- 1Department of Food Science and Technology, University of California, Davis, Davis, CA, USA
- 2Agricultural Biotechnology Center, National Chung Hsing University, Taichung, Taiwan
- 3Pennington Biomedical Research Center, Baton Rouge, LA, USA
- 4Louisiana State University Agricultural Center, Baton Rouge, LA, USA
- 5Western Human Nutrition Research Center, Davis, CA, USA
The intestinal microbiota are integral to understanding the relationships between nutrition and health. Therefore, fecal sampling and processing protocols for metagenomic surveys should be sufficiently robust, accurate, and reliable to identify the microorganisms present. We investigated the use of different fecal preparation methods on the bacterial community structures identified in human stools. Complete stools were collected from six healthy individuals and processed according to the following methods: (i) randomly sampled fresh stool, (ii) fresh stool homogenized in a blender for 2 min, (iii) randomly sampled frozen stool, and (iv) frozen stool homogenized in a blender for 2 min, or (v) homogenized in a pneumatic mixer for either 10, 20, or 30 min. High-throughput DNA sequencing of the 16S rRNA V4 regions of bacterial community DNA extracted from the stools showed that the fecal microbiota remained distinct between individuals, independent of processing method. Moreover, the different stool preparation approaches did not alter intra-individual bacterial diversity. Distinctions were found at the level of individual taxa, however. Stools that were frozen and then homogenized tended to have higher proportions of Faecalibacterium, Streptococcus, and Bifidobacterium and decreased quantities of Oscillospira, Bacteroides, and Parabacteroides compared to stools that were collected in small quantities and not mixed prior to DNA extraction. These findings indicate that certain taxa are at particular risk for under or over sampling due to protocol differences. Importantly, homogenization by any method significantly reduced the intra-individual variation in bacteria detected per stool. Our results confirm the robustness of fecal homogenization for microbial analyses and underscore the value of collecting and mixing large stool sample quantities in human nutrition intervention studies.
Introduction
The intestinal microbiota provide vital contributions to gastrointestinal (GI) and systemic health. The composition of bacterial species and associated metabolites in the human GI tract have been associated with multiple disorders, such as obesity and inflammatory bowel diseases (Ley et al., 2006; Flint et al., 2012; Hooper et al., 2012; Tremaroli and Backhed, 2012; Kabat et al., 2014). Importantly, these bacteria not only serve as indicators for disease but also as targets for therapeutic intervention. Many of the recent developments to our understanding of the intestinal microbiome have been the result of the application of next generation DNA sequencing methods targeting bacterial 16S rRNA genes for phylogenetic and taxonomic assessments of bacterial community membership (Liu et al., 2012). Because of the difficulty in collecting intestinal contents from human subjects, feces are typically used as the source to identify and characterize the intestinal microbiota. Although microbial composition differs along the length of the digestive tract (Eckburg et al., 2005; Stearns et al., 2011), fecal bacteria were shown to have a greater diagnostic value for patients with irritable bowel syndrome than those located in the gut mucosa (Rangel et al., 2015). Therefore, methods used to sample and examine the microorganisms in human fecal material should be optimally designed to ensure precision, accuracy, and a lack of significant bias introduced either by sample collection, homogenization, DNA extraction and sequencing, or data analysis.
Numerous studies have examined the effects of storage conditions, DNA extraction methods, and PCR parameters on the detection and quantification of bacteria in human stools. Several reports concluded that bacterial community composition was not affected by incubating human feces at room temperature for 24 h or 4°C for 48 h or freezing immediately after collection at either -20 or -80°C (Wu G.D. et al., 2010; Cardona et al., 2012; Carroll et al., 2012; Hang et al., 2014; Fouhy et al., 2015; Tedjo et al., 2015). The application of nucleic acid stabilizing reagents (such as RNAlater) have shown promise, but also significant limitations. Although the microbiota of stools stored in RNAlater were stable for 7 days at room temperature (Flores et al., 2015) and were subject-specific over time (Voigt et al., 2015), the incorporation of RNAlater for fecal sample storage decreased DNA quality (Dominianni et al., 2014) and yields (Gorzelak et al., 2015). The microbial diversity (Dominianni et al., 2014) and proportions of certain bacterial taxa were also affected (Nechvatal et al., 2008; Flores et al., 2015; Gorzelak et al., 2015).
Different commercial DNA extraction kits were found to be capable of recovering sufficient quantities of DNA (Scupham et al., 2007; Nechvatal et al., 2008; Salonen et al., 2010; Wu G.D. et al., 2010; Sergeant et al., 2012; Yuan et al., 2012; Hang et al., 2014; Kennedy et al., 2014; Santiago et al., 2014; Vishnivetskaya et al., 2014; Wesolowska-Andersen et al., 2014; Wagner Mackenzie et al., 2015), but yielded proportional changes in identified taxa (Salonen et al., 2010; Wu G.D. et al., 2010; Sergeant et al., 2012; Yuan et al., 2012; Hang et al., 2014; Kennedy et al., 2014; Santiago et al., 2014; Vishnivetskaya et al., 2014; Wesolowska-Andersen et al., 2014). Notably, physical shearing of cells by bead-beating improves DNA recovery (Salonen et al., 2010; Yuan et al., 2012; Santiago et al., 2014; Vishnivetskaya et al., 2014) and generally increases the proportions of Gram-positive bacteria that are difficult to lyse by enzymatic methods (Scupham et al., 2007; Sergeant et al., 2012).
PCR parameters, including the DNA polymerase, oligonucleotides, annealing temperature, and barcoding strategy varied the outcome in bacterial surveys (Frank et al., 2008; Engelbrektson et al., 2010; Wu J.Y. et al., 2010; Sergeant et al., 2012; Brandariz-Fontes et al., 2015; Franzen et al., 2015; Sinclair et al., 2015; Tremblay et al., 2015; Zheng et al., 2015; D’Amore et al., 2016; Derakhshani et al., 2016). The number of PCR amplification cycles altered estimates of low abundance bacterial taxa (Gonzalez et al., 2012) but did not change conclusions of the overall community structure (Wu J.Y. et al., 2010). The impacts of DNA sequencing platforms, including Ion Torrent, 454 pyrosequencing, and Illumina, on the outcomes of assessments of bacterial composition in stool samples were minor (Salipante et al., 2014; Sinclair et al., 2015; Tremblay et al., 2015; D’Amore et al., 2016). In contrast, bioinfomatics approaches can result in significant differences (Bokulich et al., 2013; Majaneva et al., 2015).
Despite these seemingly exhaustive comparisons of microbial analysis techniques, stool preparation methods have not been well-tested. Although the collection of small aliquots of stool is sufficient to perform bacterial analysis (e.g., 200 mg or less), it is not clear how well the bacterial species are distributed throughout the fecal contents. Therefore it is not known whether sampling from different spatial locations in the stool without homogenization is adequate to obtain data that are representative of the stool as a whole. This is particularly relevant for human studies wherein people consumer different foods throughout the day. Moreover, there has not been a comparative analysis of different fecal preparation methods, and less labor-intensive methods have often been favored. In preparation for optimal processing of stool samples from a study of the effects of dietary resistant starch in prediabetic participants, we measured the intra- and inter-individual variations in stool samples with and without freezing and with and without homogenization to evaluate the extent to which the bacterial proportions change as a result of processing steps.
Materials and Methods
Sample Collection and Preparation
The study design is provided in Figure 1. Complete stools were collected from six healthy individuals [Subjects (S) 1–6] and processed within 2 h after collection. A portion of the stool was frozen at -80°C, and the remaining was directly sampled (Fre_U) in <1 g portions or homogenized by blending. For blending, fresh stool was homogenized in a blender (Ninja Master Prep, QB900B, Euro-Pro Operating, Boston, MA) for 2 min (Fre_B). The frozen stool was randomly chipped in <1 g portions (Fro_U), mixed in a blender for 2 min (Fro_B), or homogenized in a pneumatic mixer for either 10, 20, or 30 min (P10, P20, and P30) (Miracle Paint Sport DC-1-C, Minneapolis, MN, USA). For each of those methods, the stools were processed while frozen. Sterile distilled water (4°C) was added to stools in a proportion of 1.5 times the sample weight (63.7 ± 29.9 g) for homogenization in the blender and pneumatic mixer. For homogenization in the pneumatic mixer, the frozen stool and 25 stainless steel balls (0.8 cm in diameter) were agitated in 3.79 l containers. A total of six replicates were collected for each of the seven preparation methods for each of the six participants and stored at -80°C until DNA extraction. The parent study [STARCH study] was approved by the PBRC’s institutional review board, was registered on clinicaltrials.gov (NCT01708694), and all participants provided written informed consent.
