- 1Department of Marine Sciences, University of Georgia, Athens, GA, United States
- 2Department of Microbiology, University of Georgia, Athens, GA, United States
We compared the composition of microbial communities obtained by sequencing 16S rRNA gene amplicons with taxonomy derived from metatranscriptomes from the same samples. Samples were collected from alkaline, hypersaline Mono Lake, California, USA at five depths that captured the major redox zones of the lake during the onset of meromixis. The prokaryotic community was dominated by bacteria from the phyla Proteobacteria, Firmicutes, and Bacteroidetes, while the picoeukaryotic chlorophyte Picocystis dominated the eukaryotes. Most (80%) of the abundant (>1% relative abundance) OTUs recovered as amplicons of 16S rRNA genes have been reported in previous surveys, indicating that Mono Lake's microbial community has remained stable over 12 years that have included periods of regular, annual overturn interspersed by episodes of prolonged meromixis that result in extremely reducing conditions in bottom water. Metatranscriptomic sequences binned predominately to the Gammaproteobacteria genera Thioalkalivibrio (4–13%) and Thioalkalimicrobium (0–14%); and to the Firmicutes genera Dethiobacter (0–5%) and Clostridium (1–4%), which were also abundant in the 16S rRNA gene amplicon libraries. This study provides insight into the taxonomic affiliations of transcriptionally active communities of the lake's water column under different redox conditions.
Introduction
Mono Lake, located in California, USA east of the Sierra Nevada Mountains on the western edge of the Great Basin, has been studied extensively due to its unusual chemistry and biology (reviewed in Melack et al., 2017). Mono Lake is an athalassic hypersaline (~90 g/L) and alkaline (pH ~9.8) terminal lake with geothermal inputs leading to elevated arsenic concentrations (~200 μM). Thermal stratification leads to the formation of a seasonal oxycline and to anoxic conditions in the lake's hypolimnion. The lake is generally monomictic, but undergoes periods of prolonged stratification (meromixis) following wet winters (Melack and Jellison, 1998). Microbial respiration below the oxycline leads to the reduction of arsenate to arsenite. Sulfide accumulation, especially in the monimolimnion, leads to the formation of thioarsenic compounds (Hollibaugh et al., 2005).
Several studies of the lake's biogeochemistry have focused on the role of microorganisms in arsenic geochemistry (reviewed in Oremland et al., 2004, 2017). These studies have used isolates (Switzer-Blum et al., 1998; Sorokin et al., 2002; Hoeft et al., 2004, 2007; Fisher and Hollibaugh, 2008), enrichment cultures (Hollibaugh et al., 2006; Budinoff and Hollibaugh, 2008; Fisher and Hollibaugh, 2008; Edwardson et al., 2014), and clone libraries of functional gene amplicons (Giri et al., 2004; Lin et al., 2005; Nercessian et al., 2005; Scholten et al., 2005; Lecleir et al., 2007). A more recent study (Edwardson and Hollibaugh, 2017) used metatranscriptomics to analyze microbial communities active in the As and S biogeochemical cycles in the lake. Other aspects of the biogeochemistry of Mono Lake that have been studied include ammonia and methane oxidation (Joye et al., 1999; Ward et al., 2000; Lin et al., 2005), sulfur cycling (Scholten et al., 2005; Hollibaugh et al., 2006), chitin degradation (Lecleir et al., 2004), and carbon fixation (Giri et al., 2004; Oremland et al., 2017).
The composition, diversity and distribution of microbes in aquatic communities are important ecological parameters, reflecting both functional aspects of these assemblages as well as the influence of environmental conditions on the survival and growth of specific organisms (Fuhrman et al., 2015). Analysis of 16S rRNA gene sequences has emerged as the method of choice for studying the composition of microbial communities (e.g., Fierer and Jackson, 2006; Sogin et al., 2006; Gilbert et al., 2012). Community composition has been studied in extreme environments, including Mono Lake, using libraries of cloned 16S rRNA gene amplicons (Humayoun et al., 2003; Foti et al., 2008; Mesbah et al., 2008; Antony et al., 2014), despite limited sequencing depth afforded by the necessity of cloning amplicons. The deeper coverage of amplicon libraries afforded by high-throughput sequencing provides a more detailed and complete analysis of microbial community composition and dynamics (Harris et al., 2013; Lanzén et al., 2013; Schneider et al., 2013; Yelton et al., 2013; Podell et al., 2014; Rascovan et al., 2016). Metagenomic surveys (e.g., Venter et al., 2004; Rusch et al., 2007) provide an alternative approach for assessing the abundance and taxonomic composition of microbial communities, while surveys of genes transcribed (mRNA) by microbial communities (metatranscriptomics, e.g., Poretsky et al., 2006; Gifford et al., 2011; Satinsky et al., 2014) allows identification of both the organisms and pathways that may be biogeochemically active in a given community.
Previous studies of the taxonomic composition (Humayoun et al., 2003) and environmental metatranscriptomics (Poretsky et al., 2005) of Mono Lake microbial communities were based on analysis of cloned amplicons sequenced by the dideoxy chain termination technique (Sanger et al., 1977), yielding limited sequencing depth. We were interested in comparing the composition of microbial communities in the lake at the onset of stratification in 2012 with an assessment of community composition made at the end of a 5-year period of meromixis (Humayoun et al., 2003). We were also interested in comparing the distribution of transcriptionally active microorganisms (Edwardson and Hollibaugh, 2017) along the lake's redox gradient with distributions of their genomes as reflected by the distribution of 16S rRNA genes in deeply sequenced libraries of PCR amplicons, and to evaluate changes in the apparent activity of specific organisms over the range of redox conditions found in the Mono Lake water column.
Materials and Methods
Field Site and Sampling
The samples used in this study were collected during summer (July 12 and 13, 2012, lake surface elevation: 1945 m) following a winter when the lake did not mix fully (the onset of meromixis). We sampled at Station 6 (37.964167° N,−119.022000° W), in the deepest basin of the lake, in order to capture the full redox gradient and to minimize the influence of breaking internal waves on vertical mixing (Vidal et al., 2013) and hence the distribution and activity of microbes. Duplicate samples were collected from depths of 10, 15, 18, 25, and 31 m to sample communities from the major redox zones of the lake (see Figure 1). Samples were taken as described previously (Hollibaugh et al., 2005; Edwardson and Hollibaugh, 2017). Briefly, vertical profiles of relevant environmental variables: photosynthetically active radiation (PAR, LiCor 2π quantum sensor, 400–700 nm, μE m−2 s−2), fluorescence (WetLabs fluorometer, relative fluorescence units), dissolved oxygen (SBE 43, mg L−1) and attenuation coefficient (WetLabs transmissometer, 600 nm wavelength light source, 10 cm path length, m−1) were obtained using sensors mounted on the frame holding a SeaBird SBE 19 CTD (conductivity, temperature, depth) recorder, modified and calibrated for use in Mono Lake. Water samples for total sulfide analysis (Cline, 1969) and arsenic speciation (Planer-Friedrich et al., 2007) were collected as described previously (Hollibaugh et al., 2005; Edwardson and Hollibaugh, 2017).
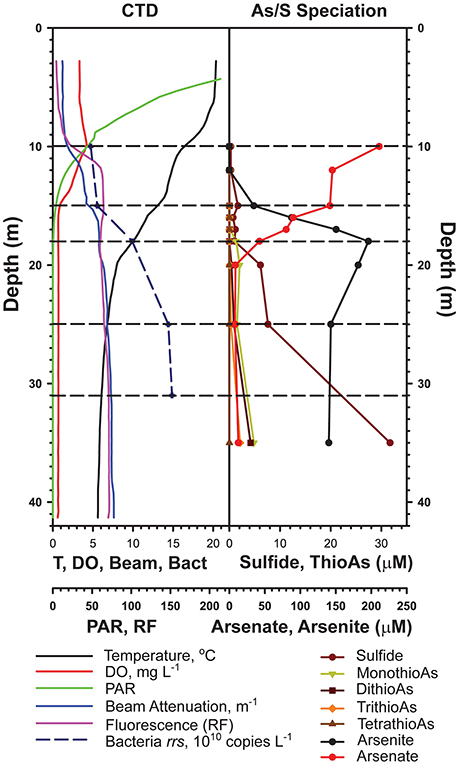
Figure 1. Water column profiles of physicochemical variables measured on July 12, 2012 (from Edwardson and Hollibaugh, 2017).
Nucleic Acid Sampling and Processing
Water for 16S rRNA gene analysis and synthesis of subtractive hybridization probes (see below) was collected from 5 L Niskin bottles into foil-wrapped polycarbonate bottles and stored in an insulated cooler on ice until processed further. Septum-capped bottles were filled to leave no head space and only one sample was taken from each depth. Water was filtered through Sterivex GV, 0.22 μm pore-size, cartridge filters (EMD Millipore, Billerica, MA) using a peristaltic pump within 8 h of collection. DNA was extracted from the filters using a lysozyme, proteinase K, sodium dodecyl sulfate digestion, followed by phenol-chloroform extraction as described previously (Kalanetra et al., 2009). Quantitative PCR (qPCR) of Bacteria 16S rRNA genes in this DNA was used to estimate the depth distribution of prokaryotes in the lake at the time of sampling (Kalanetra et al., 2009).
Samples for mRNA analysis were collected by pumping water from 31 m on July 12 and from 10 to 25 m on July 13. Duplicate samples of total particulate RNA (~0.5–2 L) were pumped sequentially from the sampling depth through 142 mm diameter, 0.2 μm pore-size Supor membrane filters. The filters were placed in 15 mL polypropylene centrifuge tubes and immediately frozen in liquid nitrogen, then transported to the laboratory where they were stored at −80°C until extracted. The elapsed time from beginning the filtration at a given depth until the filter was placed in liquid nitrogen was <15 min. Total RNA was extracted from particles retained by the filters using bead beating and RNEasy Mini Kits (Gifford et al., 2011; Edwardson and Hollibaugh, 2017), with internal standards added as described previously (Satinsky et al., 2013).
16S rRNA Gene Sequencing
We analyzed the distribution of 16S rRNA genes in each of our samples by sequencing libraries of PCR amplicons using 454 Pyrosequencing (Roche Diagnostics) technology (“pyrosequences” or “pyrosequenced libraries” hereinafter). We amplified the V4-V5 region of the 16S rRNA gene using primers 515F (Caporaso et al., 2011) and 907R (Armitage et al., 2012) that amplify both Bacteria and Archaea 16S rRNA genes. The forward primer also contained the Roche LIB-L Adapter A and a 10 nt MID tag on the 5′ end. A different MID tag was used for each depth (two samples per MID tag). The reverse primer contained the Roche LIB-L Adapter B on the 5′ end. PCR was performed in triplicate reactions for each sample using Q5® High-Fidelity polymerase (NEB). Each 25 μL reaction contained the following: Q5 Reaction Buffer (5 μL of 5X), 200 nM of each primer, 200 μM each dNTP and 0.02 U/μL of Q5 polymerase, and 1 μL (12–61 ng) DNA template. The PCR program used was: 98°C for 30 s, 25 cycles of 98°C for 10 s, 60°C for 10 s and 72°C for 10 s, with a final step of 72°C for 2 min. Individual PCR samples were run on a 1% agarose gel and single bands of the expected length were cut and extracted using a QiaQuick Spin kit (Qiagen). The triplicates were pooled and an additional cleanup step was performed with a QiaQuick Spin kit to concentrate them. A final cleanup was performed using the AmpureXP kit (Beckman Genomics). Products were quantified using the PicoGreen kit (Life Technologies) and pooled at an equivalent weight. Samples were sequenced on approximately 1/60th of a plate at the Georgia Genomics Facility using 454 Titanium® chemistry.
