- 1Maryland Institute for Applied Environmental Health, University of Maryland School of Public Health, College Park, MD, United States
- 2Center for Bioinformatics and Computational Biology, University of Maryland, College Park, College Park, MD, United States
- 3Institute for Genome Sciences and Department of Microbiology and Immunology, University of Maryland School of Medicine, Baltimore, MD, United States
Agricultural ponds have a great potential as a means of capture and storage of water for irrigation. However, pond topography (small size, shallow depth) leaves them susceptible to environmental, agricultural, and anthropogenic exposures that may influence microbial dynamics. Therefore, the aim of this project was to characterize the bacterial and viral communities of pond water in the Mid-Atlantic United States with a focus on the late season (October–December), where decreasing temperature and nutrient levels can affect the composition of microbial communities. Ten liters of freshwater from an agricultural pond were sampled monthly, and filtered sequentially through 1 and 0.2 μm filter membranes. Total DNA was then extracted from each filter, and the bacterial communities were characterized using 16S rRNA gene sequencing. The remaining filtrate was chemically concentrated for viruses, DNA-extracted, and shotgun sequenced. Bacterial community profiling showed significant fluctuations over the sampling period, corresponding to changes in the condition of the pond freshwater (e.g., pH, nutrient load). In addition, there were significant differences in the alpha-diversity and core bacterial operational taxonomic units (OTUs) between water fractions filtered through different pore sizes. The viral fraction was dominated by tailed bacteriophage of the order Caudovirales, largely those of the Siphoviridae family. Moreover, while present, genes involved in virulence/antimicrobial resistance were not enriched within the viral fraction during the study period. Instead, the viral functional profile was dominated by phage associated proteins, as well as those related to nucleotide production. Overall, these data suggest that agricultural pond water harbors a diverse core of bacterial and bacteriophage species whose abundance and composition are influenced by environmental variables characteristic of pond topology and the late season.
Introduction
Growing urbanization and climate variability have placed immense pressure on the finite supply of groundwater available for agricultural irrigation. As a result, the exploration of alternative irrigation water sources, including recycled water and pond water, has become a global priority (Scheierling et al., 2006; Parsons et al., 2010; Ortega-Reig et al., 2014). While there is no universal standard, ponds are generally defined as small (1 m2 to ∼50,000 m2), shallow, standing water bodies that can either permanently or temporarily collect freshwater (Biggs et al., 2005; De Meester et al., 2005; Céréghino et al., 2008). These small water bodies are known to house a rich tapestry of aquatic plant and macroinvertebrate species, even greater than that of other larger water bodies (e.g., lakes and rivers) (Biggs et al., 2005). Moreover, the high terrestrial and aquatic interchange of ponds may enable both small free-living (<1 μm) and large/particle-associated bacteria to proliferate (Søndergaard et al., 2005; Roiha et al., 2016). Therefore, assessing the microbial diversity and interactions of these complex water bodies is a critical first step with regard to completing a comprehensive characterization of pond biodiversity, and evaluating the suitability of pond freshwater for agricultural applications, such as the irrigation of food crops.
A growing body of literature has defined several bacterial phyla that are abundant in freshwater and markedly different from that of marine systems (Zwart et al., 2002; Newton et al., 2011). Previous studies have reported a widespread distribution of Actinobacteria (lineage acI and acIV) among various freshwater sites, in some cases composing greater than 50% of the total bacterial community abundance (Glöckner et al., 2000; Newton et al., 2011). The Proteobacteria phylum in freshwater is also abundant, composed largely of Betaproteobacteria. This is in contrast to marine systems where Alphaproteobacteria typically dominates (Newton et al., 2011). Furthermore, aquatic microbial communities are influenced by a variety of seasonal factors, such as pH, temperature, and water retention time (Yannarell et al., 2003; Lindström et al., 2005), as well as top-down regulation from predators (e.g., bacteriophage) (Hennes et al., 1995; Chow et al., 2014). However, the majority of studies on the bacterial community composition of freshwater habitats come from large lakes and rivers, and very few have included an analysis of viral populations.
Bacteriophage, viruses that infect bacteria, are the most abundant biological entities in aquatic systems and play an important role in microbial community composition and ecology (Maranger and Bird, 1995; Wilhelm and Matteson, 2008). For instance, phage lysis results in the release of the host’s internal cellular contents (e.g., organic carbon, nitrogen), which then becomes a part of the pool of dissolved organic material (DOM). This phenomenon, known as the viral shunt, increases the level of available DOM for other microbes and is suggested to promote bacterial respiration and growth (Wilhelm and Suttle, 1999; Suttle, 2007; Bonilla-Findji et al., 2008). Several studies have surveyed viral diversity through the use of widely, although not universally, distributed marker genes, such as polA. Family A DNA polymerase, polA, which encodes the Pol I protein, is the principal polymerase for phage genome replication, and is suggested to be predictive of viral lifestyle based off a single amino acid substitution (Doublie et al., 1998; Schmidt et al., 2014; Wommack et al., 2015). A phenylalanine (wildtype) or tyrosine at amino acid position 762 (relative to Escherichia coli) is predictive of virulent phage (i.e., lytic replication), while a leucine substitution at this site seems to be predictive of a temperate lifestyle (i.e., lysogenic replication) (Schmidt et al., 2014). Other studies have surveyed viral communities and diversity through shotgun sampling of genomic DNA from viral concentrates (Wommack et al., 2015).
Similar to most bacterial analyses, the majority of studies of viral metagenomes (viromes) have been created from marine samples, which have provided astounding insights into how phage affect the ecology and biology of their hosts (Suttle, 2007; Rohwer and Thurber, 2009). In addition, there have been several viromes created from large freshwater lakes in the Artic (de Cárcer et al., 2015), the Antarctic (López-Bueno et al., 2009), France (Roux et al., 2012), North America (Djikeng et al., 2009; Green et al., 2015; Mohiuddin and Schellhorn, 2015; Watkins et al., 2016), and Ireland (Skvortsov et al., 2016). While limited in their scope, these studies have provided some of the first data on freshwater phage ecology, demonstrating that, like their hosts, phage diversity is influenced by environmental factors. However, only a few studies have evaluated freshwater viromes from small lakes/ponds and fewer have looked at freshwater viromes in conjunction with a temporal analysis of fine scale host diversity (Fancello et al., 2013; Saxton et al., 2016).
Therefore, we aimed to assess the bacterial and viral components of a temperate agricultural pond in the Mid-Atlantic, United States during the late growing season (October–December), a time when declining temperature and nutrient levels may impact the structure and function of the microbial assemblages. Specifically, we used 16S rRNA gene and shotgun metagenomic sequencing to: (i) survey the bacterial consortium utilizing different filter pore sizes (1 and 0.2 μm); (ii) characterize the diversity and abundance of the bacteriophage within the viral community; and (iii) compare the phylogeny of pond viromes across time using the phylogenetically relevant, and biologically meaningful, Pol I protein.
Materials and Methods
Study Site and Sample Collection
Ten-liter water samples were collected in October 2016, November 2016, and December 2016 from a temperate freshwater agricultural pond in central Maryland, United States (maximum depth of ca. 3.35 meters and a surface area of ca. 0.26 ha). A Honda WX10TA (32 GPM) water pump was used to collect water 15–30 cm below the surface into a sterile polypropylene carboy. Samples were kept in the dark at 4°C and processed within 24 h of collection. In addition, a ProDSS digital sampling system (YSI, Yellow Springs, OH, United States) was used to measure, in triplicate: the water temperature (°C), conductivity (SPC uS/cm), pH, dissolved oxygen (%), oxidation/reduction potential (mV), turbidity (FNU), nitrate (mg/L), and chloride (mg/L).
Sample Preparation
Viral and microbial fractions were separated through peristaltic filtration followed by an iron-based flocculation and resuspension of viral particles. Two 142 mm polycarbonate in-line filter holders (Geotech, CO, United States), one equipped with a 142-mm diameter Whatman 1 μm polycarbonate filter (Sigma-Aldrich, MO, United States) and one with a 142-mm diameter 0.2 μm membrane filter (Pall Gelman Sciences, MI, United States), were attached via sterile 1.6 mm PVC tubing. Each water sample was then filtered sequentially through the 1 μm polycarbonate filter followed by the 0.2 μm membrane filter using a Watson Marlow 323 Series Peristaltic Pump (Watson-Marlow, Falmouth, Cornwall, United Kingdom). No prefiltration steps were conducted prior to the sample processing described above. After filtration, both filters (1 and 0.2 μm) were dissected into four equal quadrants and stored at -80°C until DNA extraction. The iron chloride procedure (John et al., 2011) was then used on the resulting filtrate to concentrate viral particles. Briefly, 1 mL FeCl3 solution (4.83 g FeCl3 into 100 ml H2O) was added to the filtered pond water and incubated in the dark for 1 h. Flocculated viral particles were then filtered onto 142-mm 1 μm polycarbonate filters (Sigma-Aldrich, MO, United States) and stored at 4°C in the dark until resuspension. For viral resuspension, filters were rocked overnight at 4°C in 10 mL of 0.1M EDTA-0.2M MgCl2-0.2 M Ascorbate Buffer, described in detail elsewhere (John et al., 2011). To ensure total removal of free DNA contamination, resuspended viral particles were subjected to a DNase I (Sigma-Aldrich, MO, United States) treatment for 2 h and again passed through a 33-mm diameter sterile syringe filter with a 0.2 μm pore size (Millipore Corporation, MA, United States).
Viral DNA Extraction and Shotgun Sequencing
For the virome analysis, DNA was extracted from 500 μl of the treated viral concentrate using the AllPrep DNA/RNA Mini Kit (Qiagen, CA, United States) per the manufacturer’s instructions and quantified with a HS DNA Qubit fluorescent concentration assay. For each sample, DNA was used in the tagmentation reaction, followed by 13 cycles of PCR amplification using Nextera i7 & i5 index primers & 2× Kapa master mix per the modified Nextera XT protocol. The final libraries were then quantitated by KAPA SYBR FAST qPCR kit and sequenced on the Illumina HiSeq 4000 (Illumina, San Diego, CA, United States).
Microbial DNA Extraction, 16S rRNA Gene PCR Amplification, and Sequencing
For the 16S rRNA gene analysis, DNA was extracted from each of the four filter quadrants from the 1 and 0.2 μm filters utilizing an enzymatic and mechanical lysis procedure described in detail elsewhere (Chopyk et al., 2017a,b).
The V3-V4 hypervariable region of the 16S rRNA gene was PCR-amplified and sequenced on the Illumina HiSeq (Illumina, San Diego, CA, United States) utilizing a dual-indexing strategy for multiplexed sequencing developed at the Institute for Genome Sciences (Fadrosh et al., 2014), described in detail elsewhere (Chopyk et al., 2017a,b).
