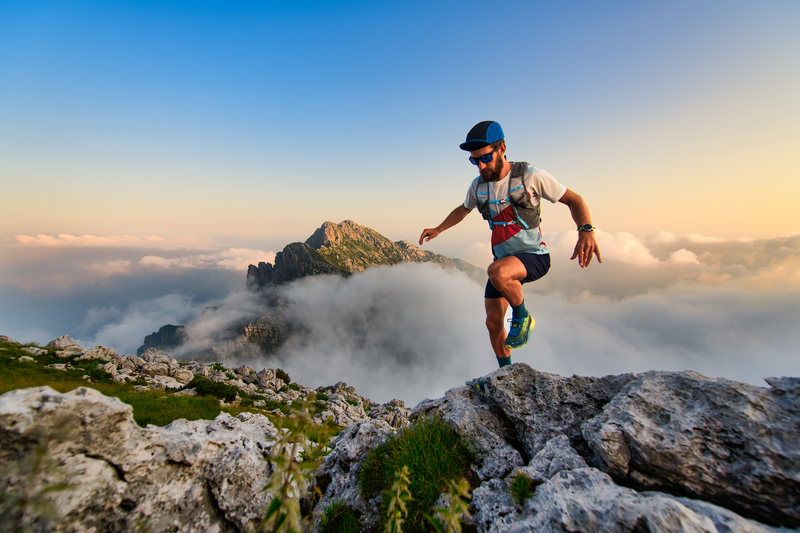
94% of researchers rate our articles as excellent or good
Learn more about the work of our research integrity team to safeguard the quality of each article we publish.
Find out more
PERSPECTIVE article
Front. Microbiol. , 18 February 2016
Sec. Microbiotechnology
Volume 7 - 2016 | https://doi.org/10.3389/fmicb.2016.00182
This article is part of the Research Topic Applied Microbiology for Chemical Syntheses View all 32 articles
The enzyme subclass of glycosyltransferases (GTs; EC 2.4) currently comprises 97 families as specified by CAZy classification. One of their important roles is in the biosynthesis of disaccharides, oligosaccharides, and polysaccharides by catalyzing the transfer of sugar moieties from activated donor molecules to other sugar molecules. In addition GTs also catalyze the transfer of sugar moieties onto aglycons, which is of great relevance for the synthesis of many high value natural products. Bacterial GTs show a higher sequence similarity in comparison to mammalian ones. Even when most GTs are poorly explored, state of the art technologies, such as protein engineering, domain swapping or computational analysis strongly enhance our understanding and utilization of these very promising classes of proteins. This perspective article will focus on bacterial GTs, especially on classification, screening and engineering strategies to alter substrate specificity. The future development in these fields as well as obstacles and challenges will be highlighted and discussed.
Glycosyltransferases (GTs) represent a subclass of enzymes that catalyze the synthesis of glycosidic linkages by the transfer of a sugar residue from a donor substrate to an acceptor. Acceptor substrates are mono-, di-, or oligo- carbohydrates, as well as proteins, lipids, DNA, and numerous other small molecules (Lairson et al., 2008). Therefore they play essential roles in biosynthesis pathways of oligo- and polysaccharides, as well as protein glycosylation and formation of valuable natural products (Lairson et al., 2008). Amongst the donor substrates, nucleotide-sugar conjugates represent the most prominent substrates (∼65%), but also lipid phosphate sugars and phosphate sugars are used (Ardèvol and Rovira, 2015). The mechanism for the regio- and stereo-specific transfer of the distinct sugar can occur via the inverting or retaining mechanism, which also defines the stereo-chemical outcome (α- or β-glucosides). The inverting mechanism follows a single displacement mechanism by a nucleophilic attack of the acceptor on the C-1 of the sugar donor inverting the anomeric stereochemistry. This mechanism is widely accepted and chemically elucidated (Schuman et al., 2013).
For retaining GTs different mechanisms have been proposed and the exact mechanism is still a matter of debate (Schuman et al., 2013). Latest findings based on quantum mechanics and molecular mechanics dynamic simulations indicate that two different enzyme families might have evolved, which follow either a double displacement (two SN2 reactions) mechanism or a front-face mechanism. One factor influencing the distinct mechanism will be the presence or absence of a putative nucleophile residue near the anomeric carbon of the donor sugar. Furthermore a competition between the front-face and double displacement mechanism was calculated by QM/MM for nucleophile-containing GTs (Rojas-Cervellera et al., 2013). The departure of the leaving group and the nucleophilic attack occur in an asynchronous manner at the same face of the glycoside (Ardèvol and Rovira, 2015).
For classification of GTs several approaches are used. The most prominent one is the classification by amino acid sequence similarities, as basically done by the Carbohydrate-Active enZYmes Database (CAZy). The CAZy database groups the different GTs into families. It comprises 97 families based on ∼215,930 entries (at 27th November, 2015). Additionally ∼4,015 sequences are not classified to this date. Nomenclature of the families is performed by use of GT and the following number of the GT family. Next to the EC 2.4 families, the CAZy classification also includes six families, belonging to EC 3.X and EC 5.X with around 395 entries. In 2012 the CAZy classification contained only ∼87,000 entries which were divided into 90 families, showing the fast development in the field of sequence identification of GTs (Gloster, 2014). In November 2015, the three families 36, 46, and 86 are still listed, but do not contain any sequences since no characterized members (GT-46) exist, or they have been deleted and merged with other GT-families (GT-36 and GT-86) based on newest findings. From the ∼215,930 listed sequences less than 1% (1,919) has been characterized. Structures are available for 161 of these, distributed over 41 families, which include solely three crystal structures, two of them for bacterial GTs. The statistical insights of the several GT-families as classified by CAZy are displayed in in Table 1.
