for this difference. Firstly, in the assembled analysis the statistical tests are done independently of the annotation while in the unassembled analysis only counts of annotated genes and taxa are used. Secondly, gene counts per sample are generally higher when obtained through MEGAN compared to mapping samples back to contigs. This is because a gene binned by MEGAN as an individual KEGG or COG identifier may represent multiple Genbank reference genes with different alignments along that gene. On the basis of our findings, where differential gene expression analysis of soil or rhizosphere metatranscriptomes is to be performed we recommend using a de novo assembly approach so that DE analysis is independent of the annotation, and the use of six or more replicate samples to improve the similarity of biological replicate metatranscriptomes for statistical analyses.
In a recent review of the soil microbiome of disease suppressive soils, Schlatter et al. (2017) suggested that for Rhizoctonia suppression, a unified theory for the mechanism responsible remains elusive. In a study of the rhizosphere metatranscriptome of sugar beet seedlings in a R. solani AG2-2IIIB suppressive soil inoculated with R. solani, Chapelle et al. (2015) found increased expression of oxidative stress genes and ppGpp metabolism by rhizosphere bacteria. Our field-based experimental design differed from this pot trial (Chapelle et al., 2015) and instead found evidence of multiple oxidative stress genes for the detoxification of ROS and superoxide radicals expressed in the rhizosphere of plants collected from a non-suppressive field, where symptoms of Rhizoctonia bare patch disease were present (Figure S1). In view of the complexity of gene functions uncovered in metatranscriptomic analyses and that Rhizoctonia infection of cereal crops occurs over long periods, i.e., seedling to physiological maturity (Gupta et al., 2012), we highlight the need for future targeted and temporally-based approaches to further investigate the role of specific differentially expressed microbial taxa and genes e.g., polyketide cyclases, that may be potential key mechanisms for the suppression of Rhizoctonia root rot disease. In summary, this field-based metatranscriptomic study has characterised the active functions and taxonomy of the rhizosphere microbiome in soils which are suppressive and non-suppressive for R. solani AG8.
Author Contributions
HH: Obtained funding for the study, conceived and designed the experiments, conducted field sampling, carried out the bioinformatic analyses of the metatranscriptomes and wrote the paper; JW: Carried out the RNA extractions and metatranscriptome library preparations; KS: Conducted the differential expression analyses in edgeR, NCBI BLAST analyses and edited the paper; VG: Contributed to the design of the experiments, conducted field sampling, analysis of the qPCR and disease assessment data, and contributed to the manuscript preparation; PM: Contributed to manuscript writing and editing.
Funding
This work was financially supported by the Grains Research and Development Corporation (Soil Biology Initiative-II) (Project DAV00105) and Agriculture Victoria Research, Department of Economic Development, Jobs, Transport and Resources.
Conflict of Interest Statement
The authors declare that the research was conducted in the absence of any commercial or financial relationships that could be construed as a potential conflict of interest.
Acknowledgments
We thank Robin Manley who owns the farm at Avon and Lloyd Mudge who farms the site for their support, cooperation, and access to the site. We are grateful to Vilnis Ezernieks and Claire Allan of DEDJTR, Valquiria Fortunato, Li Jing, and CSIRO staff for their assistance in multiple sampling field trips to Avon. We thank Alexis Marshall for her assistance in field trips, differential expression analyses, and manuscript editing. Dr Traci Webster processed our samples on the HiSeq 2000. Nick Evans and Andrew Mather provided support in bioinformatics. Dr. Carolyn Bath assisted in manuscript revisions.
Supplementary Material
The Supplementary Material for this article can be found online at: https://www.frontiersin.org/articles/10.3389/fmicb.2018.00859/full#supplementary-material
References
Alabouvette, C. (1986). Fusarium-wilt suppressive soils from the Chateaurenard region: review of a 10-year study. Agronomie 6, 273–284. doi: 10.1051/agro:19860307
Almasudy, A. M., You, M. P., and Barbetti, M. J. (2015). Influence of fungicidal seed treatments and soil type on severity of root disease caused by Rhizoctonia solani AG-8 on wheat. Crop Prot. 75, 40–45. doi: 10.1016/j.cropro.2015.05.007
Anees, M., Tronsmo, A., Edel-Hermann, V., Gautheron, N., Faloya, V., and Steinberg, C. (2010). Biotic changes in relation to local decrease in soil conduciveness to disease caused by Rhizoctonia solani. Eur. J. Plant Pathol. 126, 29–41. doi: 10.1007/s10658-009-9517-0
Ashburner, M., Ball, C. A., Blake, J. A., Botstein, D., Butler, H., Cherry, J. M., et al. (2000). Gene ontology: tool for the unification of biology. The gene ontology consortium. Nat. Genet. 25, 25–29. doi: 10.1038/75556
Baetz, U., and Martinoia, E. (2014). Root exudates: the hidden part of plant defense. Trends Plant Sci. 19, 90–98. doi: 10.1016/j.tplants.2013.11.006
Baker, K., and Cook, R. J. (1974). Biological Control of Plant Pathogens. New York, NY: WH Freeman and Company.