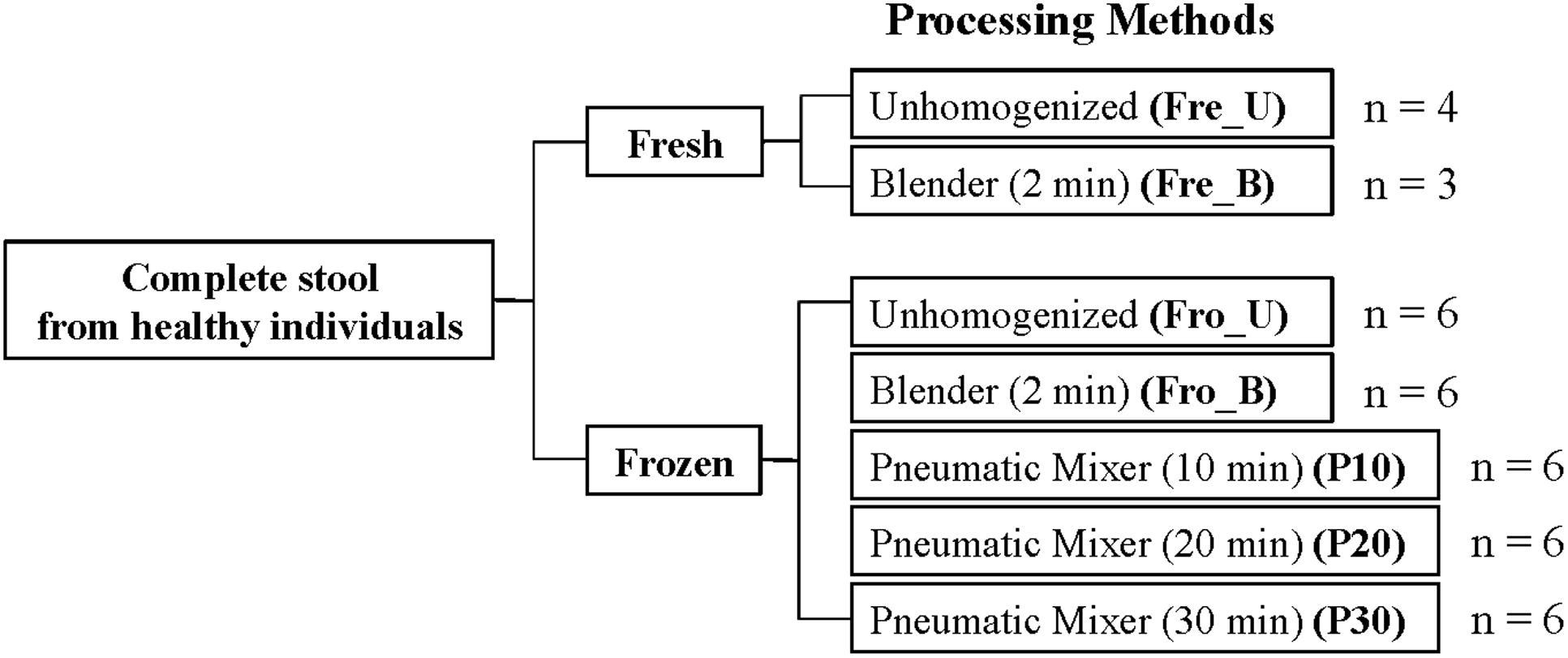
FIGURE 1. Fecal sample processing study design. An “n” indicates the number of human subjects for which stools were tested. For each processing method, six technical replicates were performed.
DNA Extraction
DNA was extracted and purified with either the QIAamp DNA Stool Mini Kit (Qiagen, Hilden, Germany; for samples from S1 to S3) or QIAamp Fast DNA Stool Mini Kit (for samples from S4 to S6) according to kit availability and with the following modifications: A total of 200–250 mg processed stool was added to tubes containing 300 mg of 0.1 mm zirconium beads (BioSpec Products, Inc. Bartlesville, OK, USA) suspended in 100 μl lysis buffer (200 mM of NaCl, 100 mM of Tris-HCl, 20 mM of EDTA and 20 mg/ml of lysozyme). After 30 min incubation with 500 rpm mixing at 37°C, the ASL buffer from the QIAamp DNA stool mini kit (or InhibitEX buffer from QIAamp Fast DNA Stool Mini Kit) was added into the sample mixture for homogenization with two cycles on a MP FastPrep-24 instrument (MP Bio MP Biomedicals LLC, Santa Ana, CA, USA) at 6.5 m/s for 1 min. The supernatant was further processed using QIAamp kits according to the manufacturer’s instructions and the purified DNA was stored at -20°C until PCR amplification.
Library Preparation for Illumina Sequencing
The V4 region of the 16S rRNA gene was amplified using primers 515F and 806R (Caporaso et al., 2011). An eight-nucleotide unique barcode was appended onto the 5′ end of the forward primer for sample identification as previously described (Yin et al., 2014). The target was amplified in a 50 μl reaction containing 5 ng of template DNA, 0.8 U Ex Taq DNA polymerase (Takara Bio Inc. Kyoto, Japan), 1× Ex Taq buffer, 2.5 mM MgCl2, 0.1 μM of each primer, and 200 μM dNTP mixture. Thermocycling reactions were as follows: 3 min of denaturation at 94°C, followed by 35 cycles (S1–S3) or 30 cycles (S4–S6) of 45 s at 94°C, 60 s at 50°C and 40 s at 72°C, and with a final extension for 10 min at 72°C. A total of 5 μl of each PCR product was pooled and visualized on an agarose gel prior to purification with a Wizard SV Gel and PCR Clean-Up System (Promega, Fitchburg, WI, USA). The concentration of the purified replicon mixture was measured on a NanoDrop (Thermo Scientific, Waltham, MA, USA) and then used for DNA library preparation and paired-end Illumina Mi-Seq (PE250) sequencing (Illumina Inc., San Diego, CA, USA) at the UC Davis Genome Center1.
16S rRNA Gene Sequence Analysis
Sequence analysis was performed using the pipeline QIIME version 1.8.0 (Caporaso et al., 2010). DNA sequences with an error in the barcode or those that did not pass a minimum average quality score of 30 were excluded while demultiplexing (-e 0 and -s 30). This resulted in an average of 67,056 ± 11,041 reads per sample, each with an average length of 222 ± 0.3 bases. The sequences were then clustered into OTUs by the open reference OTU picking approach in QIIME with default settings using the UCLUST method (Edgar, 2010) based on 97% sequence similarity against the Greengenes reference database version 13_8 (DeSantis et al., 2006). Upon removing OTUs constituting less than 0.005% of the total observed counts, a total of 935 OTUs in all stool samples remained. For alpha and beta diversity analysis, the samples were rarefied to 10,000 reads. The alpha diversity indices including observed OTUs, PD whole tree, and Shannon diversity were determined. Beta diversity was assessed by the weighted UniFrac distance (Lozupone et al., 2011) and Bray-Curtis dissimilarity, and visualized using PCoA. For alpha diversity and the within processing method distance, raw sequencing data were divided into six groups based on person and analyzed following the process as described above. Raw sequences are available in the NCBI SRA under BoProject ID PRJNA295801.
Statistical Analysis
Differences in alpha diversity of observed OTUs, PD whole tree, and Shannon indices were assessed by the Kruskal–Wallis test followed by Dunn’s post hoc test with a default Bonferroni correction in Graphpad Prism 6 (GraphPad Software Inc., La Jolla, CA, USA). The differences in the relative abundance of bacterial taxa were compared with the Mann–Whitney U-test or Kruskal–Wallis test followed by FDR correction in R “stats” package (R 3.1.1). The Dunn’s post hoc test in R “dunn.test” package was used to specify the processing method where the taxa were significantly altered. A P value < 0.05 was considered significant.
Results
Inter-individual Variation in Fecal Bacterial Composition Is Not Altered by Processing Method
Stools from six individuals were collected and prepared as described in Figure 1. PCR amplicons of bacterial community 16S rRNA gene V4 regions were subjected to high-throughput DNA sequencing and analysis. Comparisons of all stool preparation methods showed that the six individuals each harbored a distinct fecal microbial profile according to the weighted Unifrac metric (Figure 2). Although the different fecal preparation methods did result in some alterations to the bacterial diversity among the individuals, the dispersive level was inconsistent for each person (Figure 2). Bray–Curtis dissimilarity metrics confirmed that that subject was the prominent factor for sample clustering (data not shown). Taxonomic comparisons showed the inter-individual differences among the stools (Figure 3). At the phylum level, individuals were predominantly populated by Firmicutes and with variable levels of Bacteroides and Actinobacteria (Figure 3). Hierarchical clustering of the log-transformed proportions of the most abundant taxa confirmed that the bacterial composition in the fecal samples grouped according to subject and not by processing method (Figure 4).