16S rRNA Gene Data Analysis
16S rRNA gene reads were initially screened for low quality bases and short read lengths (Fadrosh et al., 2014), paired reads were merged using PANDAseq (Masella et al., 2012), de-multiplexed, trimmed of artificial barcodes and primers, and assessed for chimeras using UCHIME in de novo mode, as implemented in Quantitative Insights Into Microbial Ecology (QIIME; version 1.9.1-20150604) (Caporaso et al., 2010). Quality-controlled reads were clustered at 97% de novo into operational taxonomic units (OTUs) with the SILVA 16S database (Quast et al., 2012) in QIIME (Caporaso et al., 2010). All sequences taxonomically assigned to chloroplasts were removed from further downstream analysis. When appropriate, data was normalized using metagenomeSeq’s cumulative sum scaling to account for uneven sampling depth (Paulson et al., 2013b).
To visualize the relative abundance of bacterial phyla, stacked bar charts were generated using ggplot2 (Wickham, 2009). In addition, bacterial taxa were summarized at the genera level in QIIME (level = 6) and those with a maximum relative abundance greater than 1% in at least one sample were used to build a heatmap via R (ver. 3.3.2) and vegan heatplus (Ploner, 2012).
Significance tests were conducted using a Tukey’s HSD Test between filter size fractions and among sampling dates. Additionally, Pearson correlation coefficients were calculated to identify associations between the water characteristics and the relative abundance of the bacterial phyla/genera.
Alpha diversity was calculated using the R packages: Bioconductor (Huber et al., 2015), metagenomeSeq (Paulson et al., 2013a), vegan (Oksanen et al., 2007), phyloseq (McMurdie and Holmes, 2013), fossil (Vavrek, 2011), biomformat (McMurdie and Paulson, 2016), and ggplot2 (Wickham, 2009) on data rarefied to an even sampling depth (55,307 sequences) and tested for significance using a Tukey’s HSD Test.
Beta diversity was determined through principle coordinates analysis (PCoA) plots of Bray–Curtis, Weighted and Unweighted UniFrac distances calculated using the R package phyloseq and tested for significance with ANOSIM (9,999 permutations) (Beals, 1984; Clarke, 1993; Lozupone et al., 2011; McMurdie and Holmes, 2013).
Core bacterial OTUs for each filter fraction, and the sample as a whole, were defined as OTUs in 100% of samples determined with QIIME’s compute_core_microbiome.py script (Caporaso et al., 2010). Core OTUs were then visualized using ggplot2, and Krona (Wickham, 2009; Ondov et al., 2011).
Virome Metagenomic Analysis
The paired-end reads were quality trimmed using Trimmomatic (ver. 0.36) (Bolger et al., 2014), merged with FLASh (ver. 1.2.11) (Magoč and Salzberg, 2011), and assembled de novo with metaSPAdes (ver. 3.10.1) without read error correction (Nurk et al., 2017). Taxonomic classifications were assigned to contigs by searching predicted peptide open reading frames (ORFs) against the peptide SEED and Phage SEED databases (retrieved 11/17/2017) (Overbeek et al., 2005). Briefly, peptide ORFs were predicted from virome contigs using MetaGene (Noguchi et al., 2006) and were searched against the SEED and Phage SEED databases using protein-protein BLAST (BLASTp ver. 2.6.0+) (E value ≤ 1e-3) (Altschul et al., 1990). Taxonomy was assigned to contigs using ORF BLASTp hits to SEED sequences with NCBI taxonomy IDs. A contig is assigned the NCBI taxonomy ID with the maximum sum bit score across all ORF BLASTp hits in the contig. Scripts performing these assignments are available at https://github.com/dnasko/baby_virome.
Functional classifications were assigned to ORFs by searching predicted peptide ORFs against the same peptide SEED database. Peptide ORFs were searched against the SEED databases using BLASTp (E value ≤ 1e-3). ORFs were assigned to a SEED subsystem with the maximum sum bit score among all of the ORF’s hits. Only functions associated with viral hits were considered for this analysis.
Counts for taxonomy and functions identified in each virome are based on normalized contig and peptide ORF abundances, respectively. The abundance for each contig was estimated by recruiting all quality controlled reads to the assembled contigs using Bowtie2 (ver. 2.3.3) in very sensitive local mode (Langmead and Salzberg, 2012). Coverage for each contig was calculated using Samtools depth (Li et al., 2009) and a custom parser available at https://github.com/dnasko/baby_virome. ORF abundances are calculated by computing the coverage of the contig within the ORF’s start and stop coordinates. To compare abundance measurements between viromes they are normalized to account for sequencing effort and assembly/recruitment proficiency. Briefly, each contig/ORF’s coverage is divided by the giga base pairs (Gbp) of reads able to recruit back to contigs/ORFs. Taxonomic and functional data were visualized using ggplot2 (Wickham, 2009). Additionally, Pearson correlation coefficients were calculated to identify the associations between the water characteristics and the abundance of the predicted viral taxa.
For the phylogenetic marker gene, predicted ORFs were queried against Pol I UniRef90 (Suzek et al., 2007) clusters using protein-protein BLAST (BLASTp) (Altschul et al., 1990) with an E value cutoff ≤ 1e-5. Significant hits were filtered based on length (≥ 100 amino acids) and then confirmed to be Pol I via NCBI’s Conserved Domain BLAST online tool (Marchler-Bauer et al., 2011). The sequences were then aligned with MAFFT using the FFT-NS-i × 1000 algorithm (Katoh et al., 2002). To obtain biologically meaningful data on the Pol I-containing phage, a region of interest (I547 to N923 in E. coli IAI39) containing the Phe762 position relative to E. coli IAI39 was selected and used to create an unrooted maximum likelihood tree with 100 bootstrap replicates using Geneious 6.0.5 (Kearse et al., 2012) with PhyML (Guindon and Gascuel, 2003). Those with a Phe762 or Tyr762 were defined as generally virulent phage, while those with a Leu762 were defined as generally temperate (Schmidt et al., 2014). Abundances for each Pol I peptide were calculated as described above.
Data Deposition
16S rRNA gene sequences were deposited in NCBI Sequence Read Archive under the accession numbers SRX3387709-SRX3387732. Viral metagenomic reads were also deposited in NCBI’s Sequence Read Archive under the accession numbers SRS2698857, SRS2698856, and SRS2698858.
Results
Water Characteristics
Water properties are described in Table 1. Overall, ambient temperature during sampling, water temperature, nitrate and chloride levels, and turbidity decreased during the study period. Conversely, pH, precipitation levels, conductivity (SPC uS/cm), oxidation/reduction potential (mV), and dissolved oxygen (%) were highest in December.
16S rRNA Gene Sequencing Effort
In total, 24 samples were PCR-amplified for the 16S rRNA gene and sequenced: four quadrants each from the 1 and 0.2 μm filters from each sampling date (October, November, and December). After sequence quality filtering, 7,489 OTUs (97% identity) were identified from a total of 2.5 million sequences across all samples, with an average number of ca. 103,000 (± ca. 36,000 SD) sequences per sample and an average of ca. 2,100 (± ca. 500) OTUs.
Bacterial Community Composition and Temporal Variations
The most abundant pond water phyla were Actinobacteria, Proteobacteria, and Bacteroidetes in all samples, however, their average relative abundance fluctuated over the time course and between filter pore sizes (Figure 1A and Supplementary Table S1, S2). For instance, Actinobacteria was significantly (p < 0.05) higher at all time points in the 0.2 μm fraction, whereas Chloroflexi, Firmicutes, Cyanobacteria, and Proteobacteria were significantly higher in the 1 μm fraction at all time points (Figure 1A and Supplementary Table S1). From October to December in both fractions the relative abundance of Bacteroidetes increased significantly (p < 0.05), whereas TM7 and Cyanobacteria decreased (Supplementary Table S2).
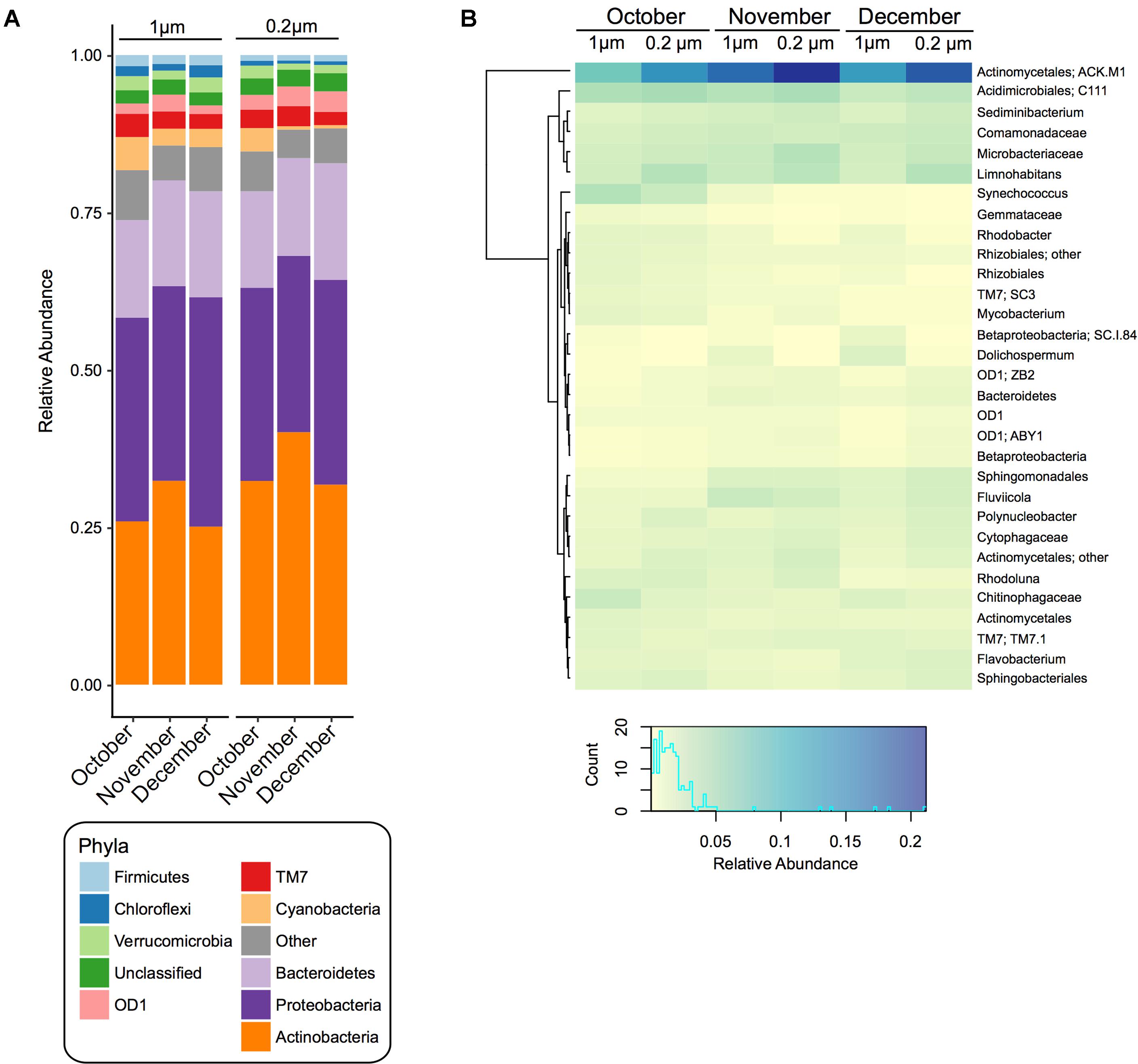
FIGURE 1. Bacterial community composition and diversity of 1 and 0.2 μm filter fractions over time. (A) Stacked bar chart of the relative abundance of the bacterial community composition at the phylum level within pond samples from each month (October, November, and December) and each filter fraction. (B) Heatmap based on the relative abundance of the bacterial community composition at the genus level. Displayed are genera or lowest available taxonomic assignments representing more than 1% in at least one of the pond samples. Pooled samples are clustered using Manhattan distance. Color key depicts the spectrum of relative abundance with a histogram of the counts of individual values.
