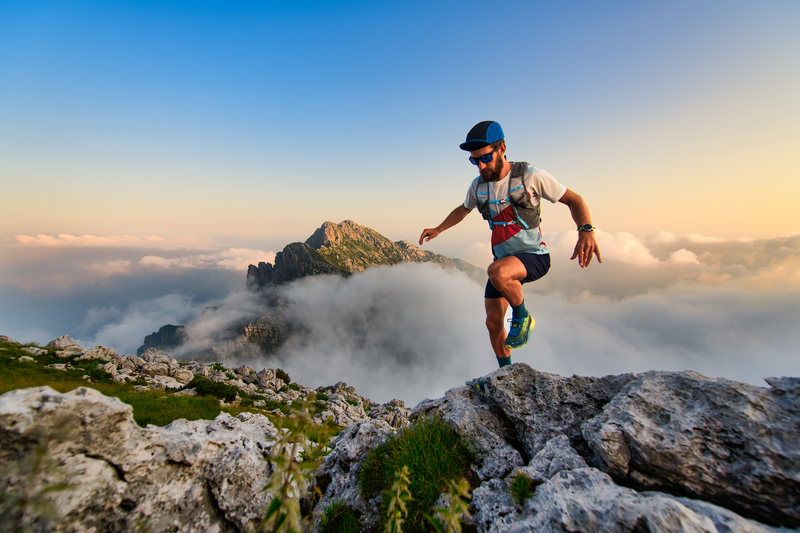
95% of researchers rate our articles as excellent or good
Learn more about the work of our research integrity team to safeguard the quality of each article we publish.
Find out more
ORIGINAL RESEARCH article
Front. Microbiol. , 03 August 2016
Sec. Evolutionary and Genomic Microbiology
Volume 7 - 2016 | https://doi.org/10.3389/fmicb.2016.01180
Codon usage bias, as a combined interplay from mutation and selection, has been intensively studied in Escherichia coli. However, codon usage analysis in an E. coli pangenome remains unexplored and the relative importance of mutation and selection acting on core genes and strain-specific genes is unknown. Here we perform comprehensive codon usage analyses based on a collection of multiple complete genome sequences of E. coli. Our results show that core genes that are present in all strains have higher codon usage bias than strain-specific genes that are unique to single strains. We further explore the forces in influencing codon usage and investigate the difference of the major force between core and strain-specific genes. Our results demonstrate that although mutation may exert genome-wide influences on codon usage acting similarly in different gene sets, selection dominates as an important force to shape biased codon usage as genes are present in an increased number of strains. Together, our results provide important insights for better understanding genome plasticity and complexity as well as evolutionary mechanisms behind codon usage bias.
As an important organism in biotechnology and microbiology, the completion of whole genome sequencing of Escherichia coli accomplished in 1997 (Blattner et al., 1997) has laid a significant foundation for fully studying its genome (Zimmer, 2009; Lukjancenko et al., 2010). Since then, many studies performed analyses on E. coli at different aspects for characterizing its genome diversity (Rasko et al., 2008; Touchon et al., 2009; Lukjancenko et al., 2010), horizontal gene transfer (Jain et al., 1999; Ochman et al., 2000; Gogarten and Townsend, 2005), pathogenicity (Kaper et al., 2004; Croxen and Finlay, 2010), and evolutionary process (Clermont et al., 2000; Elena and Lenski, 2003; Lewis et al., 2010). Among them, codon usage studies have been extensively conducted in E. coli, demonstrating heterogeneity in synonymous codon usage and revealing that codon usage bias principally arises from a complex interplay between mutation and selection (Bulmer, 1991; Sharp et al., 1993; dos Reis et al., 2003; Hershberg and Petrov, 2008; Plotkin and Kudla, 2011).
Initially, studies on E. coli have identified selection as a major force since codon usage in highly expressed genes is positively correlated with tRNA abundance (Ikemura, 1981, 1985; Gouy and Gautier, 1982). Subsequently, evidence has further accumulated that mutation is also an important driving force shaping heterogeneous codon usage in a variety of bacteria, including E. coli (Sueoka, 1988; Knight et al., 2001; Chen et al., 2004). Meanwhile, it has been argued that mutation alone cannot lead to nonrandom nucleotide composition in many bacteria species (Hershberg and Petrov, 2010; Hildebrand et al., 2010) and selection may play an important role in driving GC content variation (Stoletzki and Eyre-Walker, 2007; Sharp et al., 2010; Raghavan et al., 2012). Recent studies have shown that another confounding factor, namely, GC-biased gene conversion, which is believed to be independent from selection, may provoke the nonrandomness of base composition and the heterogeneity of synonymous codon usage in E. coli as well as other bacteria (Touchon et al., 2009; Lassalle et al., 2015; Reichenberger et al., 2015). Although codon usage has been extensively studied in E. coli, it can be seen that the relative importance of mutation and selection operating on codon usage has been still controversial (Knight et al., 2001; Stoletzki and Eyre-Walker, 2007; Ran et al., 2014) and previous studies performed codon usage analysis primarily on individual genomes (dos Reis et al., 2003).