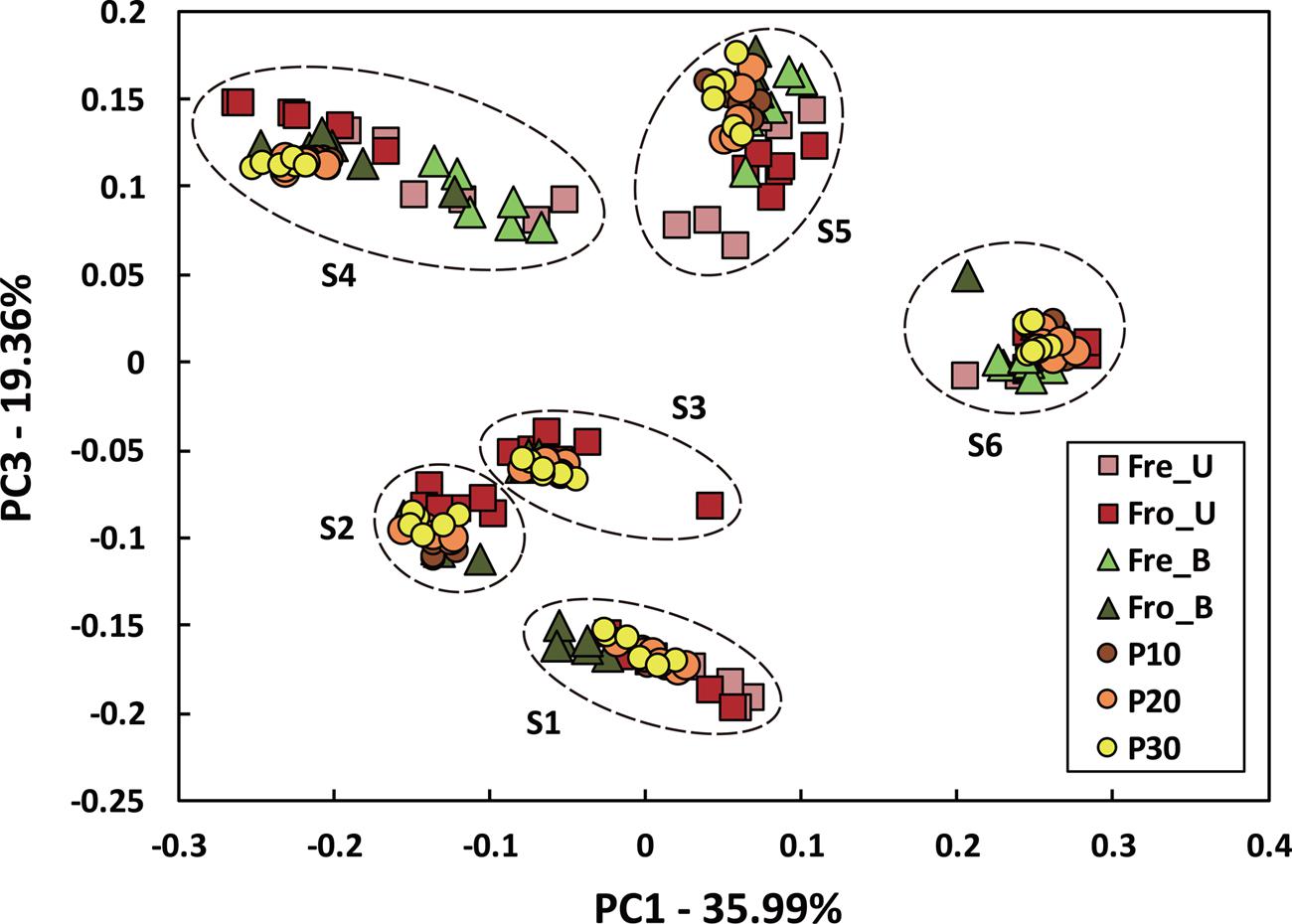
FIGURE 2. Inter- and intra-individual variation in microbiota composition according to different fecal processing methods. Bacterial diversity was visualized by PCoA using the weighted UniFrac metric and colored according to processing method. An “S” indicates subject number.
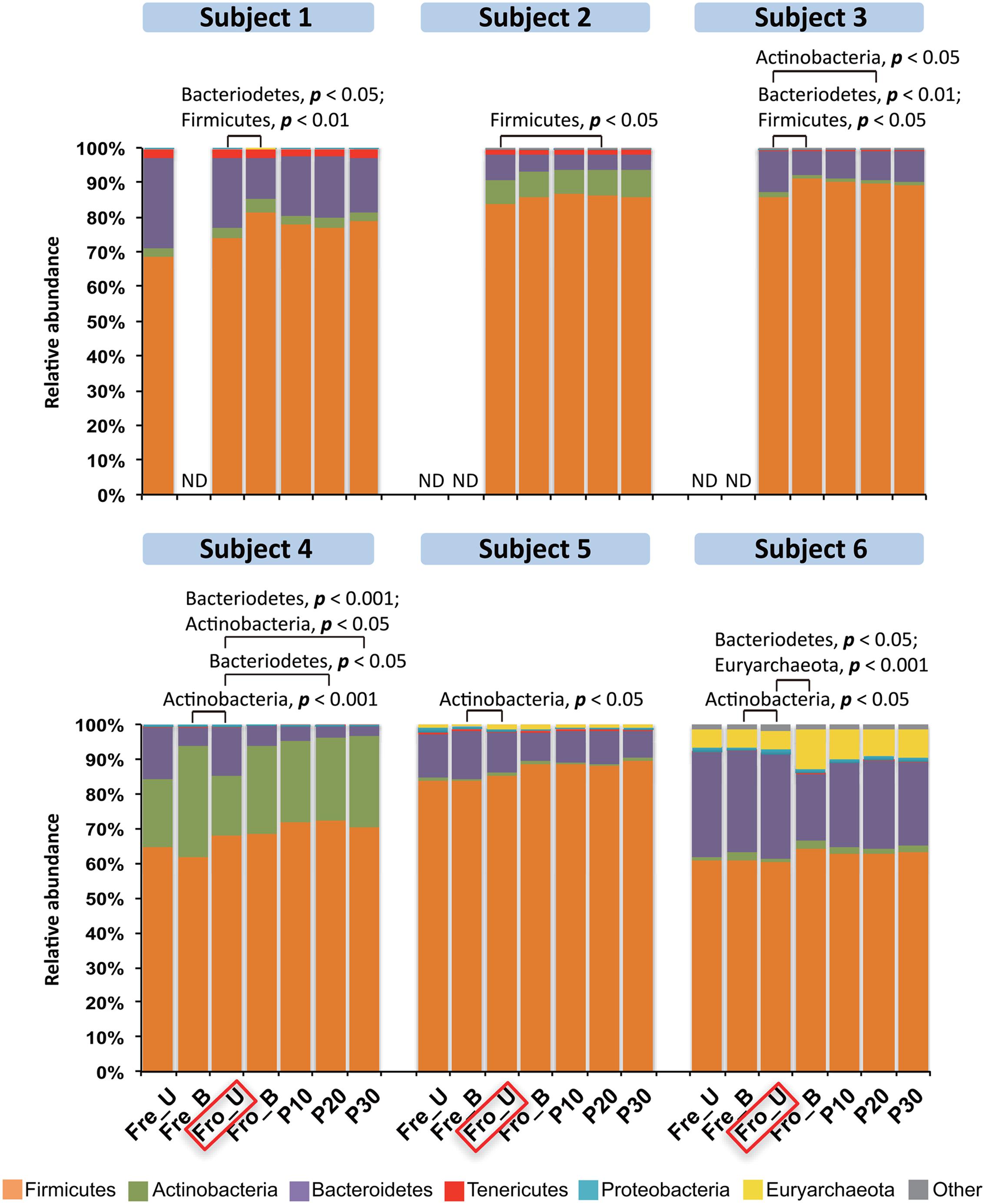
FIGURE 3. Phylum-level distributions among the fecal microbiota in six human subjects. Bars represent the mean abundance of each phylum (n = 6 replicates per person per stool sample). Phyla constituting <2% of all sequences are designated as “Other” and, overall, this category constituted less than 4% of the bacteria detected. Frozen un-homogenized samples were used as the reference for paired comparison (indicated in the red box). Significant differences were identified by Mann–Whitney U-test. ND, the microbiota was not measured with that processing method.