At the genera level, summarized via QIIME, there were 31 taxa that were greater than 1% in at least one sample (Figure 1B). Of these, 8 had a significantly (p < 0.05) higher relative abundance at all time points in the 0.2 μm fraction than the 1 μm fraction (ACK.M1, Limnohabitans, Microbacteriaceae, Sediminibacterium, Polynucleobacter, Actinomycetales, Cytophagaceae, ZB2), while six were significantly (p < 0.05) greater in the 1 μm fraction compared to the 0.2 μm fraction (Synechococcus, Chitinophagaceae, Dolichospermum, Rhizobiales, SC.I.84, Gemmataceae) (Figure 1B and Supplementary Table S3).
Moreover, within each fraction there were significant (p < 0.05) changes in the relative abundance of the bacterial taxa over the sampling period (Figure 1B and Supplementary Table S4). For instance, in the 1 and 0.2 μm fractions, ACK.M1, Fluviicola, Sphingomonadales, Dolichospermum, Flavobacterium, Bacteroidetes, SC.I.84, and Betaproteobacteria increased significantly (p < 0.05) from October to December, while C111, Synechococcus, Chitinophagaceae, Rhodoluna, Actinomycetales, Rhodobacter, Rhizobiales, Mycobacterium, Rhizobiales, SC3, and Gemmataceae decreased significantly (p < 0.05). In addition to the taxa above, in the 1 μm fraction there was also a significant (p < 0.05) increase in Comamonadaceae and Polynucleobacter and a significant decrease in Sphingobacteriales and OD1 between the October and December sampling dates. For the 0.2 μm fraction, there was also a significant (p < 0.05) increase in the relative abundance of Sediminibacterium, Cytophagaceae, ZB2, and ABY1 between the October and December sampling dates.
Relationships Between Water Characteristics and Bacterial Abundance
Despite the small sample size (n = 3), several bacterial phyla showed significant correlations (p < 0.05) with the measured water characteristics (Supplementary Figure S1A). In both filter fractions, the relative abundance of Actinobacteria was negatively correlated with the level of dissolved oxygen. In just the 1 μm fraction, the relative abundance of Verrucomicrobia and Proteobacteria were positively correlated with dissolved oxygen and pH, respectively, while TM7 was positively correlated with both water temperature and turbidity. In addition, in just the 0.2 μm fraction, the relative abundance of Firmicutes showed positive correlations with pH and oxidation/reduction. Conversely, the relative abundance of Bacteroidetes, TM7, and OD1 were negatively related to, chloride, pH, and nitrate, respectively (p < 0.05). At the genera level in both filter fractions, Synechococcus was positively correlated with nitrate (Supplementary Figure S1B).
Bacterial Alpha Diversity
Alpha diversity was computed for Observed OTUs and Shannon diversity and tested for significance between filter size and over time within each fraction (Figure 2).
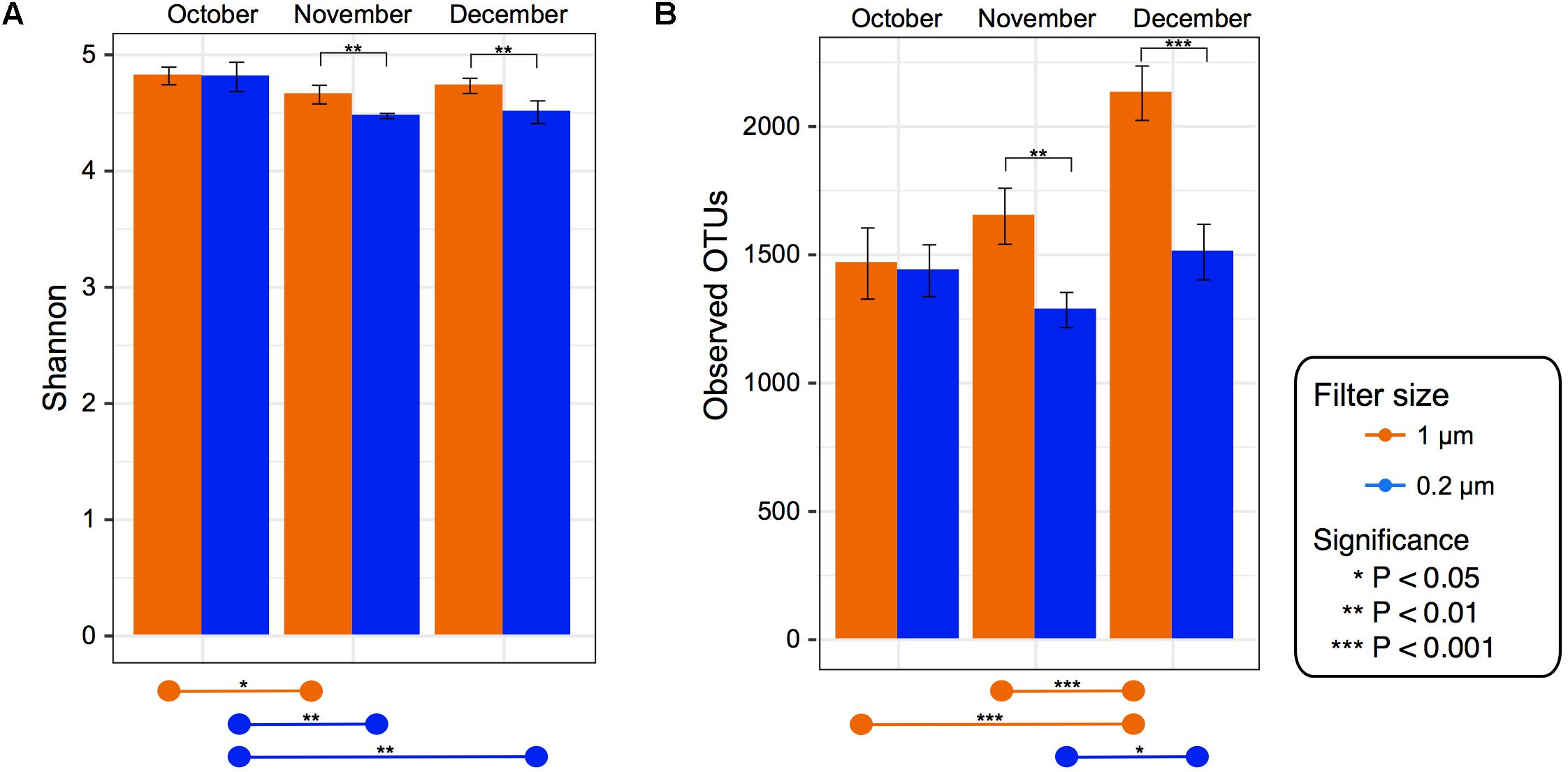
FIGURE 2. Alpha diversity for each filter fraction in late season pond water. Bar charts of alpha diversity measured using (A) Shannon index and (B) Observed OTUs. Color denotes filter pore size, either 1 μm (orange) or 0.2 μm (blue). Pairwise significance between filter size denoted by brackets within graph for each date. Significance within each filter among time points denoted by lines at bottom of the figure. Error bars denote standard deviation.
Despite the 0.2 μm fraction containing significantly higher levels of some of the dominant genera, the 1 μm fraction had a significantly higher Shannon index and observed OTU value in the November and December (p < 0.05) samples (Figure 2). When testing within each fraction over time, the Shannon index values in the 1 μm fraction were significantly (p < 0.05) higher in October than November. In the 0.2 μm fraction the Shannon index values were significantly higher in October than both November and December (Figure 2). However, the Observed OTU values in the 1 μm fraction were significantly higher in December than both October and November, while in the 0.2 μm fraction December was only significantly higher than November.
Bacterial Beta Diversity
Beta diversity comparisons using PCoA plots of Bray–Curtis distances computed for all samples revealed significant clustering by date (R = 0.94, p = 0.001) along axis 1, which accounted for nearly 45% of the variation (Figure 3). Samples along axis 2 (17% of the variation) appeared to cluster by filter size (1 μm vs. 0.2 μm). This trend was also observed both utilizing weighted (R = 0.7458, p = 0.001) and unweighted (R = 0.8524, p = 0.001) UniFrac (Figure 3) distances. Additionally, within each date, samples clustered by filter pore size: December (R = 1, p = 0.022), November (R = 1, p = 0.022), and October (R = 1, p = 0.029) (Supplementary Figure S2).