The availability of complete genome sequences of multiple different strains for a given species, collectively constituting this species pangenome, offers a new strategy to fully capture bacterial genome plasticity and complexity and to unveil the underlying evolutionary mechanisms associated with a wide diversity of environments (Medini et al., 2005; Vernikos et al., 2015). As a pangenome is composed of core genes that are present in all strains, dispensable genes that are present in two or more strains, and strain-specific genes that are unique to single strains, genome sequences of multiple E. coli strains enable in-depth analyses on codon usage in a pangenome context. Recent studies conducted pangenome analysis based on multiple E. coli strains, primarily focusing on identification of core genome, and dispensable genome (Lukjancenko et al., 2010), comparison of commensal and pathogenic isolates (Rasko et al., 2008), and investigation of gene variation and phylogeny inference (Kaas et al., 2012). However, codon usage analysis in an E. coli pangenome remains unexplored and importantly, very little is known about the relative importance of mutation and selection acting on core genes and strain-specific genes. Toward this end, here we perform comprehensive codon usage analyses based on a collection of multiple complete genome sequences of E. coli, explore the major force in shaping biased codon usage in the E. coli pangenome, and investigate whether mutation and selection act differentially on synonymous codon usage between core genes and strain-specific genes.
We retrieved 61 complete genome sequences of E. coli from the National Center for Biotechnology Information (NCBI) (ftp://ftp.ncbi.nlm.nih.gov/genomes/all/), and summarized their details in Table S1. To reduce redundancy of these retrieved genomes, we selected the strains that are evolutionarily divergent based on the genomic blast dendrogram. As a result, a collection of 26 genome sequences was used for pangenome analysis (Figure S1). For each strain, horizontally transferred genes were identified by Islandviewer (Dhillon et al., 2015) and detailed information of horizontally transferred genes identified for all strains were summarized into Table S2. We obtained RNA-Seq data for 4 E. coli strains from SRA (http://www.ncbi.nlm.nih.gov/sra/; accession numbers: SRR1184439, SRR1183094, SRR1185100, and SRR915686). Reads were filtered using FASTX-Toolkit (http://hannonlab.cshl.edu/fastx_toolkit/index.html) and mapped to the reference genomes with Bowtie2 (Langmead and Salzberg, 2012). We downloaded tRNA copy number data for E. coli from GtRNAdb (Chan and Lowe, 2016).
Based on a total of 95,439 genes from 26 strains, we used OrthoMCL (Fischer et al., 2011) and PanGP (Zhao et al., 2014) for pangenome analyses. As a result, we clustered all genes into 6797 clusters and further grouped the E. coli pangenome into five gene sets: strain-specific genes (that are present in only one strain; n = 1812 in 1723 clusters), lowly-shared genes (that are shared between 2 and 9 strains; n = 6728 in 1467 clusters), moderately-shared genes (that are shared found between 10 and 17 strains; n = 5776 in 398 clusters), highly-shared genes (that are shared between 18 and 25 strains; n = 24,697 in 1041 clusters), and core genes (that are present in all 26 strains; n = 59,426 in 2168 clusters, Table S3). However, considering that genomes may have paralogs (e.g., 59,426 vs. 26 × 2168 = 56,368 in core genes), therefore, for each cluster, we defined representative genes as genes after removal of paralogs. Analyzed results thereinafter were based on all genes, while those based on representative genes that lead to consistent conclusions were presented as Supplementary Materials.
