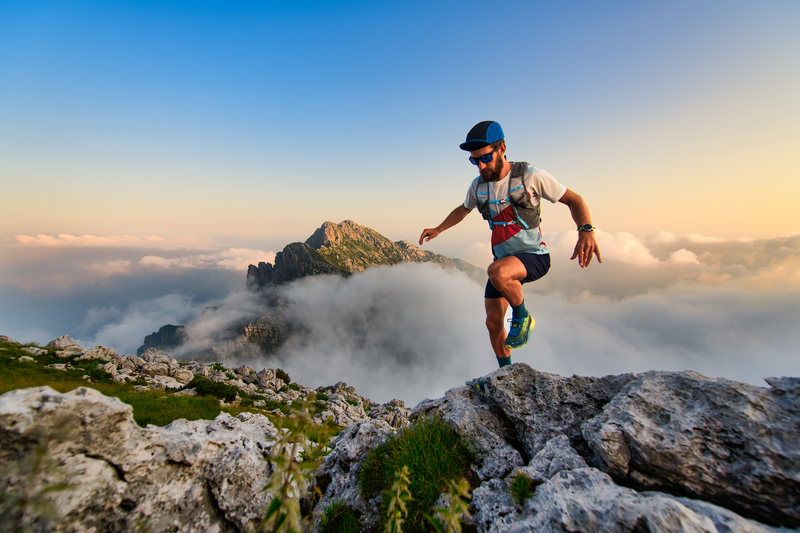
94% of researchers rate our articles as excellent or good
Learn more about the work of our research integrity team to safeguard the quality of each article we publish.
Find out more
ORIGINAL RESEARCH article
Front. Microbiol. , 02 October 2015
Sec. Aquatic Microbiology
Volume 6 - 2015 | https://doi.org/10.3389/fmicb.2015.01038
This article is part of the Research Topic Anthropogenic impacts on the microbial ecology and function of aquatic environments View all 21 articles
The aim of this study was to investigate the planktonic and the holobiont Madracis decactis (Scleractinia) microbial diversity along a turbulence-driven upwelling event, in the world's most isolated tropical island, St Peter and St Paul Archipelago (SPSPA, Brazil). Twenty one metagenomes were obtained for seawater (N = 12), healthy and bleached holobionts (N = 9) before, during and after the episode of high seawater turbulence and upwelling. Microbial assemblages differed between low turbulence-low nutrient (LLR) and high-turbulence-high nutrient (HHR) regimes in seawater. During LLR there was a balance between autotrophy and heterotrophy in the bacterioplankton and the ratio cyanobacteria:heterotrophs ~1 (C:H). Prochlorales, unclassified Alphaproteobacteria and Euryarchaeota were the dominant bacteria and archaea, respectively. Basic metabolisms and cyanobacterial phages characterized the LLR. During HHR C:H < < 0.05 and Gammaproteobacteria approximated 50% of the most abundant organisms in seawater. Alteromonadales, Oceanospirillales, and Thaumarchaeota were the dominant bacteria and archaea. Prevailing metabolisms were related to membrane transport, virulence, disease, and defense. Phages targeting heterotrophs and virulence factor genes characterized HHR. Shifts were also observed in coral microbiomes, according to both annotation–indepent and -dependent methods. HHR bleached corals metagenomes were the most dissimilar and could be distinguished by their di- and tetranucleotides frequencies, Iron Acquision metabolism and virulence genes, such as V. cholerae-related virulence factors. The healthy coral holobiont was shown to be less sensitive to transient seawater-related perturbations than the diseased animals. A conceptual model for the turbulence-induced shifts is put forward.
Marine microbial communities are recognized as engines of globally important processes, such as the marine carbon, nitrogen and sulfur cycles (Falkowski et al., 2008; Fuhrman, 2009). Only recently with the introduction of molecular techniques have satisfactory descriptions of natural microbial assemblages been generated (Fierer and Jackson, 2006; Rusch et al., 2007; Costello et al., 2009). Nevertheless, most marine ecosystems are understudied. As a result, there is limited information on the diversity of microbial assemblages in changing environments and on the environmental drivers of microbial diversity shifts (Karl, 2002).