Metatranscriptomes
Ribosomal RNA was depleted from the RNA pool using subtractive hybridization probes (Stewart et al., 2010) synthesized from Mono Lake DNA collected as described above. Probes were synthesized from PCR products amplified using the primers described in Stewart et al. (2010), but with 25 μL reactions using Q5® High-Fidelity polymerase (NEB) following the manufacturers recommended reaction conditions and a modified amplification protocol: 98°C for 30 s, 30 cycles of 98°C for 10 s, annealing (Eub16S: 63°C, Eub23S: 55°C, Arch16S: 67°C, Arch23S: 64°C, Euk18S: 65°C, Euk28S: 61°C) for 30 s, 72°C for 40, 60, or 80 s (16/18S, 23S, and 28S reactions, respectively), and 72°C for 2 min. One PCR reaction was performed for each DNA replicate from each depth (n = 10) and all were pooled and purified with a QiaQuick PCR Cleanup kit (Qiagen) and concentrated using a SpeedVac 120 (Savant), then a second round of purification was performed with the AmpureXP kit (Beckman Genomics) to remove remaining primers and primer dimers. The Arch16S PCR did not yield a usable product and thus was excluded from probe synthesis. Ribosomal RNA-depleted samples were amplified using the MessageAmpII-Bacteria kit (Ambion). Double stranded cDNA was prepared using a combination of first and second strand kits: SuperScript III First Strand Synthesis (Life Technologies) primed with random hexamers, and NEBNext mRNA Second Strand synthesis module (NEB). Double stranded cDNA was purified with a PureLink PCR cleanup kit (Life Technologies) followed by ethanol precipitation. The cDNA was sheared (Covaris instrument) to a targeted ~225 bp insert size, and libraries were prepared using the TruSeq DNA (Illumina) kit with custom indices developed by the Georgia Genomics Facility at the University of Georgia. Samples were pooled and sequenced on one lane of an Illumina HiSeq2500 in Rapid Run mode with the 150PE (300 cycle) kit at HudsonAlpha Genomic Services Lab (Huntsville, AL).
Bioinformatics of 16S rRNA Sequences
Sequences were processed using QIIME versions 1.5 (initial sample processing and de-noising) and 1.8 (OTU picking and taxonomic assignment) (Caporaso et al., 2010). Samples were split and filtered using default quality control settings, with the additional removal of all reads with ambiguous bases (Huse et al., 2007) and all reads longer than 500 bp. Reads were de-noised using Denoiser (Reeder and Knight, 2010) and checked for chimeras using USEARCH 6.1 de novo chimera picking (UCHIME) (Edgar, 2010; Edgar et al., 2011). OTUs were picked using the open reference method with USEARCH 6.1 at a 97% identity cutoff. The SILVA rRNA database, release 111 (Quast et al., 2013), was used for reference-based OTU picking and for taxonomic assignment using UCLUST (Edgar, 2010). Additional taxonomic assignments for representative sequences of OTUs that remained “unassigned” after this step were made using SINA (Pruesse et al., 2012) against SILVA release 119, or using the RDP classifier (Wang et al., 2007). The QIIME taxonomic assignments of a number of sequences were compared to SINA taxonomic assignments to verify of their QIIME assignments. Chloroplast, mitochondria, and singleton OTUs, as well as OTUs that failed to align with the SILVA 16S reference alignment were removed. Representative sequences of each OTU were used to search against the NCBI nr database using BLASTN (Altschul et al., 1990).
Sequences analyzed in a previous study of the microbial diversity of Mono Lake by Humayoun et al. (2003), who sampled different depths from the same redox zones at Station 6 (2 m, aerobic; 17.5 m, microaerophilic, 23 m, anoxic; and 35 m, sulfidic, see Humayoun et al., 2003), were downloaded from NCBI GenBank (n = 274). All sequences that spanned the region amplified by the 16S rRNA gene primers we used here (515F/907R) were aligned in Geneious and trimmed to the length of the pyrosequenced amplicons (~375 nt, n = 174). These sequences were processed in QIIME as described above to determine OTUs at 97% identity and to define the taxonomy of sequences from the Humayoun et al. (2003) study in the same manner as our pyrosequences. We retained singletons (n = 48) in this analysis due to the small size of the Humayoun et al. (2003) database. The number of sequences assigned to each OTU was determined manually. We also included sequences from other stations and depths sampled during that same study that were available in GenBank but not analyzed by Humayoun et al. (2003). Relative abundances of each OTU were determined for each depth at Station 6. Alpha and beta diversity analyses were performed on the 454 amplicons using the R package phyloseq (McMurdie and Holmes, 2013). Reads were randomly subsampled to the lowest number of reads per sample (1,588) prior to alpha and beta diversity analysis. Raw reads were deposited in the NCBI SRA under accession number SRP074130 (BioProject PRJNA319794).
Bioinformatics Analysis of Metatranscriptomes
FASTQ files were paired using PEAR (version 0.9.2; Zhang et al., 2014) with a minimum overlap of 1 and no statistical tests. PRINSEQ (version 0.20.3; Schmieder and Edwards, 2011) was used to trim and perform quality control using the following parameters: 10–90% GC content, minimum length 35 bp, mean quality score of 20, trim from 3′ to 5′ ends with a sliding scale window of 3 and step of 1 with a minimum mean quality score of 20, trim >5 uncalled bases from ends, trim >5 A/T tails, and allow only 1 uncalled base (any reads with uncalled bases were later removed). RiboPicker (version 0.4.3; Schmieder et al., 2012) was used to identify rRNA reads in metatranscriptome libraries. The default parameters (50% coverage, 75% identity, 30 base align length, BWA-SW Z-best value of 3) and the non-redundant rRNA database were used. FASTQ files were converted to FASTA files using FASTX-Toolkit (http://hannonlab.cshl.edu/fastx_toolkit/) default settings, which removes any reads that contain uncalled bases.
A custom BLAST database containing sequences of internal standards provided by Satinsky and Moran (Satinsky et al., 2014) was created using BLAST+ (Camacho et al., 2009). A BLASTN (Altschul et al., 1990) search against the custom database was used to count reads assigned to internal standards. Recovery was calculated using counts of hits to sequences of standards with bit scores >50, divided by the number of internal standard sequences added to each sample (Satinsky et al., 2013). These sequences were then removed from the input FASTA files using a QIIME script (filter_fasta.py; Caporaso et al., 2010). A local RapSearch2 (Zhao et al., 2012) database of all protein sequences in the RefSeq (Tatusova et al., 2014) database (release 64) was downloaded from NCBI. Rapsearch2 was used to annotate metatranscriptome reads using an e-value cutoff of 10−5, keeping only the top hit. Further processing removed all hits with bit scores <40 (Gifford et al., 2011). Custom scripts were used to fully annotate and assign taxonomy to hits. Absolute abundances (transcripts/L) of transcripts for each unique gene (locus_tag) were calculated by multiplying count of hits to that locus_tag in each library by the factor determined from recovery of internal standard reads, divided by volume filtered for each sample. Relative abundance of each unique transcript (locus_tag) in each library (% of all transcripts in that library) was calculated as the absolute abundance of that transcript divided by the total number of transcripts in that library. We used averages of these relative abundances for the 2 libraries from each depth in subsequent comparisons.
We performed an additional screening step to focus our analysis confidently on protein-coding transcripts. Hits to records with RefSeq annotations that contained the terms “hypothetical” or “putative” were removed from the transcript data set manually using text searches. This filter likely removed hits to some transcripts that encode valid proteins whose function is either not known or that are annotated incorrectly, but it also removed incorrect assignments to non-protein encoding transcripts (Tripp et al., 2011). Disproportionally frequent hits to “cell wall hydrolases” were also found in the dataset. In one case (library 31A), the top hit to this annotation accounted for 11% of all Bacteria transcripts, whereas the largest bin for sequences that did not contain this term only accounted for 2% of the transcripts in the library. We analyzed each of the hits that made up >1% of “hypothetical/cell wall hydrolases” further using a TBLASTN search against the nr/nt nucleotide database to determine the identity of the best hit to the RefSeq protein database. This analysis (not shown) revealed misannotated ribosomal RNAs, small RNAs or ribozymes, as has been reported previously (Tripp et al., 2011). Thus, annotations containing the following words or phrases: “hydrolase,” “predicted protein,” “uncharacterized protein,” and “cell wall-associated hydrolase” were deemed to be inaccurate and hits to them were removed from the data set. Reads are deposited in the NCBI SRA under accession number SRP068308 (BioProject PRJNA308451).
Phylogenetic Analysis
The phylogeny of the 16S rRNA gene sequences obtained from pyrosequencing was compared with phylogeny from the sequences obtained by Sanger-sequencing cloned amplicons from Humayoun et al. (2003) as follows. Representative sequences from all OTUs that accounted for >1% relative abundance in the pyrosequence database (n = 34) were aligned with OTUs with >1% relative abundance in the Sanger sequence database (n = 60), and with 16S rRNA genes retrieved from genomes corresponding to taxa that represented >1% relative abundance in the metatranscriptomic dataset. All sequences were trimmed to the length of the pyrosequenced reads (~375 bp) and aligned using the SINA aligner (Pruesse et al., 2012). Alignments were imported into Geneious 8 (Kearse et al., 2012), and neighbor-joining consensus trees (Jukes-Cantor distances) with 100 bootstrap replicates were built, with Halobacterium salinarum as the outgroup. Three separate trees (Proteobacteria, Firmicutes, and Other Phyla) were constructed in this manner.
Results
Chemical Characteristics of Mono Lake
Mono Lake Station 6, the site of many previous microbiological studies of the lake, was sampled at five discrete depths chosen based on the chemical profile of the lake at the time of sampling (Edwardson and Hollibaugh, 2017, Figure 1). The epilimnion is characterized by the highest temperatures (>15°C), highest light, highest dissolved oxygen concentrations, and is subject to intense grazing by brine shrimp, Artemia monica (Jellison and Melack, 1993). We sampled at the base of the epilimnion (10 m), the base of the oxycline (15 m), and near the base of the thermocline (18 m). The dissolved oxygen concentration was 0.83 mg L−1 at 15 m, and decreased to the instrument's limit of detection at 15.8 m (0.68 mg L−1), thus the 15 m sample is considered to be suboxic. We sampled the anoxic hypolimnion at 25 and 31 m, sulfide was present at 31 m.