To avoid artifacts caused by methodology, we adopted multiple different measures for estimating codon usage bias, including CDC (Codon Deviation Coefficient; Zhang et al., 2012), CAI (Codon Adaptation Index; Sharp and Li, 1987), Nc (Effective Number of Codons; Wright, 1990), and Nc′ (a variant of Nc) (Novembre, 2002). It is noted that CAI and CDC produce values varying from 0 (no bias) to 1 (maximum bias), whereas Nc and Nc′ range from 20 (maximum bias) to 61 (no bias). To investigate the variation trend of codon usage bias across different gene sets and examine whether different measures present consistent trends, therefore, we rescaled Nc and Nc′ to make them range from 0 (no bias) to 1 (maximum bias) by using the formula (61–X)/41, where X = Nc or Nc′. To avoid stochastic errors, genes that are shorter than 100 codons were excluded from this analysis, as codon usage estimation might be biased in shorter genes (Kessler and Dean, 2014). In neutrality-plot, GC12 is the mean of GC contents averaged over the first two codon positions (viz., GC1 and GC2, respectively). The cosine similarity metric was used to estimate the degree of similarity between tRNA abundance and relative synonymous codon usage (RSCU) and formulated as below,
where n is the total number of the codons and for a given codon k, Xk is tRNA copy number and Yk is the RSCU value. The cosine similarity metric ranges from 0 (completely different) to 1 (identical).
As a useful statistical method to analyze the deviation of the RSCU value, COA provides a major trend of factors related to codon usage in different gene sets. In COA, genes were plotted into a 59-dimensional hyperspace according to the usage of the 59 informative codons, excluding AUG, UAA, UAG, UGA, and UGG. Generally, if the variability of one axis is >10%, this axis indicates a major variation trend (Greenacre, 1984).
It is an effective way to explore heterogeneity in codon usage by plotting Nc values against GC3 (Wright, 1990). In ENC-plot, estimated Nc was obtained by ENCprime (Novembre, 2002) and expected Nc was calculated using the formula 2 + X + 29/[X2 + (1−X)2], where X = GC3. In general, if a gene is under strong mutation rather than selection, estimated Nc will be close to expected Nc, with no or slight deviation. Otherwise, a large deviation between estimated Nc and expected Nc indicates strong selection in influencing this gene's codon usage.
All statistical tests were carried out using the statistical analysis software SPSS. The differences in CDC, CAI, Nc, Nc′, nucleotide compositions, and similarity between tRNA abundance and RSCU were analyzed by one-way ANOVA. Spearman's rank correlation analysis was used in COA and expression level correlation.
We build the E. coli pangenome based on a collection of complete genome sequences from 26 closely divergent isolates (Table S1). Considering the possibility of acquisition of genes by horizontal transfer, which consequently may lead to higher heterogeneity in codon usage as well as nucleotide composition (Koonin et al., 2001), we perform pangenome analysis for all E. coli genes by removal of horizontally transferred genes (Table S2) and identify genes that are present in 1–26 isolates, respectively (Figure 1; see Section Materials and Methods). As a result, we obtain 2168 core gene clusters (that are present in all 26 isolates) and 1723 strain-specific gene clusters (that are present in only one isolate) (Figure 1A). Noticeably, core genes and strain-specific genes are relatively abundant, presumably indicating that E. coli is not only conservative in core functions but also is active in gene birth for adaptation to new environments (Davids and Zhang, 2008). When more isolates are included, the E. coli pangenome becomes larger and the size of core genes decreases dramatically to be smooth at larger number of isolates (Figure 1B), indicating that E. coli is an open genome (Tettelin et al., 2008; Lukjancenko et al., 2010).
Figure 1. E. coli pangenome and core genes based on 26 isolates. (A) Number of gene clusters shared in 1–26 isolates, respectively. According to their presence in different number of isolates, genes were further grouped into five gene sets: strain-specific genes, lowly-shared genes, moderately-shared genes, highly-shared genes, and core genes. (B) Pangenome size and core-genome size when the number of isolates varies from 1 to 26.
As GC content is highly related to synonymous codon usage, we first investigate whether a gene's GC content is dependent on its presence in different number of isolates (Figure 2A and Figure S2A). We find that GC content is higher in core genes and lower in strain-specific genes, exhibiting a positive correlation with gene presence. As GC contents at three different codon positions (denoted as GC1, GC2, GC3, respectively) correlate closely yet differentially with the overall GC content (Hu et al., 2007), we further investigate the trend of positional GC contents across different gene sets. Intriguingly, GC1 and GC3 correlate positively with gene presence in the pangenome, presenting comparable trends as GC content does. On the contrary, GC2 is relatively constant across all examined gene sets, probably due to stronger selection at this position since any substitution in the second codon position leads to the amino acid replacement and protein structure variation (Gu et al., 2004). As previous studies have shown that gene length is positively correlated with GC content (Oliver and Marin, 1996; Li and Du, 2014), we further examine the variation of gene length in all five gene sets. Consistently, core genes tend to be longer than strain-specific genes (Figure 2B and Figure S2B). Taken together, with an increased presence in more isolates, genes tend to have higher GC contents and longer sequences.