Bakker, M., Glover, J., Mai, J., and Ll, K. (2010). Plant community effects on the diversity and pathogen suppressive activity of soil Streptomycetes. Appl. Soil Ecol. 46, 35–42. doi: 10.1016/j.apsoil.2010.06.003
Banerjee, S., Palit, R., Sengupta, C., and Standing, D. (2010). Stress induced phosphate solubilization by Arthrobacter sp. and Bacillus sp. isolated from tomato rhizosphere. Aust. J. Crop Sci. 4, 378–383.
Barnett, S. J., Roget, D. K., and Ryder, M. H. (2006). Suppression of Rhizoctonia solani AG-8 induced disease on wheat by the interaction between Pantoea, Exiguobacterium, and Microbacteria. Aust. J. Soil Res. 44, 331–342. doi: 10.1071/SR05113
Berg, G., Egamberdieva, D., Lugtenberg, B., and Hagemann, M. (2010). “Symbiotic plant-microbe interactions: stress protection, plant growth promotion and biocontrol by Stenotrophomonas,” in Symbioses and Stress: Joint Ventures in Biology, 1st Edn., Vol. 17, Cellular Origin, Life in Extreme Habitats and Astrobiology (Dordrecht: Springer), 445–460. doi: 10.1007/978-90-481-9449-0_22
Berg, G., Marten, P., and Ballin, G. (1996). Stenotrophomonas maltophilia in the rhizosphere of oilseed rape — occurrence, characterization and interaction with phytopathogenic fungi. Microbiol. Res. 151, 19–27. doi: 10.1016/S0944-5013(96)80051-6
Berg, G., Roskot, N., and Smalla, K. (1999). Genotypic and phenotypic relationships between clinical and environmental isolates of Stenotrophomonas maltophilia. J. Clin. Microbiol. 37, 3594–3600.
Boeckmann, B., Blatter, M.-C., Famiglietti, L., Hinz, U., Lane, L., Roechert, B., et al. (2005). Protein variety and functional diversity: swiss-prot annotation in its biological context. C. R. Biol. 328, 882–899. doi: 10.1016/j.crvi.2005.06.001
Bolger, A. M., Lohse, M., and Usadel, B. (2014). Trimmomatic: a flexible trimmer for Illumina sequence data. Bioinformatics 30, 2114–2120. doi: 10.1093/bioinformatics/btu170
Bowen, G. D., and Rovira, A. D. (1999). “The rhizosphere and its management to improve plant growth,” in Advances in Agronomy, Vol. 66, ed D. L. Sparks (Academic Press), 1–102. doi: 10.1016/S0065-2113(08)60425-3
Bryant, D. M., Johnson, K., Ditommaso, T., Tickle, T., Couger, M. B., Payzin-Dogru, D., et al. (2017). A tissue-mapped axolotl de novo transcriptome enables identification of limb regeneration factors. Cell Rep. 18, 762–776. doi: 10.1016/j.celrep.2016.12.063
Buchfink, B., Xie, C., and Huson, D. H. (2015). Fast and sensitive protein alignment using DIAMOND. Nat. Meth. 12, 59–60. doi: 10.1038/nmeth.3176
Burdman, S., Bahar, O., Parker, J. K., and De La Fuente, L. (2011). Involvement of type IV pili in pathogenicity of plant pathogenic bacteria. Genes 2, 706–735. doi: 10.3390/genes2040706
Cacciari, I., Giovannozzi-Sermanni, G., Grappelli, A., and Lippi, D. (1971). Nitrogen fixation by Arthrobacter sp. I. Taxonomic study and evidence of nitrogenase activity of two new strains. Ann. Microbiol. Enzimol. 21, 97–105.
Cha, J.-Y., Han, S., Hong, H.-J., Cho, H., Kim, D., Kwon, Y., et al. (2015). Microbial and biochemical basis of a Fusarium wilt-suppressive soil. ISME J. 10, 119–129. doi: 10.1038/ismej.2015.95
Chapelle, E., Mendes, R., Bakker, P. A. H. M., and Raaijmakers, J. M. (2015). Fungal invasion of the rhizosphere microbiome. ISME J. 10, 265–268. doi: 10.1038/ismej.2015.82
Chapon, A., Guillerm, A. Y., Delalande, L., Lebreton, L., and Sarniguet, A. (2002). Dominant colonisation of wheat roots by Pseudomonas fluorescens Pf29A and selection of the indigenous microflora in the presence of the take-all fungus. Eur. J. Plant Pathol. 108, 449–459. doi: 10.1023/A:1016099707119
Cook, R. J. (2006). Toward cropping systems that enhance productivity and sustainability. Proc. Natl. Acad. Sci. U.S.A. 103, 18389–18394. doi: 10.1073/pnas.0605946103
Cook, R. J., and Baker, K. F. (1983). The Nature and Practice of Biological Control of Plant Pathogens. Saint Paul, MN: American Phytopathology Society.