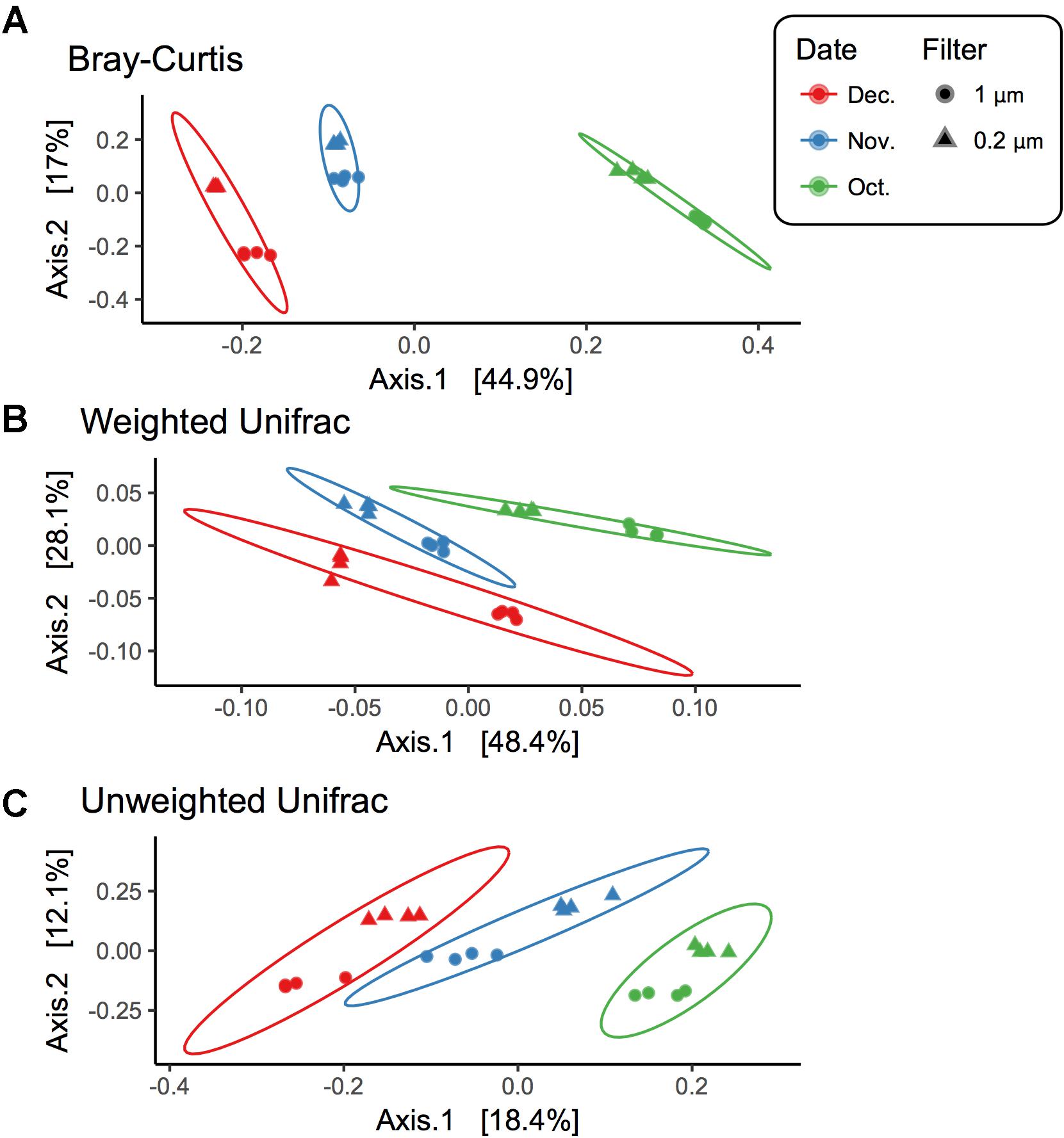
FIGURE 3. Beta diversity for each filter fraction in late season pond water. PCoA plots of beta diversity measured using (A) Bray–Curtis, (B) Weighted UniFrac, and (C) Unweighted UniFrac. Shape denotes filter pore size, either 1 μm (circle) or 0.2 μm (triangle), and color denotes month when water was sampled, October (green), November (blue), and December (red). Ellipses are drawn at 95% confidence intervals for each month.
Core OTUs
There were 277 core OTUs present in the farm pond water over the 3 months across both fractions (Figure 4). These were largely Actinobacteria (127 OTUs), followed by: Proteobacteria (82 OTUs), Bacteroidetes (43 OTUs), TM7 (9 OTUs), OD1 (4 OTUs), Verrucomicrobia (2 OTUs), Firmicutes (2 OTUs), Chloroflexi (2 OTUs), Cyanobacteria (1 OTU), WS5 (1 OTU), SR1 (1 OTU), GN02 (1 OTU), Fusobacteria (1 OTU), and Chlamydiae (1 OTU). For the 0.2 μm fraction, there was a unique core of 152 OTUs, largely Actinobacteria (90 OTUs), followed by Proteobacteria (33 OTUs), and Bacteroidetes (23 OTUs), Verrucomicrobia (2 OTUs), TM7 (1 OTU), GN02 (1 OTU), Chlamydiae (1 OTU), and Spirochaetes (1 OTU). The unique core for the 1 μm fraction was more diverse in bacterial phyla compared to the 0.2 μm. It consisted largely of Proteobacteria (78 OTUs), followed by Actinobacteria (16 OTUs), Bacteroidetes (16 OTUs), Cyanobacteria (6 OTUs), Firmicutes (6 OTUs), Chloroflexi (5 OTUs), TM7 (5 OTUs), Planctomycetes (4 OTUs), Acidobacteria (4 OTUs), Verrucomicrobia (3 OTUs), Gemmatimonadetes (2 OTUs), OD1 (1 OTU), and WS5 (1 OTU).
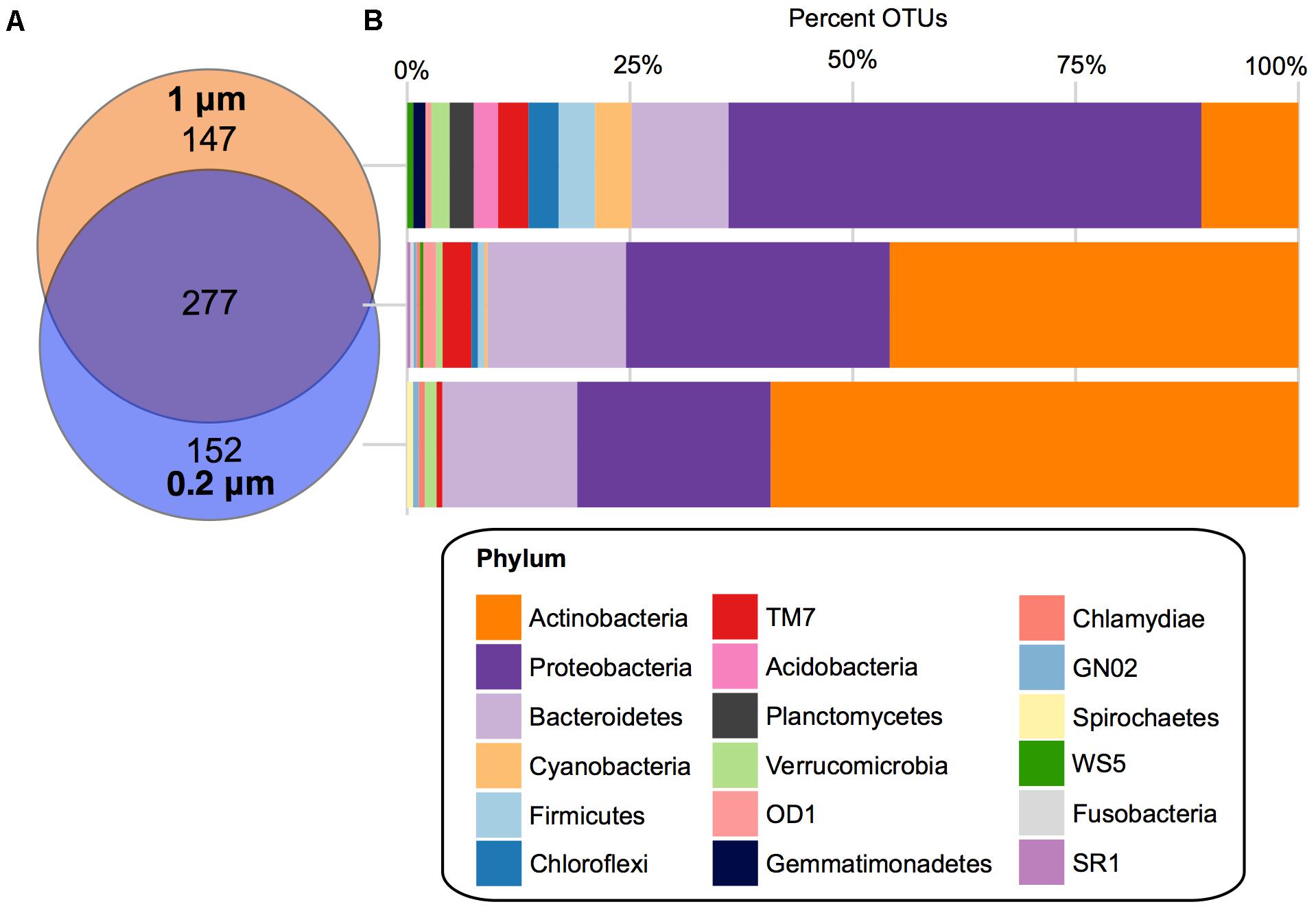
FIGURE 4. Core OTUs for 1 and 0.2 μm filter fractions. (A) Venn diagram depicting the unique and shared OTUs that occurred in 100% of samples in the 1 μm (orange) and 0.2 μm (blue) filter fractions. (B) Stacked bar charts of the percentage of OTUs assigned each phylum within the shared and unique cores.
When looking at the core bacterial taxa at a lower taxonomic level, in the 0.2 μm fraction the Actinobacteria OTUs were dominated by Actinomycetales ACK-M1 (70%), followed by Rhodoluna (4%) under the family Microbacteriaceae (Supplementary Figure S3B). The Proteobacteria were largely Limnohabitans (24%) and Polynucleobacter (30%) and the Bacteroidetes were Sediminibacterium (22%) and a large unclassified family of Cytophagaceae (35%) (Supplementary Figure S3B). Within the unique core of the 1 μm fraction, the only prominent genus of the Proteobacteria was Rhobacter at 5%, followed by Novosphingobium at 3% and 17 other genera (each at 1%) (Supplementary Figure S3A). At a higher taxonomic level, a family of largely unclassified Rhizobiales was also abundant (23%). The Actinobacteria were dominated by Acidimicrobiales C111 (25%), Solirubrobacterales (13%), and a large proportion of mostly unclassified Actinomycetales (44%). Finally, in the Bacteroidetes phylum of the 1 μm fraction there was mostly Fluviicola at 13%, as well as Leadbetterella, Runella, and Sediminibacterium, which were each at 6%.
Shotgun Sequencing Effort and Assembly
Each sample from the viral fraction was sequenced on the Illumina HiSeq for a total of 89,645,509 read pairs (35,522,822 from October; 32,988,451 from November; 21,134,236 from December). We assembled the reads from all sampling dates to construct a total of 872,200 contigs (272,687 from October; 409,758 from November; 189,755 from December). The mean contig length was 593 nucleotides in October (range from 55 to 366,802 nucleotides), 641 in November (range from 55 to 286,691 nucleotides), and 655 in December (range from 55 to 331,733 nucleotides). The GC content was similar among the three sampling dates: October (Mean 46.51%, Median 45.42%); November (Mean 46.18%, Median 45.59%); and December (Mean 46.12%, Median 45.21%).
Viral Taxonomic Composition and Abundance
Similar to other studies, a large portion of the assembled contigs within the viral fraction had no known homologs (42% October, 51% November, and 42% December could be assigned taxonomy) (Krishnamurthy and Wang, 2017). For those that did have a hit we calculated the normalized abundance. From this we found that 47% (October), 27% (November), and 53% (December) of the assigned taxonomic composition were homologous to viruses; the vast majority of which were from the dsDNA bacteriophage Caudovirales (99%, 98%, and 99%) (Figure 5A). Within the Caudovirales, Siphoviridae dominated at all time points followed by Myoviridae and then Podoviridae. Other viral categories included those homologous to ssDNA viruses Inoviridae, dsDNA viruses Tectiviridae, Ligamenvirales, Bicaudaviridae, and other unclassified bacteriophage (Figure 5A). For the dominant viral families (Podoviridae, Siphoviridae, and Myoviridae), there were no water characteristic that significantly correlated with their abundances; however, there were several bacterial taxa whose relative abundance correlated with the abundance of the dominant viral families (Supplementary Figure S4). For instance, at the phylum level the relative abundance of OD1 in the 1 μm fraction negatively correlated with the abundance of Siphoviridae, while in the 0.2 μm the relative abundance of TM7 negatively correlated with the abundance of Podoviridae (Supplementary Figure S4).