Community Profiling by 16S rRNA Analysis
We obtained between 4,137 and 16,913 high quality 16S rRNA gene reads from each pyrosequenced library from each depth (Table 1). Chloroplast sequences accounted for 5–57% of the reads (Table 1) and made up an increasing proportion of the reads as depth increased. Chloroplast sequences were dominated (>99%) by one OTU, which was ≥99% similar to the 16S rRNA gene sequence from Picocystis salinarum CCMP1897 chloroplasts and identical to a plastid sequence retrieved from Mono Lake by Humayoun et al. (2003).
We obtained a combined total of 238 OTUs (236 Bacteria, 2 Archaea) from all samples and all depths. The distribution by depth of these OTUs, with read counts and relative abundance is presented Table S1, while their full taxonomy is presented in Table S2. Community richness and inverse Simpson diversity were lowest at 10 m, increased at 15–25 m, then decreased at 31 m (Figure S1). Weighted UniFrac analysis of beta diversity (Hamady et al., 2009) showed that the communities were structured by depth, with the 10 and 31 m samples most dissimilar from the others, and the 15, 18, 25, and 31 m samples structured along a gradient with the two MDS axes representing 94.8% of the variation (Figure S1). Proteobacteria (27–40%), Bacteroidetes (30–33%), Firmicutes (1–28%), and Actinobacteria (4–15%) were the most abundant phyla, and only one other phylum (Spirochaetes, 1–4%) made up more than 1% of the population at any depth (Figure 2).
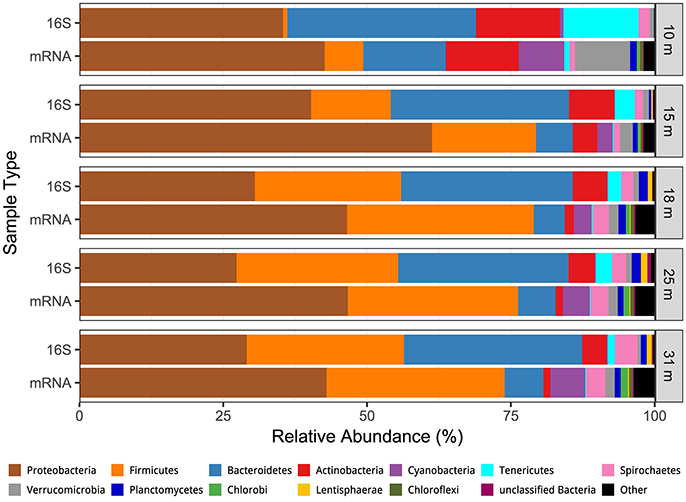
Figure 2. Comparison of the depth distribution of transcriptionally active microbial phyla (mRNA) the depth distribution of phyla derived from 16S rRNA genes.
Forty of the 238 observed OTUs were present at all five depths with 34 OTUs greater than 1% relative abundance at any depth, and 10 OTUs greater than 1% relative abundance at all depths (Table 2). These core OTUs made up 60–74% of the overall microbial community at all depths. The most abundant OTU (OTU 8, 5.9–19.9% relative abundance, Table 2) was classified as a Bacteroidetes (class Cytophagia) related to clone ML602J-37, which was retrieved from Mono Lake by Humayoun et al. (2003). Another Cytophagia (represented by clone ML310M-34) was also present at all depths (1.1–7.7%), but was more abundant in samples from below the oxycline. Five additional core OTUs included two Actinobacteria (Microbacteriaceae, 1.8–4.0%; Nitriliruptor, 2.0–8.7%), an Alphaproteobacteria (unclassified Rhodobacteraceae, 4.3–6.8%), a Gammaproteobacteria (Marinicella, 1.4–5.6%), and Spirochaeta (1.3–2.7%).
All but three of these OTUs (ML2012 OTUs 80, 150, and 166) were most similar to sequences retrieved from Mono Lake. Additionally, many of the taxonomic assignments were to taxonomic groups represented in the SILVA database by sequences retrieved from Mono Lake (e.g., ML60J37, ML635J-40 aquatic group, ML-A-10; Table 2), suggesting the presence in Mono Lake of organisms representing previously undescribed genera (and in some cases higher taxonomic levels).
Transcriptionally Active Taxa
Between 8 and 18 million paired reads were obtained per library, with an average read length of ~240 nt (See Edwardson and Hollibaugh, 2017). At the domain level 68% of the cDNA sequences were affiliated with Bacteria, 30.4% with Eukarya, 0.6% with Archaea, and 0.8% with Viruses. Forty-five to fifty-nine percent of the Bacteria hits to RefSeq proteins were for hypothetical proteins or were misannotations that were subsequently removed from the analysis (see Materials and Methods and Edwardson and Hollibaugh, 2017). Figure 2 compares the phylum-level composition of the transcriptionally active microbial community with the composition determined from analysis of 16S rRNA gene sequences. Sequences from Proteobacteria accounted for 43–61% of the cDNA sequences in libraries from all depths. Firmicutes (7–32% of transcripts) and Bacteroidetes (5–14% of transcripts) were the next most abundant transcriptionally active phyla. Bacteroidetes transcripts were more abundant at 10 m than at other depths, and Firmicutes transcripts were abundant in samples from the anoxic water column (15–31 m). Actinobacteria transcripts were abundant (14%) at 10 m but less abundant at anoxic depths.
Transcript abundance ranged from ~0.25 to ~1.5 trillion transcripts per liter, on average, at each depth, increasing with depth (Figure S2). Closer examination of the differences in relative abundance derived from 16S gene OTUs vs. transcripts (Figure 3) reveal the greatest differences within the Bacteroidetes and Proteobacteria phyla. In general, the Bacteroidetes are relatively more abundant in the 16S OTU dataset than in the metatranscriptome dataset. The opposite is true for Proteobacteria. The greatest differences at the class level are for the Cytophagia and Bacteroidia classes in the Bacteroidetes (more abundant in 16S OTUs) and in the Delta- and Gammaproteobacteria (more abundant as OTU's derived from transcripts).
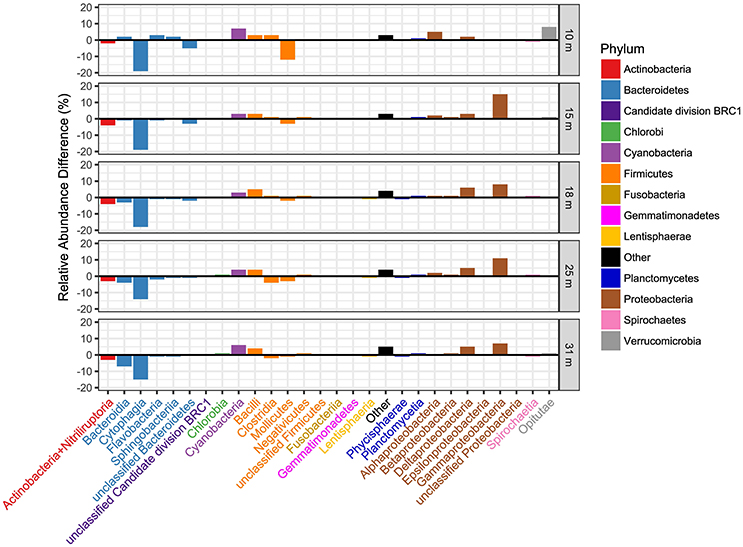
Figure 3. Comparison of the distribution of transcriptionally active taxa with taxa determined from 16S rRNA gene sequences at the taxonomic level of class and by sample depth. The relative abundance of OTUs derived from 16S rRNA was subtracted from the relative abundance of the same OTUs derived from transcript hits, thus positive bars indicate dominance of that class in transcript bins. Class names are colored by phylum as indicated in the legend.
We determined the contribution of abundant (>1% of the transcript pool) genera to the metatranscriptome at each depth (Table 3). The genus-level bins that contained the most transcripts in samples from 10 m include Spiribacter (4%) and Thioalkalivibrio (4%), two Gammaproteobacteria in the Ectothiorhodospiraceae family. Other abundant transcriptionally active taxa from 10 m include the cyanobacteria Cyanobium (3%) and Synechococcus (2%), as well as Actinobacteria, Bacterioidetes, and Verrucomicrobia. Transcripts from the genus Thioalkalivibrio, a haloalkaliphilic Gammaproteobacteria (Sorokin et al., 2001), were abundant at all depths below 15 m. Transcripts from Thioalkalimicrobium, another haloalkaliphilic Gammaproteobacteria, were the most abundant bin at 15 m (14% of the libraries). These two taxa accounted for 27% of the transcripts at 15 m. Clostridium species were more abundant at depths ≥15 m than at shallower depths, and we observed an increase in the abundance of transcripts from the genus Dethiobacter, a sulfide-oxidizing denitrifier in the order Clostridiales (Sorokin et al., 2008; Thorup et al., 2017), at 18 m. At this depth, transcripts assigned to Dethiobacter comprised the third largest taxonomic bin following bins for the chemolithotrophic sulfur oxidizers Thioalkalivibrio and Thioalkalimicrobium, both 9%. We also saw an increase from 18 to 31 m in the abundance of transcripts from known sulfate-reducing genera of the Deltaproteobacteria. Transcripts related to obligate anaerobic Firmicutes and Spirochetes taxa increased in abundance at 25 and 31 m, and a decrease in the abundance of transcripts related to sulfur oxidizing bacteria was seen at these depths as well.