Figure 2. Distribution of GC contents (A) and gene length (B) across five gene sets in the E. coli pangenome. GC contents at three codon positions are denoted as GC1, GC2, GC3, respectively.
As E. coli genes have different evolutionary histories and accordingly may have experienced differential forces from mutation and selection shaping synonymous codon usage, here we estimate CUBs for E. coli genes in the context of pangenome. Clearly, core genes possess more biased codon usage than strain-specific genes (P < 0.05; Figures 3A–D and Figure S3). Specifically, core genes have highest CUBs, followed by highly-shared, moderately-shared, and lowly-shared genes, whereas strain-specific genes present lowest CUBs. This result is consistently observed by different CUB measures (Figures 3A–D and Figure S3), although they adopt different strategies for CUB estimation. To further examine what genes possess higher CUBs in different gene sets, we sort genes in term of CUB and find that the top 10 in core genes are most ribosomal proteins (that are believed to be highly expressed), whereas the top 10 in strain-specific genes are almost hypothetical proteins (Table S4).
Figure 3. Codon usage bias in the E. coli pangenome estimated by four different measures, viz., (A) CDC, (B) CAI, (C) Nc, and (D) Nc′. Correlation between CAI and gene expression level was examined in (E) strain-specific genes, (F) lowly-shared genes, (G) moderately-shared genes, (H) highly-shared genes, and (I) core genes, respectively.
As biased codon usage is thought to arise from selection for translational efficiency and/or accuracy, it is believed that a positive correlation between CUB and gene expression level is indicative of translational selection (Ikemura, 1981; Plotkin and Kudla, 2011; Ma et al., 2014). To decipher whether translational selection is also associated with gene presence in the context of a pangenome, we collect RNA-Seq data (Figure S4) for E. coli and examine the correlation between CUB and gene expression level in five different gene sets where genes are shared in different numbers of isolates (Table S5). As a result, we find that the correlation between CUB and gene expression level is positively stronger in core genes by comparison with strain-specific genes, indicating that translational selection acts stronger in core genes (Figures 3E–I and Figure S5).
Stronger translational selection indicates that synonymous codon usage is more biased toward tRNA abundance. To further validate the result derived from gene expression level, we examine the similarity between tRNA abundance and RSCU among different gene sets (Figure 4 and Figure S6). A higher similarity suggests close correspondence between codon usage and tRNA abundance. Consistently, we observe that the similarity between tRNA abundance and RSCU is positively correlated with gene presence and core genes take the highest similarity, indicating that codon usage in core genes is more biased toward tRNA abundance, viz., core genes are under the strongest translational selection among five gene sets. Taken together, in contrast to other genes, core genes tend to have higher CUBs and experience stronger translational selection.
Figure 4. Similarity between tRNA abundance and relative synonymous codon usage. The cosine similarity metric was used, indicating the degree of similarity between tRNA abundance and relative synonymous codon usage and ranging from 0 (completely different) to 1 (identical).
To dissect factors influencing codon usage in different E. coli genes, we first perform correspondence analysis on RSCU across all five different gene sets (Figure S7). Considering that the first principal axis can explain the majority of codon usage (>10%), we then analyze the correlation between the first axis and 65 factors for each gene set (Table S6). We find that there is a significant correlation between GC3 and the first axis in strain-specific genes (R = 0.92, P < 1e-300), but its absolute value drops gradually as genes are present in more isolates (0.92 in lowly-shared genes, 0.91 in moderately-shared genes, and 0.61 in highly-shared genes) and is significantly lower in core genes (R = 0.28, P < 1e-300). On the other hand, CAI presents opposite trends that core genes have the highest significant correlation (R = 0.94, P < 1e-300) and strain-specific genes have the lowest correlation (R = 0.63, P = 3.3e-201). Collectively, these results demonstrate that selection in connection with expression level indicated by CAI dominates core genes, whereas mutation reflected by GC3 dominates strain-specific genes. However, it should be noted that mutation is a genome-wide force in shaping synonymous codon usage (Chen et al., 2004) and accordingly may act similarly in each gene set. In spite of this, our results clearly show that core genes are under stronger selection than strain-specific genes, indicating that factors influencing codon usage variation are heterogeneous in different gene sets.
As ENC-plot is widely used to investigate the influence of mutation and selection acting on codon usage (Wright, 1990), we plot Nc against GC3 (Figures 5A–E and Figures S8A–E) to identify the main factor in shaping heterogeneous codon usage in the pangenome context. Agreeing with results presented above, most strain-specific genes are around the expected ENC curve, indicating that these genes are driven primarily by mutation (Figure 5A), whereas core genes are deviated from the expected curve, suggesting that selection is a major force operating on core genes (Figure 5E). Quantitatively, we estimate the percentage of genes that are deviated from the expected curve and clearly find the core genes present higher deviations than strain-specific genes (Figures 5A–E and Table S7). These results suggest that although mutation exerts genome-wide influences on codon usage (Chen et al., 2004), selection dominates as an important factor to influence codon usage in core genes.