Cook, R. J., Thomashow, L. S., Weller, D. M., Fujimoto, D., Mazzola, M., Bangera, G., et al. (1995). Molecular mechanisms of defense by rhizobacteria against root disease. Proc. Natl. Acad. Sci. U.S.A. 92, 4197–4201. doi: 10.1073/pnas.92.10.4197
Damon, C., Lehembre, F., Oger-Desfeux, C., Luis, P., Ranger, J., Fraissinet-Tachet, L., et al. (2012). Metatranscriptomics reveals the diversity of genes expressed by eukaryotes in forest soils. PLoS ONE 7:e28967. doi: 10.1371/journal.pone.0028967
de Menezes, A., Clipson, N., and Doyle, E. (2012). Comparative metatranscriptomics reveals widespread community responses during phenanthrene degradation in soil. Environ. Microbiol. 14, 2577–2588. doi: 10.1111/j.1462-2920.2012.02781.x
De Souza, J. T., Weller, D. M., and Raaijmakers, J. M. (2003). Frequency, diversity, and activity of 2,4-diacetylphloroglucinol-producing fluorescent Pseudomonas spp. in Dutch take-all decline soils. Phytopathology 93, 54–63. doi: 10.1094/PHYTO.2003.93.1.54
Donn, S., Almario, J., Muller, D., Moënne-Loccoz, Y., Gupta, V. V. S. R., Kirkegaard, J. A., et al. (2014). Rhizosphere microbial communities associated with Rhizoctonia damage at the field and disease patch scale. Appl. Soil Ecol. 78, 37–47. doi: 10.1016/j.apsoil.2014.02.001
Doornbos, R. F., Loon, L. C., and Bakker, P. A. H. M. (2011). Impact of root exudates and plant defense signaling on bacterial communities in the rhizosphere. A review. Agron. Sustain. Dev. 32, 227–243. doi: 10.1007/s13593-011-0028-y
Dunne, C., Crowley, J. J., Moenne-Locco, Y., Dowling, D. N., De Bruijn, F. J., and O'gara, F. (1997). Biological control of Pythium ultimum by Stenotrophomonas maltophilia W81 is mediated by an extracellular proteolytic activity. Microbiology 143, 3921–3931. doi: 10.1099/00221287-143-12-3921
Fernández-González, A. J., Martínez-Hidalgo, P., Cobo-Díaz, J. F., Villadas, P. J., Martínez-Molina, E., Toro, N., et al. (2017). The rhizosphere microbiome of burned holm-oak: potential role of the genus Arthrobacter in the recovery of burned soils. Sci. Rep. 7:6008. doi: 10.1038/s41598-017-06112-3
Fierer, N., Bradford, M. A., and Jackson, R. B. (2007). Toward an ecological classification of soil bacteria. Ecology 88, 1354–1364. doi: 10.1890/05-1839
Finn, R. D., Coggill, P., Eberhardt, R. Y., Eddy, S. R., Mistry, J., Mitchell, A. L., et al. (2016). The Pfam protein families database: towards a more sustainable future. Nucleic Acids Res. 44, D279–D285. doi: 10.1093/nar/gkv1344
Foley, R. C., Kidd, B. N., Hane, J. K., Anderson, J. P., and Singh, K. B. (2016). Reactive oxygen species play a role in the infection of the necrotrophic fungi, Rhizoctonia solani in wheat. PLoS ONE 11:e0152548. doi: 10.1371/journal.pone.0152548
Garbeva, P., Hordijk, C., Gerards, S., and De Boer, W. (2014). Volatile-mediated interactions between phylogenetically different soil bacteria. Front. Microbiol. 5:289. doi: 10.3389/fmicb.2014.00289
Garoutte, A., Cardenas, E., Tiedje, J., and Howe, A. (2016). Methodologies for probing the metatranscriptome of grassland soil. J. Microbiol. Methods 131, 122–129. doi: 10.1016/j.mimet.2016.10.018
Goldfarb, K. C., Karaoz, U., Hanson, C. A., Santee, C. A., Bradford, M. A., Treseder, K. K., et al. (2011). Differential growth responses of soil bacterial taxa to carbon substrates of varying chemical recalcitrance. Front. Microbiol. 2:94. doi: 10.3389/fmicb.2011.00094
Gómez Expósito, R., De Bruijn, I., Postma, J., and Raaijmakers, J. M. (2017). Current insights into the role of rhizosphere bacteria in disease suppressive soils. Front. Microbiol. 8:2529. doi: 10.3389/fmicb.2017.02529
Govindasamy, V., Franco, C. M. M., and Gupta, V. V. S. R. (2013). “Endophytic actinobacteria: diversity and ecology,” in Advances in Endophytic Research, eds V. C. Verma and A. C. Gange (Berlin: Springer-Verlag GmbH), 27–59.
Gupta, V. S. R., Rovira, A., and Roget, D. (2011). “Principles and management of soil biological factors for sustainable rainfed farming systems,” in Rainfed Farming Systems, eds P. Tow, I. Cooper, I. Partridge, and C. Birch (Dordrecht: Springer), 149–184.
Gupta, V. V. S. R., Mckay, A., Diallo, S., Smith, D., Cook, A., Kirkegaard, J., et al. (2012). “Rhizoctonia solani AG8 inoculm levels in Australian soils are influenced by crop rotation and summer rainfall,” in 7th Australasian Soilborne Diseases Symposium (Freemantle, WA: Australasian Plant Pathology Society).