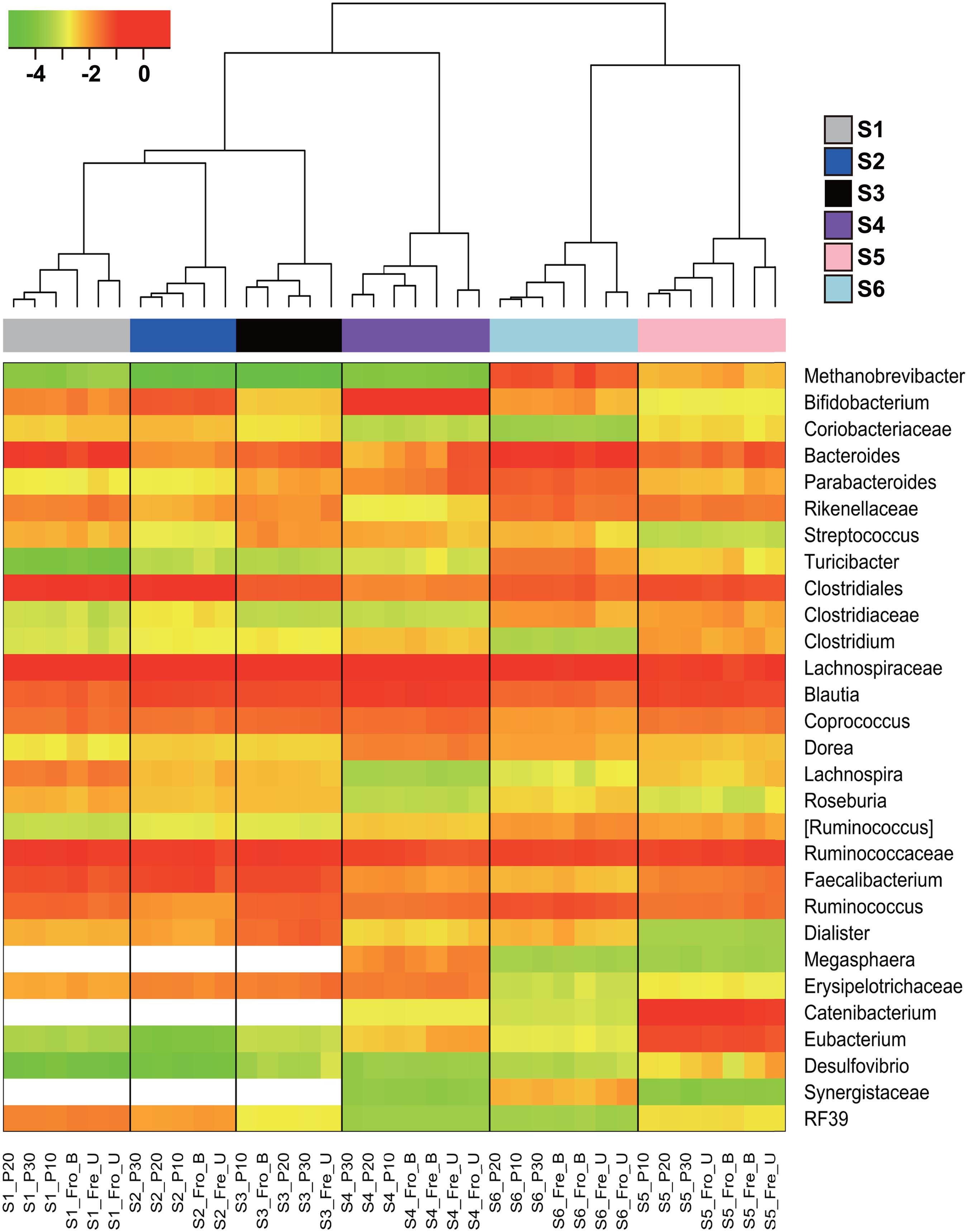
FIGURE 4. Hierarchical clustering of the dominant bacterial taxa in fecal samples. The heat map shows the log10-transformed relative abundance of 29 bacterial taxa. Taxa were excluded if they constituted less than 1% in all fecal samples. [Ruminococcus] indicates the OTUs assigned as Ruminococcus belonging to the Lachnospiraceae family. The x-axis contains the subject sample designation including the individuals (S1–S6) and processing methods. The blank cells indicate taxa that were below the detection limit.
Intra-individual Variation in Fecal Bacterial Composition is Inconsistently Altered by Processing Method
According to alpha-diversity metrics (number of observed OTUs, PD, and Shannon index), there were no significant differences in bacterial diversity between unhomogenized fresh and frozen feces for any of the six individuals (Supporting Information Table S1). Blending of either frozen or fresh stool also did not result in significant intra-individual alterations to species richness, with the exception of S5 for which there was a significant reduction in PD for the blended fresh (P = 0.037) and frozen (P = 0.025) fecal material compared to the Fro_U stool samples and reductions for fresh (P = 0.002) and frozen (P = 0.002) blended stools according to the Shannon index for S2 and S4, respectively (Supporting Information Table S1). The observed OTUs and PD were also similar between fecal samples with the application of the pneumatic mixer for different lengths of time (Supporting Information Table S1). Changes were found for three individuals, however, significance was limited to one or two of the alpha-diversity metrics, no single homogenization time was consistently affected nor was species richness repeatedly increased or decreased with mixing.
Because the fecal sample preparation methods did not result in consistent global changes in bacterial diversity, we next examined for impacts of those methods on the relative abundance of individual taxa. For each subject, bacterial proportions in stool samples that were homogenized tended to be more similar to each other than samples that were not homogenized (Figure 4). Consistent with these differences was the general increase in proportions of Fimicutes and Actinobacteria, phyla-containing Gram-positive bacteria, for frozen stools that were homogenized (Figure 3). Although the ratios of Firmicutes to Bacteroidetes increased for some stools after homogenization, the change was not consistent (Figure 3).
At deeper taxonomic levels, certain taxa were consistently and reproducibly altered within individual stools depending on freezing or homogenization. Among the 40 identified bacterial families, intra-individual proportions of Ruminococcaceae were significantly changed for all individuals, indicating that this family might be susceptible to processing bias (Supporting Information Table S2). In particular, the relative abundance of the genus Faecalibacterium, a member of the Ruminococcaceae family (Firmicutes phylum), was significantly modified by at least one processing method for all of the stools tested (Supporting Information Table S2). Pairwise comparisons of Faecalibacterium relative abundances for each subject showed that there was no single processing method that consistently resulted in changes to the proportions of this genus. However, homogenization in general tended to increase the proportions of Faecalibacterium, maximally up to 2.1-fold, compared to the unhomogenized samples for each individual (Figure 5A; Supporting Information Table S3). Importantly, these results were consistent even with the application of two different DNA extraction methods and PCR amplification conditions (data not shown).
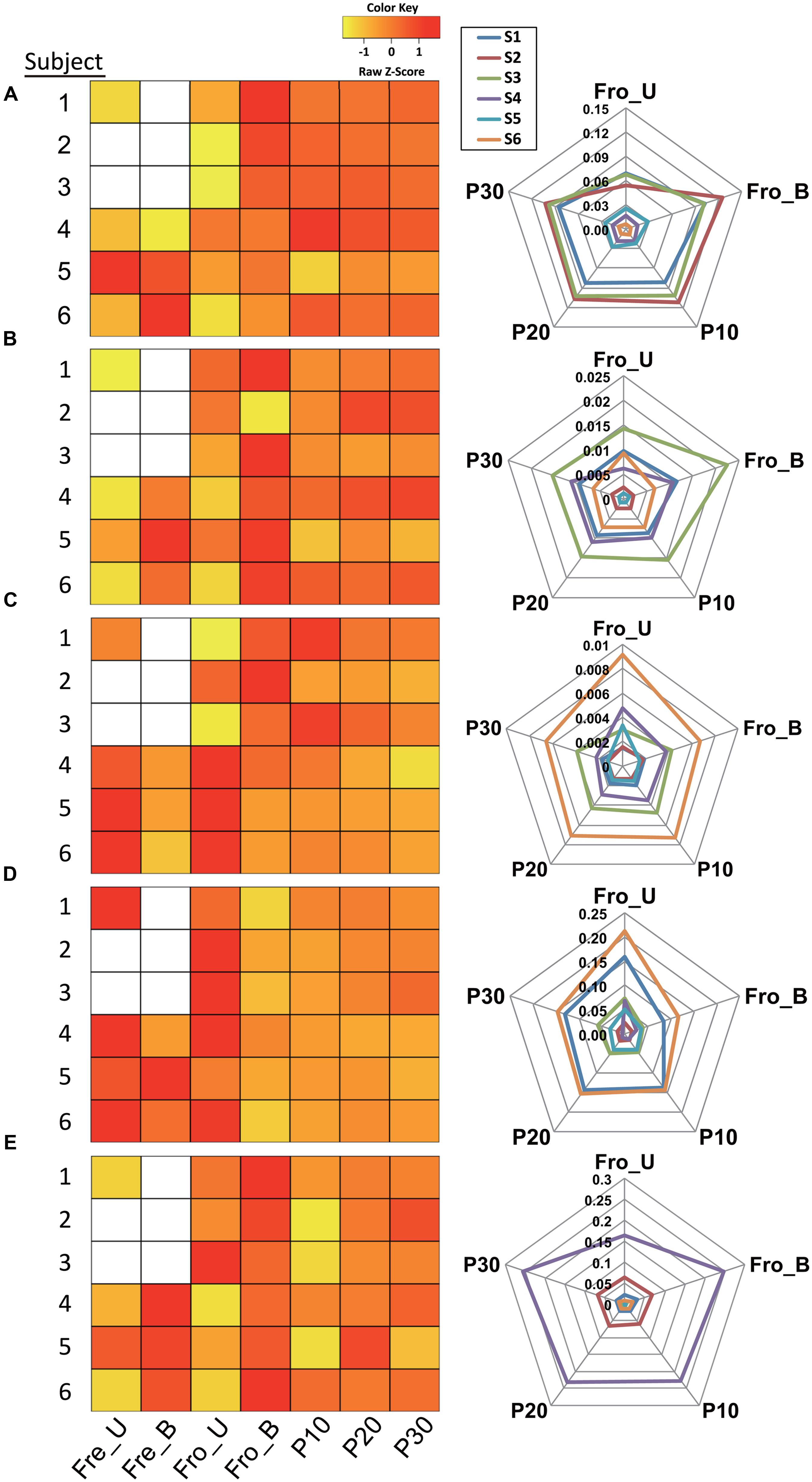
FIGURE 5. Fecal processing method effects on bacterial taxa. Proportional changes of the genera (A) Faecalibacterium, (B) Streptococcus, (C) Oscillospira, (D) Bacteroides, and (E) Bifidobacterium depending on processing method. The relative abundances in heat maps were scaled by z-score. Blank cells indicate stool samples for which data is not available. The radar charts show the relative abundances for five processing methods.
