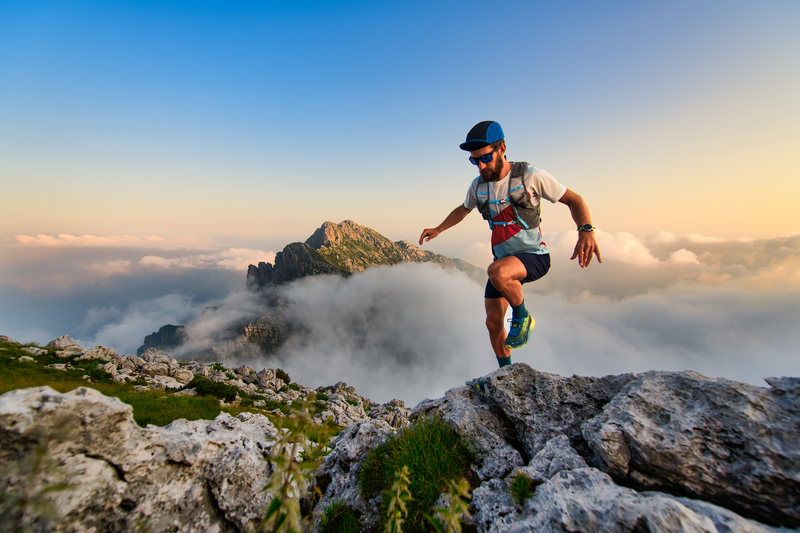
94% of researchers rate our articles as excellent or good
Learn more about the work of our research integrity team to safeguard the quality of each article we publish.
Find out more
ORIGINAL RESEARCH article
Front. Microbiol. , 16 December 2016
Sec. Aquatic Microbiology
Volume 7 - 2016 | https://doi.org/10.3389/fmicb.2016.01949
Community assembly processes generate shifts in species abundances that influence ecosystem cycling of carbon and nutrients, yet our understanding of assembly remains largely separate from ecosystem-level functioning. Here, we investigate relationships between assembly and changes in microbial metabolism across space and time in hyporheic microbial communities. We pair sampling of two habitat types (i.e., attached and planktonic) through seasonal and sub-hourly hydrologic fluctuation with null modeling and temporally explicit multivariate statistics. We demonstrate that multiple selective pressures—imposed by sediment and porewater physicochemistry—integrate to generate changes in microbial community composition at distinct timescales among habitat types. These changes in composition are reflective of contrasting associations of Betaproteobacteria and Thaumarchaeota with ecological selection and with seasonal changes in microbial metabolism. We present a conceptual model based on our results in which metabolism increases when oscillating selective pressures oppose temporally stable selective pressures. Our conceptual model is pertinent to both macrobial and microbial systems experiencing multiple selective pressures and presents an avenue for assimilating community assembly processes into predictions of ecosystem-level functioning.
The collective effects of community assembly processes (e.g., dispersal, drift, and selection) on microbial metabolism of carbon and nutrients in the environment are poorly understood, and they constitute a key knowledge gap in process-based modeling of biogeochemical cycles. Selection and dispersal both have the potential to impact rates of microbial metabolism. For example, selection can enhance metabolism via species sorting mechanisms that optimize the microbiome for a given environment (Van der Gucht et al., 2007; Lindström and Langenheder, 2012), while dispersal limitation can inhibit immigration of metabolic diversity, and in some cases, lead to a maladapted and poorly functioning community (Telford et al., 2006; Lindström and Östman, 2011; Hanson et al., 2012; Peres et al., 2016). The extent to which community assembly processes regulate metabolism is contingent on myriad spatiotemporal dynamics including the geographic distance separating communities, the rate of environmental change, and historical abiotic conditions (Fukami et al., 2010; Graham et al., 2014; Nemergut et al., 2014; Hawkes and Keitt, 2015; Graham et al., 2016). Yet, we lack a conceptual basis for how multiple community assembly processes jointly influence microbial metabolism (Prosser et al., 2007; Gonzalez et al., 2012; Shade et al., 2013; Graham et al., 2016).
Community assembly processes act through space and time to impact community membership, which then impacts microbial metabolism (Vellend, 2010; Nemergut et al., 2013). For instance, communities experiencing a history of strong and consistent selection may contain taxa that are well-adapted to their environment and exhibit high metabolic rates. Alternatively, intense, unyielding selection may eliminate microbial taxa that metabolize scarce resource pools and impair community metabolic functioning (Grime, 1998; Loreau and Hector, 2001; Knelman and Nemergut, 2014). In this case, more diverse communities (e.g., those experiencing higher rates of dispersal or counteracting selective pressures) would be expected to exhibit higher and more consistent rates of metabolism than those structured by one dominant selective pressure. Dispersal limitation can inhibit the ability of organisms to reach their optimal environment, resulting in lower rates of community metabolism, while high rates of dispersal may either reduce or enhance microbial metabolism, respectively, by allowing for immigration of maladapted organisms or by increasing biodiversity (Hooper et al., 2012; Nemergut et al., 2014).
Additionally, individual taxa are differentially impacted by community assembly processes. Only some taxa contain traits that are under selection in given environmental conditions, and changes in the environment may affect some taxa to a greater extent than others (Poff, 1997; Lebrija-Trejos et al., 2010; Knelman and Nemergut, 2014; Krause et al., 2014). For example, a change in a certain nutrient should have a larger influence on taxa that directly metabolize it than those that utilize it as a secondary resource. Similarly, traits that facilitate dispersal (e.g., spore formation) are preferentially contained within certain taxa (Martiny et al., 2006; Tremlová and Münzbergová, 2007) such that changes in abiotic transport mechanisms should have contrasting effects on taxa with or without traits that facilitate dispersal. Thus, taxon-specific relationships between assembly processes and microbial communities may inform our understanding of the ecological processes influencing changes in environmental microbiomes beyond trends we observe at the community-level.
Environmental transition zones present a unique opportunity for examining interactions between microbial metabolism and both long- and short-term assembly processes, as they experience extreme spatiotemporal variation in physicochemical characteristics and microbial community composition across tractable spatial and temporal scales. Here, we leverage inherent variation in hydrology, habitat heterogeneity, and aerobic respiration in a zone of subsurface groundwater-surface water mixing (hereafter termed “hyporheic zone”) to examine the interplay of community assembly processes and microbial metabolism through time. Hyporheic zones are subsurface regions below and adjacent to rivers and streams that experience mixing between surface water and groundwater. The groundwater-surface water mixing within the hyporheic zone can result in blending of complementary resources and, in turn, elevated rates of microbial metabolism relative to other systems (Hancock et al., 2005; Boulton et al., 2010).
We employ null modeling in conjunction with temporally explicit multivariate statistics to characterize assembly processes driving shifts in microbial communities and microbial metabolism in the Columbia River hyporheic zone. We examine two co-occurring, yet ecologically distinct, habitat types—attached and planktonic communities. Further, we extend the analysis of community assembly processes to individual taxa that likely contribute to observed shifts in microbial metabolism. Our results culminate in a broadly applicable conceptual model coupling changes in selective environments, trait abundance, and ecosystem-level functioning through time.