Use of CAZy database for classification toward substrate specificity is done by conserved three-dimensional architecture for the structures of all nucleotide sugar dependent GTs. All structures of GTs solved to date adopt one of three folds, termed GT-A, GT-B, and GT-C (Gloster, 2014). The GT-A enzymes are generally dependent on divalent metal ions and comprise two β/α/β Rossmann-like domains. A highly conserved DXD motif within the active side coordinates the metal ion, which stabilizes the charged phosphate groups of the nucleotide sugar donor, therefore supporting departure of the leaving group. The three dimensional architecture of GT-B enzymes comprises two β/α/β Rossmann-like domains that face each other. These enzymes are generally independent of metal ions, and the active site is spatially located in between the two Rossmann-like domains. The residues of the active side are involved in leaving group departure. In 2003 there was identified the third family, the GT-C enzymes, which are hydrophobic integral membrane proteins having a modified DXD signature in the first extracellular loop, and mainly using lipid phosphate linked sugar donors (Liu and Mushegian, 2003; Gloster, 2014). The limited amount of structural folds in GTs might result from the potential evolutionary origin of only few precursors’ sequences. Only GTs belonging to GT2 and GT4 are found in ancient Archaea, and it is assumed that the other families may have evolved from these two families (Coutinho et al., 2003). The next step toward increased functional prediction and substrate specificity is the classification into clans that is performed by grouping families displaying similar fold, analogous catalytic apparatus and identical mechanism (Coutinho et al., 2003; Osmani et al., 2009). These approaches will end up in so called subfamilies to further increase the functional prediction, but are also guided by the need of the researcher. Mammalian and bacterial GTs show only a very low identity on amino acid sequence level even if they synthesize the same glycan linkages (Brockhausen, 2014). Plant and bacterial GTs also have a low identity on nucleotide and amino acid level, which is the same for bacterial GTs amongst themselves, but similarities might be identified on structural level (Gloster, 2014). Next to their role in protein- and natural product glycosylation or oligo- and polysaccharide biosynthesis, they can be used for tailored chemo-enzymatic synthesis of novel, modified natural products (Luzhetskyy and Bechthold, 2008). Additionally, they play essential roles in fundamental biological processes and might be exploited for novel medical applications (Wu et al., 2012; Zhan et al., 2015). Especially by in-depth characterization and altered sugar functionality of GTs, this emerging field may develop rapidly.
Screening of GT activity is essential to characterize the GTs identified in sequenced genomes and proof the predicted activity and specificity. Within the last years several assays for GTs have been published, and were reviewed by Palcic and Sujino (2001) and Wagner and Pesnot (2010) with focus on fluorescence based methods. In general, the depletion of the acceptor or the nucleotide donor or the formation of the nucleotide or the glycosylated product can be monitored. Because of the absence of changes in the fluorescence or UV/visible spectra by GT activity, other methods to monitor substrate conversion are needed. Additionally, the assays have to be highly sensitive, caused by the low concentration of GTs in natural sources. Therefore, radiochemical assays represent a good choice for quantitative monitoring of GT activity by use of commercially available radiolabeled donors, and following separation of the unreacted donor from labeled reaction products. Depending on the acceptors (saccharide, protein etc.), different separation (Electrophoresis, ion exchange Chromatography, Thin layer Chromatography, organic solvent extraction) and analysis techniques (GC-MS) can be applied (Palcic and Sujino, 2001). Within the last years, several scintillation proximity assays have been developed to realize high throughput screening (HTS) without tedious washing steps. These assays function by immobilizing the acceptor of the glycosylation reaction on scintillation-coated microspheres, which emit a light signal, when the radio emitting labeled sugar comes in close proximity during the reaction (Hood et al., 1998; Miyashiro et al., 2005; Ahsen et al., 2008).