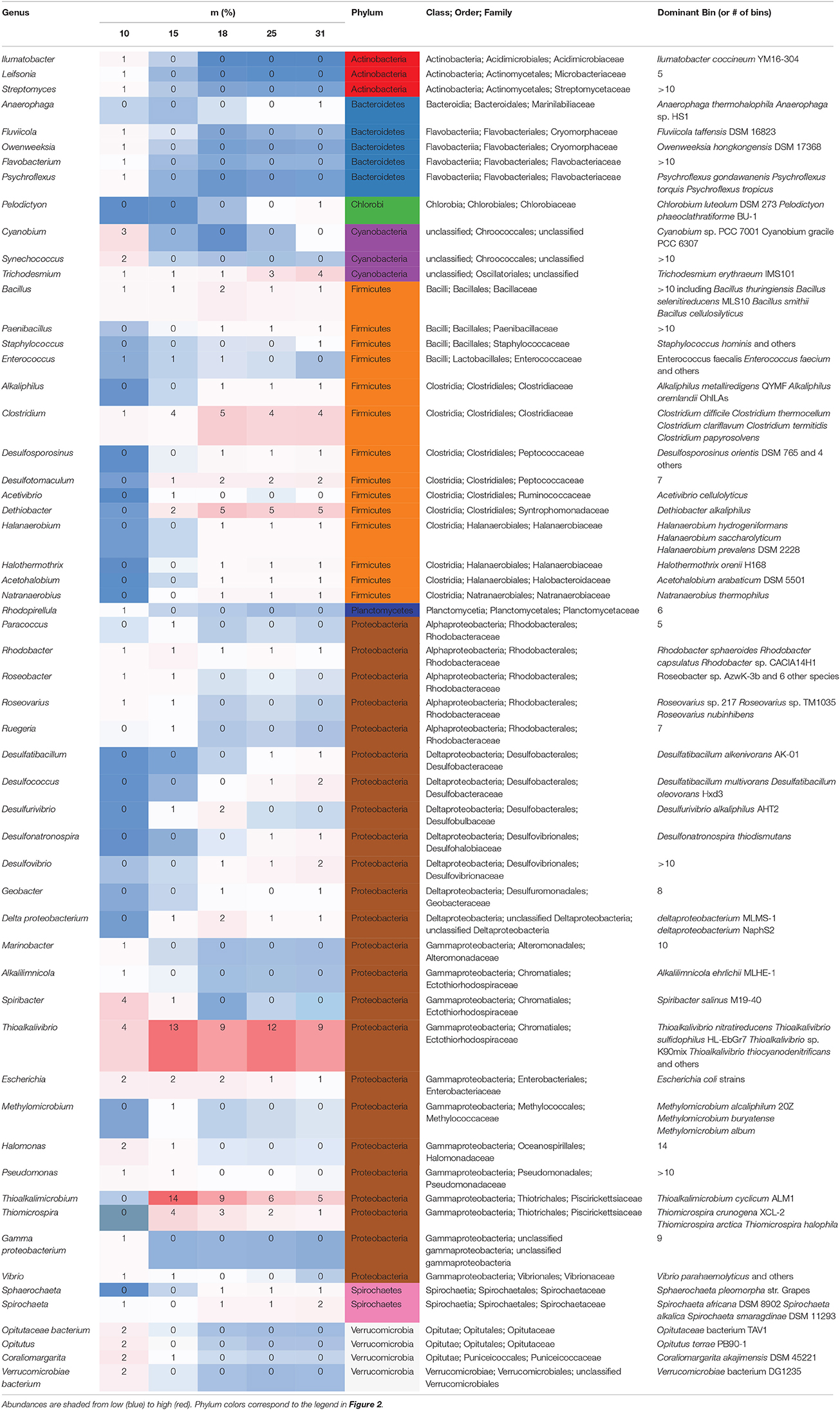
Table 3. Genera >1% relative abundance (rounded) in metatranscriptomes at any depth with classification and top genome bins.
Phylogenetic Analysis
The top BLASTN hits to the NCBI nr database of representative sequences from pyrosequenced OTUs were used as reference sequences in phylogenetic trees (Figures 4–6). Eleven OTUs from pyrosequenced libraries were identical to OTUs from the Humayoun et al. (2003) Sanger-sequenced libraries. The relative abundances of OTUs in the Humayoun et al. (2003) libraries, the pyrosequenced libraries, and OTUs derived from taxonomic assignments of metatranscriptomic sequences were similar; however, there were large discrepancies in a few cases (Figure 3). The relative abundance of Actinobacteria OTU 83 was only 3% in pyrosequenced libraries from 18 m, whereas the most closely related OTU in the 17.5 m sample from the Humayoun et al. (2003) dataset accounted for almost 40% of all sequences in our 18 m sample. In addition, our pyrosequenced libraries contained no OTUs corresponding to a group of Firmicutes OTUs from 23 and 35 m in Humayoun et al. (2003). Sequences from this group were not closely related to any 16S rRNA gene reference sequence, with the closest hit being to Dethiobacter alkaliphilus at ~90% identity. In fact, the abundant OTUs within the Firmicutes (and Tenericutes, which group phylogenetically within the Firmicutes) from pyrosequenced libraries were only 87–96% similar to 16S reference sequences currently in the NCBI database. In contrast, all of the Proteobacteria OTUs from our pyrosequenced libraries (Figure 4), had ≥97% identity to a sequence in the NCBI database. Some of the OTUs (Dethiobacter OTU 36, Paenibacillaceae OTUs 155 and 64, Desulfurivibrio OTU 20) that we obtained were most closely related to sequences obtained from sulfide-oxidizing, arsenate-reducing enrichment cultures raised from Mono Lake water (Hollibaugh et al., 2006), rather than to sequences retrieved directly from a water column sample. The other major OTUs in the pyrosequence database consisted of Verrucomicrobia, Actinobacteria, Bacteroidetes (Flavobacteria, Chitinophaga), Spirochetes, Cyanobacteria, and Planctomycetes (Figure 6). None of the OTUs we obtained by pyrosequencing were related to the Cyanobacteria or Chlorobium OTUs present in metatranscriptome taxonomic bins. This could be due to specificities of the primers we used to amplify 16S rRNA genes.
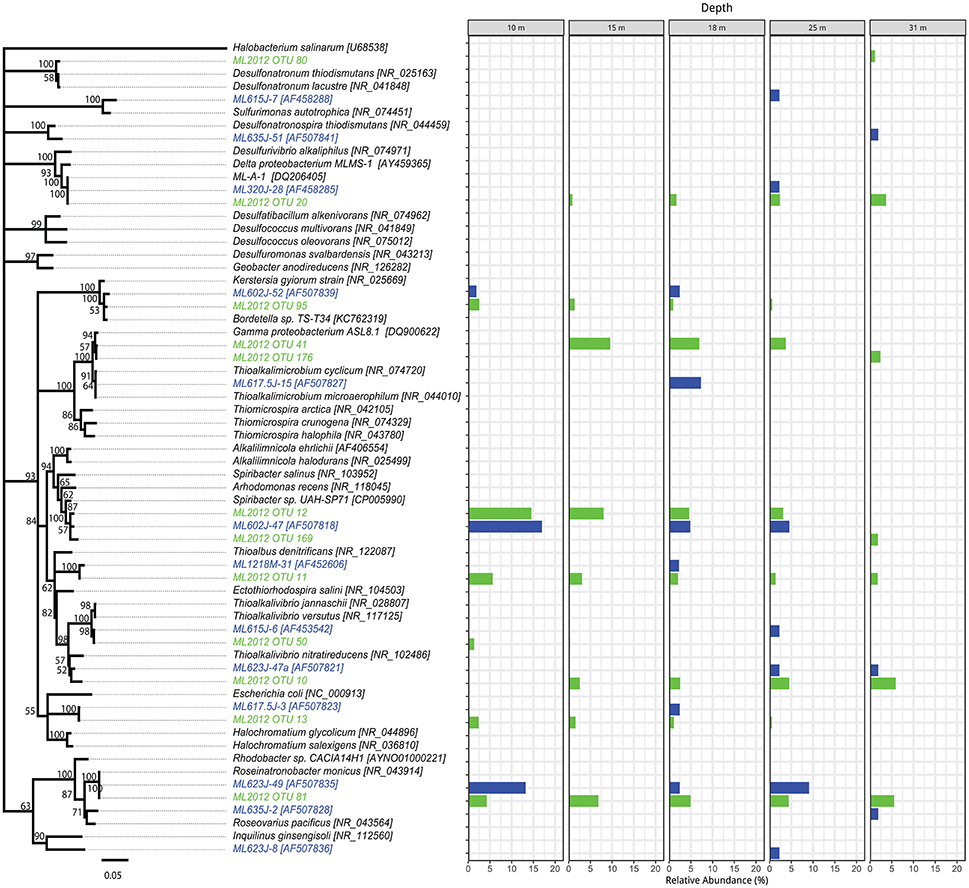
Figure 4. Phylogenetic tree showing the relative abundance of Proteobacteria OTUs by depth. Taxa and bars shown in green represent 16S rRNA OTUs derived from tag pyrosequencing. Taxa and bars shown in blue represent 16S rRNA OTUs derived from sequences of cloned amplicons reported in Humayoun et al. (2003). Taxa shown in black represent 16S rRNA gene reference sequences for bins accounting for >1% relative abundance in the metatranscriptome from that depth (both samples combined). The outgroup is Halobacterium salinarum.
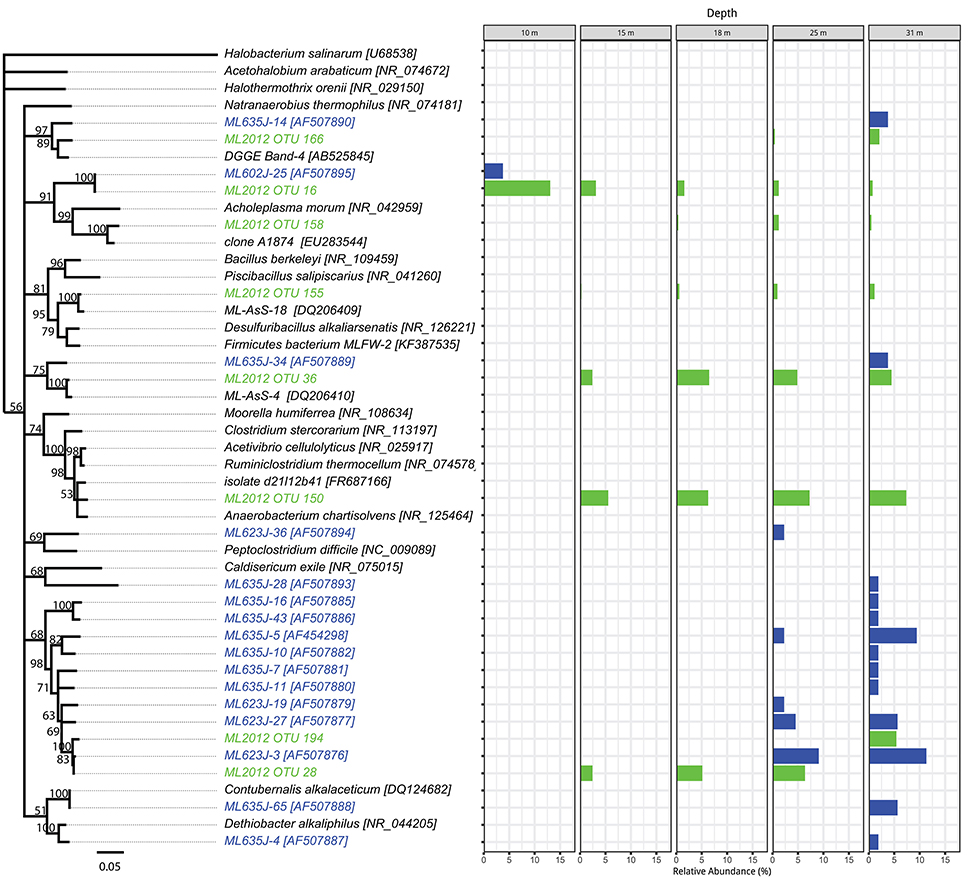
Figure 5. Phylogenetic tree showing the relative abundance of Firmicutes OTUs by depth. Taxa and bars shown in green represent 16S rRNA OTUs derived from tag pyrosequencing. Taxa and bars shown in blue represent 16S rRNA OTUs derived from sequences of cloned amplicons reported in Humayoun et al. (2003). Taxa shown in black represent 16S rRNA reference sequences for bins accounting for >1% relative abundance in the metatranscriptome from that depth (both samples combined). The outgroup is Halobacterium salinarum.