This study was conducted in Hanford Reach of the Columbia River adjacent to the Hanford 300A (approximately 46°22′ 15.80′′N, 119° 16′ 31.52′′W) in eastern Washington, as described elsewhere (Slater et al., 2010; Zachara et al., 2013; Stegen et al., 2016), from March to November 2014. The hyporheic zone of the Columbia River experiences geographic variation in groundwater-surface water mixing, porewater geochemistry, and microbial community composition on sub-hourly to annual timescales (Peterson and Connelly, 2004; Arntzen et al., 2006; Slater et al., 2010; Lin et al., 2012; Stegen et al., 2012, 2016; Zachara et al., 2013). Accordingly, the Hanford Reach of the Columbia River embodies a model system to facilitate the integration of community ecology and microbial metabolism.
We monitored physicochemical conditions for three hydrologically-connected geographic zones (nearshore, inland, river) via aqueous sampling (Table S1). The inland environment is characterized by an unconfined aquifer within the Hanford formation and more recent illuvial deposits, and it maintains a distinct hydrologic environment with stable temperatures (∼15°C) and high concentrations of anions and inorganic carbon relative to the river. River water contains high concentrations of organic material and low concentrations of ions with seasonally variable temperatures. The waters from these discrete hydrologic environments experience dynamic mixing in a nearshore hyporheic zone that is regulated by fluctuations in river stage; we focus on ecological dynamics within this zone. To monitor groundwater-surface water mixing across space and time, we utilize Cl- as a conservative tracer for groundwater contributions to hyporheic porewater chemistry as employed by Stegen et al. (2016).
Detailed sampling and analytical methods are in the Supplementary Material. Attached and planktonic communities were obtained from deployed colonization substrate and aqueous samples in the hyporheic zone. Samples to construct the regional species pool for null models were simultaneously obtained at three inland wells and at one location in the Columbia River (n = 0-4 at each sampling event, a full breakdown of sample size is listed in Table S2). These samples were collected at three-week intervals from March through November 2014, with the first planktonic samples collected in March and the first attached samples collected after a 6-week incubation period from piezometers installed to 1.2 m depth near the riverbed. Samples collected at each time point were assumed to represent sub-hourly variation in hydrologic conditions, as hydrology fluctuates at sub-hourly rates within a single day in our system (Arntzen et al., 2006), whereas samples collected across the full sampling period were assumed to represent seasonal variation.
Aqueous samples were obtained by pumping water from piezometers adjacent to colonization substrates and used to derive physicochemical conditions as well as to sample planktonic communities. Attached microbial communities were sampled by deploying mesh stainless steel incubators of locally sourced colonization substrate in piezometers within one meter of piezometers from which aqueous samples were obtained. All incubators were deployed 6 weeks prior to removal. For each sample, we collected the following data according to procedures outlined in the Supplementary Material: physicochemical characteristics (aqueous samples only), microbial metabolism (aerobic metabolism assayed with Resazurin) per unit active biomass (ATP) defined by Raz:ATP (attached samples only), and 16S rRNA amplicon sequences (all samples). Sequences are publically available at doi: 10.6084/m9.figshare.4264148.
We implemented null modeling methodology developed by Stegen et al. (2013, 2015) using R software1 to disentangle community assembly processes (Supplementary Material). The approach uses pairwise phylogenetic distances between communities, calculated using the mean-nearest-taxon-distance (βMNTD) metric (Webb et al., 2008; Fine and Kembel, 2011), to infer the strength of selection. Communities were evaluated for significantly less turnover than expected (βNTI < -2, homogeneous selection) or more turnover than expected (βNTI > 2, variable selection) by comparing observed βMNTD values to the mean of a null distribution of βMNTD values—and normalizing by its standard deviation—to yield βNTI (Stegen et al., 2012). Pairwise community comparisons that did not deviate from the null βMNTD distribution were evaluated for the influences of dispersal limitation and homogenizing dispersal by calculating the Raup-Crick metric extended to account for species relative abundances (RCbray), as per Stegen et al. (2013, 2015). Observed Bray-Curtis dissimilarities were compared to the null distribution to derive RCbray. RCbray values > 0.95, > -0.95 and < 0.95, or < -0.95 were assumed to indicate dispersal limitation, no dominant assembly process, or homogenizing dispersal, respectively. Significance levels for βNTI and RCbray are based on standard deviations—|βNTI| = 2 denotes two standard deviations from the mean of the null distribution—and alpha values—|RCbray| = 0.95 reflects significance at the 0.05 level. Inferences from both βNTI and RCbray have previously been shown to be robust (Dini-Andreote et al., 2015; Stegen et al., 2015).
Regressions and one-sided Mann Whitney U tests were conducted using the base statistics package in R. Variation in community composition was assessed with PERMANOVA in QIIME (Caporaso et al., 2010). We fit porewater characteristics to NMDS plots of Bray-Curtis dissimilarities with and without stratifying by time within attached and planktonic communities using the ‘vegan’ package in R (999 permutations, Oksanen et al., 2013). Further details are available in the Supplementary Material.
Because we observed large seasonal differences in species richness in both attached and planktonic communities, we performed similarity percentage analysis (SIMPER) in ‘vegan’ to identify individual species driving community dissimilarity (Clarke, 1993). SIMPER was conducted across time periods of high and low species richness within attached and planktonic communities separately (i.e., communities from sampling points with high vs. low richness in each habitat type). We grouped communities by sampling date (determined by the average species richness in communities at each time point) to control for seasonal effects. Further, we used SIMPER to identify species that differentiated attached and planktonic communities regardless of differences in richness (i.e., all attached communities vs. all planktonic communities).
We extracted taxonomic groups of organisms at the class-level containing at least one species identified as having a significant impact on community composition by SIMPER (P < 0.05) for subsequent analyses. Organisms were grouped at the class-level to provide sufficient statistical power for analysis. Mantel tests were used to compare the average relative abundance of taxonomic groups identified by SIMPER across samples to associated βNTI and RCbray values (‘vegan’, 999 permutations). Finally, we compared dissimilarity in species richness between samples within and across attached and planktonic communities to βNTI and further, if -2 < βNTI < 2, to RCbray using Mantel tests to infer community assembly processes generating species turnover between samples.
We observed distinct temporal trends in microbial communities and in groundwater-surface water mixing, characterized by an abrupt increase in Cl- concentration (Figure 1A) and decrease in NPOC (Figure 1B) associated with a seasonal shift in water stage (Figure S1 and Table S1). Temperature peaked during August and followed a smooth temporal trend (Figure 1C). The composition of both attached (PERMANOVA, R2 = 0.44, P = 0.001) and planktonic (PERMANOVA, R2 = 0.44, P = 0.001) communities also changed across our sampling period, but attached and planktonic communities remained taxonomically distinct through time (PERMANOVA, R2 = 0.19, P = 0.001). Main taxa in each environment are presented in Table S3.
FIGURE 1. Changes in (A) chloride concentration, (B) NPOC concentration, (C) temperature, and (D) species richness across our sampling period are depicted in Figure 1. Chloride and NPOC concentration show abrupt shifts beginning at our July 22 sampling point (vertical dashed lines). P-values in (A) and (B) denote one-sided Mann-Whitney U test results of samples taken before versus on or after July 22, while trends through time in (A) and (B) are displayed using locally weighted scatterplot smoothing (LOWESS). Quadratic polynomials were fit to temperature and species richness data and plotted in (C,D). Triangles in (D) represent planktonic communities; X’s represent attached communities.