Non-radioactive highly sensitive assays are realized by use of immunological methods such as antibodies or lectins, directly identifying the reaction products (Cummings and Etzler, 2009). Mostly glycolipid acceptors are used, which have to be removed after the reaction. Within the last years several HT-methods have been developed, which adsorb the acceptor to the surface of the micro titer plate (MTP)-wells, turning the acceptor into an immobilized product, which can be specifically stained after washing steps (Lira-Navarrete et al., 2011). Spectrophotometric assays can be realized by coupling different enzymes. For example, NDP that is released during glycosyl transfer can react with phosphenolpyruvate (pyruvate kinase), which then releases pyruvate that can be detected by following the decrease of 340 nm for the oxidation of NADH (lactate dehydrogenase). Several adapted versions have been developed, which can be applied in high throughput and small volume also for membrane bound GTs (Brown et al., 2012). A pragmatic approach is the use of pH-sensitive assays. The hydrolysis of the sugar-nucleotide donor substrates into the corresponding nucleoside diphosphate results in the release of proton equivalents for all GT-catalyzed glycosylation reactions. These protons cause a color change of pH-indicators, as shown for the first time by use of phenol red (Deng and Chen, 2004) or bromothymol blue (Persson and Palcic, 2008). Another assay variant based on malachite green monitors the free phosphate (Pi) as released from leaving nucleotides by phosphatases (Wu et al., 2011). The highly specific phosphatases do not act on sugar-nucleotides as substrate. Therefore, the concentration of released Pi is directly proportional to the sugar molecules transferred, enabling additional measurement of kinetic parameters. These kinds of colorimetric assays can be easily applied in HT-studies (Shen et al., 2010). Fluorescence based methods combine high sensitivity with operational simplicity and suitability for HTS (Gribbon and Sewing, 2003). Chemosensors with high binding selectivity toward pyrophosphate monoesters were successfully used to read out GT activity as well as inhibitor screening (Wongkongkatep et al., 2006). Assays for UTP/UDP, GDP and CMP selective fluorescent probes were developed (Chen et al., 2009) and commercial nucleotide immunodetection systems (TranscreenerTM) are available (Lowery and Kleman-Leyer, 2006). Additionally, more and more coupled assay variants arise within the last years (Kumagai et al., 2014). The use of mass spectrometry (MS) obtained high impact for GT screening and characterization (Norris et al., 2001; Yang et al., 2005; Ban et al., 2012; Lauber et al., 2015). A highly sophisticated HTS approach based on immobilized oligosaccharide acceptors, placed on gold-coated islands in the geometry of a 348-well MTP exist (Ban et al., 2012). By mixing and incubating unpurified, in vitro expressed proteins with different sugar donors on the immobilized acceptor molecules and following MS analysis enables characterization of GT specificity as well as additional kinetic information. This example impressively shows the power of MS-based methods, to screen and characterize GTs. One of the main obstacles of GT screening and characterization approaches is the membrane localization of GTs (associated or integral). Latest findings in the field of synthetic membranes, such as nano-disks might massively enhance the functional expression and in vitro screening options of GTs (Inagaki et al., 2013). Additionally, optimized expression and biotransformation systems such as Pichia Pastoris will massively enhance the screening and characterization efficiency (Ahmad et al., 2014; Ge et al., 2014).
The acceptor and donor specificity in GTs reside in different well separated domains. Engineering of GT sequences is a powerful tool for altering the acceptor and donor specificity by targeting the corresponding domains. Enhanced insights into the glycosylation mechanism was obtained by structural information for, e.g., plant GTs (Wang, 2009). For the GT-A fold enzymes the N- and C-terminal domains show dissimilar architecture. The N-terminal domain consists of several β-sheets, which all are flanked by α-helical Rossmann folds and are is responsible for recognition of the sugar-nucleotide donor (Davies et al., 2005; Jank et al., 2007; Mittler et al., 2007; Erb et al., 2009). The C-terminal domain mainly contains mixed β-sheets and is responsible for the binding of the acceptor molecule. In the case of GT-B fold GTs, the N- and C -terminal domains are formed by two similar Rossmann folds and have reversed functions. The N-terminal region includes the acceptor binding site and the C-terminal domain binds the donor substrate (Erb et al., 2009). The C-terminal domains show higher similarities since they recognize the same or similar donors, whereas the N-terminal domain shows lower similarity due to the greater varieties of acceptor molecules (Wang, 2009). The C- and N-terminal domains are connected via a linker and form a cleft which acts in the case of GT-B fold containing UDP-GTs as substrate binding site. There are several examples, which describe minor mutations to significantly alter the acceptor or donor acceptance of selected GTs (Hoffmeister et al., 2001, 2002; Williams et al., 2007, 2008b). Williams and Thorson (2008) developed a fluorescence-based HTS in conjunction with error-prone PCR/saturation mutagenesis to modify proficiency and promiscuity of GTs, resulting in 200–300-fold improved enzyme activity (2008). The massively altered substrate specificity (13 different UDP-sugars) was reached by mutation of three different amino acid substituents, which were identified to serve as “hot-spots” for directed evolution. The exchange of acceptor and donor recognizing domains was described to be partly successful. In some cases it was described that swapping of larger sequence elements successfully altered the substrate specificity, whereas exchange of the whole C- or N-terminal regions might also lead to inactive versions (Kohara et al., 2007), indicating that the acceptor recognition is not strictly encoded in the single domains. Sequential domain swapping approaches can be successfully applied to identify amino acids (even single ones are described) which are responsible for the altered regioselectivity of glycosylation (Cartwright et al., 2008). For bacterial GTs several swapping experiments are described which lead to chimeric GT variants having an exchanged specificity (Fischbach et al., 2007; Hansen et al., 2009; Krauth et al., 2009; Park et al., 2009). These experiments were mainly performed with highly homologous GTs and the true modular nature of, e.g., GT-B enzymes has yet to be proved (Williams et al., 2008a). Especially in the field of bacterial GTs involved in polysaccharide production only limited information concerning substrate specificity on structural level is available (Naegeli et al., 2014). But recent activities showed enhanced possibilities to further predict the substrate and product specificity (Sánchez-Rodríguez et al., 2014).