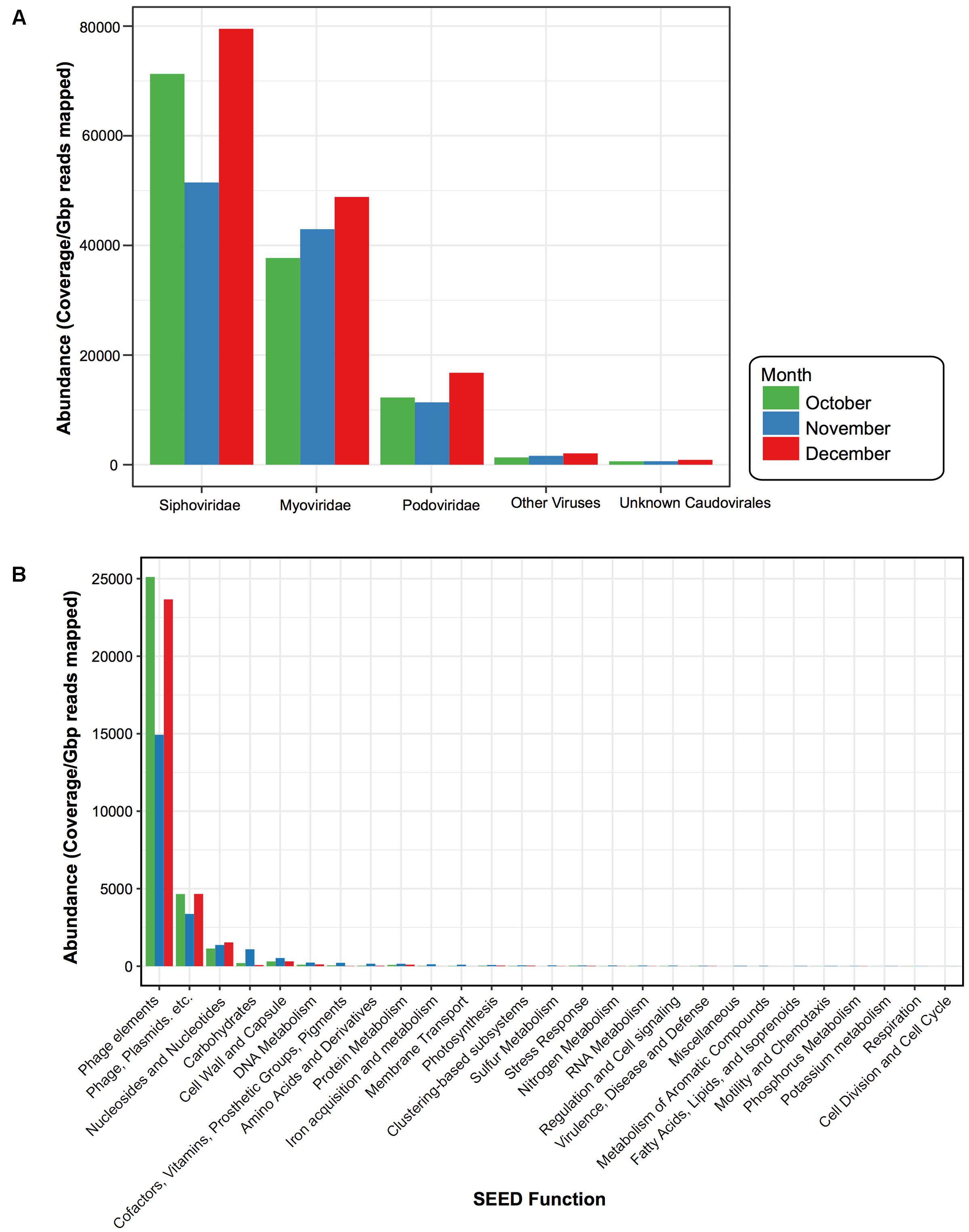
FIGURE 5. Viral taxonomy and function for each sampling date. Bar plots comparing the normalized abundance of viruses with homology to known (A) viral taxa and (B) functional SEED categories. Bar color denotes month when water was sampled, October (green), November (blue), and December (red). Phage elements refer to the SEED functional category “Phages, Prophages, Transposable elements” and Phage, Plasmids, etc. denote the SEED functional category “Phages, Prophages, Transposable elements, Plasmids.” Abundance determined by calculating coverage/Gbp reads mapped.
Viral Functional Composition
ORFs from the virus-assigned contigs were functionally annotated using the SEED Subsystems (Overbeek et al., 2005). Again, to compare these viral categories across the 3 months, we calculated the normalized abundance for each of the ORFs assigned to the SEED functional categories (Figure 5B). While ORFs homologous to virulent genes were present (e.g., Multidrug Resistance Efflux Pumps, Zinc Resistance, Copper Homeostasis) they were not abundant within the time period. The majority (93% October, 80% November, and 92% December) were Phage Elements, defined by the SEED subsystem hierarchy (Overbeek et al., 2005) as either “Phages, Prophages, Transposable elements,” which were largely phage structural genes (e.g., capsid, scaffolding) or “Phages, Prophages, Transposable elements, and Plasmids,” which were genes related to phage replication and packaging (e.g., terminase, integrase, helicase, primase). This was followed by “Nucleosides & Nucleotides” (4% October, 6% November, and 5% December), which were largely genes involved in ribonucleotide reduction.
Viral Marker Gene: Polymerase A
A total of 842 confirmed Pol I peptides were extracted from our assembled pond water viromes (271 October, 320 November, and 251 December). From these, only 228 spanned the region of interest and were included in the phylogenic analysis: 68 in October, 83 in November, and 77 in December. The phylogenetic analysis (Figure 6) showed that the Pol I peptides grouped largely by their 762 position, whereas the sampling dates were distributed among the different clades. Again, to compare the Pol Is we calculated the normalized abundance for each. While the majority of Pol I peptides had the Leu substitution at the 762 site (184), followed by Tyr (27) and then the wildtype Phe (17), the clade at the highest abundance was those with the wildtype Phe mutation. Because this clade was so abundant, we used BLASTp to assess the top hit, which were uncultured bacteriophage from the Dry Tortugas surface water (Schmidt et al., 2014) and the seawater collected from the deep chlorophyll maximum of the Mediterranean Sea (Mizuno et al., 2013) (E value < 1e-300).
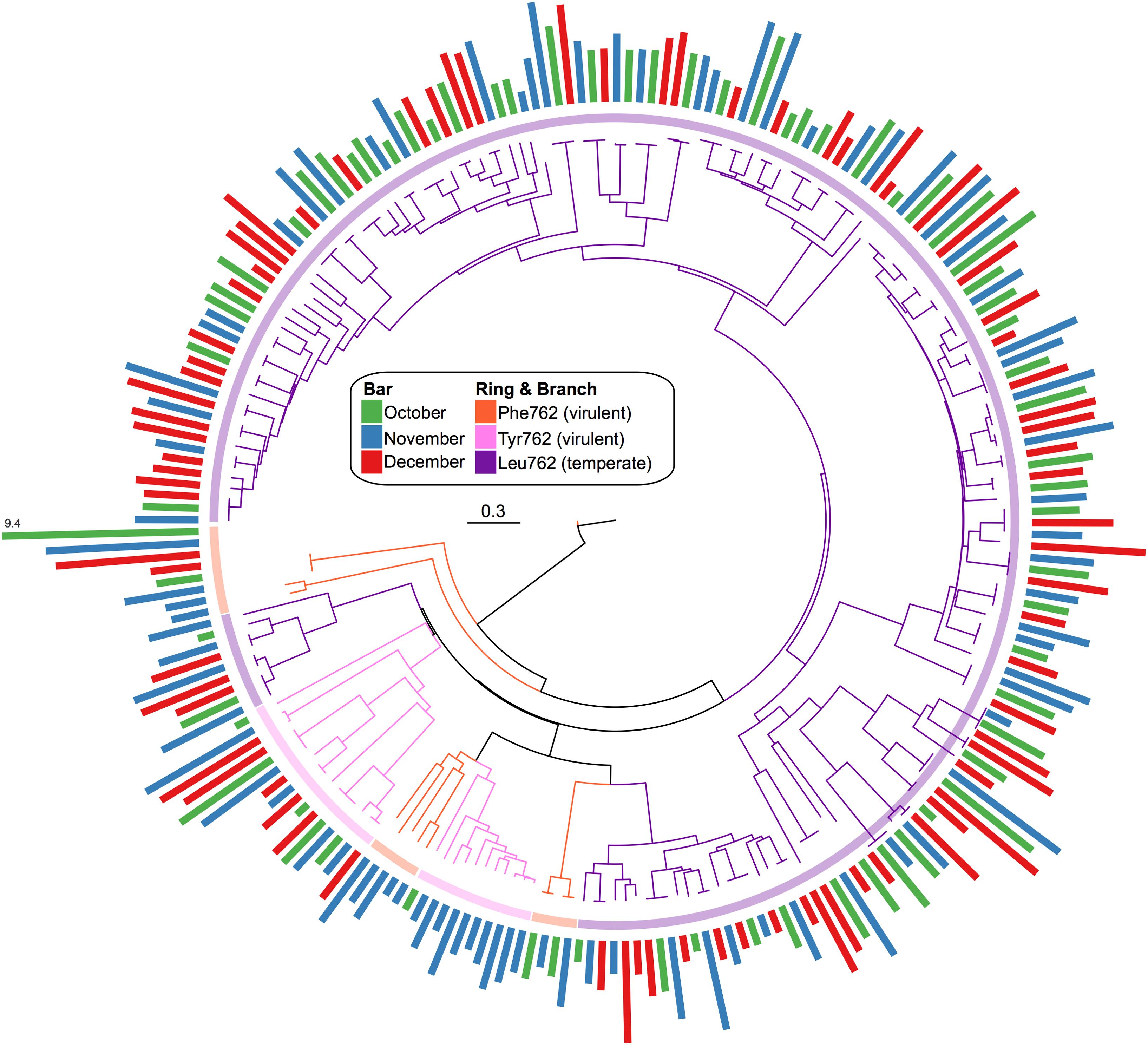
FIGURE 6. Phylogenic tree of viral Pol I peptides for each sampling date. Unrooted maximum likelihood tree with 100 bootstrap replicates of representative Pol I peptide sequences. Branches and inner ring colored by 762 position residues, Phe762 (orange), Tyr762 (pink), and Leu762 (purple). Radial bar chart represents the log2-normalized abundance of each peptide (coverage/Gbp reads mapped) and is colored by sampling date, October (green), November (blue), and December (red).
Discussion
While ponds represent a potential source of irrigation water, provided adequate filtering and monitoring technologies are employed, it is important to keep in mind their role in the ecosphere. Their small size and shallow depth enables a complex community of aquatic plants and macroinvertebrate species, as well as an interacting community of microorganisms.