Species richness in both attached and planktonic communities mirrored temperature trends, with the highest number of species observed during the warmest summer months (Figure 1D). Richness was more tightly correlated with temperature (Figure S2, regression, attached: R2 = 0.25, P = 0.001, planktonic: R2 = 0.22, P = 0.002) than Cl- (regression, attached: P = 0.01, R2 = 0.14, planktonic: P > 0.05) and NPOC (regression, attached: P = 0.04, R2 = 0.10, planktonic: P > 0.05). Finally, within both environments, community dissimiliarity increased in concert with differences in species richness (Figure 2A).
FIGURE 2. (A) Bray-Curtis dissimilarity within each sampling time point increased as mean species richness increased in attached (X’s) and planktonic (triangles) communities. In addition, βNTI values across differences in species richness are shown for (B) attached, (C) planktonic, and (D) attached vs. planktonic communities. Horizontal lines at βNTI = -2 and βNTI = 2 denote thresholds for assembly processes. βNTI values less than -2 suggest assembly is governed by homogeneous selection, while values greater than 2 suggest assembly is governed by variable selection. Stochastic assembly processes (dispersal limitation, homogenizing dispersal) and undominated assembly processes lie between βNTI -2 and 2. The proportion of βNTI values within each category are listed as text in (B-D). A linear regression trend line is depicted in (C) with significance assessed via Mantel test.
Dissimilarity in microbial communities indicated a possible role for different assembly processes governing species composition among (i.e., within attached and planktonic separately) and across (i.e., attached vs. planktonic) habitats. We investigated assembly processes across differences in richness to identify processes that impacted species addition to each habitat, and we found that assembly varied between habitats (Figures 2B,C). βNTI was positively correlated with differences in species richness in planktonic samples (Mantel, P = 0.001, r = 0.41, Figure 2C), with weaker correlations in attached communities (Mantel, P = 0.02, r = 0.24, Figure 2B) and between attached and planktonic communities (Mantel, P = 0.006, r = -0.26, Figure 2D).
Because selection was a substantial driver of all microbial communities, we further examined the impact of aqueous physicochemistry on communities at both sub-hourly (within sampling date) and seasonal (across all sampling dates) timescales using stratified and unstratified NMDS analysis. Permutations in stratified NMDS are constrained within the specified grouping (in our case, sampling date), thus controlling for the grouping factor (see Oksanen et al., 2013). Here, we infer significant relationships when stratifying by time to represent dynamics occurring within each sampling date and those occurring in analyses without stratification to represent dynamics across sampling dates. Planktonic communities were correlated to more environmental variables than attached communities at a sub-hourly timescale (NMDS stratified by time, Figures 3A,B; Table S4). Conversely, attached communities correlated more tightly with physicochemical attributes over a seasonal timescale (unstratified NMDS, Figures 3A,B).
FIGURE 3. Non-metric multidimensional scaling (NMDS) analysis was conducted on Bray-Curtis distances within (A) planktonic and (B) attached communities. Colors denote seasonal shifts in community structure along a gradient from March (red) to November (blue). Physicochemical characteristics were fit to each plot with (blue arrows) and without (red arrows) stratifying permutations by sampling time to assess short- and long-term community responses, respectively, to the aqueous environment.
Similarity percentage analysis revealed species driving differences among attached and planktonic communities during periods of high versus low species richness (Table S5). We extracted phylogenetic classes of organisms containing species identified by SIMPER and examined relationships between their relative abundances and βNTI and RCbray. All significant correlations with absolute r values greater than 0.30 are listed in Table S5. Taxa in planktonic communities exhibited no relationships with βNTI (Table S6), but the mean relative abundance of many taxa, including Thaumarchaeota (positive, Figure 4A), a class of Acidobacteria (positive, Figure 4B), Actinobacteria (negative, Figure 4C), and Alphaproteobacteria (negative, Figure 4D), displayed correlations with RCbray. The mean relative abundance of Parvarcheota and a class of candidate phyla OP3 (koll11) were positively correlated with βNTI derived from comparisons across attached and planktonic communities (Figures 4E,F). Finally, within attached communities, βNTI was correlated with the mean relative abundance of Thaumarchaeota (positive, Figure 4G) and Betaproteobacteria (negative, Figure 4H). No correlations were observed between RCbray and taxa within attached communities or across attached-vs.-planktonic communities (Table S6).
FIGURE 4. Relationships between βNTI or RCbray and the mean abundance (across samples) of selected taxa identified by SIMPER analysis are depicted in Figure 4. (A-D) Demonstrate relationships of Thaumarchaeota, Acidobacteria-6, Actinobacteria, and Alphaproteobacteria, respectively, versus RCbray in planktonic communities. Horizontal lines at βNTI = -2 (homogeneous selection) and βNTI = 2 (variable selection) and vertical lines at RCbray = -0.95 (homogenizing dispersal) and 0.95 (dispersal limitation) denote thresholds for assembly processes. Trend lines in all panels were derived from linear regressions and significance was assessed via Mantel test. (E,F) Show relationships of βNTI with Parvarchaeota and koll11 in attached vs. planktonic communities; while (G,H) denote relationships of βNTI with Thaumarchaeota and Betaproteobacteria within attached communities, respectively.
We also observed a seasonal increase in the abundance of Thaumarchaeota in attached communities and a concomitant decrease in Betaproteobacteria (Figure 5A) that corresponded with shifts in hydrology (Figure 5B). Oxygenated conditions persisted throughout our sampling period, and Raz:ATP increased seasonally within attached communities, an effect that correlated with day of year (Figure 5C) but not temperature, NPOC concentration, or hydrology (regression: temperature P = 0.10, NPOC P = 0.21, log(Cl-) P = 0.15). The relative abundance of Thaumarchaeota and Betaproteobacteria in attached communities also correlated positively and negatively, respectively, with Raz:ATP (Figure 5D) and exhibited contrasting responses to porewater physicochemical properties (Table S7).
FIGURE 5. (A,B) Changes in Thaumarchaeota and Betaproteobacteria across changes in time (A) and chloride concentration (B). Trend lines in (A) denote linear (Betaproteobacteria) and quadratic (Thaumarchaeota) regressions. The vertical line and statistics in (B) denote one-sided Mann-Whitney U test results of Betaproteobacteria and Thaumarchaeota when chloride concentrations are above or below the maximum Cl- concentration in the Columbia River (1.83 mg/L). (C) Shows increases in aerobic respiration normalized to active biomass (Raz:ATP) through time. Finally, (D) shows relationships of Betaproteobacteria and Thaumarchaeota with Raz:ATP. Trend lines and associated statistics in (C,D) were derived with linear regressions. Thaumarchaeota and Betaproteobacteria are shown as closed squares and open triangles, respectively, in (A,B,D) with trends for each group shown with a solid (Thaumarchaeota) or dashed (Betaproteobacteria) line.
Our results show pronounced seasonal changes in hydrologic and microbial characteristics within the Columbia River hyporheic zone, as well as variation in the importance of selection and dispersal in structuring attached vs. planktonic communities. These community assembly processes operated at distinct timescales in each habitat and were also associated with changes in the relative abundance of certain taxa. In particular, changes in selection exhibited contrasting relationships with putative heterotrophic and autotrophic taxa in attached communities. Further, changes in community-level microbial metabolism correlated with the abundance of these same taxa in attached communities. Based on our findings, we present a conceptual model applicable within both macrobial and microbial systems that links trait selection, organismal fitness, and ecosystem-level functioning in habitats that are characterized by opposing selective pressures across multiple timescales.