Haas, B. J., Papanicolaou, A., Yassour, M., Grabherr, M., Blood, P. D., Bowden, J., et al. (2013). De novo transcript sequence reconstruction from RNA-Seq: reference generation and analysis with Trinity. Nat. Protoc. 8, 1494–1512. doi: 10.1038/nprot.2013.084
Hjort, K., Bergström, M., Adesina, M. F., Jansson, J. K., Smalla, K., and Sjöling, S. (2010). Chitinase genes revealed and compared in bacterial isolates, DNA extracts and a metagenomic library from a phytopathogen-suppressive soil. FEMS Microbiol. Ecol. 71, 197–207. doi: 10.1111/j.1574-6941.2009.00801.x
Ho, A., Di Lonardo, D. P., and Bodelier, P. L. (2017). Revisiting life strategy concepts in environmental microbial ecology. FEMS Microbiol. Ecol. 93:fix006. doi: 10.1093/femsec/fix006
Holmes, D. E., Shrestha, P. M., Walker, D. J. F., Dang, Y., Nevin, K. P., Woodard, T. L., et al. (2017). Metatranscriptomic evidence for direct interspecies electron transfer between Geobacter and Methanothrix species in methanogenic rice paddy soils. Appl. Environ. Microbiol. 83:e00223–17. doi: 10.1128/AEM.00223-17
Hultman, J., Waldrop, M. P., Mackelprang, R., David, M. M., Mcfarland, J., Blazewicz, S. J., et al. (2015). Multi-omics of permafrost, active layer and thermokarst bog soil microbiomes. Nature 521, 208–212. doi: 10.1038/nature14238
Huson, D., Auch, A., Qi, J., and Schuster, S. (2007). MEGAN analysis of metagenomic data. Genome Res. 17, 377–386. doi: 10.1101/gr.5969107
Hynes, H. J. (1933). Purple patch of wheat and oats. A disease caused by the fungus Rhizoctonia solani. Agric. Gazette New South Wales 44, 879–883.
Kai, M., Effmert, U., Berg, G., and Piechulla, B. (2007). Volatiles of bacterial antagonists inhibit mycelial growth of the plant pathogen Rhizoctonia solani. Arch. Microbiol. 187, 351–360. doi: 10.1007/s00203-006-0199-0
Kämpfer, P. (2015). “Buttiauxella,” in Bergey's Manual of Systematics of Archaea and Bacteria (John Wiley & Sons, Ltd). doi: 10.1002/9781118960608.gbm01139
Kanehisa, M., Goto, S., Sato, Y., Furumichi, M., and Tanabe, M. (2012). KEGG for integration and interpretation of large-scale molecular datasets. Nucleic Acids Res. 40, D109–D114. doi: 10.1093/nar/gkr988
Kanehisa, M., Goto, S., Sato, Y., Kawashima, M., Furumichi, M., and Tanabe, M. (2014). Data, information, knowledge and principle: back to metabolism in KEGG. Nucleic Acids Res. 42, D199–D205. doi: 10.1093/nar/gkt1076
Kim, Y., and Liesack, W. (2015). Differential assemblage of functional units in paddy soil microbiomes. PLoS ONE 10:e0122221. doi: 10.1371/journal.pone.0122221
Kinkel, L. L., Schlatter, D. C., Bakker, M. G., and Arenz, B. E. (2012). Streptomyces competition and co-evolution in relation to plant disease suppression. Res. Microbiol. 163, 490–499. doi: 10.1016/j.resmic.2012.07.005
Klein, E., Ofek, M., Katan, J., Minz, D., and Gamliel, A. (2013). Soil suppressiveness to Fusarium disease: shifts in root microbiome associated with reduction of pathogen root colonization. Phytopathology 103, 23–33. doi: 10.1094/PHYTO-12-11-0349
Kopylova, E., Noé, L., and Touzet, H. (2012). SortMeRNA: fast and accurate filtering of ribosomal RNAs in metatranscriptomic data. Bioinformatics 28, 3211–3217. doi: 10.1093/bioinformatics/bts611
Kyselková, M., Almario, J., Kopecký, J., Ságová-Marecková, M., Haurat, J., Muller, D., et al. (2014). Evaluation of rhizobacterial indicators of tobacco black root rot suppressiveness in farmers' fields. Environ. Microbiol. Rep. 6, 346–353. doi: 10.1111/1758-2229.12131
Kyselková, M., Kopecký, J., Frapolli, M., Défago, G., Ságová-Marecková, M., Grundmann, G. L., et al. (2009). Comparison of rhizobacterial community composition in soil suppressive or conducive to tobacco black root rot disease. ISME J. 3, 1127–1138. doi: 10.1038/ismej.2009.61
Landa, B. B., Mavrodi, D. M., Thomashow, L. S., and Weller, D. M. (2003). Interactions between strains of 2,4-diacetylphloroglucinol-producing Pseudomonas fluorescens in the rhizosphere of wheat. Phytopathology 93, 982–994. doi: 10.1094/PHYTO.2003.93.8.982
Langmead, B., Trapnell, C., Pop, M., and Salzberg, S. L. (2009). Ultrafast and memory-efficient alignment of short DNA sequences to the human genome. Genome Biol. 10:R25. doi: 10.1186/gb-2009-10-3-r25