Difficulties with high-level expression, purification, and crystallization hampers crystal structure determinations for GTs (Breton et al., 2006). Additionally, the ratio of loops to secondary elements is high in GTs. Most of these loops have a high flexibility, therefore resulting in a low electron density, thus limiting the detailed description of the catalytic domains. Additionally, GTs show a donor substrate induced conformational change (open and closed active conformation), which mainly involves the flexible loops (Boix et al., 2001, 2002; Qasba et al., 2002; Ramakrishnan et al., 2002). The low degree of sequence similarity within most of the CAZY families renders molecular modeling difficult. Fold recognition as theoretical approach, named as “threading” (Godzik, 2003) categorizes GTs, but still had some limitations so far and needed experimental proof. Additionally, the weak scores in fold recognition of many GTs might indicate not yet identified novel folds, as recently shown, when a forth fold (GT-D) was proposed (Zhang et al., 2014). But, multivariate sequence analysis in combination with fold recognition proofed to be useful for predicting folds and mechanisms for Escherichia coli and Synechocystis GTs (Rosén et al., 2004). However, most models still have a low confidence index for flexible loops and the highly variable regions, what represent the major problem for modeling acceptor sites. Therefore it can only be applied when the target and the template have sufficient identity, to allow docking of nucleotide sugars and acceptors (Heissigerová et al., 2003). Specificity toward the sugar donor and acceptor was shown to be determined by a few critical residues in the binding site (Meech et al., 2012; Naegeli et al., 2014). Especially the flexible loops involved in GT mechanism have been the subject of studies by molecular dynamic (MD) simulation (Persson et al., 2001; Ramakrishnan and Qasba, 2001; Gunasekaran and Nussinov, 2004; Šnajdrová et al., 2004). These results demonstrate the correlated motions of several loops as well as the importance of contacts between loops in the mechanism (Breton et al., 2006). Docking of substrates is a difficult task because of the presence of phosphate and divalent cation as well as the flexibility of the nucleotide sugar, but appropriate energy parameters have been developed (Petrova et al., 1999) and latest reports show highly promising results to improve our understanding and prediction of substrate specificity of especially bacterial GTs (Zhang et al., 2014; Pandey et al., 2015; Zuegg et al., 2015).
Most computational methods make use of sequence-based comparisons for accurate prediction of substrate specificity. However, these approaches are rather limited due to the high sequence variability within the GT families. Sánchez-Rodríguez et al. (2014) used a sequence-based strategy combined with a network-based approach to infer the putative substrate classes of these predicted GTs thereby taking into account genomic organization. Due to the determination of several GT structures in the recent years (Gloster, 2014), structure-based approaches might be a promising alternative for substrate specificity prediction in the future. However, accurate ligand-protein binding affinity prediction, for a set of similar binders, is a major challenge. In general, docking calculations alone perform unsatisfactorily in these settings. But docking calculations, followed by MDs simulations and free energy calculations can be applied to improve the predictions, keeping in mind that glycosylation pattern of bacterial GTs is highly diverse and complex (e.g., rare sugars). Therefore, the transferred sugar moiety might not have been discovered yet (Figure 1).
FIGURE 1. General Flow-chart for the specific characterization of glycosyltransferases (GTs), based on optimized modeling and docking experiments in combination with final binding affinity estimation, to predict substrate specificity.
A number of studies have shown that refining docking calculations by performing MD and free energy calculations starting from docked ligand positions can increase the accuracy of binding affinity predictions (Stjernschantz et al., 2006; Carlsson et al., 2008; Wünsch et al., 2012; Jiang et al., 2013). The improved accuracy of the simulations is mainly due to the increased level of molecular details, using a flexible and explicitly solvated protein. To this end, one could try to identify binding pockets on the GT structures by using docking calculations of the sugar molecules. Moreover, one could then calculate and compare the binding affinities of different sugar molecules by means of MD simulations combined with free energy calculations, e.g., with the Molecular Mechanics Poisson-Boltzmann Surface Area method (MM-PBSA) to determine substrate specificity (Kollman et al., 2000). Additionally, further approaches such as HTS crystallization and characterization (Zhu et al., 2013) of bacterial GTs are necessary to enhance our knowledge on bacterial GTs, especially involved in oligo and polysaccharide biosynthesis. Additional improved structural, modeling, and mutational studies are needed and on the way to further progress in the understanding of these highly attractive class of enzymes. These approaches in combination with novel expression systems and sophisticated tools to analyze integral membrane GTs as well as carbohydrate analysis (Rühmann et al., 2014) will enable efficient utilization of this enzyme class to efficiently tailor natural products.
JS, DH, and VS outlined the structure of the manuscript. JS and DH wrote the main parts of the manuscript. JS, NS, and NW did statistics on CAZy database and were involved in writing. All authors read and approved the final manuscript
The authors declare that the research was conducted in the absence of any commercial or financial relationships that could be construed as a potential conflict of interest.
This work was supported by the German Research Foundation (DFG) and the Technische Universität München within the funding programme Open Access Publishing.
Ahmad, M., Hirz, M., Pichler, H., and Schwab, H. (2014). Protein expression in Pichia pastoris: recent achievements and perspectives for heterologous protein production. Appl. Microbiol. Biotechnol. 98, 5301–5317. doi: 10.1007/s00253-014-5732-5
Ahsen, O. V., Voigtmann, U., Klotz, M., Nifantiev, N., Schottelius, A., Ernst, A., et al. (2008). A miniaturized high-throughput screening assay for fucosyltransferase VII. Anal. Biochem. 372, 96–105. doi: 10.1016/j.ab.2007.08.029
Ardèvol, A., and Rovira, C. (2015). Reaction mechanisms in carbohydrate-active enzymes: glycoside hydrolases and glycosyltransferases. insights from ab initio quantum mechanics/molecular mechanics dynamic simulations. J. Am. Chem. Soc. 137, 7528–7547. doi: 10.1021/jacs.5b01156
Ban, L., Pettit, N., Li, L., Stuparu, A. D., Cai, L., Chen, W., et al. (2012). Discovery of glycosyltransferases using carbohydrate arrays and mass spectrometry. Nat. Chem. Biol. 8, 769–773. doi: 10.1038/nchembio.1022
Boix, E., Swaminathan, G. J., Zhang, Y., Natesh, R., Brew, K., and Acharya, K. R. (2001). Structure of UDP Complex of UDP-galactose:β-Galactoside-α-1,3-galactosyltransferase at 1.53-Å resolution reveals a conformational change in the catalytically important C terminus. J. Biol. Chem. 276, 48608–48614.