Hyporheic microbial community composition shifted in conjunction with seasonal changes in organic carbon concentration, temperature, and groundwater-surface water mixing conditions. These variables each explained some variation in temporal community dissimilarity within attached and planktonic communities, indicating potential influences of selection (e.g., mediated by environmental change) and dispersal (e.g., mediated by hydrologic transport) over microbial community composition (Table S8). Both selection by the geochemical environment and dispersal from local sediment communities have been demonstrated within the groundwater aquifer in our system (Stegen et al., 2012); however, the balance of these two processes in structuring hyporheic microbial communities remains unclear.
Our results indicate that homogeneous selection (i.e., consistently imposed selection for a given set of traits, βNTI < -2) was the dominant assembly process in attached communities, while planktonic communities were influenced by a combination of homogeneous selection, variable selection (i.e., selective pressures that change through space or time, βNTI > 2), and spatial processes (i.e., dispersal, -2 < βNTI < 2 and |RCbray| > 0.95). Because planktonic communities consistently displayed higher βNTI values in comparison to attached communities, often with -2 < βNTI < 2, dispersal processes may play a greater role in structuring planktonic vs. attached communities. In contrast, attached communities always yielded large negative βNTI values suggesting that features of the physical sediment environment may impose stronger selective pressures than aqueous physicochemistry.
Because we observed pronounced seasonal trends in species richness associated with changes in the environment, we used null modeling to assess the extent to which selection versus dispersal influenced community composition across changes in species richness. Homogenous selection prevailed in attached communities as differences in species richness increased (Figure 2B), indicating strong and consistent selective pressures imposed by a relatively stable environment across temporal changes in microbial diversity. Strong consistent selection through time in attached communities suggests that the physical substrate may inherently contain a limited number of ecological niches—potentially related to mineralogy or physical structure—with slow changes in available niche space. In this case, temporal increases in richness were likely due to the addition of taxa that were ecologically similar to existing taxa and occupied similar niche space.
In contrast, differences in planktonic species richness were positively correlated with βNTI, with βNTI values supporting a role for more variable selection as differences in richness increased (Figure 2C). In this case, increases in species richness were likely due to the addition of taxa occupying newly available niche space generated by changes in the selective environment, yielding new taxa that were ecologically dissimilar to existing taxa.
Variation in assembly processes between attached and planktonic communities may be due to inherent differences among these environments, such as influences of mineralogy (Carson et al., 2007; Jorgensen et al., 2012), physical matrix composition (Vos et al., 2013; Breulmann et al., 2014), and/or relative rates of change in environment characteristics (discussed below). Further, differences in assembly processes and niche dynamics between the attached and planktonic communities may also be reflective of differing rates of organismal response to fluctuations in the hyporheic environment.
The timescales at which selection imposes constraints on microbial community composition are poorly understood (Shade et al., 2012; Nemergut et al., 2013). Here, we provide new insights into these timescales within the hyporheic zone, showing that selection on planktonic communities operates at the timescale of shifting porewater conditions (sub-hourly to seasonal), while selection on attached communities operates primarily at seasonal timescales (Figure 3). Both communities experienced a seasonal change in composition; however, variation in planktonic communities correlated with porewater physicochemistry at both the sub-hourly and seasonal timescales (Figure 3A, red and blue arrows, respectively). In our system, planktonic organisms may therefore be influenced by short-term fluctuations in groundwater-surface mixing, either through rapid changes in the selective environment or dispersal via hydrologic transport.
In contrast, selection on attached communities was detectable only at the seasonal timescale and exhibited resistance to short-term hydrologic fluctuations (Figure 3B). This short-term stability could be facilitated by a number of potentially complementary mechanisms. For example, attached communities may reside within biofilms, whereby microbial cells are imbedded in a matrix of extracellular polymeric substances. Biofilms are prevalent in aquatic systems and buffer communities against fluctuations in the hydrologic environment (Battin et al., 2016). Attached microbial communities may also have adhesion mechanisms (Hori and Matsumoto, 2010) that confer stability. Community assembly processes, such as priority effects, may also contribute to relatively slow rates of community turnover (Fukami, 2004; Fukami et al., 2010). Attached communities contained more species (on average) than planktonic communities (Figure 1D), further suggesting that temporal stability in attached communities may be enhanced by high species richness reducing susceptibility to invasion (Stachowicz et al., 2002).
Taxon-specific assembly processes are masked when relating βNTI and RCbray to environmental variables. To elucidate taxon-specific selection and dispersal mechanisms, we compared the relative abundance of taxa identified by SIMPER to βNTI and RCbray values.
In light of rapid hydrologic fluctuations in the porewater environment, relationships between RCbray and taxa abundances in planktonic communities provide evidence for a role of taxon-specific dispersal mechanisms (Table S6). In particular, positive relationships of Thaumarchaeota (Figure 4A) and a class of Acidobacteria (Figure 4B) with RCbray and negative relationships of Actinobacteria (Figure 4C) and Alphaproteobacteria (Figure 4D) with RCbray were among the strongest correlations (Table S6). These positive or negative relationships indicate higher or lower relative abundances, respectively, under higher levels of dispersal limitation. No relationships existed between planktonic taxa abundances and βNTI.
Although we cannot be certain of the mechanisms responsible for RCbray-abundance relationships in planktonic communities, the trends we observed help elucidate ecological dynamics impacting the abundance of microbial taxa within hyporheic zones. For example, taxa showing positive relationships with RCbray—Acidobacteria and Thaumarchaeota—are widely distributed globally (Francis et al., 2005; Fierer and Jackson, 2006; Jones et al., 2009; Pester et al., 2011), suggesting these organisms may be able to disperse under community-level dispersal limitation. Conversely, taxa exhibiting negative relationships with RCbray have physiologies that may diminish dispersal ability. Alphaproteobacteria can produce filaments that aid in attachment (Kragelund et al., 2006; Jones et al., 2007), and dispersal limitation has been demonstrated in soil Actinobacteria (Eisenlord et al., 2012). Thus, negative relationships between these taxa and RCbray may reflect an enhanced ability of these organisms to persist locally relative to other community members.
Additionally, when we examined assembly processes governing differences between attached and planktonic communities, we observed selection for microbial taxa in planktonic communities with unique ecological properties (Table S6). No correlations with RCbray were found in these comparisons; however, we identified positive relationships between βNTI and the average relative abundance of two classes of organisms—a candidate class of archaea (Parvarchaeota, Figure 4D) and a class of the candidate phylum OP3 (koll11, Figure 4E). Positive correlations between a particular taxon and βNTI across habitat types imply that the taxon increases in abundance as the habitats diverge in selective environments (i.e., selection becomes more variable).
In our system, Parvarchaeota and koll11 were almost exclusively found in planktonic communities suggesting that selection in the porewater environment favors these organisms. Although the specific selective pressures regulating the abundance of these organisms are unknown, archaea and members of the PVC superphyla to which OP3 belongs have a cell membrane lacking peptidoglycan that conveys resistance to common antibiotics and have the genetic potential to metabolize C1 compounds such as methane (Fuerst and Sagulenko, 2011). The distinctive features of these organisms and abundance within our system merits future investigating into their role in carbon cycling in hyporheic environments.