Figure 5. ENC-plot (A–E) and neutrality-plot (F–J) across different gene sets. The expected and estimated values in ENC-plot are indicated by solid and hollow circles, respectively. For any given gene, if its deviation value, calculated by (expected–estimated)/expected, is greater than a threshold (default = 0.15), this gene is assumed to deviate from the expected ENC curve. Similar results can be found in Table S7 when considering different thresholds.
To further validate the result derived from ENC-plot, we also perform neutrality-plot that is based on nucleotide contents to quantify the relative ratio between mutation and selection (Sueoka, 1988). Based on the E. coli pangenome, we hypothesize that nucleotide content at the third codon position is different from that at the first two codon positions, which is expected to be more pronounced in core genes than strain-specific genes. To test this hypothesis, we conduct neutrality-plot in different gene sets (Figures 5F–J and Figures S8F–J). We find that the correlation between GC3 and GC12 (mean value of GC1 and GC2) is significantly positive in strain-specific genes (R = 0.63, P = 3.6e-204; Figure 5F), but drops gradually in highly-shared, moderately-shared, and lowly-shared gene sets (Figures 5G–I), and becomes very weak or nearly absent in core genes (R = 0.06, P = 3.1e-42; Figure 5J). These results show that strain-specific genes have smaller differences in nucleotide composition between GC3 and GC12, whereas core genes have the larger difference. In addition, the slope of GC3–GC12 regression function decreases from 0.32 in strain-specific genes to 0.03 in core genes. It should be noted that the slope equals to 0 represents no effect of directional mutation pressure (complete selective constraints) and 1 stands for the complete neutrality (Sueoka, 1988).
Taken collectively, results derived from ENC-plot and neutrality-plot provide evidences that core genes are under stronger selection than strain-specific genes. Agreeing with previous studies that translational selection is found in E. coli (dos Reis et al., 2003), our results provide further detailed evidence from the pangenome level that stronger translational selection in E. coli is contributed considerably by core genes. Considering that core genes are majorly comprised by housekeeping genes (Bentley, 2009) and encode basic functions and phenotypical traits related to the basic biology of the species (Medini et al., 2005; Monk et al., 2013), stronger selection provides high translational accuracy to minimize the missense and nonsense errors (Stoletzki and Eyre-Walker, 2007; Hershberg and Petrov, 2008) and accelerates the translation elongation in protein expression (Ran et al., 2014), which is advantageous for genome stability in species evolution. As for strain-specific genes, mutation and weak selection combined contribute to formation of new genes, which increases the genome plasticity and species diversity, provides supplementary biochemical pathways (Medini et al., 2005) and acquires selective advantages (Mongodin et al., 2013) for certain strains that live in different circumstances. Therefore, our results provide important insights for better understanding genome plasticity and complexity as well as evolutionary mechanisms behind codon usage bias.
SS analyzed the data and drafted the manuscript. JX and ZZ designed the research. HZ and ZZ revised the manuscript. All authors have approved the final version of the article. All authors agree to be accountable for all aspects of the work in ensuring that questions related to the accuracy or integrity of any part of the work are appropriately investigated and resolved.
This work was supported by grants from National Programs for High Technology Research and Development (863 Program; 2014AA021503 and 2015AA020108) and the “100-Talent Program” of Chinese Academy of Sciences.
The authors declare that the research was conducted in the absence of any commercial or financial relationships that could be construed as a potential conflict of interest.
The Supplementary Material for this article can be found online at: http://journal.frontiersin.org/article/10.3389/fmicb.2016.01180
Bentley, S. (2009). Sequencing the species pan-genome. Nat. Rev. Microbiol. 7, 258–259. doi: 10.1038/nrmicro2123
Blattner, F. R., Plunkett, G., Bloch, C. A., Perna, N. T., Burland, V., Riley, M., et al. (1997). The complete genome sequence of Escherichia coli K-12. Science 277, 1453–1462.
Bulmer, M. (1991). The selection-mutation-drift theory of synonymous codon usage. Genetics 129, 897–907.