The proportions of several other genera and their corresponding families were also affected by processing method for stools from the majority (≥4) of human subjects. Within the Firmicutes phylum, Streptococcus (family Streptococcaceae), Oscillospira (family Ruminococcaceae), and SMB53 (candidate genus; family Clostridiaceae) were significantly affected by one or more processing methods at both the family and genus levels (Supporting Information Table S2). The SMB53 genus has yet to be defined and therefore is not discussed further here. For five out of six subjects, the proportions of Streptococcus increased after the stools were homogenized by blender (Figure 5B; Supporting Information Table S3). Conversely, the pneumatic mixer resulted in a lower relative abundance of Oscillospira for stools from four out of six subjects (Figure 5C; Supporting Information Table S3).
For the Bacteroidetes phylum, proportions of Bacteroides (Bacteroidaceae family) and Parabacteroides (Porphyromona daceae family) were modified by processing method (Supporting Information Table S2). Bacteroides proportions were between 0.2- and 0.78-fold lower following homogenization of frozen stool (by blending or pneumatic mixer) for the majority of subjects (Figure 5D; Supporting Information Table S3). Similar trends were found for Parabacteroides (data not shown).
Lastly, quantities of Bifidobacterium, a member of the Actinobacteria phylum, were modified. The proportions of Bifidobacterium and effects of processing method varied among subjects. However, the levels of this genus tended to increase when the stools were processed in the blender (Figure 5E; Supporting Information Table S3).
Homogenization-Reduced Intra-sample Variability
The within-group, weighted UniFrac distances of the homogenized fecal samples were significantly lower than found for stools that were not homogenized prior to DNA extraction (Figure 6). These results show that homogenization by either blending or the pneumatic mixer improves the consistency of the results between technical replicates. Moreover, freezing the stools also influenced the results because the within-group UniFrac distances of fecal samples that were frozen prior to blending (Fro_B) were significantly lower than those that were “fresh” and homogenized shortly after collection (Fre_B) (P < 0.0001 by the Mann–Whitney U-test; Figure 6).
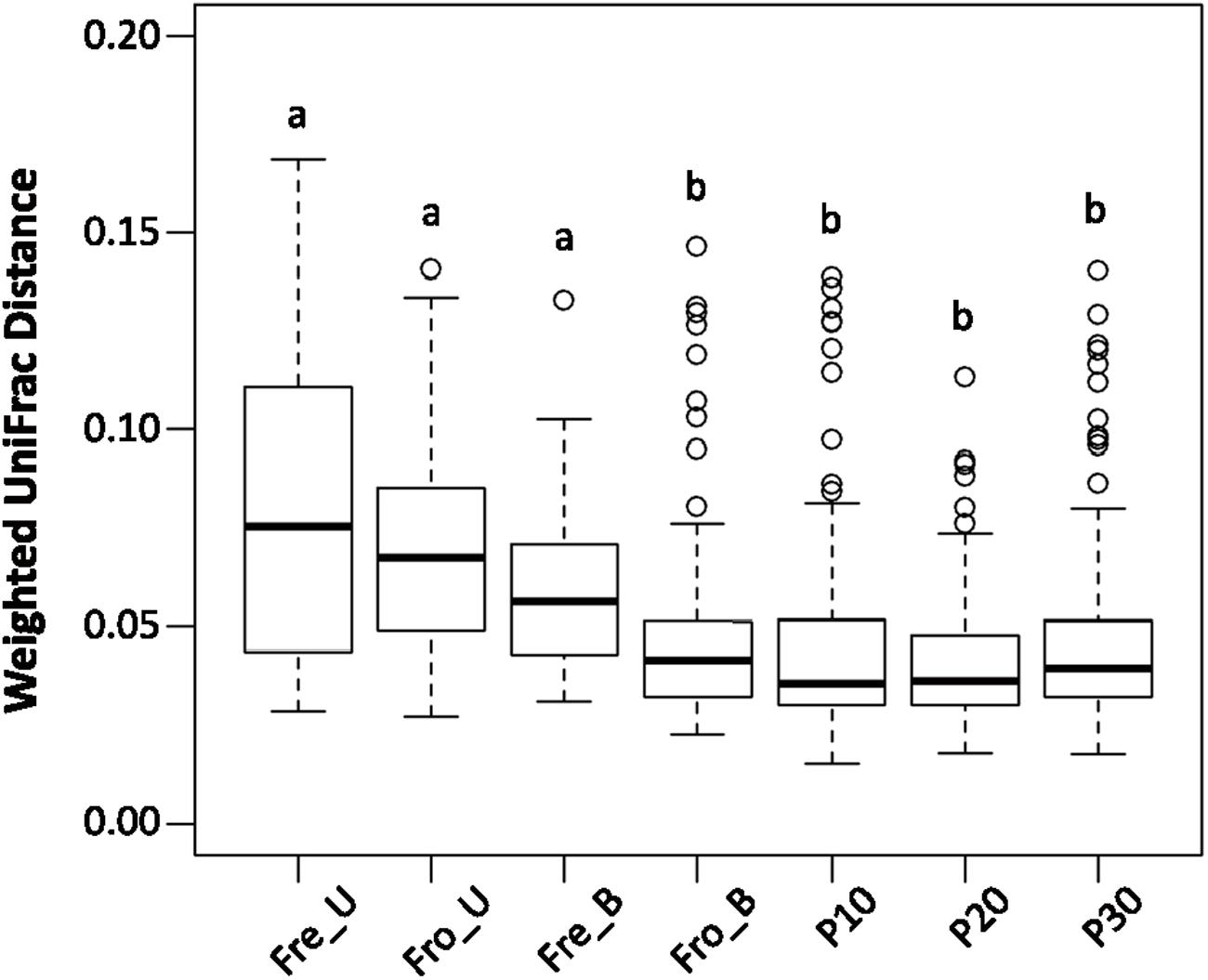
FIGURE 6. Homogenization decreased sample variation among replicates. Fecal samples were grouped according to processing method for calculation of the within group weighted UniFrac distances for each individual. Boxes that do not share a same letter are significantly different (p < 0.05) according to the Kruskal–Wallis test with the Dunn’s post hoc test.
Discussion
We found that the inter-individual variation in fecal microbiota between subjects was clearly greater than any difference introduced either by freezing or homogenization. Intra-individual comparisons showed that there was no method that reproducibly altered the alpha and beta diversity of the bacteria in the same way among stools from all subjects. Instead, our findings strongly indicate that there are certain families and genera that are more vulnerable to over/under representation in stools based on the processing method and hence might be more susceptible to potential biases caused by the manner in which fecal samples are collected, stored, and processed.
Our findings are in agreement with other studies showing that current protocols for multiplexed, 16S rRNA gene sequence assessments of fecal bacterial diversity are sufficiently robust that inter-individual variation in fecal microbiota is not overcome despite large differences in stool preparation and DNA sequencing and analysis methods (Salonen et al., 2010; Wu G.D. et al., 2010; Carroll et al., 2012; Dominianni et al., 2014; Hang et al., 2014; Santiago et al., 2014; Wesolowska-Andersen et al., 2014; Fouhy et al., 2015; Tedjo et al., 2015; Voigt et al., 2015; Wagner Mackenzie et al., 2015). Importantly, biases introduced by sample preparation and analysis methods are minor compared to the large changes in intestinal bacterial composition caused by diet, antibiotics, and disease (Willing et al., 2011; Perez-Cobas et al., 2013; David et al., 2014; Conlon and Bird, 2015; Arora and Bäckhed, 2016; Matijasic et al., 2016). The greater impact of these biologically, as opposed to technically, induced differences shows that human fecal bacteria can be monitored for species diversity and richness, even with procedural distinctions.
Consistent with this premise, the relative abundances of the dominant bacterial phyla were not consistently affected by freezing or homogenization. Although the proportions of Gram-positive bacteria and the corresponding Firmicutes to Bacteroidetes ratios tended to increase following homogenization (either by blending or pneumatic mixer), no phylum was repeatedly, significantly enriched (or depleted).