Finally, we observed changes in the abundance of two major taxa—Betaproteobacteria and Thaumarchaeota—within attached communities that correlated with changes in βNTI. Members of Betaproteobacteria increased in relative abundance with increases in the strength of homogeneous selection (Figure 4H), which occurred during times of low groundwater intrusion (Figures 5A,B). In contrast, members of Thaumarchaeota (Figure 4G) increased as homogeneous selection waned during times of high groundwater intrusion (Figures 5A,B). In this scenario positive correlations between a taxon and βNTI indicate that the taxon becomes more abundant as homogeneous selection wanes, and thus, that selection targets traits outside the taxon. In contrast, negative correlations between a taxon and βNTI should indicate that the primary selective pressure is for traits contained within that taxon. Because organisms with wider niche breadths are favored by selection in a greater variety of environmental conditions, positive relationships should be more probable for organisms occupying niches defined by a narrow subset of environmental attributes, whereas negative relationships should be more probable for organisms occupying broader niches. Indeed, Betaproteobacteria and Thaumarchaeota, respectively, contain organisms with diverse and limited metabolic capabilities (Amakata et al., 2005; Yang et al., 2005; Sato et al., 2009; Pester et al., 2011; Beam et al., 2014; Weber et al., 2015).
Shifts in the relative abundances of Betaproteobacteria and Thaumarchaeota also correlate with changes in groundwater-surface water mixing and in rates of microbial metabolism, denoting a link between community assembly processes and seasonal trends in autotrophic vs. heterotrophic metabolism. Betaproteobacteria is a metabolically diverse taxon, exhibiting a range of aerobic and facultative metabolisms including methylotrophy (Kalyuzhnaya et al., 2006), ammonia-oxidation (Freitag et al., 2006), nitrogen fixation (Rees et al., 2009), phototrophy (Gifford et al., 2013), and a variety of heterotrophic metabolisms (Amakata et al., 2005; Yang et al., 2005; Sato et al., 2009). Although we cannot be certain of the primary metabolic role(s) of these organisms, a positive correlation between their abundance and NPOC concentration supports their important contribution to heterotrophy in this system (Table S7). Betaproteobacteria remained in high abundance relative to other organisms throughout our study period, despite a seasonal decline, possibly indicating a broad metabolic role for these organisms. In contrast, metabolic activity of Thaumarchaeota is primarily constrained to ammonia-oxidation (Pester et al., 2011; Beam et al., 2014; Weber et al., 2015). Thaumarchaeota abundance was negatively correlated with NPOC, consistent with their involvement in ammonia-oxidation (Table S7). The relative abundance of Thaumarchaeota also correlated with physicochemical properties associated with increased groundwater-surface water mixing (SO42, NO3, IC, Table S7), suggesting a heightened importance of Thaumarchaeota-mediated nitrification when enhanced groundwater discharge into the hyporheic zone leads to organic carbon limitation (Taylor and Townsend, 2010).
Taken together, we hypothesize that selective pressures, both from sediment composition and from porewater physicochemistry, favor diverse heterotrophs within Betaproteobacteria. However, when the porewater environment changes due to a seasonal change in groundwater-surface water mixing, selective pressures shift to facilitate ammonia-oxidizing Thaumarchaeota. More broadly, we propose that community composition in dynamic environments is often the product of multiple selective pressures that operate across different timescales, resulting in an increase of specialist organisms during periods in which selection by an oscillating environment (e.g., hydrologic change) opposes that of a temporally stable environment (e.g., consistent sediment chemistry).
Importantly, changes in community composition in our system are associated with a seasonal increase in microbial metabolism, consistent with work in both micro- and macroecology demonstrating that productivity increases with niche diversification (Hooper et al., 2005; Cardinale et al., 2007; Cardinale, 2011; Gravel et al., 2011; Hunting et al., 2015). Specifically, we observed positive correlations between Raz:ATP and Thaumarchaeota abundance coincident with negative correlations between Raz:ATP and Betaproteobacteria (Figure 5D). We therefore infer that seasonally fluctuating selective pressures from the porewater environment impact microbial metabolism via their influences on niche dynamics. We propose that community-level niche diversification generated by a seasonal rise of specialized autotrophs leads to an increase in community metabolic activity despite selection for heterotrophs imposed by the consistent sediment environment.
Our findings lead to a conceptual model describing relationships between trait selection, organismal fitness, and ecosystem functioning for communities experiencing multiple selective pressures (Figure 6). The conceptual model focuses on the combined influences of stable and oscillating selective pressures, which should be prevalent across ecosystems. For example, in terrestrial ecosystems, physical soil properties and soil water content are relatively stable and oscillating, respectively, from the perspective of associated plant communities. Likewise, in subsurface systems (such as presented here), sediment geochemistry is relatively stable over monthly timescales and hydrologic conditions are continuously fluctuating generating dual selective pressures for benthic organisms.
FIGURE 6. Figure depicts a conceptual model describing relationships between trait selection, organismal fitness, and microbial metabolism for communities experiencing dual selective pressures. (A) Selection for a trait follows a continuous gradient within a stable environment (light blue) and oscillating environment (blue to red gradient). Organisms that contain opposing traits (dashed vs. solid lines) are favored at each end of the spectrum, delineated here as to the left (selection against trait 1 and for trait 2) or right (selection for trait 1 and against trait 2) of the vertical gray line. Given selection in a stable environment denoted by the black dot in (A), variation in homogeneous selection (B) is driven by the magnitude and direction of selection in the oscillating environment. When selection in the oscillating environment opposes selection in the stable environment, homogeneous selection decreases (B) and microbial metabolism increases (C) due to an increase in realized niche space and biodiversity. Blue and red triangles in (B,C) correspond to oscillating selection locations on the fitness landscape in (A).
We specifically hypothesize that selection imposed by a stable feature of the environment can favor organisms possessing traits that oppose traits selected for by the oscillating environment (Figure 6A). Because the stable environment applies consistent selective pressures, shifts in the strength of homogeneous selection are driven by changes in the oscillating environment. For instance, given a stable environment represented by the black dot in Figure 6A, the strength of homogeneous selection in Figure 6B is influenced by selection imparted by the oscillating environment, denoted as a gradient from blue to red. In this scenario, increased selection from the oscillating environment causes a decrease in homogeneous selection that results in niche diversification and enhanced microbial metabolism (Figures 6B,C).
While future work is needed to validate our conceptual model, our research represents a key step forward in spatiotemporal ecological research by assimilating shifts in community composition, assembly processes, and microbial metabolism. We develop this model by repeatedly sampling attached and planktonic microbial communities within an environmental transition zone over a 9-month time period. Our results indicate a rise in specialist autotrophs associated with increased rates of microbial metabolism when the direction of selection imposed by an oscillating hydrologic environment opposes that of stable selective pressures imposed by sediments. Further, we show that assembly processes operate at different temporal scales in planktonic and attached communities and have taxon-specific effects within each community. Our conceptual model can be applied in a range of ecological contexts beyond microbiology-for example, as a framework for understanding relationships between environmental change and competition under the Stress-Dominance hypothesis in macroecology (Brown et al., 1996; Normand et al., 2009) and for developing predictive models of species distributions under novel climate scenarios. As a whole, our work is an advancement in the integration of individual and community-level ecology theory with ecosystem function and develops a conceptual framework for coordinating assembly processes, changes in species abundance, and predictions of ecosystem-level functioning in response to environmental change.
EG was responsible for analyzing all data and writing with guidance from JS and JF. All other authors contributed substantially to experimental design, data collection, and manuscript revisions.
The authors declare that the research was conducted in the absence of any commercial or financial relationships that could be construed as a potential conflict of interest.
This research was supported by the US Department of Energy (DOE), Office of Biological and Environmental Research (BER), as part of Subsurface Biogeochemical Research Program’s Scientific Focus Area (SFA) at the Pacific Northwest National Laboratory (PNNL). PNNL is operated for DOE by Battelle under contract DE-AC06-76RLO 1830. A portion of the research was performed using Institutional Computing at PNNL.