Boix, E., Zhang, Y., Swaminathan, G. J., Brew, K., and Acharya, K. R. (2002). Structural basis of ordered binding of donor and acceptor substrates to the retaining glycosyltransferase, α-1,3-galactosyltransferase. J. Biol. Chem. 277, 28310–28318. doi: 10.1074/jbc.M202631200
Breton, C., Šnajdrová, L., Jeanneau, C., Koča, J., and Imberty, A. (2006). Structures and mechanisms of glycosyltransferases. Glycobiology 16, 29R–37R. doi: 10.1093/glycob/cwj016
Brockhausen, I. (2014). Crossroads between bacterial and mammalian glycosyltransferases. Front. Immunol. 5:492. doi: 10.3389/fimmu.2014.00492
Brown, C., Leijon, F., and Bulone, V. (2012). Radiometric and spectrophotometric in vitro assays of glycosyltransferases involved in plant cell wall carbohydrate biosynthesis. Nat. Protoc. 7, 1634–1650. doi: 10.1038/nprot.2012.089
Carlsson, J., Boukharta, L., and Åqvist, J. (2008). Combining docking, molecular dynamics and the linear interaction energy method to predict binding modes and affinities for non-nucleoside inhibitors to HIV-1 reverse transcriptase. J. Med. Chem. 51, 2648–2656. doi: 10.1021/jm7012198
Cartwright, A. M., Lim, E. K., Kleanthous, C., and Bowles, D. J. (2008). A kinetic analysis of regiospecific glucosylation by two glycosyltransferases of Arabidopsis thaliana: domain swapping to introduce new activities. J. Biol. Chem. 283, 15724–15731. doi: 10.1074/jbc.M801983200
Chen, X., Jou, M. J., and Yoon, J. (2009). An “Off-On” type UTP/UDP selective fluorescent probe and its application to monitor glycosylation process. Org. Lett. 11, 2181–2184. doi: 10.1021/ol9004849
Coutinho, P. M., Deleury, E., Davies, G. J., and Henrissat, B. (2003). An evolving hierarchical family classification for glycosyltransferases. J. Mol. Biol. 328, 307–317. doi: 10.1016/S0022-2836(03)00307-3
Cummings, R. D., and Etzler, M. E. (2009). Antibodies and Lectins in Glycan Analysis. New York, NY: Cold Spring Harbor Laboratory Press.
Davies, G. J., Gloster, T. M., and Henrissat, B. (2005). Recent structural insights into the expanding world of carbohydrate-active enzymes. Curr. Opin. Struct. Biol. 15, 637–645. doi: 10.1016/j.sbi.2005.10.008
Deng, C., and Chen, R. R. (2004). A pH-sensitive assay for galactosyltransferase. Anal. Biochem. 330, 219–226. doi: 10.1016/j.ab.2004.03.014
Erb, A., Weiß, H., Härle, J., and Bechthold, A. (2009). A bacterial glycosyltransferase gene toolbox: generation and applications. Phytochemistry 70, 1812–1821. doi: 10.1016/j.phytochem.2009.05.019
Fischbach, M. A., Lai, J. R., Roche, E. D., Walsh, C. T., and Liu, D. R. (2007). Directed evolution can rapidly improve the activity of chimeric assembly-line enzymes. Proc. Natl. Acad. Sci. U.S.A. 104, 11951–11956. doi: 10.1073/pnas.0705348104
Ge, C., Gomez-Llobregat, J., Skwark, M. J., Ruysschaert, J. M., Wieslandg glyer, A., and Linden, M. (2014). Membrane remodeling capacity of a vesicle-inducincosyltransferase. FEBS J. 281, 3667–3684. doi: 10.1111/febs.12889
Gloster, T. M. (2014). Advances in understanding glycosyltransferases from a structural perspective. Curr. Opin. Struct. Biol. 28, 131–141. doi: 10.1016/j.sbi.2014.08.012
Gribbon, P., and Sewing, A. (2003). Fluorescence readouts in HTS: no gain without pain? Drug Discov. Today 8, 1035–1043. doi: 10.1016/S1359-6446(03)02895-2
Gunasekaran, K., and Nussinov, R. (2004). Modulating functional loop movements: the role of highly conserved residues in the correlated loop motions. ChemBioChem 5, 224–230. doi: 10.1002/cbic.200300732
Hansen, E. H., Osmani, S. A., Kristensen, C., Møller, B. L., and Hansen, J. (2009). Substrate specificities of family 1 UGTs gained by domain swapping. Phytochemistry 70, 473–482. doi: 10.1016/j.phytochem.2009.01.013
Heissigerová, H., Breton, C., Moravcová, J., and Imberty, A. (2003). Molecular modeling of glycosyltransferases involved in the biosynthesis of blood group A, blood group B, Forssman, and iGb3 antigens and their interaction with substrates. Glycobiology 13, 377–386. doi: 10.1093/glycob/cwg042
Hoffmeister, D., Ichinose, K., and Bechthold, A. (2001). Two sequence elements of glycosyltransferases involved in urdamycin biosynthesis are responsible for substrate specificity and enzymatic activity. Chem. Biol. 8, 557–567. doi: 10.1016/S1074-5521(01)00039-4
Hoffmeister, D., Wilkinson, B., Foster, G., Sidebottom, P. J., Ichinose, K., and Bechthold, A. (2002). Engineered urdamycin glycosyltransferases are broadened and altered in substrate specificity. Chem. Biol. 9, 287–295. doi: 10.1016/S1074-5521(02)00114-X