Latz, E., Eisenhauer, N., Rall, B. C., Allan, E., Roscher, C., Scheu, S., et al. (2012). Plant diversity improves protection against soil-borne pathogens by fostering antagonistic bacterial communities. J. Ecol. 100, 597–604. doi: 10.1111/j.1365-2745.2011.01940.x
Lenc, L., Kwaśna, H., Sadowski, C., and Grabowski, A. (2015). Microbiota in wheat roots, rhizosphere and soil in crops grown in organic and other production systems. J. Phytopathol. 163, 245–263. doi: 10.1111/jph.12313
Levy, E., Eyal, Z., Chet, I., and Hochman, A. (1992). Resistance mechanisms of Septoria tritici to antifungal products of Pseudomonas. Physiol. Mol. Plant Pathol. 40, 163–171. doi: 10.1016/0885-5765(92)90057-3
Li, B., and Dewey, C. N. (2011). RSEM: accurate transcript quantification from RNA-Seq data with or without a reference genome. BMC Bioinformatics 12:323. doi: 10.1186/1471-2105-12-323
Liu, D., Anderson, N. A., and Kinkel, L. L. (1996). Selection and characterization of strains of Streptomyces suppressive to the potato scab pathogen. Can. J. Microbiol. 42, 487–502. doi: 10.1139/m96-066
Löbmann, M. T., Vetukuri, R. R., Zinger, L. D., Alsanius, B. W., Grenville-Briggs, L. J., and Walter, A. J. (2016). The occurrence of pathogen suppressive soils in Sweden in relation to soil biota, soil properties, and farming practices. Appl. Soil Ecol. 107, 57–65. doi: 10.1016/j.apsoil.2016.05.011
Lu, J., and Holmgren, A. (2014). The thioredoxin antioxidant system. Free Radic. Biol. Med. 66, 75–87. doi: 10.1016/j.freeradbiomed.2013.07.036
Macnish, G. C. (1983). Rhizoctonia patch in Western Australian Grain Belt. Aust. Plant Pathol. 12, 49–50. doi: 10.1071/APP9830049
Macnish, G. C. (1988). Changes in take-all (Gaeumannomyces graminis var. tritici), Rhizoctonia root rot (Rhizoctonia solani) and soil pH in continuous wheat with annual applications of nitrogenous fertiliser in Western Australia. Aust. J. Exp. Agric. 28, 333–341.
Malik, A. A., Chowdhury, S., Schlager, V., Oliver, A., Puissant, J., Vazquez, P. G. M., et al. (2016). Soil fungal:bacterial ratios are linked to altered carbon cycling. Front. Microbiol. 7:1247. doi: 10.3389/fmicb.2016.01247
Marchi, M., Boutin, M., Gazengel, K., Rispe, C., Gauthier, J.-P., Guillerm-Erckelboudt, A.-Y., et al. (2013). Genomic analysis of the biocontrol strain Pseudomonas fluorescens Pf29Arp with evidence of T3SS and T6SS gene expression on plant roots. Environ. Microbiol. Rep. 5, 393–403. doi: 10.1111/1758-2229.12048
Marzban, A., Ebrahimipour, G., and Danesh, A. (2016). Bioactivity of a novel glycolipid produced by a halophilic Buttiauxella sp. and improving submerged fermentation using a response surface method. Molecules 21:1256. doi: 10.3390/molecules21101256
Masuda, Y., Itoh, H., Shiratori, Y., Isobe, K., Otsuka, S., and Senoo, K. (2017). Predominant but previously-overlooked prokaryotic drivers of reductive nitrogen transformation in paddy soils, revealed by metatranscriptomics. Microbes Environ. 32, 180–183. doi: 10.1264/jsme2.ME16179
Mauchline, T. H., Chedom-Fotso, D., Chandra, G., Samuels, T., Greenaway, N., Backhaus, A., et al. (2015). An analysis of Pseudomonas genomic diversity in take-all infected wheat fields reveals the lasting impact of wheat cultivars on the soil microbiota. Environ. Microbiol. 17, 4764–4778. doi: 10.1111/1462-2920.13038
Mavrodi, O. V., Walter, N., Elateek, S., Taylor, C. G., and Okubara, P. A. (2012). Suppression of Rhizoctonia and Pythium root rot of wheat by new strains of Pseudomonas. Biol. Control 62, 93–102. doi: 10.1016/j.biocontrol.2012.03.013
McCarthy, D. J., Chen, Y., and Smyth, G. K. (2012). Differential expression analysis of multifactor RNA-Seq experiments with respect to biological variation. Nucleic Acids Res. 40, 4288–4297. doi: 10.1093/nar/gks042
Mcdonald, H. J., and Rovira, A. D. (1985). “Development of an inoculation technique for Rhizoctonia solani and its application to screening cereal cultivars for resistance,” in Ecology and Soilborne Plant Pathogens, eds C. A. Parker, A. D. Rovira, K. J. Moore, P. T. Wong, and F. J. Kollmorgen (St. Paul, MA: APS Press), 174–176.