However, changing water levels and anthropogenic factors associated with irrigation systems may interrupt the delicate balance of microbial life, which can ultimately impact higher trophic levels. Therefore, it is critical to carefully manage our use, or contamination, of these systems in search of irrigable water.
Here, the studied pond was dominated by Actinobacteria, Proteobacteria, and Bacteroidetes, common phyla found in most freshwater lakes (Newton et al., 2011). However, as the late season progressed there appeared to be changes in the bacterial community composition that correlated with specific environmental factors. For instance, Actinobacteria fluctuated throughout the sampling period, and was negatively correlated with the percentage of dissolved oxygen (Figure 1A and Supplementary Figure S1A). A previous study of the Luhuitou fringing coral reef also reported a negative correlation between dissolved oxygen and the abundance of several Actinobacteria species that may be due to their anaerobic capabilities (Kuang et al., 2015). This relationship may help to explain the fluctuations in freshwater Actinobacteria abundances and diversity described in other seasons (Allgaier and Grossart, 2006). At a lower taxonomic level, the relative abundance of Synechococcus decreased significantly throughout the late season (Figure 1B and Supplementary Table S4). This is not surprising as the growth rate of Synechococcus is known to decrease with temperature and nitrogen levels, which occurred during the sampling period (Sakamoto and Bryant, 1997, 1999).
While the changes in relative abundance described above were apparent in both filter fractions (1 and 2 μm), there were differences in alpha diversity, beta diversity, and core bacterial composition between the two fractions (Figures 1–3 and Supplementary Figure S1). For instance, the 0.2 μm core microbiota was dominated by Actinobacteria, largely Actinomycetales ACK-M1, a known representative in most freshwater habitats (Van der Gucht et al., 2005) and free-living Proteobacteria, Limnohabitans, and Polynucleobacter, (Hahn et al., 2005, 2010; Šimek et al., 2010) (Figure 4 and Supplementary Figure S3). Whereas, the core of the 1 μm filter fraction was dominated by a diverse set of Proteobacteria, largely of the order Rhizobiales and Rhodobacteriales, previously found to dominate the particle-associated fraction in a marine pelagic trench (Eloe et al., 2011) (Figure 4 and Supplementary Figure S3).
Furthermore, the bacterial richness was significantly higher in the 1 μm fraction in December and November (Figure 2). This suggests that the diversity of the large/attached bacteria recovered from the 1 μm filter were greater in the later months (November and December) compared to the smaller/free-living bacterial communities of the 0.2 μm filter. This increased diversity in the larger fraction agrees with studies investigating the differences between the particle-associated and free-living bacteria in other aquatic systems, such as the Baltic and Mediterranean Seas (Crespo et al., 2013; Rieck et al., 2015). While the alpha diversity trends were similar between the two filter fractions, the increasing richness was more prominent in the 1 μm filter fraction (Figure 2). There can be several factors that may influence this discrepancy in temporal diversity, such as changing protozoan grazing rates and/or viral lysis impacting the microbial food web (Šimek et al., 1999; Hahn and Höfle, 2001; Weinbauer et al., 2007). Additionally, it can be suggested that the attached/large bacteria of the 1 μm fraction may have been better equipped to compete in an environment of decreased water temperature, nitrate, and chloride levels (Figure 1). In fact, previous studies have reported particle-associated bacteria have larger genomes compared to streamlined free-living bacteria (Smith et al., 2013). This reservoir of genetic material may allow for a more adaptive lifestyle in the face of changing conditions, competing bacterial populations and predators.
The viral fraction was dominated by sequences homologous to the tailed, double stranded DNA bacteriophage of the order Caudovirales (Figure 5A). Within the Caudovirales order, however, the family of generally temperate Siphoviruses was the most abundant at each time point (Figure 5A). This is in contrast to other freshwater systems, where Myoviruses and Podoviruses (Lakes Ontario, Erie, Matoaka, and Michigan (Fancello et al., 2013; Green et al., 2015; Mohiuddin and Schellhorn, 2015) were found to dominate. Conversely, Siphoviruses were reported to be abundant in sediments (Breitbart et al., 2004) and terrestrial subsurface environments (Edwards and Rohwer, 2005). Siphovirus abundance within the pond water sampled here may reflect the pond’s unique topology compared to larger lakes. Ponds and small lakes have a higher terrestrial-aquatic interchange than larger freshwater systems due to their close contact with the adjacent terrestrial environment (Palik et al., 2001; Søndergaard et al., 2005). This large littoral zone may promote the influx of terrestrial/sediment Siphoviruses. Additionally, because the dominant lifecycle of cultured Siphoviruses (Suttle, 2005) is lysogenic, their abundance may be indicative of environmental stress, (e.g., changes in nutrients, pH or temperature) activating the lytic-lysogenic switch and thus increasing their presence in the free phage fraction (McDaniel et al., 2002). This agrees with the phylogenetic analysis of the informative viral marker gene family A DNA polymerase, polA (Pol I protein). In this case, the Pol I proteins were temporally persistent and dominated by the Leu762 mutation, suggested to be indicative of temperate phage (Figure 6) (Schmidt et al., 2014).
This abundance of temperate phage is important to note as they can alter the genetic architecture of their hosts, which can in turn influence the surrounding microbial community and environment. For instance, temperate phage can transduce bacterial DNA and potentially alter host biology, such as maintaining host photosynthesis during infection or encoding virulence and antibiotic resistance genes (Wagner and Waldor, 2002; Lindell et al., 2004; Balcazar, 2014). In this case, while present, genes involved in virulence/antimicrobial resistance were not enriched within the viral fraction during the study period (Figure 5B). However, the samples did contain a high abundance of genes involved in Nucleosides & Nucleotides production, largely ribonucleotide reduction genes. This is not surprising as ribonucleotide reductases (RNRs) have been observed frequently within aquatic viral metagenomic libraries and namely in Myoviruses (Angly et al., 2006; Bench et al., 2007; Wommack et al., 2015).
From these data we were able to characterize the bacterial and viral taxonomic and functional components within an agricultural pond over time. In several cases, we observed the abundance of the dominant viral families correlated with the relative abundance of bacterial taxa. However, more work is necessary to track and model these interacting species. A more thorough analysis connecting phage with their hosts, such as through the use of the Clustered regularly interspaced short palindromic repeats (CRISPR) system, is warranted to provide a more nuanced assessment of their relationship in pond water and potential impact in the microbial food web (e.g., viral shunt). Additionally, other parameters like phosphate, dissolved organic carbon, chlorophyll concentration, and protozoan grazing rates might also exert some influence on the temporal dynamics observed here and should be included in future studies of freshwater ponds. Overall, this analysis serves as a baseline of the diversity and dynamics of three distinct microbial fractions in agricultural pond water.
Author Contributions
JC performed processing of water samples following collection, conducted the majority of the bioinformatic and biostatistical analyses following sequence processing, and wrote and edited the manuscript. DN performed metagenomic assemble and abundance calculations. SA and AB participated in the sample collection and aided in DNA extraction. AS and EM contributed to the study design, protocol development, data analysis and interpretation, and manuscript preparation. All authors read, edited, and approved the final manuscript.
Funding
This work was supported by the United States Department of Agriculture-National Institute of Food and Agriculture, Grant number 2016-68007-25064, that established CONSERVE: A Center of Excellence at the Nexus of Sustainable Water Reuse, Food and Health, and the Computation and Mathematics for Biological Networks (COMBINE) NSF Research Traineeship program (NSF: 1632976).
Conflict of Interest Statement
The authors declare that the research was conducted in the absence of any commercial or financial relationships that could be construed as a potential conflict of interest.
Supplementary Material
The Supplementary Material for this article can be found online at: https://www.frontiersin.org/articles/10.3389/fmicb.2018.00792/full#supplementary-material
References
Allgaier, M., and Grossart, H.-P. (2006). Diversity and seasonal dynamics of Actinobacteria populations in four lakes in northeastern Germany. Appl. Environ. Microbiol. 72, 3489–3497. doi: 10.1128/AEM.72.5.3489-3497.2006
Altschul, S. F., Gish, W., Miller, W., Myers, E. W., and Lipman, D. J. (1990). Basic local alignment search tool. J. Mol. Biol. 215, 403–410. doi: 10.1016/S0022-2836(05)80360-2
Angly, F. E., Felts, B., Breitbart, M., Salamon, P., Edwards, R. A., Carlson, C., et al. (2006). The marine viromes of four oceanic regions. PLoS Biol. 4:e368. doi: 10.1371/journal.pbio.0040368
Balcazar, J. L. (2014). Bacteriophages as vehicles for antibiotic resistance genes in the environment. PLoS Pathog. 10:e1004219. doi: 10.1371/journal.ppat.1004219
Beals, E. W. (1984). Bray-Curtis ordination: an effective strategy for analysis of multivariate ecological data. Adv. Ecol. Res. 14, 1–55. doi: 10.1016/S0065-2504(08)60168-3