Hood, C. M., Kelly, V. A., Bird, M. I., and Britten, C. J. (1998). Measurement of α(1-3) fucosyltransferase activity using scintillation proximity. Anal. Biochem. 255, 8–12. doi: 10.1006/abio.1997.2449
Inagaki, S., Ghirlandob, R., and Grisshamme, R. (2013). Biophysical characterization of membrane proteins in nanodiscs. Methods 59, 287–300. doi: 10.1016/j.ymeth.2012.11.006
Jank, T., Giesemann, T., and Aktories, K. (2007). Rho-glucosylating Clostridium difficile toxins A and B: new insights into structure and function. Glycobiology 17, 15R–22R. doi: 10.1093/glycob/cwm004
Jiang, Q.-Q., Bartsch, L., Sicking, W., Wich, P. R., Heider, D., Hoffmann, D., et al. (2013). A new approach to inhibit human [small beta]-tryptase by protein surface binding of four-armed peptide ligands with two different sets of arms. Organ. Biomol. Chem. 11, 1631–1639. doi: 10.1039/c3ob27302d
Kohara, A., Nakajima, C., Yoshida, S., and Muranaka, T. (2007). Characterization and engineering of glycosyltransferases responsible for steroid saponin biosynthesis in Solanaceous plants. Phytochemistry 68, 478–486. doi: 10.1016/j.phytochem.2006.11.020
Kollman, P. A., Massova, I., Reyes, C., Kuhn, B., Huo, S., Chong, L., et al. (2000). Calculating structures and free energies of complex molecules:? combining molecular mechanics and continuum models. Acc. Chem. Res. 33, 889–897. doi: 10.1021/ar000033j
Krauth, C., Fedoryshyn, M., Schleberger, C., Luzhetskyy, A., and Bechthold, A. (2009). Engineering a function into a glycosyltransferase. Chem. Biol. 16, 28–35. doi: 10.1016/j.chembiol.2008.12.003
Kumagai, K., Kojima, H., Okabe, T., and Nagano, T. (2014). Development of a highly sensitive, high-throughput assay for glycosyltransferases using enzyme-coupled fluorescence detection. Anal. Biochem. 447, 146–155. doi: 10.1016/j.ab.2013.11.025
Lairson, L. L., Henrissat, B., Davies, G. J., and Withers, S. G. (2008). Glycosyltransferases: structures, functions, and mechanisms. Annu. Rev. Biochem. 77, 521–555. doi: 10.1146/annurev.biochem.76.061005.092322
Lauber, J., Handrick, R., Leptihn, S., Durre, P., and Gaisser, S. (2015). Expression of the functional recombinant human glycosyltransferase GalNAcT2 in Escherichia coli. Microb. Cell Fact. 14:3. doi: 10.1186/s12934-014-0186-0
Lira-Navarrete, E., Valero-González, J., Villanueva, R., Martínez-Júlvez, M., Tejero, T., Merino, P., et al. (2011). Structural insights into the mechanism of protein O-Fucosylation. PLoS ONE 6:e25365. doi: 10.1371/journal.pone.0025365
Liu, J., and Mushegian, A. (2003). Three monophyletic superfamilies account for the majority of the known glycosyltransferases. Protein Sci. 12, 1418–1431. doi: 10.1110/ps.0302103
Lowery, R. G., and Kleman-Leyer, K. (2006). Transcreener: screening enzymes involved in covalent regulation. Expert Opin. Ther. Targets 10, 179–190. doi: 10.1517/14728222.10.1.179
Luzhetskyy, A., and Bechthold, A. (2008). Features and applications of bacterial glycosyltransferases: current state and prospects. Appl. Microbiol. Biotechnol. 80, 945–952. doi: 10.1007/s00253-008-1672-2
Meech, R., Rogers, A., Zhuang, L., Lewis, B. C., Miners, J. O., and Mackenzie, P. I. (2012). Identification of residues that confer sugar selectivity to UDP glycosyltransferase 3A (UGT3A) enzymes. J. Biol. Chem. 287, 24122–24130. doi: 10.1074/jbc.M112.343608
Mittler, M., Bechthold, A., and Schulz, G. E. (2007). Structure and action of the C–C bond-forming glycosyltransferase UrdGT2 involved in the biosynthesis of the antibiotic urdamycin. J. Mol. Biol. 372, 67–76. doi: 10.1016/j.jmb.2007.06.005
Miyashiro, M., Furuya, S., and Sugita, T. (2005). A high-throughput screening system for α1–3 fucosyltransferase-VII inhibitor utilizing scintillation proximity assay. Anal. Biochem. 338, 168–170. doi: 10.1016/j.ab.2004.11.028
Naegeli, A., Michaud, G., Schubert, M., Lin, C.-W., Lizak, C., Darbre, T., et al. (2014). Substrate specificity of cytoplasmic N-glycosyltransferase. J. Biol. Chem. 289, 24521–24532. doi: 10.1074/jbc.M114.579326
Norris, A. J., Whitelegge, J. P., Faull, K. F., and Toyokuni, T. (2001). Analysis of enzyme kinetics using electrospray ionization mass spectrometry and multiple reaction monitoring: fucosyltransferase V†. Biochemistry 40, 3774–3779. doi: 10.1021/bi010029v