Mckay, A., Bogacki, P., Desbiolles, J., Correll, R., Gupta, V., Hüberli, D., et al. (2014). “Rhizoctonia control improved by liquid banding of fungicides,” in 2014 Grains Research Update for Advisers, ed J. Crane (Adelaide, SA: ORM communications), 245–249.
McSpadden Gardener, B. B., and Weller, D. M. (2001). Changes in populations of rhizosphere of wheat bacteria associated with take-All disease. Appl. Environ. Microbiol. 67, 4414–4425. doi: 10.1128/AEM.67.10.4414-4425.2001
Mendes, R., Garbeva, P., and Raaijmakers, J. M. (2013). The rhizosphere microbiome: significance of plant beneficial, plant pathogenic, and human pathogenic microorganisms. FEMS Microbiol. Rev. 37, 634–663. doi: 10.1111/1574-6976.12028
Mendes, R., Kruijt, M., De Bruijn, I., Dekkers, E., Van Der Voort, M., Schneider, J. H. M., et al. (2011). Deciphering the rhizosphere microbiome for disease-suppressive bacteria. Science 27, 1097–1100. doi: 10.1126/science.1203980
Mohamed, R., Groulx, E., Defilippi, S., Erak, T., Tambong, J. T., Tweddell, R. J., et al. (2017). Physiological and molecular characterization of compost bacteria antagonistic to soil-borne plant pathogens. Can. J. Microbiol. 63, 411–426. doi: 10.1139/cjm-2016-0599
Mousa, W. K., and Raizada, M. N. (2015). Biodiversity of genes encoding anti-microbial traits within plant associated microbes. Front. Plant Sci. 6:231. doi: 10.3389/fpls.2015.00231
Murray, G. M., and Brennan, J. P. (2009). Estimating disease losses to the Australian wheat industry. Aust. Plant Pathol. 38, 558–570. doi: 10.1071/AP09053
Murray, G. M., and Brennan, J. P. (2010). Estimating disease losses to the Australian barley industry. Aust. Plant Pathol. 39, 85–96. doi: 10.1071/AP09064
Nacke, H., Fischer, C., Thürmer, A., Meinicke, P., and Daniel, R. (2014). Land use type significantly affects microbial gene transcription in soil. Microb. Ecol. 67, 919–930. doi: 10.1007/s00248-014-0377-6
Newman, M. M., Lorenz, N., Hoilett, N., Lee, N. R., Dick, R. P., Liles, M. R., et al. (2016). Changes in rhizosphere bacterial gene expression following glyphosate treatment. Sci. Total Environ. 553, 32–41. doi: 10.1016/j.scitotenv.2016.02.078
Ophel-Keller, K., Mckay, A., Hartley, D., and Herdina Curran, J. (2008). Development of a routine DNA-based testing service for soilborne diseases in Australia. Aust. Plant Pathol. 37, 243–253. doi: 10.1071/AP08029
Overbeek, R., Olson, R., Pusch, G. D., Olsen, G. J., Davis, J. J., Disz, T., et al. (2014). The SEED and the Rapid Annotation of microbial genomes using Subsystems Technology (RAST). Nucleic Acids Res. 42, D206–D214. doi: 10.1093/nar/gkt1226
Pankhurst, C. E., Mcdonald, H. J., Hawke, B. G., and Kirkby, C. A. (2002). Effect of tillage and stubble management on chemical and microbiological properties and the development of suppression towards cereal root disease in soils from two sites in NSW, Australia. Soil Biol. Biochem. 34, 833–840. doi: 10.1016/S0038-0717(02)00014-7
Paulitz, T. C., Schroeder, K. L., and Schillinger, W. F. (2010). Soilborne pathogens of cereals in an irrigated cropping system: effects of tillage, residue management, and crop rotation. Plant Dis. 94, 61–68. doi: 10.1094/PDIS-94-1-0061
Pearson, G. A., Lago-Leston, A., Cánovas, F., Cox, C. J., Verret, F., Lasternas, S., et al. (2015). Metatranscriptomes reveal functional variation in diatom communities from the Antarctic Peninsula. ISME J. 9, 2275–2289. doi: 10.1038/ismej.2015.40
Penton, C. R., Gupta, V. V. S. R., Tiedje, J. M., Neate, S. M., Ophel-Keller, K., Gillings, M., et al. (2014). Fungal community structure in disease suppressive soils assessed by 28S LSU gene sequencing. PLoS ONE 9:e93893. doi: 10.1371/journal.pone.0093893
Philippot, L., Raaijmakers, J. M., Lemanceau, P., and Van Der Putten, W. H. (2013). Going back to the roots: the microbial ecology of the rhizosphere. Nat. Rev. Microbiol. 11, 789–799. doi: 10.1038/nrmicro3109
Pieterse, C. M. J., Zamioudis, C., Berendsen, R. L., Weller, D. M., Vanwees, S. C. M., and Bakker, P. A. H. M. (2014). Induced systemic resistance by beneficial microbes. Annu. Rev. Phytopathol. 52, 347–375. doi: 10.1146/annurev-phyto-082712-102340
Powell, S., Szklarczyk, D., Trachana, K., Roth, A., Kuhn, M., Muller, J., et al. (2012). eggNOG v3.0: orthologous groups covering 1133 organisms at 41 different taxonomic ranges. Nucleic Acids Res. 40, D284–D289. doi: 10.1093/nar/gkr1060
Raaijmakers, J. M., Paulitz, T. C., Steinberg, C., Alabouvette, C., and Moënne-Loccoz, Y. (2009). The rhizosphere: a playground and battlefield for soilborne pathogens and beneficial microorganisms. Plant Soil 321, 341–361. doi: 10.1007/s11104-008-9568-6
Raaijmakers, J. M., and Weller, D. (1998). Natural plant protection by 2,4-diacetylphloroglucionl-producing Pseudomonas spp. in take-all decline soils. Mol. Plant Microbe Interact. 11, 144–152. doi: 10.1094/MPMI.1998.11.2.144