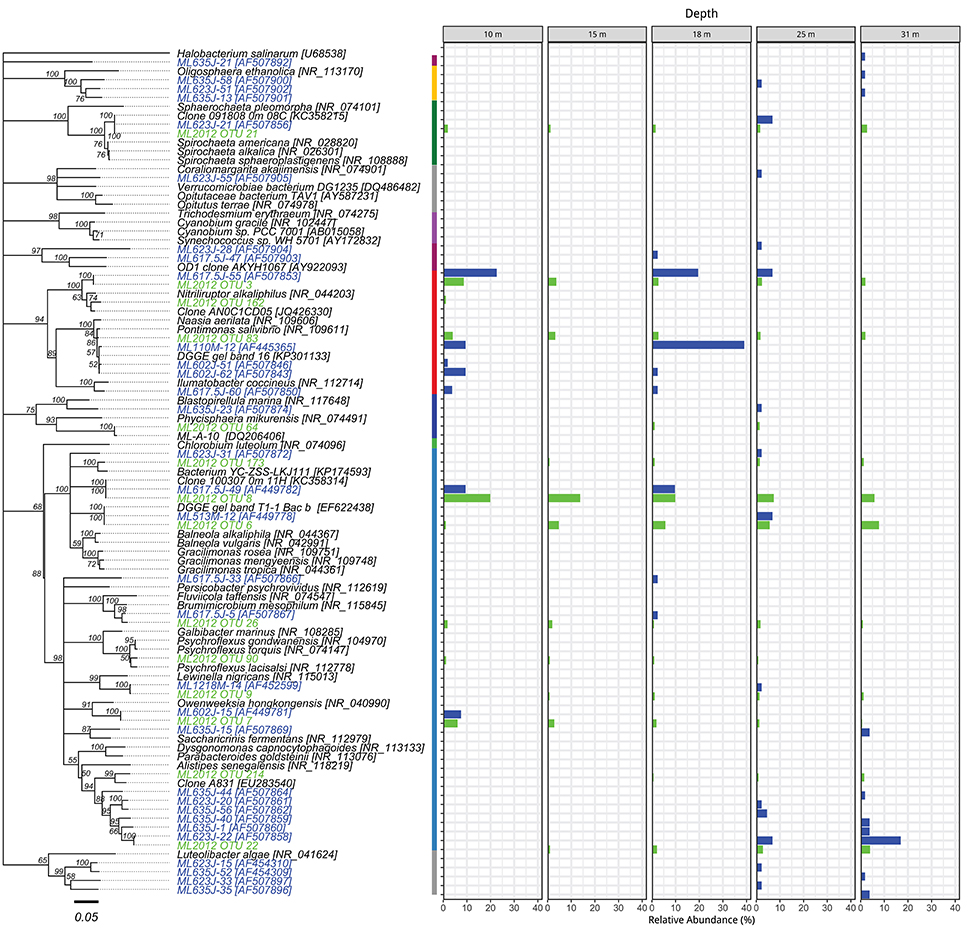
Figure 6. Phylogenetic tree showing the relative abundance of OTUs from Actinobacteria, Bacteroidetes, and other bacteria by depth. The colored segments of the vertical bar between the tree and the charts indicates the phylum associated with the adjacent branch of the tree, colors correspond to the legend of Figure 2. Taxa and bars shown in green represent 16S rRNA OTUs derived from tag pyrosequencing. Taxa and bars shown in blue represent 16S rRNA OTUs derived from sequences of cloned amplicons reported in Humayoun et al. (2003). Taxa shown in black represent 16S rRNA gene reference sequences for bins accounting for >1% relative abundance in the metatranscriptome from that depth (both samples combined). The outgroup is Halobacterium salinarum.
Discussion
Unlike a previous study of the phylogenetic composition of Mono Lake microbial communities (Humayoun et al., 2003), we found a set of OTUs that were present at all depths in the lake, possibly as a result of sampling at the beginning, vs. the end, of a period of meromixis, although the low sequencing depth of the previous analysis could have led to missing these taxa. The relative abundance of these OTUs did not vary greatly between depths. In some cases, their relative abundance was greater than their relative abundance in metatranscriptomic bins. This could indicate presence of inactive or dormant cells (e.g., spores) or relic DNA (Carini et al., 2016). Seven OTUs made up 23–45% of the community at all depths. These include two Cytophagia OTUs (12–21% relative abundance) most closely related (90–93% identity) to Gracilimonas (a facultative aerobe) and Balneola (aerobic) species (Urios et al., 2008; Choi et al., 2009). An Alphaproteobacteria (Rhodobacteraceae) OTU (4–7% relative abundance) was 99% similar to purple non-sulfur Roseinatronobacter monicus isolated from Mono Lake (Boldareva et al., 2007). This species is an obligate aerobe. Related Rhodobaca species can grow under anaerobic conditions if illuminated, thus the persistence of these OTUs just below the oxycline might be a consequence of photoheterotrophic “maintenance” or, alternatively, the organism might be associated with microaerophilic conditions maintained by oxygenic photosynthesis of Picocystis, which is abundant at this depth. However, it is unlikely that irradiance is adequate to support photoheterotrophy or oxygenic photosynthesis below the chemocline (Figure 1). Recovery of this OTU from those depths may be due to the presence of DNA from cells in a stationary phase, or it is a relic (Carini et al., 2016) left over from the last deep mixing event, or to vertical transport of cells associated with sinking particles.
An OTU related to Nitriliruptor species (Actinobacteria) contributed 4–7% of the population at all depths. The most closely related strain is the aerobe Nitriliruptor alkaliphilus, an isobutyronitrile-degrading haloalkaliphile isolated from enrichment cultures inoculated with water from Soda Lakes (Sorokin et al., 2009). The genome of Nitriliruptor alkaliphilus was released after we analyzed our metatranscriptomes; however, if this genome had been included in the analysis it is likely that it would have recruited hits. This observation serves to underline the taxonomic biases of the approaches we tested (metagenomics/metatranscriptomics vs. amplicon sequencing) and sequence databases (a broader 16S rRNA reference database vs. a narrower reference database of sequenced genomes).
Spiribacter has been found previously in moderately halophilic environments and is a strict aerobe, which could indicate why it was only found at oxic (10 m) and suboxic (15 m) depths in Mono Lake. Thioalkalivibrio has been isolated previously from Soda Lakes, including Mono Lake (Sorokin et al., 2001, 2002). A Cyanobium strain was isolated from Mono Lake samples and characterized, but counts by epifluorescence microscopy showed that cells were more abundant in samples from aphotic depths than nearer the surface (Budinoff and Hollibaugh, 2007). However, we found more transcripts of this organism at 10 m than at depth, supporting the hypothesis that the Cyanobium cells found in the aphotic zone are inactive or represent a flux sinking from the euphotic zone of the lake.
Soda lakes typically contain an abundance of diverse reduced sulfur compounds and one of the major processes in soda lake biogeochemistry is sulfur cycling (Sorokin, 2011). Sulfur cycling microbial taxa from soda lakes are well-represented by cultured isolates (Sorokin et al., 2013). As expected, OTUs representing many sulfur-oxidizing bacteria were found in both the pyrosequenced 16S rRNA gene libraries and in taxonomic bins generated from metatranscriptomic samples collected at 15–31 m.
Thioalkalimicrobium (OTU 41) and Thioalkalivibrio (OTUs 10 and 50) made up 5–12% of the OTUs in pyrosequenced libraries and 12–24% of the OTUs derived from metatranscriptomic taxonomic bins in these samples. The abundance of Thioalkalimicrobium decreased with depth but Thioalkalivibrio abundance increased in anoxic water below 15 m. Both taxa should have decreased in abundance with depth, as they are both believed to be aerobic sulfur oxidizers (Sorokin et al., 2002). Although some Thioalkalivibrio have the ability to use alternative terminal electron acceptors such as nitrate (Sorokin, 2011), we did not find any evidence that this process was occurring (e.g., transcripts of nitrate reductase) in the metatranscriptomes (data not shown). The likely role of these two organisms as the dominant sulfur-oxidizing bacteria in Mono Lake was not surprising, as both Thioalkalivibrio jannaschii and Thioalkalimicrobium cyclicum were isolated from Mono Lake (Sorokin et al., 2002). In addition, Dethiobacter alkaliphilus, the most abundant metatranscriptomic taxonomic bin in the Firmicutes phylum, is a sulfide-oxidizing denitrifier (Sorokin et al., 2008; Thorup et al., 2017).
Many different sulfate-reducing bacteria (various Deltaproteobacteria and OTU 20) appear between 18 and 31 m. This was expected as sulfate reduction rates are higher at depth in the lake, particularly when it is strongly stratified (Oremland et al., 2000).
Previous work on nitrogen cycling in Mono Lake (Carini and Joye, 2008) identified a peak in ammonia oxidation rates at 12–14 m, coinciding with the presence of ammonia-oxidizing bacteria of the genus Nitrosomonas. We found few (<1% relative abundance) OTUs related to ammonia-oxidizing Bacteria or Archaea in either our pyrosequenced libraries or in taxonomic bins derived from metatranscriptomes. Moreover, metatranscriptomes contained little evidence for the presence of ammonia-oxidizing Archaea. Transcripts of genes from ammonia-oxidizing bacteria such as Nitrosomonas, which was identified as the dominant taxon of ammonia-oxidizing bacteria by Carini and Joye (2008), were in low abundance, and we found no OTUs representing other known nitrifying bacteria in the pyrosequenced libraries. One possible explanation for the activity Carini and Joye (2008) observed is the presence in Mono Lake of methanotrophs (e.g., Methylomicrobium and Methyloglobus, Nercessian et al., 2005), which were also present in the metatranscriptomes. Some methane-oxidizers can also oxidize ammonia (Nyerges and Stein, 2009).
We observed increasing relative abundance with depth (1–4%) of transcripts that were identified initially as the cyanobacteria Trichodesmium. We assembled these transcripts using the Geneious assembler with default settings to analyze them further. The consensus sequences obtained were searched against contigs from the Mono Lake Picocystis draft genome (C. Saltikov, unpublished) using BLASTN. Approximately 90% of the hits were ≥97% identical and 25% (278/1,180) were 100% identical to Picocystis. Further, these hits were to a single Picocystis consensus sequence of 87,248 bp. This sequence is 86% similar (nucleotide identity) to the Picocystis salinarum chloroplast from San Francisco Bay (Lemieux et al., 2014), indicating that the contig was likely derived from Picocystis chloroplasts. Interestingly, a Trichodesmium-related Oscillatoriales species, Phormidium, which was identified in nitrogen-fixing aggregates in Mono Lake (Oremland, 1990), was not detected in our samples, likely because it grows attached to solid substrates at the lake's edge and floats at the lake's surface when detached.