Osmani, S. A., Bak, S., and Møller, B. L. (2009). Substrate specificity of plant UDP-dependent glycosyltransferases predicted from crystal structures and homology modeling. Phytochemistry 70, 325–347. doi: 10.1016/j.phytochem.2008.12.009
Palcic, M. M., and Sujino, K. (2001). Assays for Glycosyltransferases. Trends Glycosci. Glycotechnol. 13, 361–370. doi: 10.4052/tigg.13.361
Pandey, V., Dhar, Y., Gupta, P., Bag, S., Atri, N., Asif, M., et al. (2015). Comparative interactions of withanolides and sterols with two members of sterol glycosyltransferases from Withania somnifera. BMC Bioinformatics 16:120. doi: 10.1186/s12859-015-0563-7
Park, S.-H., Park, H.-Y., Sohng, J. K., Lee, H. C., Liou, K., Yoon, Y. J., et al. (2009). Expanding substrate specificity of GT-B fold glycosyltransferase via domain swapping and high-throughput screening. Biotechnol. Bioeng. 102, 988–994. doi: 10.1002/bit.22150
Persson, K., Ly, H. D., Dieckelmann, M., Wakarchuk, W. W., Withers, S. G., and Strynadka, N. C. J. (2001). Crystal structure of the retaining galactosyltransferase LgtC from Neisseria meningitidis in complex with donor and acceptor sugar analogs. Nat. Struct. Mol. Biol. 8, 166–175. doi: 10.1038/84168
Persson, M., and Palcic, M. M. (2008). A high-throughput pH indicator assay for screening glycosyltransferase saturation mutagenesis libraries. Anal. Biochem. 378, 1–7. doi: 10.1016/j.ab.2008.03.006
Petrova, P., Koca, J., and Imberty, A. (1999). Potential energy hypersurfaces of nucleotide-sugars: Ab initio calculations, force-field parametrization, and exploration of the flexibility. J. Am. Chem. Soc. 121, 5535–5547. doi: 10.1021/ja983854g
Qasba, P. K., Ramakrishnan, B., and Boeggeman, E. (2002). Substrate-induced conformational changes in glycosyltransferases. Trends Biochem. Sci. 30, 53–62. doi: 10.1016/j.tibs.2004.11.005
Ramakrishnan, B., Balaji, P. V., and Qasba, P. K. (2002). Crystal structure of β1,4-galactosyltransferase complex with UDP-Gal reveals an oligosaccharide acceptor binding site. J. Mol. Biol. 318, 491–502. doi: 10.1016/S0022-2836(02)00020-7
Ramakrishnan, B., and Qasba, P. K. (2001). Crystal structure of lactose synthase reveals a large conformational change in its catalytic component, the β1,4-galactosyltransferase-I1. J. Mol. Biol. 310, 205–218. doi: 10.1006/jmbi.2001.4757
Rojas-Cervellera, V., Ardèvol, A., Boero, M., Planas, A., and Rovira, C. (2013). Formation of a covalent glycosyl–enzyme species in a retaining glycosyltransferase. Chem. A Eur. J. 19, 14018–14023. doi: 10.1002/chem.201302898
Rosén, M. L., Edman, M., Sjöström, M., and Wieslander, Å (2004). Recognition of fold and sugar linkage for glycosyltransferases by multivariate sequence analysis. J. Biol. Chem. 279, 38683–38692. doi: 10.1074/jbc.M402925200
Rühmann, B., Schmid, J., and Sieber, V. (2014). Fast carbohydrate analysis via liquid chromatography coupled with ultra violet and electrospray ionization ion trap detection in 96-well format. J. Chromatogr. A 1350, 44–50. doi: 10.1016/j.chroma.2014.05.014
Sánchez-Rodríguez, A., Tytgat, H., Winderickx, J., Vanderleyden, J., Lebeer, S., and Marchal, K. (2014). A network-based approach to identify substrate classes of bacterial glycosyltransferases. BMC Genomics 15:349. doi: 10.1186/1471-2164-15-349
Schuman, B., Evans, S. V., and Fyles, T. M. (2013). Geometric attributes of retaining glycosyltransferase enzymes favor an orthogonal mechanism. PLoS ONE 8:e71077. doi: 10.1371/journal.pone.0071077
Shen, R., Wang, S., Ma, X., Xian, J., Li, J., Zhang, L., et al. (2010). An easy colorimetric assay for glycosyltransferases. Biochemistry (Mosc) 75, 944–950. doi: 10.1134/S0006297910070187
Šnajdrová, L., Kulhánek, P., Imberty, A., and Koča, J. (2004). Molecular dynamics simulations of glycosyltransferase LgtC. Carbohydr. Res. 339, 995–1006. doi: 10.1016/j.carres.2003.12.024
Stjernschantz, E., Marelius, J., Medina, C., Jacobsson, M., Vermeulen, N. P. E., and Oostenbrink, C. (2006). Are automated molecular dynamics simulations and binding free energy calculations realistic tools in lead optimization? An evaluation of the linear interaction energy (LIE) method. J. Chem. Inform. Model. 46, 1972–1983. doi: 10.1021/ci0601214
Wagner, G. K., and Pesnot, T. (2010). Glycosyltransferases and their assays. Chembiochem 11, 1939–1949. doi: 10.1002/cbic.201000201
Wang, X. (2009). Structure, mechanism and engineering of plant natural product glycosyltransferases. FEBS Lett. 583, 3303–3309. doi: 10.1016/j.febslet.2009.09.042