Ramette, A., Moënne-Loccoz, Y., and Défago, G. (2006). Genetic diversity and biocontrol potential of fluorescent pseudomonads producing phloroglucinols and hydrogen cyanide from Swiss soils naturally suppressive or conducive to Thielaviopsis basicola-mediated black root rot of tobacco. FEMS Microbiol. Ecol. 55, 369–381. doi: 10.1111/j.1574-6941.2005.00052.x
Robinson, M. D., Mccarthy, D. J., and Smyth, G. K. (2010). edgeR: a Bioconductor package for differential expression analysis of digital gene expression data. Bioinformatics 26, 139–140. doi: 10.1093/bioinformatics/btp616
Roget, D. (1995). Decline in root rot (Rhizoctonia solani AG-8) in wheat in a tillage and rotation experiment at Avon, South Australia. Aust. J. Exp. Agric. 35, 1009–1013. doi: 10.1071/EA9951009
Roget, D. K., Coppi, J. A., and Herdina Gupta, V. V. S. R. (1999). “Assessment of suppression to Rhizoctonia solani in a range of soils across SE Australia,” in First Australasian SoilBorne Diseases Symposium, ed R. C. Magarey (Brisbane, QLD: BSES).
Roget, D. K., Neate, S. M., and Rovira, A. D. (1996). Effect of sowing point design and tillage practice on the incidence of Rhizoctonia root rot, take-all and cereal cyst nematode in wheat and barley. Aust. J. Exp. Agric. 36, 683–693. doi: 10.1071/EA9960683
Romero, D., Traxler, M. F., López, D., and Kolter, R. (2011). Antibiotics as signal molecules. Chem. Rev. 111, 5492–5505. doi: 10.1021/cr2000509
Rovira, A. D. (1986). Influence of crop rotation and tillage on Rhizoctonia bare patch of wheat. Phytopathology 76, 669–673. doi: 10.1094/Phyto-76-669
Ryan, R. P., Monchy, S., Cardinale, M., Taghavi, S., Crossman, L., Avison, M. B., et al. (2009). The versatility and adaptation of bacteria from the genus Stenotrophomonas. Nat. Rev. Microbiol. 7, 514–525 doi: 10.1038/nrmicro2163
Sagova-Mareckova, M., Daniel, O., Omelka, M., Kristufek, V., Divis, J., and Kopecky, J. (2015). Determination of factors associated with natural soil suppressivity to potato common scab. PLoS ONE 10:e0116291. doi: 10.1371/journal.pone.0116291
Sanguin, H., Sarniguet, A., Gazengel, K., Moënne-Loccoz, Y., and Grundmann, G. L. (2009). Rhizosphere bacterial communities associated with disease suppressiveness stages of take-all decline in wheat monoculture. New Phytol. 184, 694–707. doi: 10.1111/j.1469-8137.2009.03010.x
Schillinger, W. F., and Paulitz, T. C. (2006). Reduction of Rhizoctonia bare patch in wheat with barley rotations. Plant Dis. 90, 302–306. doi: 10.1094/PD-90-0302
Schillinger, W. F., and Paulitz, T. C. (2014). Natural suppression of Rhizoctonia bare patch in a long-term no-till cropping systems experiment. Plant Dis. 98, 389–394. doi: 10.1094/PDIS-04-13-0420-RE
Schlatter, D., Kinkel, L., Thomashow, L., Weller, D., and Paulitz, T. (2017). Disease suppressive soils: new insights from the soil microbiome. Phytopathology 107, 1284–1297. doi: 10.1094/PHYTO-03-17-0111-RVW
Schmidt, C. S., Alavi, M., Cardinale, M., Müller, H., and Berg, G. (2012). Stenotrophomonas rhizophila DSM14405T promotes plant growth probably by altering fungal communities in the rhizosphere. Biol. Fertility Soils 48, 947–960. doi: 10.1007/s00374-012-0688-z
Smith-Unna, R., Boursnell, C., Patro, R., Hibberd, J. M., and Kelly, S. (2016). TransRate: reference-free quality assessment of de novo transcriptome assemblies. Genome Res. 26, 1134–1144. doi: 10.1101/gr.196469.115
Stewart, F. J., Sharma, A. K., Bryant, J. A., Eppley, J. M., and Delong, E. F. (2011). Community transcriptomics reveals universal patterns of protein sequence conservation in natural microbial communities. Genome Biol. 12:R26. doi: 10.1186/gb-2011-12-3-r26
Stutz, E., Défago, G., and Kern, H. (1986). Naturally occurring fluorescent pseudomonads involved in suppression of black root rot of tobacco. Phytopathology 76, 181–185. doi: 10.1094/Phyto-76-181
Tatusov, R. L., Galperin, M. Y., Natale, D. A., and Koonin, E. V. (2000). The COG database: a tool for genome-scale analysis of protein functions and evolution. Nucleic Acids Res. 28, 33–36. doi: 10.1093/nar/28.1.33
Tveit, A. T., Urich, T., and Svenning, M. M. (2014). Metatranscriptomic analysis of Arctic peat soil microbiota. Appl. Environ. Microbiol. 80, 5761–5772. doi: 10.1128/AEM.01030-14
Tyc, O., De Jager, V. C. L., Van Den Berg, M., Gerards, S., Janssens, T. K. S., Zaagman, N., et al. (2017). Exploring bacterial interspecific interactions for discovery of novel antimicrobial compounds. Microb. Biotechnol. 10, 910–925. doi: 10.1111/1751-7915.12735
Warren, R. M., Chng, S. F., and Butler, R. C. (2016). Functional characteristics of New Zealand wheat rhizosphere Pseudomonas fluorescens isolates and their potential to inhibit in-vitro growth of Gaeumannomyces graminis var. tritici. N. Z. Plant Prot. 69, 48–56.