Chan, P. P., and Lowe, T. M. (2016). GtRNAdb 2.0: an expanded database of transfer RNA genes identified in complete and draft genomes. Nucleic Acids Res. 44, D184–D189. doi: 10.1093/nar/gkv1309
Chen, S. L., Lee, W., Hottes, A. K., Shapiro, L., and McAdams, H. H. (2004). Codon usage between genomes is constrained by genome-wide mutational processes. Proc. Natl. Acad. Sci. U.S.A. 101, 3480–3485. doi: 10.1073/pnas.0307827100
Clermont, O., Bonacorsi, S., and Bingen, E. (2000). Rapid and simple determination of the Escherichia coli phylogenetic group. Appl. Environ. Microbiol. 66, 4555–4558. doi: 10.1128/AEM.66.10.4555-4558.2000
Croxen, M. A., and Finlay, B. B. (2010). Molecular mechanisms of Escherichia coli pathogenicity. Nat. Rev. Microbiol. 8, 26–38. doi: 10.1038/nrmicro2265
Davids, W., and Zhang, Z. (2008). The impact of horizontal gene transfer in shaping operons and protein interaction networks–direct evidence of preferential attachment. BMC Evol. Biol. 8:23. doi: 10.1186/1471-2148-8-23
Dhillon, B. K., Laird, M. R., Shay, J. A., Winsor, G. L., Lo, R., Nizam, F., et al. (2015). IslandViewer 3: more flexible, interactive genomic island discovery, visualization and analysis. Nucleic Acids Res. 43, W104–W108. doi: 10.1093/nar/gkv401
dos Reis, M., Wernisch, L., and Savva, R. (2003). Unexpected correlations between gene expression and codon usage bias from microarray data for the whole Escherichia coli K-12 genome. Nucleic Acids Res. 31, 6976–6985. doi: 10.1093/nar/gkg897
Elena, S. F., and Lenski, R. E. (2003). Evolution experiments with microorganisms: the dynamics and genetic bases of adaptation. Nat. Rev. Genet. 4, 457–469. doi: 10.1038/nrg1088
Fischer, S., Brunk, B. P., Chen, F., Gao, X., Harb, O. S., Iodice, J. B., et al. (2011). Using OrthoMCL to assign proteins to OrthoMCL-DB groups or to cluster proteomes into new ortholog groups. Curr. Protoc. Bioinformatics 12, 11–19. doi: 10.1002/0471250953.bi0612s35
Gogarten, J. P., and Townsend, J. P. (2005). Horizontal gene transfer, genome innovation and evolution. Nat. Rev. Microbiol. 3, 679–687. doi: 10.1038/nrmicro1204
Gouy, M., and Gautier, C. (1982). Codon usage in bacteria: correlation with gene expressivity. Nucleic Acids Res. 10, 7055–7074.
Greenacre, M. J. (1984). Theory and Applications of Correspondence Analysis. London: Academic Press.
Gu, W., Zhou, T., Ma, J., Sun, X., and Lu, Z. (2004). The relationship between synonymous codon usage and protein structure in Escherichia coli and Homo sapiens. Biosystems 73, 89–97. doi: 10.1016/j.biosystems.2003.10.001
Hershberg, R., and Petrov, D. A. (2008). Selection on codon bias. Annu. Rev. Genet. 42, 287–299. doi: 10.1146/annurev.genet.42.110807.091442
Hershberg, R., and Petrov, D. A. (2010). Evidence that mutation is universally biased towards AT in bacteria. PLoS Genet. 6:e1001115. doi: 10.1371/journal.pgen.1001115
Hildebrand, F., Meyer, A., and Eyre-Walker, A. (2010). Evidence of selection upon genomic GC-content in bacteria. PLoS Genet. 6:e1001107. doi: 10.1371/journal.pgen.1001107
Hu, J., Zhao, X., Zhang, Z., and Yu, J. (2007). Compositional dynamics of guanine and cytosine content in prokaryotic genomes. Res. Microbiol. 158, 363–370. doi: 10.1016/j.resmic.2007.02.007
Ikemura, T. (1981). Correlation between the abundance of Escherichia coli transfer RNAs and the occurrence of the respective codons in its protein genes: a proposal for a synonymous codon choice that is optimal for the E. coli translational system. J. Mol. Biol. 151, 389–409.
Ikemura, T. (1985). Codon usage and tRNA content in unicellular and multicellular organisms. Mol. Biol. Evol. 2, 13–34.