The Supplementary Material for this article can be found online at: http://journal.frontiersin.org/article/10.3389/fmicb.2016.01949/full#supplementary-material
Amakata, D., Matsuo, Y., Shimono, K., Park, J. K., Yun, C. S., Matsuda, H., et al. (2005). Mitsuaria chitosanitabida gen. nov., sp. nov., an aerobic, chitosanase-producing member of the ‘Betaproteobacteria’. Int. J. Syst. Evol. Microbiol. 55, 1927–1932. doi: 10.1099/ijs.0.63629-0
Arntzen, E. V., Geist, D. R., and Dresel, P. E. (2006). Effects of fluctuating river flow on groundwater/surface water mixing in the hyporheic zone of a regulated, large cobble bed river. River Res. Appl. 22, 937–946. doi: 10.1002/rra.947
Battin, T. J., Besemer, K., Bengtsson, M. M., Romani, A. M., and Packmann, A. I. (2016). The ecology and biogeochemistry of stream biofilms. Nat. Rev. Microbiol. 14, 251–263. doi: 10.1038/nrmicro.2016.15
Beam, J. P., Jay, Z. J., Kozubal, M. A., and Inskeep, W. P. (2014). Niche specialization of novel Thaumarchaeota to oxic and hypoxic acidic geothermal springs of Yellowstone National Park. ISME J. 8, 938–951. doi: 10.1038/ismej.2013.193
Boulton, A. J., Datry, T., Kasahara, T., Mutz, M., and Stanford, J. A. (2010). Ecology and management of the hyporheic zone: stream-groundwater interactions of running waters and their floodplains. J. N. Am. Benthol. Soc. 29, 26–40. doi: 10.1899/08-017.1
Breulmann, M., Masyutenko, N. P., Kogut, B. M., Schroll, R., Dörfler, U., Buscot, F., et al. (2014). Short-term bioavailability of carbon in soil organic matter fractions of different particle sizes and densities in grassland ecosystems. Sci. Total Environ. 497, 29–37. doi: 10.1016/j.scitotenv.2014.07.080
Brown, J. H., Stevens, G. C., and Kaufman, D. M. (1996). The geographic range: size, shape, boundaries, and internal structure. Annu. Rev. Ecol. Syst. 27, 597–623. doi: 10.1146/annurev.ecolsys.27.1.597
Caporaso, J. G., Kuczynski, J., Stombaugh, J., Bittinger, K., Bushman, F. D., Costello, E. K., et al. (2010). QIIME allows analysis of high-throughput community sequencing data. Nat. Methods 7, 335–336. doi: 10.1038/nmeth.f.303
Cardinale, B. J. (2011). Biodiversity improves water quality through niche partitioning. Nature 472, 86–89. doi: 10.1038/nature09904
Cardinale, B. J., Wright, J. P., Cadotte, M. W., Carroll, I. T., Hector, A., Srivastava, D. S., et al. (2007). Impacts of plant diversity on biomass production increase through time because of species complementarity. Proc. Natl. Acad. Sci. U.S.A. 104, 18123–18128. doi: 10.1073/pnas.0709069104
Carson, J. K., Rooney, D., Gleeson, D. B., and Clipson, N. (2007). Altering the mineral composition of soil causes a shift in microbial community structure. FEMS Microbiol. Ecol. 61, 414–423. doi: 10.1111/j.1574-6941.2007.00361.x
Clarke, K. R. (1993). Non-parametric multivariate analyses of changes in community structure. Aust. J. Ecol. 18, 117–143. doi: 10.1111/j.1442-9993.1993.tb00438.x
Dini-Andreote, F., Stegen, J. C., Van Elsas, J. D., and Salles, J. F. (2015). Disentangling mechanisms that mediate the balance between stochastic and deterministic processes in microbial succession. Proc. Natl. Acad. Sci. U.S.A. 112, E1326–E1332. doi: 10.1073/pnas.1414261112
Eisenlord, S. D., Zak, D. R., and Upchurch, R. A. (2012). Dispersal limitation and the assembly of soil Actinobacteria communities in a long-term chronosequence. Ecol. Evol. 2, 538–549. doi: 10.1002/ece3.210
Fierer, N., and Jackson, R. B. (2006). The diversity and biogeography of soil bacterial communities. Proc. Natl. Acad. Sci. U.S.A. 103, 626–631. doi: 10.1073/pnas.0507535103
Fine, P. V. A., and Kembel, S. W. (2011). Phylogenetic community structure and phylogenetic turnover across space and edaphic gradients in western Amazonian tree communities. Ecography 34, 552–565. doi: 10.1111/j.1600-0587.2010.06548.x
Francis, C. A., Roberts, K. J., Beman, J. M., Santoro, A. E., and Oakley, B. B. (2005). Ubiquity and diversity of ammonia-oxidizing archaea in water columns and sediments of the ocean. Proc. Natl. Acad. Sci. U.S.A. 102, 14683–14688. doi: 10.1073/pnas.0506625102
Freitag, T. E., Chang, L., and Prosser, J. I. (2006). Changes in the community structure and activity of betaproteobacterial ammonia-oxidizing sediment bacteria along a freshwater–marine gradient. Environ. Microbiol. 8, 684–696. doi: 10.1111/j.1462-2920.2005.00947.x
Fuerst, J. A., and Sagulenko, E. (2011). Beyond the bacterium: planctomycetes challenge our concepts of microbial structure and function. Nat. Rev. Microbiol. 9, 403–413. doi: 10.1038/nrmicro2578
Fukami, T. (2004). Assembly history interacts with ecosystem size to influence species diversity. Ecology 85, 3234–3242. doi: 10.1890/04-0340
Fukami, T., Dickie, I. A., Paula Wilkie, J., Paulus, B. C., Park, D., Roberts, A., et al. (2010). Assembly history dictates ecosystem functioning: evidence from wood decomposer communities. Ecol. Lett. 13, 675–684. doi: 10.1111/j.1461-0248.2010.01465.x
Gifford, S. M., Sharma, S., Booth, M., and Moran, M. A. (2013). Expression patterns reveal niche diversification in a marine microbial assemblage. ISME J. 7, 281–298. doi: 10.1038/ismej.2012.96
Gonzalez, A., King, A., Robeson, M. S. I. I., Song, S., Shade, A., Metcalf, J. L., et al. (2012). Characterizing microbial communities through space and time. Curr. Opin. Biotechnol. 23, 431–436. doi: 10.1016/j.copbio.2011.11.017
Graham, E. B., Knelman, J. E., Schindlbacher, A., Siciliano, S., Breulmann, M., Yannarell, A., et al. (2016). Microbes as engines of ecosystem function: when does community structure enhance predictions of ecosystem processes? Front. Microbiol. 7:214. doi: 10.3389/fmicb.2016.00214
Graham, E. B., Wieder, W. R., Leff, J. W., Weintraub, S. R., Townsend, A. R., Cleveland, C. C., et al. (2014). Do we need to understand microbial communities to predict ecosystem function? A comparison of statistical models of nitrogen cycling processes. Soil Biol. Biochem. 68, 279–282.