Diversity Relative to Previous Studies and Other Soda Lakes
Mono Lake microbial communities seem to have remained stable, or returned to the same composition, after multiple mixing events over the 12 years between sampling efforts, despite the significant physicochemical differences in the lake following prolonged meromixis when sampled in 2000 vs. 2012. A number of OTUs that were abundant in the Humayoun et al. (2003) study were not represented by any OTUs in our pyrosequencing dataset. Some of the differences between these studies can be attributed to sequencing depth and primer biases, but Humayoun et al. (2003) determined the composition of the Mono Lake microbial community after 5 years of stratification. In contrast, our sampling effort occurred after only ~18 months of stratification (only 1 winter of partial mixing). The major ion composition and pH of Mono Lake are stable (Domagalski et al., 1989), thus the main physiochemical differences in the lake between July 2000 when Humayoun et al. (2003) sampled and July 2012 when we sampled was higher hypolimnion concentrations of reduced species like sulfide (>2,500 vs. 30 μM) and ammonia (>400 μM in 2000), that are produced by microbial activity or that diffused into the bottom water from sediments. The high sulfide concentration results in the formation of thioarsenic compounds in the lake (Hollibaugh et al., 2005; Planer-Friedrich et al., 2007) so that most of the reduced As in the bottom water of the lake (~200 μM) was present in the form of higher order thioarsenic species in 2000 (Hollibaugh et al., 2005), whereas most of the reduced As was present as arsenite when we sampled in 2012 (Figure 1). Furthermore, at the elevated sulfide concentrations encountered in 2000, the reduced S pool is likely to contain species like polysulfides (Domagalski et al., 1990). Thus, differences in the composition of the microbial community between 2000 and 2012, particularly at depths below the chemocline, may reflect selection for taxa that are adapted to the higher sulfide and more strongly reducing conditions encountered in 2000. The microbial community encountered in the present study may represent a more diverse, and less stratified, set of taxa as a result of the rapidly changing conditions following the recent (6–8 months) establishment of meromixis.
The distribution of alpha diversity we found was similar to that reported by Humayoun et al. (2003), where community diversity increased with depth. However, we found a decrease in community diversity at 31 m. Similar changes in community diversity with depth were found in Lake Kivu, Africa, with greater diversity reported in the anoxic regions of the lake (Inceoglu et al., 2015). The microbial communities found in Soap Lake (Washington) also have a composition similar to Mono Lake and to other soda lakes, with greater diversity in the deep, sulfidic region of the water column (Dimitriu et al., 2008). Several other studies (e.g., Wani et al., 2006; Lanzén et al., 2013) found that the composition of microbial communities in other Soda Lake environments is similar to those we found in Mono Lake. 16S rRNA gene sequences most similar to Mono Lake sequences ML1228J-1 (Firmicutes) and ML635J-40 (Bacteroidetes) were recovered from organisms cultivated from the interior of ikaite columns (pH > 10) found in the Ikka Fjord in Greenland (Schmidt et al., 2006). The physiological requirements for survival under the conditions encountered in these environments likely limits the diversity of organisms that can survive there (Oren, 1999; Mesbah and Wiegel, 2012).
Author Contributions
JH and CE designed the study, JH and CE collected the samples, CE analyzed the samples and performed bioinformatics analysis, CE and JH wrote the paper.
Conflict of Interest Statement
The authors declare that the research was conducted in the absence of any commercial or financial relationships that could be construed as a potential conflict of interest.
Acknowledgments
We would like to thank Meredith Ross, Christopher Abin, Ron Oremland, Larry Miller, the Sierra Nevada Aquatic Research Lab and Tom Crowe of Mono Lake Boat Tours for field support. We thank Britta Planer-Friedrich for analyzing samples for arsenic and thioarsenic speciation. We thank Brandon Satinsky for mRNA internal standards, Shalabh Sharma, and the Georgia Advanced Computing Resource Center at UGA for bioinformatics support and scripts. This research was supported by NSF award EAR 09-52271 to JH.
Supplementary Material
The Supplementary Material for this article can be found online at: https://www.frontiersin.org/articles/10.3389/fmicb.2018.00014/full#supplementary-material
References
Altschul, S. F., Gish, W., Miller, W., Myers, E. W., and Lipman, D. J. (1990). Basic local alignment search tool. J. Mol. Biol. 215, 403–410. doi: 10.1016/S0022-2836(05)80360-2
Antony, C. P., Shimpi, G. G., Cockell, C. S., Patole, M. S., and Shouche, Y. S. (2014). Molecular characterization of prokaryotic communities associated with Lonar Crater basalts. Geomicrobiol. J. 31, 519–528. doi: 10.1080/01490451.2013.849314
Armitage, D., Gallagher, K., Youngblut, N., Buckley, D., and Zinder, S. (2012). Millimeter-scale patterns of phylogenetic and trait diversity in a salt marsh microbial mat. Front. Microbiol. 3:293. doi: 10.3389/fmicb.2012.00293
Boldareva, E. N., Bryantseva, I. A., Tsapin, A., Nelson, K., Sorokin, D. Y., Tourova, T. P., et al. (2007). The new alkaliphilic bacteriochlorophyll a-containing bacterium Roseinatronobacter monicus sp. nov. from the hypersaline Soda Mono Lake (California, United States). Microbiology 76, 82–92. doi: 10.1134/S0026261707010122
Budinoff, C. R., and Hollibaugh, J. T. (2007). Ecophysiology of a Mono Lake picocyanobacterium. Limnol. Oceanogr. 52, 2484–2496. doi: 10.4319/lo.2007.52.6.2484
Budinoff, C. R., and Hollibaugh, J. T. (2008). Arsenite-dependent photoautotrophy by an Ectothiorhodospira-dominated consortium. ISME J. 2, 340–343. doi: 10.1038/ismej.2007.115
Camacho, C., Coulouris, G., Avagyan, V., Ma, N., Papadopoulos, J., Bealer, K., et al. (2009). BLAST+: architecture and applications. BMC Bioinformatics 10:421. doi: 10.1186/1471-2105-10-421
Caporaso, J. G., Kuczynski, J., Stombaugh, J., Bittinger, K., Bushman, F. D., Costello, E. K., et al. (2010). QIIME allows analysis of high-throughput community sequencing data. Nat. Meth. 7, 335–336. doi: 10.1038/nmeth.f.303
Caporaso, J. G., Lauber, C. L., Walters, W. A., Berg-Lyons, D., Lozupone, C. A., Turnbaugh, P. J., et al. (2011). Global patterns of 16S rRNA diversity at a depth of millions of sequences per sample. Proc. Natl. Acad. Sci. U.S.A. 108, 4516–4522. doi: 10.1073/pnas.1000080107
Carini, P., Marsden, P. J., Leff, J. W., Morgan, E. E., Strickland, M. S., and Fierer, N. (2016). Relic DNA is abundant in soil and obscures estimates of soil microbial Diversity 2:16242. doi: 10.1038/nmicrobiol.2016.242
Carini, S. A., and Joye, S. B. (2008). Nitrification in Mono Lake, California: activity and community composition during contrasting hydrological regimes. Limnol. Oceanogr. 53, 2546–2557. doi: 10.4319/lo.2008.53.6.2546
Choi, D. H., Zhang, G. I., Noh, J. H., Kim, W.-S., and Cho, B. C. (2009). Gracilimonas tropica gen. nov., sp. nov., isolated from a synechococcus culture. Int. J. Syst. Evol. Microbiol. 59, 1167–1172. doi: 10.1099/ijs.0.005512-0
Cline, J. D. (1969). Spectrophotometric determination of hydrogen sulfide in natural waters. Limnol. Oceanogr. 14, 454–458. doi: 10.4319/lo.1969.14.3.0454
Dimitriu, P. A., Pinkart, H. C., Peyton, B. M., and Mormile, M. R. (2008). Spatial and temporal patterns in the microbial diversity of a meromictic soda lake in Washington state. Appl. Environ. Microbiol. 74, 4877–4888. doi: 10.1128/AEM.00455-08
Domagalski, J. L., Eugster, H. P., and Jones, B. F. (1990). “Trace metal geochemistry of Walker, Mono, and Great Salt Lakes,” in Fluid-Mineral Interactions: A Tribute to H. P. Eugster, eds R. J. Spencer and I. M. Chou. Special Publication No. 2 (Washington, DC: The Geochemical Society), 315–353.