Jain, R., Rivera, M. C., and Lake, J. A. (1999). Horizontal gene transfer among genomes: the complexity hypothesis. Proc. Natl. Acad. Sci. U.S.A. 96, 3801–3806. doi: 10.1073/pnas.96.7.3801
Kaas, R. S., Friis, C., Ussery, D. W., and Aarestrup, F. M. (2012). Estimating variation within the genes and inferring the phylogeny of 186 sequenced diverse Escherichia coli genomes. BMC Genomics 13:577. doi: 10.1186/1471-2164-13-577
Kaper, J. B., Nataro, J. P., and Mobley, H. L. (2004). Pathogenic Escherichia coli. Nat. Rev. Microbiol. 2, 123–140. doi: 10.1038/nrmicro818
Kessler, M. D., and Dean, M. D. (2014). Effective population size does not predict codon usage bias in mammals. Ecol. Evol. 4, 3887–3900. doi: 10.1002/ece3.1249
Knight, R. D., Freeland, S. J., and Landweber, L. F. (2001). A simple model based on mutation and selection explains trends in codon and amino-acid usage and GC composition within and across genomes. Genome Biol. 2:RESEARCH0010. doi: 10.1186/gb-2001-2-4-research0010
Koonin, E. V., Makarova, K. S., and Aravind, L. (2001). Horizontal gene transfer in prokaryotes: quantification and classification. Annu. Rev. Microbiol. 55, 709–742. doi: 10.1146/annurev.micro.55.1.709
Langmead, B., and Salzberg, S. L. (2012). Fast gapped-read alignment with Bowtie 2. Nat. Methods 9, 357–359. doi: 10.1038/nmeth.1923
Lassalle, F., Perian, S., Bataillon, T., Nesme, X., Duret, L., and Daubin, V. (2015). GC-Content evolution in bacterial genomes: the biased gene conversion hypothesis expands. PLoS Genet. 11:e1004941. doi: 10.1371/journal.pgen.1004941
Lewis, N. E., Hixson, K. K., Conrad, T. M., Lerman, J. A., Charusanti, P., Polpitiya, A. D., et al. (2010). Omic data from evolved E. coli are consistent with computed optimal growth from genome-scale models. Mol. Syst. Biol. 6, 390. doi: 10.1038/msb.2010.47
Li, X. Q., and Du, D. (2014). Variation, evolution, and correlation analysis of C+G content and genome or chromosome size in different kingdoms and phyla. PLoS ONE 9:e88339. doi: 10.1371/journal.pone.0088339
Lukjancenko, O., Wassenaar, T. M., and Ussery, D. W. (2010). Comparison of 61 sequenced Escherichia coli genomes. Microb. Ecol. 60, 708–720. doi: 10.1007/s00248-010-9717-3
Ma, L., Cui, P., Zhu, J., Zhang, Z., and Zhang, Z. (2014). Translational selection in human: more pronounced in housekeeping genes. Biol. Direct 9:17. doi: 10.1186/1745-6150-9-17
Medini, D., Donati, C., Tettelin, H., Masignani, V., and Rappuoli, R. (2005). The microbial pan-genome. Curr. Opin. Genet. Dev. 15, 589–594. doi: 10.1016/j.gde.2005.09.006
Mongodin, E. F., Casjens, S. R., Bruno, J. F., Xu, Y., Drabek, E. F., Riley, D. R., et al. (2013). Inter- and intra-specific pan-genomes of Borrelia burgdorferi sensu lato: genome stability and adaptive radiation. BMC Genomics 14:693. doi: 10.1186/1471-2164-14-693
Monk, J. M., Charusanti, P., Aziz, R. K., Lerman, J. A., Premyodhin, N., Orth, J. D., et al. (2013). Genome-scale metabolic reconstructions of multiple Escherichia coli strains highlight strain-specific adaptations to nutritional environments. Proc. Natl. Acad. Sci. U.S.A. 110, 20338–20343. doi: 10.1073/pnas.1307797110
Novembre, J. A. (2002). Accounting for background nucleotide composition when measuring codon usage bias. Mol. Biol. Evol. 19, 1390–1394.
Ochman, H., Lawrence, J. G., and Groisman, E. A. (2000). Lateral gene transfer and the nature of bacterial innovation. Nature 405, 299–304. doi: 10.1038/35012500
Oliver, J. L., and Marin, A. (1996). A relationship between GC content and coding-sequence length. J. Mol. Evol. 43, 216–223.