Gravel, D., Bell, T., Barbera, C., Bouvier, T., Pommier, T., Venail, P., et al. (2011). Experimental niche evolution alters the strength of the diversity-productivity relationship. Nature 469, 89–92. doi: 10.1038/nature09592
Grime, J. (1998). Benefits of plant diversity to ecosystems: immediate, filter and founder effects. J. Ecol. 86, 902–910. doi: 10.1046/j.1365-2745.1998.00306.x
Hancock, P. J., Boulton, A. J., and Humphreys, W. F. (2005). Aquifers and hyporheic zones: towards an ecological understanding of groundwater. Hydrogeol. J. 13, 98–111. doi: 10.1007/s10040-004-0421-6
Hanson, C. A., Fuhrman, J. A., Horner-Devine, M. C., and Martiny, J. B. H. (2012). Beyond biogeographic patterns: processes shaping the microbial landscape. Nat. Rev. Microbiol. 10, 497–506. doi: 10.1038/nrmicro2795
Hawkes, C. V., and Keitt, T. H. (2015). Resilience vs. historical contingency in microbial responses to environmental change. Ecol. Lett. 18, 612–625. doi: 10.1111/ele.12451
Hooper, D. U., Adair, E. C., Cardinale, B. J., Byrnes, J. E., Hungate, B. A., Matulich, K. L., et al. (2012). A global synthesis reveals biodiversity loss as a major driver of ecosystem change. Nature 486, 105–108. doi: 10.1038/nature11118
Hooper, D. U., Chapin Iii, F., Ewel, J., Hector, A., Inchausti, P., Lavorel, S., et al. (2005). Effects of biodiversity on ecosystem functioning: a consensus of current knowledge. Ecol. Monogr. 75, 3–35. doi: 10.1111/brv.12110
Hori, K., and Matsumoto, S. (2010). Bacterial adhesion: from mechanism to control. Biochem. Eng. J. 48, 424–434. doi: 10.1016/j.bej.2009.11.014
Hunting, E. R., Vijver, M. G., Van Der Geest, H. G., Mulder, C., Kraak, M. H. S., Breure, A. M., et al. (2015). Resource niche overlap promotes stability of bacterial community metabolism in experimental microcosms. Front. Microbiol. 6:105. doi: 10.3389/fmicb.2015.00105
Jones, P. R., Cottrell, M. T., Kirchman, D. L., and Dexter, S. C. (2007). Bacterial community structure of biofilms on artificial surfaces in an estuary. Microb. Ecol. 53, 153–162. doi: 10.1007/s00248-006-9154-5
Jones, R. T., Robeson, M. S., Lauber, C. L., Hamady, M., Knight, R., and Fierer, N. (2009). A comprehensive survey of soil acidobacterial diversity using pyrosequencing and clone library analyses. ISME J. 3, 442–453. doi: 10.1038/ismej.2008.127
Jorgensen, S. L., Hannisdal, B., Lanzén, A., Baumberger, T., Flesland, K., Fonseca, R., et al. (2012). Correlating microbial community profiles with geochemical data in highly stratified sediments from the Arctic Mid-Ocean Ridge. Proc. Natl. Acad. Sci. U.S.A. 109, E2846–E2855. doi: 10.1073/pnas.1207574109
Kalyuzhnaya, M. G., De Marco, P., Bowerman, S., Pacheco, C. C., Lara, J. C., Lidstrom, M. E., et al. (2006). Methyloversatilis universalis gen. nov., sp. nov., a novel taxon within the Betaproteobacteria represented by three methylotrophic isolates. Int. J. Syst. Evol. Microbiol. 56, 2517–2522. doi: 10.1099/ijs.0.64422-0
Knelman, J. E., and Nemergut, D. R. (2014). Changes in community assembly may shift the relationship between biodiversity and ecosystem function. Front. Microbiol. 5:424. doi: 10.3389/fmicb.2014.00424
Kragelund, C., Kong, Y., Van Der Waarde, J., Thelen, K., Eikelboom, D., Tandoi, V., et al. (2006). Ecophysiology of different filamentous Alphaproteobacteria in industrial wastewater treatment plants. Microbiology 152, 3003–3012. doi: 10.1099/mic.0.29249-0
Krause, S., Le Roux, X., Niklaus, P. A., Van Bodegom, P. M., Lennon, J. T., Bertilsson, S., et al. (2014). Trait-based approaches for understanding microbial biodiversity and ecosystem functioning. Front. Microbiol. 5:251. doi: 10.3389/fmicb.2014.00251
Lebrija-Trejos, E., Pérez-García, E. A., Meave, J. A., Bongers, F., and Poorter, L. (2010). Functional traits and environmental filtering drive community assembly in a species-rich tropical system. Ecology 91, 386–398. doi: 10.1890/08-1449.1
Lin, X., Mckinley, J., Resch, C. T., Kaluzny, R., Lauber, C. L., Fredrickson, J., et al. (2012). Spatial and temporal dynamics of the microbial community in the Hanford unconfined aquifer. ISME J. 6, 1665–1676. doi: 10.1038/ismej.2012.26
Lindström, E. S., and Langenheder, S. (2012). Local and regional factors influencing bacterial community assembly. Environ. Microbiol. Rep. 4, 1–9. doi: 10.1111/j.1758-2229.2011.00257.x
Lindström, E. S., and Östman, Ö (2011). The importance of dispersal for bacterial community composition and functioning. PLoS ONE 6:e25883. doi: 10.1371/journal.pone.0025883
Loreau, M., and Hector, A. (2001). Partitioning selection and complementarity in biodiversity experiments. Nature 412, 72–76. doi: 10.1038/35083573
Martiny, J. B. H., Bohannan, B. J. M., Brown, J. H., Colwell, R. K., Fuhrman, J. A., Green, J. L., et al. (2006). Microbial biogeography: putting microorganisms on the map. Nat. Rev. Microbiol. 4, 102–112. doi: 10.1038/nrmicro1341
Nemergut, D. R., Schmidt, S. K., Fukami, T., O’neill, S. P., Bilinski, T. M., Stanish, L. F., et al. (2013). Patterns and processes of microbial community assembly. Microbiol. Mol. Biol. Rev. 77, 342–356. doi: 10.1128/MMBR.00051-12
Nemergut, D. R., Shade, A., and Violle, C. (2014). When, where and how does microbial community composition matter? Front. Microbiol. 5:497. doi: 10.3389/fmicb.2014.00497
Normand, S., Treier, U. A., Randin, C., Vittoz, P., Guisan, A., and Svenning, J. C. (2009). Importance of abiotic stress as a range-limit determinant for European plants: insights from species responses to climatic gradients. Global Ecol. Biogeogr. 18, 437–449. doi: 10.1111/j.1466-8238.2009.00451.x
Oksanen, J., Blanchet, F. G., Kindt, R., Legendre, P., Minchin, P. R., O’hara, R. B., et al. (2013). Package ‘Vegan’. Community Ecology Package, Version 2.