Domagalski, J. L., Orem, W. H., and Eugster, H. P. (1989). Organic geochemistry and brine composition in Great Salt, Mono, and Walker Lakes. Geochim. Cosmochim. Acta 53, 2857–2872. doi: 10.1016/0016-7037(89)90163-4
Edgar, R. C. (2010). Search and clustering orders of magnitude faster than BLAST. Bioinformatics 26, 2460–2461. doi: 10.1093/bioinformatics/btq461
Edgar, R. C., Haas, B. J., Clemente, J. C., Quince, C., and Knight, R. (2011). UCHIME improves sensitivity and speed of chimera detection. Bioinformatics 27, 2194–2200. doi: 10.1093/bioinformatics/btr381
Edwardson, C. F., and Hollibaugh, J. T. (2017). Metatranscriptomic analysis of prokaryotic communities active in sulfur and arsenic cycling in Mono Lake, California, USA. ISME J. 11, 2195–2208 doi: 10.1038/ismej.2017.80
Edwardson, C. F., Planer-Friedrich, B., and Hollibaugh, J. T. (2014). Transformation of monothioarsenate by haloalkaliphilic, anoxygenic photosynthetic purple sulfur bacteria. FEMS Microbiol. Ecol. 90, 858–868. doi: 10.1111/1574-6941.12440
Fierer, N., and Jackson, R. B. (2006). The diversity and biogeography of soil bacterial communities. Proc. Natl. Acad. Sci. U.S.A. 103, 626–631. doi: 10.1073/pnas.0507535103
Fisher, J. C., and Hollibaugh, J. T. (2008). Selenate-dependent anaerobic arsenite oxidation by a bacterium from Mono Lake, California. Appl. Environ. Microbiol. 74, 2588–2594. doi: 10.1128/AEM.01995-07
Foti, M. J., Sorokin, D. Y., Zacharova, E. E., Pimenov, N. V., Kuenen, J. G., and Muyzer, G. (2008). Bacterial diversity and activity along a salinity gradient in soda lakes of the Kulunda Steppe (Altai, Russia). Extremophiles 12, 133–145. doi: 10.1007/s00792-007-0117-7
Fuhrman, J. A., Cram, J. A., and Needham, D. M. (2015). Marine microbial community dynamics and their ecological interpretation. Nat. Rev. Microbiol. 13, 133–146. doi: 10.1038/nrmicro3417
Gifford, S. M., Sharma, S., Rinta-Kanto, J. M., and Moran, M. A. (2011). Quantitative analysis of a deeply sequenced marine microbial metatranscriptome. ISME J. 5, 461–472. doi: 10.1038/ismej.2010.141
Gilbert, J. A., Steele, J. A., Caporaso, J. G., Steinbruck, L., Reeder, J., Temperton, B., et al. (2012). Defining seasonal marine microbial community dynamics. ISME J. 6, 298–308. doi: 10.1038/ismej.2011.107
Giri, B. J., Bano, N., and Hollibaugh, J. T. (2004). Distribution of RuBisCO genotypes along a redox gradient in Mono Lake, California. Appl. Environ. Microbiol. 70, 3443–3448. doi: 10.1128/AEM.70.6.3443-3448.2004
Hamady, M., Lozupone, C., and Knight, R. (2009). Fast UniFrac: facilitating high-throughput phylogenetic analyses of microbial communities including analysis of pyrosequencing and PhyloChip data. ISME J. 4, 17–27. doi: 10.1038/ismej.2009.97
Harris, K. J., Caporaso, G. J., Walker, J. J., Spear, J. R., Gold, N. J., Robertson, C. E., et al. (2013). Phylogenetic stratigraphy in the Guerrero Negro hypersaline microbial mat. ISME J. 7, 50–60. doi: 10.1038/ismej.2012.79
Hoeft, S. E., Kulp, T. R., Stolz, J. F., Hollibaugh, J. T., and Oremland, R. S. (2004). Dissimilatory arsenate reduction with sulfide as electron donor: experiments with Mono Lake water and isolation of Strain MLMS-1, a chemoautotrophic arsenate respirer. Appl. Environ. Microbiol. 70, 2741–2747. doi: 10.1128/AEM.70.5.2741-2747.2004
Hoeft, S. E., Switzer-Blum, J., Stolz, J. F., Tabita, F. R., Witte, B., King, G. M., et al. (2007). Alcalilimnicola ehrlichei, sp. nov. a novel, arsenite-oxidizing haloalkaliphilic gamma-Proteobacterium capable of chemoautotrophic or heterotrophic growth with nitrate or oxygen as the electron acceptor. Int. J. Syst. Evol. Microbiol. 57, 504–512. doi: 10.1099/ijs.0.64576-0
Hollibaugh, J. T., Budinoff, C., Hollibaugh, R. A., Ransom, B., and Bano, N. (2006). Sulfide oxidation coupled to arsenate reduction by a diverse microbial community in a soda lake. Appl. Environ. Microbiol. 72, 2043–2049. doi: 10.1128/AEM.72.3.2043-2049.2006
Hollibaugh, J. T., Carini, S., Gürleyük, H., Jellison, R., Joye, S. B., Lecleir, G., et al. (2005). Arsenic speciation in Mono Lake, California: response to seasonal stratification and anoxia. Geochim. Cosmochim. Acta 69, 1925–1937. doi: 10.1016/j.gca.2004.10.011
Humayoun, S. B., Bano, N., and Hollibaugh, J. T. (2003). Depth distribution of microbial diversity in Mono Lake, a meromictic soda lake in California. Appl. Environ. Microbiol. 69, 1030–1042. doi: 10.1128/AEM.69.2.1030-1042.2003
Huse, S. M., Huber, J. A., Morrison, H. G., Sogin, M. L., and Welch, D. M. (2007). Accuracy and quality of massively parallel DNA pyrosequencing. Genome Biol. 8:R143. doi: 10.1186/gb-2007-8-7-r143
Inceoglu, Ö., Llirós, M., Crowe, S. A., García-Armisen, T., Morana, C., Darchambeau, F., et al. (2015). Vertical distribution of functional potential and active microbial communities in meromictic lake kivu. Microb. Ecol. 70, 596–611. doi: 10.1007/s00248-015-0612-9
Jellison, R., and Melack, J. M. (1993). Algal photosynthetic activity and its response to meromixis in hypersaline Mono Lake, California. Limnol. Oceanogr. 38, 818–837. doi: 10.4319/lo.1993.38.4.0818
Joye, S. B., Connell, T. L., Miller, L. G., Oremland, R. S., and Jellison, R. S. (1999). Oxidation of ammonia and methane in an alkaline, saline lake. Limnol. Oceanogr. 44, 178–188. doi: 10.4319/lo.1999.44.1.0178
Kalanetra, K. M., Bano, N., and Hollibaugh, J. T. (2009). Ammonia-oxidizing Archaea in the Arctic Ocean and Antarctic coastal waters. Environ. Microbiol. 11, 2434–2445. doi: 10.1111/j.1462-2920.2009.01974.x
Kearse, M., Moir, R., Wilson, A., Stones-Havas, S., Cheung, M., Sturrock, S., et al. (2012). Geneious Basic: an integrated and extendable desktop software platform for the organization and analysis of sequence data. Bioinformatics 28, 1647–1649. doi: 10.1093/bioinformatics/bts199
Lanzén, A., Simachew, A., Gessesse, A., Chmolowska, D., Jonassen, I., and Øvreås, L. (2013). Surprising prokaryotic and eukaryotic diversity, community structure and biogeography of Ethiopian soda lakes. PLoS ONE 8:e72577. doi: 10.1371/journal.pone.0072577
Lecleir, G. R., Buchan, A., and Hollibaugh, J. T. (2004). Chitinase gene sequences from diverse aquatic habitats reveal complex patterns of diversity. Appl. Environ. Microbiol. 70, 6977–6983. doi: 10.1128/AEM.70.12.6977-6983.2004
Lecleir, G. R., Buchan, A., Maurer, J., Moran, M. A., and Hollibaugh, J. T. (2007). Comparison of chitinolytic enzymes from an alkaline, hypersaline lake and an estuary. Environ. Microbiol. 9, 197–205. doi: 10.1111/j.1462-2920.2006.01128.x
Lemieux, C., Otis, C., and Turmel, M. (2014). Six newly sequenced chloroplast genomes from prasinophyte green algae provide insights into the relationships among prasinophyte lineages and the diversity of streamlined genome architecture in picoplanktonic species. BMC Genomics 15:857. doi: 10.1186/1471-2164-15-857
Lin, J. L., Joye, S. B., Scholten, J. C. M., Schäfer, H., McDonald, I. R., and Murrell, J. C. (2005). Analysis of methane monooxygenase genes in Mono Lake suggests that increased methane oxidation activity may correlate with a change in methanotroph community structure. Appl. Environ. Microbiol. 71, 6458–6462. doi: 10.1128/AEM.71.10.6458-6462.2005
McMurdie, P. J., and Holmes, S. (2013). phyloseq: an R package for reproducible interactive analysis and graphics of microbiome census data. PLoS ONE 8:e61217. doi: 10.1371/journal.pone.0061217
Melack, J. M., and Jellison, R. (1998). Limnological conditions in Mono Lake: contrasting monomixis and meromixis in the 1990s. Hydrobiologia 384, 21–39. doi: 10.1023/A:1003352511328
Melack, J. M., Jellison, R., Macintyre, S., and Hollibaugh, J. T. (eds.). (2017). Mono Lake: Plankton Dynamics Over Three Decades of Meromixis or Monomixis. New York, NY: Springer.
Mesbah, N. M., Abou-El-Ela, S. H., and Wiegel, J. (2008). Novel and unexpected prokaryotic diversity in water and sediments of the alkaline, hypersaline lakes of the Wadi An Natrun, Egypt. Microb. Ecol. 55, 369–369. doi: 10.1007/s00248-007-9338-7
Mesbah, N. M., and Wiegel, J. (2012). Life under multiple extreme conditions: Diversity and physiology of the halophilic alkalithermophiles. Appl. Environ. Microbiol. 78, 4074–4082. doi: 10.1128/AEM.00050-12
Nercessian, O., Kalyuzhnaya, M. G., Joye, S. B., Lidstrom, M. E., and Chistoserdova, L. (2005). Analysis of fae and fhcD Genes in Mono Lake, California. Appl. Environ. Microbiol. 71, 8949–8953. doi: 10.1128/AEM.71.12.8949-8953.2005
Nyerges, G., and Stein, L. Y. (2009). Ammonia cometabolism and product inhibition vary considerably among species of methanotrophic bacteria. FEMS Microbiol. Lett. 297, 131–136. doi: 10.1111/j.1574-6968.2009.01674.x
Oremland, R. S. (1990). Nitrogen fixation dynamics of two diazotrophic communities in Mono Lake, California. Appl. Environ. Microbiol. 56, 614-622
Oremland, R. S., Dowdle, P. R., Hoeft, S., Sharp, J. O., Schaefer, J. K., Miller, L. G., et al. (2000). Bacterial dissimilatory reduction of arsenate and sulfate in meromictic Mono Lake, California. Geochim. Cosmochim. Acta 64, 3073–3084. doi: 10.1016/S0016-7037(00)00422-1
Oremland, R. S., Saltikov, C. W., Stolz, J. F., and Hollibaugh, J. (2017). Autotrophic microbial arsenotrophy in arsenic-rich soda lakes. FEMS Microbiol. Lett. 146, 1–22. doi: 10.1093/femsle/fnx146
Oremland, R. S., Stolz, J. F., and Hollibaugh, J. T. (2004). The microbial arsenic cycle in Mono Lake, California. FEMS Microbiol. Ecol. 48, 15–27. doi: 10.1016/j.femsec.2003.12.016
Planer-Friedrich, B., London, J., McCleskey, R. B., Nordstrom, D. K., and Wallschläger, D. (2007). Thioarsenates in geothermal waters – determination, preservation, and geochemical role. Environ. Sci. Technol. 41, 5245–5251. doi: 10.1021/es070273v
Podell, S., Emerson, J. B., Jones, C. M., Ugalde, J. A., Welch, S., Heidelberg, K. B., et al. (2014). Seasonal fluctuations in ionic concentrations drive microbial succession in a hypersaline lake community. ISME J. 8, 979–990. doi: 10.1038/ismej.2013.221
Poretsky, R. S., Bano, N., Buchan, A., Hollibaugh, J. T., and Moran, M. A. (2006). “Environmental transcriptomics: a method to access expressed genes in complex microbial communities,” in Molecular Microbial Ecology Manual 3rd Edn., eds G. A. Kowalchuk, F. J. De Bruijn, I. M. Head, A. D. L. Akkermans, and J. D. Van Elsas (Dordrecht: Springer Verlag), 1892–1904.