Bench, S. R., Hanson, T. E., Williamson, K. E., Ghosh, D., Radosovich, M., Wang, K., et al. (2007). Metagenomic characterization of Chesapeake Bay virioplankton. Appl. Environ. Microbiol. 73, 7629–7641. doi: 10.1128/AEM.00938-07
Biggs, J., Williams, P., Whitfield, M., Nicolet, P., and Weatherby, A. (2005). 15 years of pond assessment in Britain: results and lessons learned from the work of Pond Conservation. Aquat. Conserv. 15, 693–714. doi: 10.1002/aqc.745
Bolger, A. M., Lohse, M., and Usadel, B. (2014). Trimmomatic: a flexible trimmer for Illumina sequence data. Bioinformatics 30, 2114–2120. doi: 10.1093/bioinformatics/btu170
Bonilla-Findji, O., Malits, A., Lefèvre, D., Rochelle-Newall, E., Lemée, R., Weinbauer, M. G., et al. (2008). Viral effects on bacterial respiration, production and growth efficiency: consistent trends in the Southern Ocean and the Mediterranean Sea. Deep Sea Res. Part II 55, 790–800. doi: 10.1016/j.dsr2.2007.12.004
Breitbart, M., Felts, B., Kelley, S., Mahaffy, J. M., Nulton, J., Salamon, P., et al. (2004). Diversity and population structure of a near–shore marine–sediment viral community. Proc. R. Soc. Lond. B 271, 565–574. doi: 10.1098/rspb.2003.2628
Caporaso, J. G., Kuczynski, J., Stombaugh, J., Bittinger, K., Bushman, F. D., Costello, E. K., et al. (2010). QIIME allows analysis of high-throughput community sequencing data. Nat. Methods 7, 335–336. doi: 10.1038/nmeth.f.303
Céréghino, R., Biggs, J., Oertli, B., and Declerck, S. (2008). The ecology of European ponds: defining the characteristics of a neglected freshwater habitat. Hydrobiologia 597, 1–6. doi: 10.1007/s10750-007-9225-8
Chopyk, J., Chattopadhyay, S., Kulkarni, P., Claye, E., Babik, K. R., Reid, M. C., et al. (2017a). Mentholation affects the cigarette microbiota by selecting for bacteria resistant to harsh environmental conditions and selecting against potential bacterial pathogens. Microbiome 5:22. doi: 10.1186/s40168-017-0235-0
Chopyk, J., Chattopadhyay, S., Kulkarni, P., Smyth, E. M., Hittle, L. E., Paulson, J. N., et al. (2017b). Temporal variations in cigarette tobacco bacterial community composition and tobacco-specific nitrosamine content are influenced by brand and storage conditions. Front. Microbiol. 8:358. doi: 10.3389/fmicb.2017.00358
Chow, C.-E. T., Kim, D. Y., Sachdeva, R., Caron, D. A., and Fuhrman, J. A. (2014). Top-down controls on bacterial community structure: microbial network analysis of bacteria, T4-like viruses and protists. ISME J. 8, 816–829. doi: 10.1038/ismej.2013.199
Clarke, K. R. (1993). Non-parametric multivariate analyses of changes in community structure. Austral Ecol. 18, 117–143. doi: 10.1111/j.1442-9993.1993.tb00438.x
Crespo, B. G., Pommier, T., Fernández-Gómez, B., and Pedrós-Alió, C. (2013). Taxonomic composition of the particle-attached and free-living bacterial assemblages in the Northwest Mediterranean Sea analyzed by pyrosequencing of the 16S rRNA. Microbiologyopen 2, 541–552. doi: 10.1002/mbo3.92
de Cárcer, D. A., López-Bueno, A., Pearce, D. A., and Alcamí, A. (2015). Biodiversity and distribution of polar freshwater DNA viruses. Sci. Adv. 1:e1400127. doi: 10.1126/sciadv.1400127
De Meester, L., Declerck, S., Stoks, R., Louette, G., Van De Meutter, F., De Bie, T., et al. (2005). Ponds and pools as model systems in conservation biology, ecology and evolutionary biology. Aquat. Conserv. 15, 715–725. doi: 10.1002/aqc.748
Djikeng, A., Kuzmickas, R., Anderson, N. G., and Spiro, D. J. (2009). Metagenomic analysis of RNA viruses in a fresh water lake. PLoS One 4:e7264. doi: 10.1371/journal.pone.0007264
Doublie, S., Tabor, S., Long, A. M., Richardson, C. C., and Ellenberger, T. (1998). Crystal structure of a bacteriophage T7 DNA replication complex at 2.2 Å resolution. Nature 391, 251–258. doi: 10.1038/34593
Edwards, R. A., and Rohwer, F. (2005). Opinion: viral metagenomics. Nat. Rev. Microbiol. 3:504. doi: 10.1038/nrmicro1163
Eloe, E. A., Shulse, C. N., Fadrosh, D. W., Williamson, S. J., Allen, E. E., and Bartlett, D. H. (2011). Compositional differences in particle-associated and free-living microbial assemblages from an extreme deep-ocean environment. Environ. Microbiol. Rep. 3, 449–458. doi: 10.1111/j.1758-2229.2010.00223.x
Fadrosh, D. W., Ma, B., Gajer, P., Sengamalay, N., Ott, S., Brotman, R. M., et al. (2014). An improved dual-indexing approach for multiplexed 16S rRNA gene sequencing on the Illumina MiSeq platform. Microbiome 2, 1–7. doi: 10.1186/2049-2618-2-6
Fancello, L., Trape, S., Robert, C., Boyer, M., Popgeorgiev, N., Raoult, D., et al. (2013). Viruses in the desert: a metagenomic survey of viral communities in four perennial ponds of the Mauritanian Sahara. ISME J. 7, 359–369. doi: 10.1038/ismej.2012.101
Glöckner, F. O., Zaichikov, E., Belkova, N., Denissova, L., Pernthaler, J., Pernthaler, A., et al. (2000). Comparative 16S rRNA analysis of lake bacterioplankton reveals globally distributed phylogenetic clusters including an abundant group of actinobacteria. Appl. Environ. Microbiol. 66, 5053–5065. doi: 10.1128/AEM.66.11.5053-5065.2000
Green, J. C., Rahman, F., Saxton, M. A., and Williamson, K. E. (2015). Metagenomic assessment of viral diversity in Lake Matoaka, a temperate, eutrophic freshwater lake in southeastern Virginia, USA. Aquat. Microb. Ecol. 75, 117–128. doi: 10.3354/ame01752
Guindon, S., and Gascuel, O. (2003). A simple, fast, and accurate algorithm to estimate large phylogenies by maximum likelihood. Syst. Biol. 52, 696–704. doi: 10.1080/10635150390235520
Hahn, M. W., and Höfle, M. G. (2001). Grazing of protozoa and its effect on populations of aquatic bacteria. FEMS Microbiol. Ecol. 35, 113–121. doi: 10.1111/j.1574-6941.2001.tb00794.x
Hahn, M. W., Kasalický, V., Jezbera, J., Brandt, U., and Šimek, K. (2010). Limnohabitans australis sp. nov., isolated from a freshwater pond, and emended description of the genus Limnohabitans. Int. J. Syst. Evol. Microbiol. 60, 2946–2950. doi: 10.1099/ijs.0.022384-0
Hahn, M. W., Pöckl, M., and Wu, Q. L. (2005). Low intraspecific diversity in a Polynucleobacter subcluster population numerically dominating bacterioplankton of a freshwater pond. Appl. Environ. Microbiol. 71, 4539–4547. doi: 10.1128/AEM.71.8.4539-4547.2005
Hennes, K. P., Suttle, C. A., and Chan, A. M. (1995). Fluorescently labeled virus probes show that natural virus populations can control the structure of marine microbial communities. Appl. Environ. Microbiol. 61, 3623–3627.
Huber, W., Carey, V. J., Gentleman, R., Anders, S., Carlson, M., Carvalho, B. S., et al. (2015). Orchestrating high-throughput genomic analysis with Bioconductor. Nat. Methods 12, 115–121. doi: 10.1038/nmeth.3252
John, S. G., Mendez, C. B., Deng, L., Poulos, B., Kauffman, A. K. M., Kern, S., et al. (2011). A simple and efficient method for concentration of ocean viruses by chemical flocculation. Environ. Microbiol. Rep. 3, 195–202. doi: 10.1111/j.1758-2229.2010.00208.x
Katoh, K., Misawa, K., Kuma, K. I., and Miyata, T. (2002). MAFFT: a novel method for rapid multiple sequence alignment based on fast Fourier transform. Nucleic Acids Res. 30, 3059–3066. doi: 10.1093/nar/gkf436
Kearse, M., Moir, R., Wilson, A., Stones-Havas, S., Cheung, M., Sturrock, S., et al. (2012). Geneious Basic: an integrated and extendable desktop software platform for the organization and analysis of sequence data. Bioinformatics 28, 1647–1649. doi: 10.1093/bioinformatics/bts199
Krishnamurthy, S. R., and Wang, D. (2017). Origins and challenges of viral dark matter. Virus Res. 239, 136–142. doi: 10.1016/j.virusres.2017.02.002
Kuang, W., Li, J., Zhang, S., and Long, L. (2015). Diversity and distribution of Actinobacteria associated with reef coral Porites lutea. Front. Microbiol. 6:1094. doi: 10.3389/fmicb.2015.01094
Langmead, B., and Salzberg, S. L. (2012). Fast gapped-read alignment with Bowtie 2. Nat. Methods 9, 357–359. doi: 10.1038/nmeth.1923
Li, H., Handsaker, B., Wysoker, A., Fennell, T., Ruan, J., Homer, N., et al. (2009). The sequence alignment/map format and SAMtools. Bioinformatics 25, 2078–2079. doi: 10.1093/bioinformatics/btp352
Lindell, D., Sullivan, M. B., Johnson, Z. I., Tolonen, A. C., Rohwer, F., and Chisholm, S. W. (2004). Transfer of photosynthesis genes to and from Prochlorococcus viruses. Proc. Natl. Acad. Sci. U.S.A. 101, 11013–11018. doi: 10.1073/pnas.0401526101
Lindström, E. S., Kamst-Van Agterveld, M. P., and Zwart, G. (2005). Distribution of typical freshwater bacterial groups is associated with pH, temperature, and lake water retention time. Appl. Environ. Microbiol. 71, 8201–8206. doi: 10.1128/AEM.71.12.8201-8206.2005
López-Bueno, A., Tamames, J., Velázquez, D., Moya, A., Quesada, A., and Alcamí, A. (2009). High diversity of the viral community from an Antarctic lake. Science 326, 858–861. doi: 10.1126/science.1179287
Lozupone, C., Lladser, M. E., Knights, D., Stombaugh, J., and Knight, R. (2011). UniFrac: an effective distance metric for microbial community comparison. ISME J. 5, 169–172. doi: 10.1038/ismej.2010.133
Magoč, T., and Salzberg, S. L. (2011). FLASH: fast length adjustment of short reads to improve genome assemblies. Bioinformatics 27, 2957–2963. doi: 10.1093/bioinformatics/btr507
Maranger, R., and Bird, D. F. (1995). Viral abundance in aquatic systems: a comparison between marine and fresh waters. Mar. Ecol. Prog. Ser. 121, 217–226. doi: 10.3354/meps121217
Marchler-Bauer, A., Lu, S., Anderson, J. B., Chitsaz, F., Derbyshire, M. K., Deweese-Scott, C., et al. (2011). CDD: a conserved domain database for the functional annotation of proteins. Nucleic Acids Res. 39, D225–D229. doi: 10.1093/nar/gkq1189
Masella, A. P., Bartram, A. K., Truszkowski, J. M., Brown, D. G., and Neufeld, J. D. (2012). PANDAseq: paired-end assembler for illumina sequences. BMC Bioinformatics 13:31. doi: 10.1186/1471-2105-13-31
McDaniel, L., Houchin, L., Williamson, S., and Paul, J. (2002). Plankton blooms: lysogeny in marine Synechococcus. Nature 415, 496–496. doi: 10.1038/415496a
McMurdie, P. J., and Holmes, S. (2013). phyloseq: an R package for reproducible interactive analysis and graphics of microbiome census data. PLoS One 8:e61217. doi: 10.1371/journal.pone.0061217
McMurdie, P. J., and Paulson, J. (2016). biomformat: An Interface Package for the BIOM File Format. Package version 1.0.0.