At other taxonomic levels, we found that processing methods can have modest effects on study outcomes. By testing for repeated, significant differences that could be attributed to bacterial families and specific genera within those families, we identified six genera susceptible to variation in stools from the majority (≥4) of the six human subjects tested. Most notably, Faecalibacterium was the only microbial taxon for which the relative abundance was significantly affected by one or more processing method in all individuals. Homogenization tended to increase the proportions of this genus, although no single processing method was responsible for these effects. Faecalibacterium is a butyrate-producing genus in the Firmicutes phylum that has been associated with good intestinal health (Miquel et al., 2013). Members of this genus are anti-inflammatory and secrete proteins that reduce activation of NF-κB pathways in rodent and cell culture models (Quevrain et al., 2016). These bacteria are negatively associated with inflammatory bowel diseases and other inflammatory conditions in humans (Sokol et al., 2008; Eppinga et al., 2016; Matijasic et al., 2016). Faecalibacterium was also found in lower proportions in individuals with Type 2 diabetes (T2D; Tilg and Moschen, 2014) and increased in a diet that promoted insulin sensitivity in obese subjects (Haro et al., 2016b).
Similar to Faecalibacterium, the levels of Streptococcus, another genus in the Firmicutes phylum, were increased in stools after homogenization. Streptococcus is commonly used in the production of fermented foods (Veiga et al., 2014) and is a prominent member of the human small intestine microbiota (Zoetendal et al., 2012). It was also recently reported that proportions of this genus are reduced in stools of individuals with T2D on a macrobiotic diet (Candela et al., 2016). Notably, because the homogenization methods applied here lowered the levels of Oscillospira, another genus associated with human health (Konikoff and Gophna, 2016), it should not be expected that homogenization will increase all physiologically relevant members of the Firmicutes phylum.
Other bacteria of medical and ecological significance were also consistently altered by homogenization such that they were either more (Bifidobacterium) or less (Bacteroides and Parabacteroides) abundant. Bifidobacterium is commonly applied as a probiotic and is an indigenous inhabitant of the large intestine of both infants and adults (Bottacini et al., 2014; Jost et al., 2015). Bacteroides and Parabacteroides are also prominent in the intestine and appear to be pivotal, diet-responsive members of the human microbiota (Wu et al., 2011; Del Chierico et al., 2016; Haro et al., 2016a). Taken together, these findings show how the fecal processing method could influence conclusions on the impacts of dietary interventions on bacterial genera with functions important for human health.
Presently, it remains to be shown whether these significantly changed taxa are either more or less vulnerable to homogenization or if there are other factors that influenced the outcomes. One possibility is that certain bacteria are more sensitive to lysis and DNA shearing/degradation and, therefore, were lost with the application of homogenization for sample processing. This effect would lower the detectable numbers of those sensitive taxa and, conversely, increase the proportions taxa able to withstand the sample processing method. Alternatively, homogenization could provide benefits by dislodging bacteria from macromolecules or aggregates and distributing them for increased access during DNA extraction. This change would enable more accurate and reliable detection of those taxa that would otherwise be missed by less rigorous processing approaches. The latter possibility is supported by our finding that homogenization of frozen stools, either by blender or pneumatic mixer, resulted in significant reductions in intra-sample variation. These findings are in agreement with homogenized fecal samples that were examined either by quantitative PCR (Gorzelak et al., 2015) or shot-gun metagenomics (Wesolowska-Andersen et al., 2014). Notably, the outcomes were not solely due to freezing because of the lack of change in bacterial diversity between the fresh and frozen unhomogenized samples.
In summary, fecal sample homogenization is a useful method for characterizing the human intestinal microbiome and reducing intra-sample variation. When at all possible, this method should be used instead of random sampling without homogenization because of the within-stool variation in microbial composition. Moreover, the intra-sample variability was similar for homogenization by blender versus pneumatic mixer. This result is robust evidence both are valid methods for homogenizing stool in research protocols. Because the blender method took only 2 min, in comparison to the more labor-intensive shaker-and-beads method (10–30 min), blending offers a time-saving approach to homogenization. Ultimately, improved understanding of fecal microbiota will be gained from the application of constructed bacterial community “standards” and improved knowledge on the localization of different bacterial taxa at micro- and macro-scales within luminal contents. Because of the sensitivity of certain taxa to be enriched or diminished as a result of fecal processing, comparisons across studies should be viewed with caution and instead more general outcomes provided.
Author Contributions
Y-HH performed experiments, analyzed data and wrote the manuscript; CP designed the experiments and revised the manuscript; AR performed experiments; MK, RM, and ER designed the experiments and revised the manuscript; MM designed the experiments and wrote the manuscript.
Conflict of Interest Statement
The authors declare that the research was conducted in the absence of any commercial or financial relationships that could be construed as a potential conflict of interest.
Acknowledgments
Funds for this research were provided through the NIH grant R01 #DK092575 (ER). Dr. Y-HH was supported by the Ministry of Education, Taiwan R.O.C., under the ATU plan.
Supplementary Material
The Supplementary Material for this article can be found online at: http://journal.frontiersin.org/article/10.3389/fmicb.2016.01643
Abbreviations
FDR, false discovery rate; Fre_B, fresh blender; Fre_U, fresh unhomogenized; Fro_B, frozen blender; Fro_U, frozen unhomogenized; OTUs, operational taxonomic units; P10, pneumatic mixer for 10 min; P20, pneumatic mixer for 20 min; P30, pneumatic mixer for 30 min; PCoA, principal coordinates analysis; PD, phylogenetic diversity; QIIME, quantitative insights into microbial ecology; SRA, Sequence Read Archive.
Footnotes
References
Arora, T., and Bäckhed, F. (2016). The gut microbiota and metabolic disease: current understanding and future perspectives. J. Intern. Med. 280, 339–349. doi: 10.1111/joim.12508
Bokulich, N. A., Subramanian, S., Faith, J. J., Gevers, D., Gordon, J. I., Knight, R., et al. (2013). Quality-filtering vastly improves diversity estimates from Illumina amplicon sequencing. Nat. Methods 10, 57–59. doi: 10.1038/nmeth.2276
Bottacini, F., Ventura, M., van Sinderen, D., and O’Connell Motherway, M. (2014). Diversity, ecology and intestinal function of bifidobacteria. Microb. Cell Fact. 13(Suppl. 1), S4–S18. doi: 10.1186/1475-2859-13-S1-S4
Brandariz-Fontes, C., Camacho-Sanchez, M., Vila, C., Vega-Pla, J. L., Rico, C., and Leonard, J. A. (2015). Effect of the enzyme and PCR conditions on the quality of high-throughput DNA sequencing results. Sci. Rep. 5, 8056–8060. doi: 10.1038/srep08056
Candela, M., Biagi, E., Soverini, M., Consolandi, C., Quercia, S., Severgnini, M., et al. (2016). Modulation of gut microbiota dysbioses in type 2 diabetic patients by macrobiotic Ma-Pi 2 diet. Br. J. Nutr. 116, 80–93. doi: 10.1017/S0007114516001045
Caporaso, J. G., Kuczynski, J., Stombaugh, J., Bittinger, K., Bushman, F. D., Costello, E. K., et al. (2010). QIIME allows analysis of high-throughput community sequencing data. Nat. Methods 7, 335–336. doi: 10.1038/nmeth.f.303
Caporaso, J. G., Lauber, C. L., Walters, W. A., Berg-Lyons, D., Lozupone, C. A., Turnbaugh, P. J., et al. (2011). Global patterns of 16S rRNA diversity at a depth of millions of sequences per sample. Proc. Natl. Acad. Sci. U.S.A. 108(Suppl. 1), 4516–4522. doi: 10.1073/pnas.1000080107
Cardona, S., Eck, A., Cassellas, M., Gallart, M., Alastrue, C., Dore, J., et al. (2012). Storage conditions of intestinal microbiota matter in metagenomic analysis. BMC Microbiol. 12:158. doi: 10.1186/1471-2180-12-158
Carroll, I. M., Ringel-Kulka, T., Siddle, J. P., Klaenhammer, T. R., and Ringel, Y. (2012). Characterization of the fecal microbiota using high-throughput sequencing reveals a stable microbial community during storage. PLoS ONE 7:e46953. doi: 10.1371/journal.pone.0046953
Conlon, M. A., and Bird, A. R. (2015). The impact of diet and lifestyle on gut microbiota and human health. Nutrients 7, 17–44. doi: 10.3390/nu7010017
D’Amore, R., Ijaz, U. Z., Schirmer, M., Kenny, J. G., Gregory, R., Darby, A. C., et al. (2016). A comprehensive benchmarking study of protocols and sequencing platforms for 16S rRNA community profiling. BMC Genomics 17:55. doi: 10.1186/s12864-015-2194-9
David, L. A., Maurice, C. F., Carmody, R. N., Gootenberg, D. B., Button, J. E., Wolfe, B. E., et al. (2014). Diet rapidly and reproducibly alters the human gut microbiome. Nature 505, 559–563. doi: 10.1038/nature12820
Del Chierico, F., Nobili, V., Vernocchi, P., Russo, A., De Stefanis, C., Gnani, D., et al. (2016). Gut microbiota profiling of pediatric NAFLD and obese patients unveiled by an integrated meta-omics based approach. Hepatology doi: 10.1002/hep.28572 [Epub ahead of print].