Waśkiewicz, A., Morkunas, I., Bednarski, W., Mai, V. C., Formela, M., Beszterda, M., et al. (2014). Deoxynivalenol and oxidative stress indicators in winter wheat inoculated with Fusarium graminearum. Toxins 6, 575–591. doi: 10.3390/toxins6020575
Watanabe, K., Matsui, M., Honjo, H., Becker, J., and Fukui, R. (2011). Effects of soil pH on rhizoctonia damping-off of sugar beet and disease suppression induced by soil amendment with crop residues. Plant Soil 347, 255–268. doi: 10.1007/s11104-011-0843-6
Weller, D. M., Landa, B. B., Mavrodi, O. V., Schroeder, K. L., De La Fuente, L., Blouin Bankhead, S., et al. (2007). Role of 2,4-Diacetylphloroglucinol-producing fluorescent Pseudomonas spp. in the defense of plant roots. Plant Biol. 9, 4–20. doi: 10.1055/s-2006-924473
Weller, D. M., Raaijmakers, J. M., Gardener, B. B., and Thomashow, L. S. (2002). Microbial populations responsible for specific soil suppressiveness to plant pathogens. Annu. Rev. Phytopathol. 40, 309–348. doi: 10.1146/annurev.phyto.40.030402.110010
Wiseman, B. M., Neate, S. M., Keller, K. O., and Smith, S. E. (1996). Suppression of Rhizoctonia solani anastomosis group 8 in Australia and its biological nature. Soil Biol. Biochem. 28, 727–732. doi: 10.1016/0038-0717(95)00178-6
Yergeau, E., Sanschagrin, S., Maynard, C., St-Arnaud, M., and Greer, C. W. (2014). Microbial expression profiles in the rhizosphere of willows depend on soil contamination. ISME J. 8, 344–358. doi: 10.1038/ismej.2013.163
Yin, C., Hulbert, S. H., Schroeder, K. L., Mavrodi, O., Mavrodi, D., Dhingra, A., et al. (2013). Role of bacterial communities in the natural suppression of Rhizoctonia solani bare patch disease of wheat (Triticum aestivum L.). Appl. Environ. Microbiol. 79, 7428–7438. doi: 10.1128/AEM.01610-13
Keywords: disease suppression, Rhizoctonia root rot, metatranscriptome assembly, differential gene expression, soilborne fungus, soil, rhizosphere, microbiome
Citation: Hayden HL, Savin KW, Wadeson J, Gupta VVSR and Mele PM (2018) Comparative Metatranscriptomics of Wheat Rhizosphere Microbiomes in Disease Suppressive and Non-suppressive Soils for Rhizoctonia solani AG8. Front. Microbiol. 9:859. doi: 10.3389/fmicb.2018.00859
Received: 15 December 2017; Accepted: 13 April 2018;
Published: 04 May 2018.
Edited by:
Essaid Ait Barka, Université de Reims Champagne Ardenne, FranceReviewed by:
Rumiana Valcheva Ray, University of Nottingham, United KingdomRichard Allen White III, RAW Molecular Systems (RMS) LLC, United States
Copyright © 2018 Hayden, Savin, Wadeson, Gupta and Mele. This is an open-access article distributed under the terms of the Creative Commons Attribution License (CC BY). The use, distribution or reproduction in other forums is permitted, provided the original author(s) and the copyright owner are credited and that the original publication in this journal is cited, in accordance with accepted academic practice. No use, distribution or reproduction is permitted which does not comply with these terms.
*Correspondence: Helen L. Hayden, aGVsZW4uaGF5ZGVuQGVjb2Rldi52aWMuZ292LmF1