Mizuno, C. M., Rodriguez-Valera, F., Kimes, N. E., and Ghai, R. (2013). Expanding the marine virosphere using metagenomics. PLoS Genet. 9:e1003987. doi: 10.1371/journal.pgen.1003987
Mohiuddin, M., and Schellhorn, H. (2015). Spatial and temporal dynamics of virus occurrence in two freshwater lakes captured through metagenomic analysis. Front. Microbiol. 6:960. doi: 10.3389/fmicb.2015.00960
Newton, R. J., Jones, S. E., Eiler, A., Mcmahon, K. D., and Bertilsson, S. (2011). A guide to the natural history of freshwater lake bacteria. Microbiol. Mol. Biol. Rev. 75, 14–49. doi: 10.1128/MMBR.00028-10
Noguchi, H., Park, J., and Takagi, T. (2006). MetaGene: prokaryotic gene finding from environmental genome shotgun sequences. Nucleic Acids Res. 34, 5623–5630. doi: 10.1093/nar/gkl723
Nurk, S., Meleshko, D., Korobeynikov, A., and Pevzner, P. A. (2017). metaSPAdes: a new versatile metagenomic assembler. Genome Res. 27, 824–834. doi: 10.1101/gr.213959.116
Oksanen, J., Kindt, R., Legendre, P., O’hara, B., Stevens, M. H. H., Oksanen, M. J., et al. (2007). The vegan package. Commun. Ecol. Pack. 10, 631–637.
Ondov, B. D., Bergman, N. H., and Phillippy, A. M. (2011). Interactive metagenomic visualization in a Web browser. BMC Bioinformatics 12:385. doi: 10.1186/1471-2105-12-385
Ortega-Reig, M., Palau-Salvador, G. I., Sempere, M. J. C., Benitez-Buelga, J., Badiella, D., and Trawick, P. (2014). The integrated use of surface, ground and recycled waste water in adapting to drought in the traditional irrigation system of Valencia. Agric. Water Manage. 133, 55–64. doi: 10.1016/j.agwat.2013.11.004
Overbeek, R., Begley, T., Butler, R. M., Choudhuri, J. V., Chuang, H.-Y., Cohoon, M., et al. (2005). The subsystems approach to genome annotation and its use in the project to annotate 1000 genomes. Nucleic Acids Res. 33, 5691–5702. doi: 10.1093/nar/gki866
Palik, B., Batzer, D. P., Buech, R., Nichols, D., Cease, K., Egeland, L., et al. (2001). Seasonal pond characteristics across a chronosequence of adjacent forest ages in northern Minnesota, USA. Wetlands 21, 532–542. doi: 10.1672/0277-5212(2001)021[0532:SPCAAC]2.0.CO;2
Parsons, L. R., Sheikh, B., Holden, R., and York, D. W. (2010). Reclaimed water as an alternative water source for crop irrigation. Hortscience 45, 1626–1629. doi: 10.1021/acs.est.5b06256
Paulson, J. N., Pop, M., and Bravo, H. C. (2013a). metagenomeSeq: Statistical Analysis for Sparse High-throughput Sequencing. Bioconductor Package: 1.10.0. Available at: http://cbcb.umd.edu/software/metagenomeSeq
Paulson, J. N., Stine, O. C., Bravo, H. C., and Pop, M. (2013b). Differential abundance analysis for microbial marker-gene surveys. Nat. Methods 10, 1200–1202. doi: 10.1038/nmeth.2658
Ploner, A. (2012). Heatplus: Heatmaps with Row and/or Column Covariates and Colored Clusters. R package version 2.
Quast, C., Pruesse, E., Yilmaz, P., Gerken, J., Schweer, T., Yarza, P., et al. (2012). The SILVA ribosomal RNA gene database project: improved data processing and web-based tools. Nucleic Acids Res. 41, d590–d596. doi: 10.1093/nar/gks1219
Rieck, A., Herlemann, D. P., Jürgens, K., and Grossart, H.-P. (2015). Particle-associated differ from free-living bacteria in surface waters of the Baltic Sea. Front. Microbiol. 6:1297. doi: 10.3389/fmicb.2015.01297
Rohwer, F., and Thurber, R. V. (2009). Viruses manipulate the marine environment. Nature 459, 207–212. doi: 10.1038/nature08060
Roiha, T., Peura, S., Cusson, M., and Rautio, M. (2016). Allochthonous carbon is a major regulator to bacterial growth and community composition in subarctic freshwaters. Sci. Rep. 6:34456. doi: 10.1038/srep34456
Roux, S., Enault, F., Robin, A., Ravet, V., Personnic, S., Theil, S., et al. (2012). Assessing the diversity and specificity of two freshwater viral communities through metagenomics. PLoS One 7:e33641. doi: 10.1371/journal.pone.0033641
Sakamoto, T., and Bryant, D. A. (1997). Growth at low temperature causes nitrogen limitation in the cyanobacterium Synechococcus sp. PCC 7002. Arch. Microbiol. 169, 10–19. doi: 10.1007/s002030050535
Sakamoto, T., and Bryant, D. A. (1999). Nitrate transport and not photoinhibition limits growth of the freshwater cyanobacterium Synechococcus species PCC 6301 at low temperature. Plant Physiol. 119, 785–794. doi: 10.1104/pp.119.2.785
Saxton, M. A., Naqvi, N. S., Rahman, F., Thompson, C. P., Chambers, R. M., Kaste, J. M., et al. (2016). Site-specific environmental factors control bacterial and viral diversity in stormwater retention ponds. Aquat. Microb. Ecol. 77, 23–36. doi: 10.3354/ame01786
Scheierling, S. M., Loomis, J. B., and Young, R. A. (2006). Irrigation water demand: a meta-analysis of price elasticities. Water Resour. Res. 42:W01411. doi: 10.1029/2005WR004009
Schmidt, H. F., Sakowski, E. G., Williamson, S. J., Polson, S. W., and Wommack, K. (2014). Shotgun metagenomics indicates novel family A DNA polymerases predominate within marine virioplankton. ISME J. 8, 103–114. doi: 10.1038/ismej.2013.124
Šimek, K., Kasalický, V., Jezbera, J., Jezberová, J., Hejzlar, J., and Hahn, M. W. (2010). Broad habitat range of the phylogenetically narrow R-BT065 cluster, representing a core group of the betaproteobacterial genus Limnohabitans. Appl. Environ. Microbiol. 76, 631–639. doi: 10.1128/AEM.02203-09
Šimek, K., Kojecká, P., Nedoma, J., Hartman, P., Vrba, J., and Dolan, J. R. (1999). Shifts in bacterial community composition associated with different microzooplankton size fractions in a eutrophic reservoir. Limnol. Oceanogr. 44, 1634–1644. doi: 10.4319/lo.1999.44.7.1634
Skvortsov, T., De Leeuwe, C., Quinn, J. P., Mcgrath, J. W., Allen, C. C., Mcelarney, Y., et al. (2016). Metagenomic characterisation of the viral community of Lough Neagh, the largest freshwater lake in Ireland. PLoS One 11:e0150361. doi: 10.1371/journal.pone.0150361
Smith, M. W., Allen, L. Z., Allen, A. E., Herfort, L., and Simon, H. M. (2013). Contrasting genomic properties of free-living and particle-attached microbial assemblages within a coastal ecosystem. Front. Microbiol. 4:120. doi: 10.3389/fmicb.2013.00120
Søndergaard, M., Jeppesen, E., and Jensen, J. P. (2005). Pond or lake: does it make any difference? Arch. Hydrobiol. 162, 143–165. doi: 10.1127/0003-9136/2005/0162-0143
Suttle, C. A. (2007). Marine viruses–major players in the global ecosystem. Nat. Rev. Microbiol. 5, 801–812. doi: 10.1038/nrmicro1750
Suzek, B. E., Huang, H., Mcgarvey, P., Mazumder, R., and Wu, C. H. (2007). UniRef: comprehensive and non-redundant UniProt reference clusters. Bioinformatics 23, 1282–1288. doi: 10.1093/bioinformatics/btm098
Van der Gucht, K., Vandekerckhove, T., Vloemans, N., Cousin, S., Muylaert, K., Sabbe, K., et al. (2005). Characterization of bacterial communities in four freshwater lakes differing in nutrient load and food web structure. FEMS Microbiol. Ecol. 53, 205–220. doi: 10.1016/j.femsec.2004.12.006
Vavrek, M. J. (2011). Fossil: palaeoecological and palaeogeographical analysis tools. Palaeontol. Electron. 14:16.
Wagner, P. L., and Waldor, M. K. (2002). Bacteriophage control of bacterial virulence. Infect. Immun. 70, 3985–3993. doi: 10.1128/IAI.70.8.3985-3993.2002
Watkins, S. C., Kuehnle, N., Ruggeri, C. A., Malki, K., Bruder, K., Elayyan, J., et al. (2016). Assessment of a metaviromic dataset generated from nearshore Lake Michigan. Mar. Freshw. Res. 67, 1700–1708. doi: 10.1071/MF15172
Weinbauer, M. G., Hornák, K., Jezbera, J., Nedoma, J., Dolan, J. R., and Šimek, K. (2007). Synergistic and antagonistic effects of viral lysis and protistan grazing on bacterial biomass, production and diversity. Environ. Microbiol. 9, 777–788. doi: 10.1111/j.1462-2920.2006.01200.x
Wickham, H. (2009). ggplot2: Elegant Graphics for Data Analysis. Berlin: Springer Science & Business Media.
Wilhelm, S. W., and Matteson, A. R. (2008). Freshwater and marine virioplankton: a brief overview of commonalities and differences. Freshw. Biol. 53, 1076–1089. doi: 10.1111/j.1365-2427.2008.01980.x
Wilhelm, S. W., and Suttle, C. A. (1999). Viruses and nutrient cycles in the sea viruses play critical roles in the structure and function of aquatic food webs. Bioscience 49, 781–788. doi: 10.2307/1313569
Wommack, K. E., Nasko, D. J., Chopyk, J., and Sakowski, E. G. (2015). Counts and sequences, observations that continue to change our understanding of viruses in nature. J. Microbiol. 53, 181–192. doi: 10.1007/s12275-015-5068-6
Yannarell, A., Kent, A., Lauster, G., Kratz, T., and Triplett, E. (2003). Temporal patterns in bacterial communities in three temperate lakes of different trophic status. Microb. Ecol. 46, 391–405. doi: 10.1007/s00248-003-1008-9
Keywords: viral metagenomics, 16S rRNA, bacteriophage, polymerase A, microbiota, virome
Citation: Chopyk J, Allard S, Nasko DJ, Bui A, Mongodin EF and Sapkota AR (2018) Agricultural Freshwater Pond Supports Diverse and Dynamic Bacterial and Viral Populations. Front. Microbiol. 9:792. doi: 10.3389/fmicb.2018.00792
Received: 20 February 2018; Accepted: 09 April 2018;
Published: 24 April 2018.
Edited by:
George S. Bullerjahn, Bowling Green State University, United StatesReviewed by:
Hélène Montanié, University of La Rochelle, FrancePradeep Ram Angia Sriram, UMR6023 Laboratoire Microorganismes Génome et Environnement (LMGE), France
Copyright © 2018 Chopyk, Allard, Nasko, Bui, Mongodin and Sapkota. This is an open-access article distributed under the terms of the Creative Commons Attribution License (CC BY). The use, distribution or reproduction in other forums is permitted, provided the original author(s) and the copyright owner are credited and that the original publication in this journal is cited, in accordance with accepted academic practice. No use, distribution or reproduction is permitted which does not comply with these terms.
*Correspondence: Amy R. Sapkota, ars@umd.edu