Derakhshani, H., Tun, H. M., and Khafipour, E. (2016). An extended single-index multiplexed 16S rRNA sequencing for microbial community analysis on MiSeq illumina platforms. J. Basic Microbiol. 56, 321–326. doi: 10.1002/jobm.201500420
DeSantis, T. Z., Hugenholtz, P., Larsen, N., Rojas, M., Brodie, E. L., Keller, K., et al. (2006). Greengenes, a chimera-checked 16S rRNA gene database and workbench compatible with ARB. Appl. Environ. Microbiol. 72, 5069–5072. doi: 10.1128/AEM.03006-05
Dominianni, C., Wu, J., Hayes, R. B., and Ahn, J. (2014). Comparison of methods for fecal microbiome biospecimen collection. BMC Microbiol. 14:103. doi: 10.1186/1471-2180-14-103
Eckburg, P. B., Bik, E. M., Bernstein, C. N., Purdom, E., Dethlefsen, L., Sargent, M., et al. (2005). Diversity of the human intestinal microbial flora. Science 308, 1635–1638. doi: 10.1126/science.1110591
Edgar, R. C. (2010). Search and clustering orders of magnitude faster than BLAST. Bioinformatics 26, 2460–2461. doi: 10.1093/bioinformatics/btq461
Engelbrektson, A., Kunin, V., Wrighton, K. C., Zvenigorodsky, N., Chen, F., Ochman, H., et al. (2010). Experimental factors affecting PCR-based estimates of microbial species richness and evenness. ISME J. 4, 642–647. doi: 10.1038/ismej.2009.153
Eppinga, H., Sperna Weiland, C. J., Thio, H. B., van der Woude, C. J., Nijsten, T. E., Peppelenbosch, M. P., et al. (2016). Similar depletion of protective Faecalibacterium prausnitzii in Psoriasis and inflammatory bowel disease, but not in Hidradenitis Suppurativa. J. Crohns Colitis 10, 1067–1075. doi: 10.1093/ecco-jcc/jjw070
Flint, H. J., Scott, K. P., Louis, P., and Duncan, S. H. (2012). The role of the gut microbiota in nutrition and health. Nat. Rev. Gastroenterol. Hepatol. 9, 577–589. doi: 10.1038/nrgastro.2012.156
Flores, R., Shi, J., Yu, G., Ma, B., Ravel, J., Goedert, J. J., et al. (2015). Collection media and delayed freezing effects on microbial composition of human stool. Microbiome 3, 33–43. doi: 10.1186/s40168-015-0092-7
Fouhy, F., Deane, J., Rea, M. C., O’Sullivan, O., Ross, R. P., O’Callaghan, G., et al. (2015). The effects of freezing on faecal microbiota as determined using MiSeq sequencing and culture-based investigations. PLoS ONE 10:e119355. doi: 10.1371/journal.pone.0119355
Frank, J. A., Reich, C. I., Sharma, S., Weisbaum, J. S., Wilson, B. A., and Olsen, G. J. (2008). Critical evaluation of two primers commonly used for amplification of bacterial 16S rRNA genes. Appl. Environ. Microbiol. 74, 2461–2470. doi: 10.1128/AEM.02272-07
Franzen, O., Hu, J., Bao, X., Itzkowitz, S. H., Peter, I., and Bashir, A. (2015). Improved OTU-picking using long-read 16S rRNA gene amplicon sequencing and generic hierarchical clustering. Microbiome 3, 43–56. doi: 10.1186/s40168-015-0105-6
Gonzalez, J. M., Portillo, M. C., Belda-Ferre, P., and Mira, A. (2012). Amplification by PCR artificially reduces the proportion of the rare biosphere in microbial communities. PLoS ONE 7:e29973. doi: 10.1371/journal.pone.0029973
Gorzelak, M. A., Gill, S. K., Tasnim, N., Ahmadi-Vand, Z., Jay, M., and Gibson, D. L. (2015). Methods for improving human gut microbiome data by reducing variability through sample processing and storage of stool. PLoS ONE 10:e134802. doi: 10.1371/journal.pone.0134802
Hang, J., Desai, V., Zavaljevski, N., Yang, Y., Lin, X., Satya, R. V., et al. (2014). 16S rRNA gene pyrosequencing of reference and clinical samples and investigation of the temperature stability of microbiome profiles. Microbiome 2, 31–45. doi: 10.1186/2049-2618-2-31
Haro, C., Garcia-Carpintero, S., Alcala-Diaz, J. F., Gomez-Delgado, F., Delgado-Lista, J., Perez-Martinez, P., et al. (2016a). The gut microbial community in metabolic syndrome patients is modified by diet. J. Nutr. Biochem. 27, 27–31. doi: 10.1016/j.jnutbio.2015.08.011
Haro, C., Montes-Borrego, M., Rangel-Zuniga, O. A., Alcala-Diaz, J. F., Gomez-Delgado, F., Perez-Martinez, P., et al. (2016b). Two healthy diets modulate gut microbial community improving insulin sensitivity in a human obese population. J. Clin. Endocrinol. Metab. 101, 233–242. doi: 10.1210/jc.2015-3351
Hooper, L. V., Littman, D. R., and Macpherson, A. J. (2012). Interactions between the microbiota and the immune system. Science 336, 1268–1273. doi: 10.1126/science.1223490
Jost, T., Lacroix, C., Braegger, C., and Chassard, C. (2015). Impact of human milk bacteria and oligosaccharides on neonatal gut microbiota establishment and gut health. Nutr. Rev. 73, 426–437. doi: 10.1093/nutrit/nuu016
Kabat, A. M., Srinivasan, N., and Maloy, K. J. (2014). Modulation of immune development and function by intestinal microbiota. Trends Immunol. 35, 507–517. doi: 10.1016/j.it.2014.07.010
Kennedy, N. A., Walker, A. W., Berry, S. H., Duncan, S. H., Farquarson, F. M., Louis, P., et al. (2014). The impact of different DNA extraction kits and laboratories upon the assessment of human gut microbiota composition by 16S rRNA gene sequencing. PLoS ONE 9:e88982. doi: 10.1371/journal.pone.0088982
Konikoff, T., and Gophna, U. (2016). Oscillospira: a central, enigmatic component of the human gut microbiota. Trends Microbiol. 24, 523–524. doi: 10.1016/j.tim.2016.02.015
Ley, R. E., Turnbaugh, P. J., Klein, S., and Gordon, J. I. (2006). Microbial ecology: human gut microbes associated with obesity. Nature 444, 1022–1023. doi: 10.1038/4441022a
Liu, L., Li, Y., Li, S., Hu, N., He, Y., Pong, R., et al. (2012). Comparison of next-generation sequencing systems. J. Biomed. Biotechnol. 2012:251364. doi: 10.1155/2012/251364
Lozupone, C., Lladser, M. E., Knights, D., Stombaugh, J., and Knight, R. (2011). UniFrac: an effective distance metric for microbial community comparison. ISME J. 5, 169–172. doi: 10.1038/ismej.2010.133
Majaneva, M., Hyytiainen, K., Varvio, S. L., Nagai, S., and Blomster, J. (2015). Bioinformatic amplicon read processing strategies strongly affect eukaryotic diversity and the taxonomic composition of communities. PLoS ONE 10:e130035. doi: 10.1371/journal.pone.0130035
Matijasic, M., Mestrovic, T., Peric, M., Cipcic Paljetak, H., Panek, M., Vranesic Bender, D., et al. (2016). Modulating composition and metabolic activity of the gut microbiota in IBD patients. Int. J. Mol. Sci. 17, 578–599. doi: 10.3390/ijms17040578
Miquel, S., Martin, R., Rossi, O., Bermudez-Humaran, L. G., Chatel, J. M., Sokol, H., et al. (2013). Faecalibacterium prausnitzii and human intestinal health. Curr. Opin. Microbiol. 16, 255–261. doi: 10.1016/j.mib.2013.06.003
Nechvatal, J. M., Ram, J. L., Basson, M. D., Namprachan, P., Niec, S. R., Badsha, K. Z., et al. (2008). Fecal collection, ambient preservation, and DNA extraction for PCR amplification of bacterial and human markers from human feces. J. Microbiol. Methods 72, 124–132. doi: 10.1016/j.mimet.2007.11.007
Perez-Cobas, A. E., Gosalbes, M. J., Friedrichs, A., Knecht, H., Artacho, A., Eismann, K., et al. (2013). Gut microbiota disturbance during antibiotic therapy: a multi-omic approach. Gut 62, 1591–1601. doi: 10.1136/gutjnl-2012-303184
Quevrain, E., Maubert, M. A., Michon, C., Chain, F., Marquant, R., Tailhades, J., et al. (2016). Identification of an anti-inflammatory protein from Faecalibacterium prausnitzii, a commensal bacterium deficient in Crohn’s disease. Gut 65, 415–425. doi: 10.1136/gutjnl-2014-307649