Plotkin, J. B., and Kudla, G. (2011). Synonymous but not the same: the causes and consequences of codon bias. Nat. Rev. Genet. 12, 32–42. doi: 10.1038/nrg2899
Raghavan, R., Kelkar, Y. D., and Ochman, H. (2012). A selective force favoring increased G+C content in bacterial genes. Proc. Natl. Acad. Sci. U.S.A. 109, 14504–14507. doi: 10.1073/pnas.1205683109
Ran, W., Kristensen, D. M., and Koonin, E. V. (2014). Coupling between protein level selection and codon usage optimization in the evolution of bacteria and archaea. mBio 5, e00956-e00914. doi: 10.1128/mBio.00956-14
Rasko, D. A., Rosovitz, M. J., Myers, G. S., Mongodin, E. F., Fricke, W. F., Gajer, P., et al. (2008). The pangenome structure of Escherichia coli: comparative genomic analysis of E. coli commensal and pathogenic isolates. J. Bacteriol. 190, 6881–6893. doi: 10.1128/JB.00619-08
Reichenberger, E. R., Rosen, G., Hershberg, U., and Hershberg, R. (2015). Prokaryotic nucleotide composition is shaped by both phylogeny and the environment. Genome Biol. Evol. 7, 1380–1389. doi: 10.1093/gbe/evv063
Sharp, P. M., Emery, L. R., and Zeng, K. (2010). Forces that influence the evolution of codon bias. Philos. Trans. R. Soc. Lond. B Biol. Sci. 365, 1203–1212. doi: 10.1098/rstb.2009.0305
Sharp, P. M., and Li, W. H. (1987). The codon Adaptation Index–a measure of directional synonymous codon usage bias, and its potential applications. Nucleic Acids Res. 15, 1281–1295.
Sharp, P. M., Stenico, M., Peden, J. F., and Lloyd, A. T. (1993). Codon usage: mutational bias, translational selection, or both? Biochem. Soc. Trans. 21, 835–841.
Stoletzki, N., and Eyre-Walker, A. (2007). Synonymous codon usage in Escherichia coli: selection for translational accuracy. Mol. Biol. Evol. 24, 374–381. doi: 10.1093/molbev/msl166
Sueoka, N. (1988). Directional mutation pressure and neutral molecular evolution. Proc. Natl. Acad. Sci. U.S.A. 85, 2653–2657.
Tettelin, H., Riley, D., Cattuto, C., and Medini, D. (2008). Comparative genomics: the bacterial pan-genome. Curr. Opin. Microbiol. 11, 472–477. doi: 10.1016/j.mib.2008.09.006
Touchon, M., Hoede, C., Tenaillon, O., Barbe, V., Baeriswyl, S., Bidet, P., et al. (2009). Organised genome dynamics in the Escherichia coli species results in highly diverse adaptive paths. PLoS Genet. 5:e1000344. doi: 10.1371/journal.pgen.1000344
Vernikos, G., Medini, D., Riley, D. R., and Tettelin, H. (2015). Ten years of pan-genome analyses. Curr. Opin. Microbiol. 23, 148–154. doi: 10.1016/j.mib.2014.11.016
Zhang, Z., Li, J., Cui, P., Ding, F., Li, A., Townsend, J. P., et al. (2012). Codon Deviation Coefficient: a novel measure for estimating codon usage bias and its statistical significance. BMC Bioinformatics 13:43. doi: 10.1186/1471-2105-13-43
Keywords: pangenome, codon usage bias, translational selection, mutation, core genes, strain-specific genes
Citation: Sun S, Xiao J, Zhang H and Zhang Z (2016) Pangenome Evidence for Higher Codon Usage Bias and Stronger Translational Selection in Core Genes of Escherichia coli. Front. Microbiol. 7:1180. doi: 10.3389/fmicb.2016.01180
Received: 22 March 2016; Accepted: 18 July 2016;
Published: 03 August 2016.
Edited by:
Feng Gao, Tianjin University, ChinaReviewed by:
Bin-Guang Ma, Huazhong Agricultural University, ChinaCopyright © 2016 Sun, Xiao, Zhang and Zhang. This is an open-access article distributed under the terms of the Creative Commons Attribution License (CC BY). The use, distribution or reproduction in other forums is permitted, provided the original author(s) or licensor are credited and that the original publication in this journal is cited, in accordance with accepted academic practice. No use, distribution or reproduction is permitted which does not comply with these terms.
*Correspondence: Huiyong Zhang, aHVpeW9uZy56aGFuZ0BoZW5hdS5lZHUuY24=
Zhang Zhang, emhhbmd6aGFuZ0BiaWcuYWMuY24=
Disclaimer: All claims expressed in this article are solely those of the authors and do not necessarily represent those of their affiliated organizations, or those of the publisher, the editors and the reviewers. Any product that may be evaluated in this article or claim that may be made by its manufacturer is not guaranteed or endorsed by the publisher.
Research integrity at Frontiers
Learn more about the work of our research integrity team to safeguard the quality of each article we publish.