Peres, C. A., Emilio, T., Schietti, J., Desmoulière, S. J., and Levi, T. (2016). Dispersal limitation induces long-term biomass collapse in overhunted Amazonian forests. Proc. Natl. Acad. Sci. U.S.A. 113, 892–897. doi: 10.1073/pnas.1516525113
Pester, M., Schleper, C., and Wagner, M. (2011). The Thaumarchaeota: an emerging view of their phylogeny and ecophysiology. Curr. Opin. Microbiol. 14, 300–306. doi: 10.1016/j.mib.2011.04.007
Peterson, R. E., and Connelly, M. P. (2004). Water movement in the zone of interaction between groundwater and the Columbia River, Hanford site, Washington. J. Hydraul. Res. 42, 53–58. doi: 10.1016/j.jconhyd.2013.02.001
Poff, N. L. (1997). Landscape filters and species traits: towards mechanistic understanding and prediction in stream ecology. J. N. Am. Benthol. Soc. 16, 391–409. doi: 10.2307/1468026
Prosser, J. I., Bohannan, B. J., Curtis, T. P., Ellis, R. J., Firestone, M. K., Freckleton, R. P., et al. (2007). The role of ecological theory in microbial ecology. Nat. Rev. Microbiol. 5, 384–392. doi: 10.1038/nrmicro1643
Rees, A. P., Gilbert, J. A., and Kelly-Gerreyn, B. A. (2009). Nitrogen fixation in the western English Channel (NE Atlantic ocean). Mar. Ecol. Prog. Ser. 374, 7–12. doi: 10.3354/meps07771
Sato, K., Kato, Y., Taguchi, G., Nogawa, M., Yokota, A., and Shimosaka, M. (2009). Chitiniphilus shinanonensis gen. nov., sp. nov., a novel chitin-degrading bacterium belonging to Betaproteobacteria. J. Gen. Appl. Microbiol. 55,147–153. doi: 10.2323/jgam.55.147
Shade, A., Caporaso, J. G., Handelsman, J., Knight, R., and Fierer, N. (2013). A meta-analysis of changes in bacterial and archaeal communities with time. ISME J. 7, 1493–1506. doi: 10.1038/ismej.2013.54
Shade, A., Peter, H., Allison, S. D., Baho, D. L., Berga, M., Bürgmann, H., et al. (2012). Fundamentals of microbial community resistance and resilience. Front. Microbiol. 3:417. doi: 10.3389/fmicb.2012.00417
Slater, L. D., Ntarlagiannis, D., Day-Lewis, F. D., Mwakanyamale, K., Versteeg, R. J., Ward, A., et al. (2010). Use of electrical imaging and distributed temperature sensing methods to characterize surface water–groundwater exchange regulating uranium transport at the Hanford 300 Area, Washington. Water Resour. Res. 46, 1–13. doi: 10.1029/2010WR009110
Stachowicz, J. J., Fried, H., Osman, R. W., and Whitlatch, R. B. (2002). Biodiversity, invasion resistance, and marine ecosystem function: reconciling pattern and process. Ecology 83, 2575–2590. doi: 10.1890/0012-9658 (2002)083[2575:BIRAME]2.0.CO;2
Stegen, J. C., Fredrickson, J. K., Wilkins, M. J., Konopka, A. E., Nelson, W. C., Arntzen, E. V., et al. (2016). Groundwater-surface water mixing shifts ecological assembly processes and stimulates organic carbon turnover. Nat. Commun. 7, 11237. doi: 10.1038/ncomms11237
Stegen, J. C., Lin, X., Fredrickson, J. K., Chen, X., Kennedy, D. W., Murray, C. J., et al. (2013). Quantifying community assembly processes and identifying features that impose them. ISME J. 7, 2069–2079. doi: 10.1038/ismej.2013.93
Stegen, J. C., Lin, X., Fredrickson, J. K., and Konopka, A. E. (2015). Estimating and mapping ecological processes influencing microbial community assembly. Front. Microbiol. 6:370. doi: 10.3389/fmicb.2015.00370
Stegen, J. C., Lin, X., Konopka, A. E., and Fredrickson, J. K. (2012). Stochastic and deterministic assembly processes in subsurface microbial communities. ISME J. 6, 1653–1664. doi: 10.1038/ismej.2012.22
Taylor, P. G., and Townsend, A. R. (2010). Stoichiometric control of organic carbon–nitrate relationships from soils to the sea. Nature 464, 1178–1181. doi: 10.1038/nature08985
Telford, R. J., Vandvik, V., and Birks, H. J. B. (2006). Dispersal limitations matter for microbial morphospecies. Science 312, 1015–1015. doi: 10.1126/science.1125669
Tremlová, K. I., and Münzbergová, Z. (2007). Importance of species traits for species distribution in fragmented landscapes. Ecology 88, 965–977. doi: 10.1890/06-0924
Van der Gucht, K., Cottenie, K., Muylaert, K., Vloemans, N., Cousin, S., Declerck, S., et al. (2007). The power of species sorting: local factors drive bacterial community composition over a wide range of spatial scales. Proc. Natl. Acad. Sci. U.S.A. 104, 20404–20409. doi: 10.1073/pnas.0707200104
Vellend, M. (2010). Conceptual synthesis in community ecology. Q. Rev. Biol. 85, 183–206. doi: 10.1086/652373
Vos, M., Wolf, A. B., Jennings, S. J., and Kowalchuk, G. A. (2013). Micro-scale determinants of bacterial diversity in soil. FEMS Microbiol. Rev. 37, 936–954. doi: 10.1111/1574-6976.12023
Webb, C. O., Ackerly, D. D., and Kembel, S. W. (2008). Phylocom: software for the analysis of phylogenetic community structure and trait evolution. Bioinformatics 24, 2098–2100. doi: 10.1093/bioinformatics/btn358
Weber, E. B., Lehtovirta-Morley, L. E., Prosser, J. I., and Gubry-Rangin, C. (2015). Ammonia oxidation is not required for growth of Group 1.1 c soil Thaumarchaeota. FEMS Microbiol. Ecol. 91:fiv001. doi: 10.1093/femsec/fiv001
Yang, H.-C., Im, W.-T., An, D.-S., Park, W.-S., Kim, I. S., and Lee, S.-T. (2005). Silvimonas terrae gen. nov., sp. nov., a novel chitin-degrading facultative anaerobe belonging to the ‘Betaproteobacteria’. Int. J. Syst. Evol. Microbiol. 55, 2329–2332. doi: 10.1099/ijs.0.63837-0
Keywords: niche, selection, dispersal, microbial community structure, aerobic respiration, ammonia oxidation, hyporheic, Hanford
Citation: Graham EB, Crump AR, Resch CT, Fansler S, Arntzen E, Kennedy DW, Fredrickson JK and Stegen JC (2016) Coupling Spatiotemporal Community Assembly Processes to Changes in Microbial Metabolism. Front. Microbiol. 7:1949. doi: 10.3389/fmicb.2016.01949
Received: 30 August 2016; Accepted: 21 November 2016;
Published: 16 December 2016.
Edited by:
Alison Buchan, University of Tennessee, USAReviewed by:
Sonja Kristine Fagervold, UniversitéPierre et Marie Curie – UPMC (Paris 6), FranceCopyright © 2016 Graham, Crump, Resch, Fansler, Arntzen, Kennedy, Fredrickson and Stegen. This is an open-access article distributed under the terms of the Creative Commons Attribution License (CC BY). The use, distribution or reproduction in other forums is permitted, provided the original author(s) or licensor are credited and that the original publication in this journal is cited, in accordance with accepted academic practice. No use, distribution or reproduction is permitted which does not comply with these terms.
*Correspondence: Emily B. Graham, ZW1pbHkuZ3JhaGFtQHBubmwuZ292
Disclaimer: All claims expressed in this article are solely those of the authors and do not necessarily represent those of their affiliated organizations, or those of the publisher, the editors and the reviewers. Any product that may be evaluated in this article or claim that may be made by its manufacturer is not guaranteed or endorsed by the publisher.
Research integrity at Frontiers
Learn more about the work of our research integrity team to safeguard the quality of each article we publish.