Rangel, I., Sundin, J., Fuentes, S., Repsilber, D., de Vos, W. M., and Brummer, R. J. (2015). The relationship between faecal-associated and mucosal-associated microbiota in irritable bowel syndrome patients and healthy subjects. Aliment. Pharmacol. Ther. 42, 1211–1221. doi: 10.1111/apt.13399
Salipante, S. J., Kawashima, T., Rosenthal, C., Hoogestraat, D. R., Cummings, L. A., Sengupta, D. J., et al. (2014). Performance comparison of Illumina and ion torrent next-generation sequencing platforms for 16S rRNA-based bacterial community profiling. Appl. Environ. Microbiol. 80, 7583–7591. doi: 10.1128/AEM.02206-14
Salonen, A., Nikkilä, J., Jalanka-Tuovinen, J., Immonen, O., Rajilić-Stojanović, M., Kekkonen, R. A., et al. (2010). Comparative analysis of fecal DNA extraction methods with phylogenetic microarray: effective recovery of bacterial and archaeal DNA using mechanical cell lysis. J. Microbiol. Methods 81, 127–134. doi: 10.1016/j.mimet.2010.02.007
Santiago, A., Panda, S., Mengels, G., Martinez, X., Azpiroz, F., Dore, J., et al. (2014). Processing faecal samples: a step forward for standards in microbial community analysis. BMC Microbiol. 14:112. doi: 10.1186/1471-2180-14-112
Scupham, A. J., Jones, J. A., and Wesley, I. V. (2007). Comparison of DNA extraction methods for analysis of turkey cecal microbiota. J. Appl. Microbiol. 102, 401–409. doi: 10.1111/j.1365-2672.2006.03094.x
Sergeant, M. J., Constantinidou, C., Cogan, T., Penn, C. W., and Pallen, M. J. (2012). High-throughput sequencing of 16S rRNA gene amplicons: effects of extraction procedure, primer length and annealing temperature. PLoS ONE 7:e38094. doi: 10.1371/journal.pone.0038094
Sinclair, L., Osman, O. A., Bertilsson, S., and Eiler, A. (2015). Microbial community composition and diversity via 16S rRNA gene amplicons: evaluating the illumina platform. PLoS ONE 10:e116955. doi: 10.1371/journal.pone.0116955
Sokol, H., Pigneur, B., Watterlot, L., Lakhdari, O., Bermudez-Humaran, L. G., Gratadoux, J. J., et al. (2008). Faecalibacterium prausnitzii is an anti-inflammatory commensal bacterium identified by gut microbiota analysis of Crohn disease patients. Proc. Natl. Acad. Sci. U.S.A. 105, 16731–16736. doi: 10.1073/pnas.0804812105
Stearns, J. C., Lynch, M. D. J., Senadheera, D. B., Tenenbaum, H. C., Goldberg, M. B., Cvitkovitch, D. G., et al. (2011). Bacterial biogeography of the human digestive tract. Sci. Rep. 1, 170–178. doi: 10.1038/srep00170
Tedjo, D. I., Jonkers, D. M., Savelkoul, P. H., Masclee, A. A., van Best, N., Pierik, M. J., et al. (2015). The effect of sampling and storage on the fecal microbiota composition in healthy and diseased subjects. PLoS ONE 10:e126685. doi: 10.1371/journal.pone.0126685
Tilg, H., and Moschen, A. R. (2014). Microbiota and diabetes: an evolving relationship. Gut 63, 1513–1521. doi: 10.1136/gutjnl-2014-306928
Tremaroli, V., and Backhed, F. (2012). Functional interactions between the gut microbiota and host metabolism. Nature 489, 242–249. doi: 10.1038/nature11552
Tremblay, J., Singh, K., Fern, A., Kirton, E. S., He, S., Woyke, T., et al. (2015). Primer and platform effects on 16S rRNA tag sequencing. Front. Microbiol. 6:771. doi: 10.3389/fmicb.2015.00771
Veiga, P., Pons, N., Agrawal, A., Oozeer, R., Guyonnet, D., Brazeilles, R., et al. (2014). Changes of the human gut microbiome induced by a fermented milk product. Sci. Rep. 4, 6328–6336. doi: 10.1038/srep06328
Vishnivetskaya, T. A., Layton, A. C., Lau, M. C., Chauhan, A., Cheng, K. R., Meyers, A. J., et al. (2014). Commercial DNA extraction kits impact observed microbial community composition in permafrost samples. FEMS Microbiol. Ecol. 87, 217–230. doi: 10.1111/1574-6941.12219
Voigt, A. Y., Costea, P. I., Kultima, J. R., Li, S. S., Zeller, G., Sunagawa, S., et al. (2015). Temporal and technical variability of human gut metagenomes. Genome Biol. 16, 73–84. doi: 10.1186/s13059-015-0639-8
Wagner Mackenzie, B., Waite, D. W., and Taylor, M. W. (2015). Evaluating variation in human gut microbiota profiles due to DNA extraction method and inter-subject differences. Front. Microbiol. 6:130. doi: 10.3389/fmicb.2015.00130
Wesolowska-Andersen, A., Bahl, M. I., Carvalho, V., Kristiansen, K., Sicheritz-Ponten, T., Gupta, R., et al. (2014). Choice of bacterial DNA extraction method from fecal material influences community structure as evaluated by metagenomic analysis. Microbiome 2, 19–29. doi: 10.1186/2049-2618-2-19
Willing, B. P., Russell, S. L., and Finlay, B. B. (2011). Shifting the balance: antibiotic effects on host-microbiota mutualism. Nat. Rev. Microbiol. 9, 233–243. doi: 10.1038/nrmicro2536
Wu, G. D., Chen, J., Hoffmann, C., Bittinger, K., Chen, Y. Y., Keilbaugh, S. A., et al. (2011). Linking long-term dietary patterns with gut microbial enterotypes. Science 334, 105–108. doi: 10.1126/science.1208344
Wu, G. D., Lewis, J. D., Hoffmann, C., Chen, Y. Y., Knight, R., Bittinger, K., et al. (2010). Sampling and pyrosequencing methods for characterizing bacterial communities in the human gut using 16S sequence tags. BMC Microbiol. 10:206. doi: 10.1186/1471-2180-10-206
Wu, J. Y., Jiang, X. T., Jiang, Y. X., Lu, S. Y., Zou, F., and Zhou, H. W. (2010). Effects of polymerase, template dilution and cycle number on PCR based 16 S rRNA diversity analysis using the deep sequencing method. BMC Microbiol. 10:255. doi: 10.1186/1471-2180-10-255
Yin, X., Yan, Y., Kim, E. B., Lee, B., and Marco, M. L. (2014). Short communication: effect of milk and milk containing Lactobacillus casei on the intestinal microbiota of mice. J. Dairy Sci. 97, 2049–2055. doi: 10.3168/jds.2013-7477
Yuan, S., Cohen, D. B., Ravel, J., Abdo, Z., and Forney, L. J. (2012). Evaluation of methods for the extraction and purification of DNA from the human microbiome. PLoS ONE 7:e33865. doi: 10.1371/journal.pone.0033865
Zheng, W., Tsompana, M., Ruscitto, A., Sharma, A., Genco, R., Sun, Y., et al. (2015). An accurate and efficient experimental approach for characterization of the complex oral microbiota. Microbiome 3, 48–58. doi: 10.1186/s40168-015-0110-9
Keywords: 16S rRNA, Faecalibacterium, feces/specimen processing method, human intestinal microbiota, fiber, obesity, diet
Citation: Hsieh Y-H, Peterson C M, Raggio A, Keenan M J, Martin R J, Ravussin E and Marco M L (2016) Impact of Different Fecal Processing Methods on Assessments of Bacterial Diversity in the Human Intestine. Front. Microbiol. 7:1643. doi: 10.3389/fmicb.2016.01643
Received: 22 July 2016; Accepted: 03 October 2016;
Published: 20 October 2016.
Edited by:
Mike Taylor, University of Auckland, New ZealandReviewed by:
David William Waite, University of Queensland, AustraliaDanielle M. R. L. Middleton, Landcare Research, New Zealand
Copyright © 2016 Hsieh, Peterson, Raggio, Keenan, Martin, Ravussin and Marco. This is an open-access article distributed under the terms of the Creative Commons Attribution License (CC BY). The use, distribution or reproduction in other forums is permitted, provided the original author(s) or licensor are credited and that the original publication in this journal is cited, in accordance with accepted academic practice. No use, distribution or reproduction is permitted which does not comply with these terms.
*Correspondence: Maria L. Marco, bW1hcmNvQHVjZGF2aXMuZWR1