Poretsky, R. S., Bano, N., Buchan, A., Lecleir, G., Kleikemper, J., Pickering, M., et al. (2005). Analysis of microbial gene transcripts in environmental samples. Appl. Environ. Microbiol. 71, 4121–4126. doi: 10.1128/AEM.71.7.4121-4126.2005
Pruesse, E., Peplies, J., and Glöckner, F. O. (2012). SINA: accurate high-throughput multiple sequence alignment of ribosomal RNA genes. Bioinformatics 28, 1823–1829. doi: 10.1093/bioinformatics/bts252
Quast, C., Pruesse, E., Yilmaz, P., Gerken, J., Schweer, T., Yarza, P., et al. (2013). The SILVA ribosomal RNA gene database project: improved data processing and web-based tools. Nucleic Acids Res. 41, D590–D596. doi: 10.1093/nar/gks1219
Rascovan, N., Maldonado, J., Vazquez, M. P., and Eugenia Farias, M. (2016). Metagenomic study of red biofilms from Diamante Lake reveals ancient arsenic bioenergetics in haloarchaea. ISME J. 10, 299–309. doi: 10.1038/ismej.2015.109
Reeder, J., and Knight, R. (2010). Rapidly denoising pyrosequencing amplicon reads by exploiting rank-abundance distributions. Nat. Methods 7, 668–669. doi: 10.1038/nmeth0910-668b
Rusch, D. B., Halpern, A. L., Sutton, G., Heidelberg, K. B., Williamson, S., Yooseph, S., et al. (2007). The sorcerer II global ocean sampling expedition: Northwest Atlantic through Eastern Tropical Pacific. PLoS Biol. 5:e77. doi: 10.1371/journal.pbio.0050077
Sanger, F., Nicklen, S., and Coulson, A. R. (1977). DNA sequencing with chain-terminating inhibitors. Proc. Natl. Acad. Sci. U.S.A. 74, 5463–5467. doi: 10.1073/pnas.74.12.5463
Satinsky, B. M., Crump, B. C., Smith, C. B., Sharma, S., Zielinski, B. L., Doherty, M., et al. (2014). Microspatial gene expression patterns in the Amazon River Plume. Proc. Natl. Acad. Sci. 111, 11085–11090. doi: 10.1073/pnas.1402782111
Satinsky, B. M., Gifford, S. M., Crump, B. C., and Moran, M. A. (2013). “Use of internal standards for quantitative metatranscriptome and metagenome analysis,” in Methods in Enzymology, ed E. F. Delong (New York, NY: Academic Press), 237–250.
Schmidt, M., Priemé, A., and Stougaard, P. (2006). Bacterial diversity in permanently cold and alkaline ikaite columns from Greenland. Extremophiles 10, 551–562. doi: 10.1007/s00792-006-0529-9
Schmieder, R., and Edwards, R. (2011). Quality control and preprocessing of metagenomic datasets. Bioinformatics 27, 863–864. doi: 10.1093/bioinformatics/btr026
Schmieder, R., Lim, Y. W., and Edwards, R. (2012). Identification and removal of ribosomal RNA sequences from metatranscriptomes. Bioinformatics 28, 433–435. doi: 10.1093/bioinformatics/btr669
Schneider, D., Arp, G., Reimer, A., Reitner, J., and Daniel, R. (2013). Phylogenetic analysis of a microbialite-forming microbial mat from a hypersaline lake of the Kiritimati Atoll, central Pacific. PLoS ONE 8:e66662. doi: 10.1371/journal.pone.0066662
Scholten, J. C. M., Joye, S. B., Hollibaugh, J. T., and Murrell, J. C. (2005). Molecular analysis of the sulfate reducing and Archaeal community in a meromictic lake (Mono Lake, California) by targeting 16s rRNA, mcrA, apsA and dsrAB reductase genes. Microb. Ecol. 50, 29–39. doi: 10.1007/s00248-004-0085-8
Sogin, M. L., Morrison, H. G., Huber, J. A., Welch, D. M., Huse, S. M., Neal, P. R., et al. (2006). Microbial diversity in the deep sea and the underexplored “rare biosphere”. Proc. Natl. Acad. Sci. U.S.A. 103, 12115–12120. doi: 10.1073/pnas.0605127103
Sorokin, D. (2011). The microbial sulfur cycle at extremely haloalkaline conditions of soda lakes. Front. Microbiol. 2:44. doi: 10.3389/fmicb.2011.00044
Sorokin, D. Y., Banciu, H., Robertson, L. A., Kuenen, J. G., Muntyan, M. S., and Muyzer, G. (2013). “Halophilic and haloalkaliphilic sulfur-oxidizing bacteria,” in The Prokaryotes, eds E. Rosenberg, E. Delong, S. Lory, E. Stackebrandt, and F. Thompson (Berlin: Springer), 529–554.
Sorokin, D. Y., Gorlenko, V. M., Tourova, T. P., Tsapin, A. I., Nealson, K. H., and Kuenen, G. J. (2002). Thioalkalimicrobium cyclicum sp. nov. and Thioalkalivibrio jannaschii sp. nov., novel species of haloalkaliphilic, obligately chemolithoautotrophic sulfur-oxidizing bacteria from Mono Lake (California). Int. J. Syst. Evol. Microbiol. 52, 913–920. doi: 10.1099/00207713-52-3-913
Sorokin, D. Y., Lysenko, A. M., Mityushina, L. L., Tourova, T. P., Jones, B. E., Rainey, F. A., et al. (2001). Thioalkalimicrobium aerophilum gen. nov., sp. nov. and Thioalkalimicrobium sibericum sp. nov., and Thioalkalivibrio versutus gen. nov., sp. nov., Thioalkalivibrio nitratis sp.nov., novel and Thioalkalivibrio denitrificancs sp. nov., novel obligately alkaliphilic and obligately chemolithoautotrophicsulfur-oxidizing bacteria from soda lakes. Int. J. Syst. Bacteriol. 51, 565–580. doi: 10.1099/00207713-51-2-565
Sorokin, D. Y., Tourova, T. P., Mußmann, M., and Muyzer, G. (2008). Dethiobacter alkaliphilus gen. nov. sp. nov., and Desulfurivibrio alkaliphilus gen. nov. sp. nov.: two novel representatives of reductive sulfur cycle from soda lakes. Extremophiles 12, 431–439. doi: 10.1007/s00792-008-0148-8
Sorokin, D. Y., Van Pelt, S., Tourova, T. P., and Evtushenko, L. I. (2009). Nitriliruptor alkaliphilus gen. nov., sp. nov., a deep-lineage haloalkaliphilic actinobacterium from soda lakes capable of growth on aliphatic nitriles, and proposal of Nitriliruptoraceae fam. nov. and Nitriliruptorales ord. nov. Int. J. Syst. Evol. Microbiol. 59, 248–253. doi: 10.1099/ijs.0.002204-0
Stewart, F. J., Ottesen, E. A., and Delong, E. F. (2010). Development and quantitative analyses of a universal rRNA-subtraction protocol for microbial metatranscriptomics. ISME J. 4, 896–907. doi: 10.1038/ismej.2010.18
Switzer-Blum, J., Burns-Bindi, A., Buzzelli, J., Stolz, J. F., and Oremland, R. S. (1998). Bacillus arsenicoselenatis sp. nov., and Bacillus selenitireducens sp. nov.: two haloalkaliphiles from Mono Lake, California that respire oxyanions of selenium and arsenic. Archiv fur Microbiologie 171, 19–30. doi: 10.1007/s002030050673
Tatusova, T., Ciufo, S., Fedorov, B., O'neill, K., and Tolstoy, I. (2014). RefSeq microbial genomes database: new representation and annotation strategy. Nucleic Acids Res. 42, D553–D559. doi: 10.1093/nar/gkt1274
Thorup, C., Schramm, A., Findlay, A. J., Finster, K. W., and Schreiber (2017). Disguised as a sulfate reducer: growth of the Deltaproteobacterium Desulfurivibrio alkaliphilus by sulfide oxidation with Nitrate. mBio 8:e00671–17 doi: 10.1128/mBio.00671-17
Tripp, H. J., Hewson, I., Boyarsky, S., Stuart, J. M., and Zehr, J. P. (2011). Misannotations of rRNA can now generate 90% false positive protein matches in metatranscriptomic studies. Nucleic Acids Res. 39, 8792–8802. doi: 10.1093/nar/gkr576
Urios, L., Intertaglia, L., Lesongeur, F., and Lebaron, P. (2008). Balneola alkaliphila sp. nov., a marine bacterium isolated from the Mediterranean Sea. Int. J. Syst. Evol. Microbiol. 58, 1288–1291. doi: 10.1099/ijs.0.65555-0
Venter, J. C., Remington, K., Heidelberg, J. F., Halpern, A. L., Rusch, D., Eisen, J. A., et al. (2004). Environmental genome shotgun sequencing of the Sargasso Sea. Science 304, 66–74. doi: 10.1126/science.1093857
Vidal, J., Macintyre, S., McPhee-Shaw, E. E., Shaw, W. J., and Monismith, S. G. (2013). Temporal and spatial variability of the internal wave field in a lake with complex morphometry. Limnol. Oceanogr. 58, 1557–1580. doi: 10.4319/lo.2013.58.5.1557
Wang, Q., Garrity, G. M., Tiedje, J. M., and Cole, J. R. (2007). Naïve Bayesian classifier for rapid assignment of rRNA sequences into the new bacterial taxonomy. Appl. Environ. Microbiol. 73, 5261–5267. doi: 10.1128/AEM.00062-07
Wani, A. A., Surakasi, V. P., Siddharth, J., Raghavan, R. G., Patole, M. S., Ranade, D., et al. (2006). Molecular analyses of microbial diversity associated with the Lonar soda lake in India: an impact crater in a basalt area. Res. Microbiol. 157, 928–937. doi: 10.1016/j.resmic.2006.08.005
Ward, B. B., Martino, D. P., Diaz, M. C., and Joye, S. B. (2000). Analysis of ammonia-oxidizing bacteria from hypersaline Mono Lake, California, on the basis of 16S rRNA sequences. Appl. Environ. Microbiol. 66, 2873–2881. doi: 10.1128/AEM.66.7.2873-2881.2000
Yelton, A. P., Comolli, L. R., Justice, N. B., Castelle, C., Denef, V. J., Thomas, B. C., et al. (2013). Comparative genomics in acid mine drainage biofilm communities reveals metabolic and structural differentiation of co-occurring archaea. BMC Genomics 14:485. doi: 10.1186/1471-2164-14-485
Zhang, J., Kobert, K., Flouri, T., and Stamatakis, A. (2014). PEAR: a fast and accurate Illumina Paired-End reAd mergeR. Bioinformatics 30, 614–620. doi: 10.1093/bioinformatics/btt593
Keywords: Mono Lake, soda lakes, alkaliphile, halophile, Picocystis
Citation: Edwardson CF and Hollibaugh JT (2018) Composition and Activity of Microbial Communities along the Redox Gradient of an Alkaline, Hypersaline, Lake. Front. Microbiol. 9:14. doi: 10.3389/fmicb.2018.00014
Received: 03 August 2017; Accepted: 05 January 2018;
Published: 31 January 2018.
Edited by:
Karla B. Heidelberg, University of Southern California, United StatesReviewed by:
Dimitry Y. Sorokin, Winogradsky Institute of Microbiology (RAS), RussiaCharles K. Lee, University of Waikato, New Zealand
Copyright © 2018 Edwardson and Hollibaugh. This is an open-access article distributed under the terms of the Creative Commons Attribution License (CC BY). The use, distribution or reproduction in other forums is permitted, provided the original author(s) and the copyright owner are credited and that the original publication in this journal is cited, in accordance with accepted academic practice. No use, distribution or reproduction is permitted which does not comply with these terms.
*Correspondence: James T. Hollibaugh, aquadoc@uga.edu