Williams, G. J., Gantt, R. W., and Thorson, J. S. (2008a). The impact of enzyme engineering upon natural product glycodiversification. Curr. Opin. Chem. Biol. 12, 556–564. doi: 10.1016/j.cbpa.2008.07.013
Williams, G. J., Goff, R. D., Zhang, C., and Thorson, J. S. (2008b). Optimizing glycosyltransferase specificity via “hot spot” saturation mutagenesis presents a catalyst for novobiocin glycorandomization. Chem. Biol. 15, 393–401. doi: 10.1016/j.chembiol.2008.02.017
Williams, G. J., and Thorson, J. S. (2008). A high-throughput fluorescence-based glycosyltransferase screen and its application in directed evolution. Nat. Protoc. 3, 357–362. doi: 10.1038/nprot.2007.538
Williams, G. J., Zhang, C., and Thorson, J. S. (2007). Expanding the promiscuity of a natural-product glycosyltransferase by directed evolution. Nat. Chem. Biol. 3, 657–662. doi: 10.1038/nchembio.2007.28
Wongkongkatep, J., Miyahara, Y., Ojida, A., and Hamachi, I. (2006). Label-free, real-time glycosyltransferase assay based on a fluorescent artificial chemosensor. Angew. Chem. Int. Ed. Engl. 45, 665–668. doi: 10.1002/anie.200503107
Wu, C.-Z., Jang, J.-K., Woo, M., Ahn, J. S., Kim, J. S., and Hong, Y.-S. (2012). Enzymatic glycosylation of nonbenzoquinone geldanamycin analogs via Bacillus UDP-glycosyltransferase. Appl. Environ. Microbiol. 78, 7680–7686. doi: 10.1128/AEM.02004-12
Wu, Z. L., Ethen, C. M., Prather, B., Machacek, M., and Jiang, W. (2011). Universal phosphatase-coupled glycosyltransferase assay. Glycobiology 21, 727–733. doi: 10.1093/glycob/cwq187
Wünsch, D., Fetz, V., Heider, D., Tenzer, S., Bier, C., Kunst, L., et al. (2012). Chemico-genetic strategies to inhibit the leukemic potential of threonine aspartase-1. Blood Cancer J. 2, e77. doi: 10.1038/bcj.2012.22
Yang, M., Brazier, M., Edwards, R., and Davis, B. G. (2005). High-throughput mass-spectrometry monitoring for multisubstrate enzymes: determining the kinetic parameters and catalytic activities of glycosyltransferases. Chembiochem 6, 346–357. doi: 10.1002/cbic.200400100
Zhan, Y., Zhao, F., Xie, P., Zhong, L., Li, D., Gai, Q., et al. (2015). Mechanism of the effect of glycosyltransferase GLT8D2 on fatty liver. Lipids Health Dis. 14, 43. doi: 10.1186/s12944-015-0040-3
Zhang, H., Zhu, F., Yang, T., Ding, L., Zhou, M., Li, J., et al. (2014). The highly conserved domain of unknown function 1792 has a distinct glycosyltransferase fold. Nat. Commun. 5:4339. doi: 10.1038/ncomms5339
Zhu, F., Wu, R., Zhang, H., and Wu, H. (2013). Structural and biochemical analysis of a bacterial glycosyltransferase. Methods Mol. Biol. (Clifton, N.J.) 1022, 29–39. doi: 10.1007/978-1-62703-465-4_3
Keywords: screening, bacterial glycosyltransferases, categorization of glycosyltransferases, substrate specificity, docking experiments, polysaccharide glycosyltransferases
Citation: Schmid J, Heider D, Wendel NJ, Sperl N and Sieber V (2016) Bacterial Glycosyltransferases: Challenges and Opportunities of a Highly Diverse Enzyme Class Toward Tailoring Natural Products. Front. Microbiol. 7:182. doi: 10.3389/fmicb.2016.00182
Received: 04 December 2015; Accepted: 02 February 2016;
Published: 18 February 2016.
Edited by:
Robert Kourist, Ruhr-University Bochum, GermanyReviewed by:
Martin Siemann-Herzberg, University of Stuttgart, GermanyCopyright © 2016 Schmid, Heider, Wendel, Sperl and Sieber. This is an open-access article distributed under the terms of the Creative Commons Attribution License (CC BY). The use, distribution or reproduction in other forums is permitted, provided the original author(s) or licensor are credited and that the original publication in this journal is cited, in accordance with accepted academic practice. No use, distribution or reproduction is permitted which does not comply with these terms.
*Correspondence: Jochen Schmid, ai5zY2htaWRAdHVtLmRl
Disclaimer: All claims expressed in this article are solely those of the authors and do not necessarily represent those of their affiliated organizations, or those of the publisher, the editors and the reviewers. Any product that may be evaluated in this article or claim that may be made by its manufacturer is not guaranteed or endorsed by the publisher.
Research integrity at Frontiers
Learn more about the work of our research integrity team to safeguard the quality of each article we publish.