Nutrient dynamics in the sea is inextricably linked to variations in physical processes. Either enhanced nutrient delivery from turbulent mixing or upwelling, or enhanced stratification can lead to shifts in microbial assemblages, with significant consequences for nutrient cycling (Cullen et al., 2002). Episodic mixing events must occur in order to balance supply and demand (Hayward, 1987, 1991). Because open-ocean microbial assemblages are metabolically active with a potential for relatively high specific growth, they are poised to respond quickly and effectively to environmental perturbations (Karl, 2002). A model of turbulence-nutrients regimes decoupled characteristics and adaptations of phytoplankton assemblages and how they relate to food web structure: (i) high turbulence—low nutrients: low biomass, slow turnover, adaptations for efficient use of light and nutrients (e.g., iron-limited, high latitude waters); (ii) LLR: smaller cells, high turnover, competition for nutrients, retention by recycling (microbial loop); (iii) low turbulence—high nutrients: larger cells, higher biomass, slower turnover, selective pressure to sequester nutrients and minimize losses (e.g., noxious toxic blooms); and (iv) HHR: larger cells, higher biomass, transient, and self-limiting selection for rapid growth (e.g., diatoms). According to this model, the microbial loop is present in all regimes but it dominates the biomass in the low turbulence—high nutrients regime (Cullen et al., 2002).
Some physical mechanisms that vertically supply nutrients from below to the oligotrophic oceanic surface layers are: (i) internal waves and tides, (ii) cyclonic mesoscale eddies, (iii) wind-driven Ekman pumping, and (iv) atmospheric storms (Karl, 1999). Internal waves are ubiquitous in deep-ocean environments (Garrett and Munk, 1972), and it appears that the high vertical shear of low-frequency internal waves contributes to occasional pulses of vertical mixing (Gregg et al., 1986; Sherman and Pinkel, 1991). Stochastic events, that may be short-lived, are of major ecological significance. They are undoubtedly undersampled by ship-based observation programs (Platt et al., 1989). Even a monthly sampling schedule, as adopted in the Hawaii Ocean Time-series (HOT) program, is considered too infrequent to register important but intermittent nutrient injections (Karl, 1999). Furthermore, most studies are restricted to the Pacific, Caribbean, and North Atlantic.
St. Peter and St. Paul Archipelago (SPSPA) are the smallest and most isolated tropical islands in the world. It comprises the most seaward Brazilian oceanic islands (~1000 km from mainland) and the unique on the northern hemisphere, lying 100 km off the equator (00°55′N; 29°22′W). Data from the Sea-viewing Wide Field-of-view Sensor (SeaWiFS) evinces the chlorophyll pattern characteristic of mesotrophic waters (0.1–0.3 mg.m−3 Chla) (Supplementary Figures 1A,B), according to the classification of Shushkina et al. (1997). SPSPA is located in the biogeochemical Western Tropical Atlantic Province (WTRA) (Longhurst et al., 1995), under the influence of the Intertropical Convergence Zone. Its formed by minute summits of the Mid-Atlantic Ridge (MAR) within the St Paul Fault Zone (FZ), between the South American and the African Plates (Supplementary Figures 2A,B), where seismicity is frequent (Campos et al., 2010). The islets are devoid of shore and consist entirely of steep scarpments extending to 60–100 m depth, with the most limited area of shallow habitat among oceanic islands (~200 m) (Robertson, 2001). Within 2 km diameter bathyal depths are reached and within 5 km depths fall within the abyssal range (−3.600 to 5.000; Supplementary Figure 2B). The South Equatorial Current (SEC) flows E-W superficially and the Atlantic Equatorial Undercurrent (EUC) flows W-E at 60–100 m depth (Edwards and Lubbock, 1983). The latter is one of the fastest, varying and least predictable among the Atlantic currents, which reaches 120 cm.seg−1 above the thermocline (Philander, 1986). A permanent thermocline may prevent deep water masses to emerge (Macedo et al., 2009) nevertheless the eventual presence of deep water Pleurommama spp. and Heterorhabdus spp at the shallow layer during the diurnal period is contradictory to the former assumption (Macedo-Soares et al., 2009). There are no reports on local hydrodynamics but, seemingly, intermittent vertical flow of deep water masses should result from the violent interaction with the geomorphology—MAR—a perpendicular barrier to the currents. In principle, the friction between the SEC and EUC masses, which flow in opposing directions, should promote and intensify episodic extensions of the thermohaline to the photic zone.
Moreira et al. (2014) reported a significant enrichment process along an 8 days period during which an ever-growing turbulence with surge pulses was observed in SPSPA. The process occurred along the lunar phase from crescent to full moon. The work performed the first (and unique to date) characterization of the culturable heterotrophic bacterial community of SPSPA. Bacterial counts (colony forming units, CFU) correlated positively with nutrients in seawater, which in turn correlated positively with turbulence—energy and frequency of the surges. In the present work we analyzed the metagenomic composition and diversity of both the planktonic microbial assemblages and in the scleractinian coral M. decactis along the same period in the same locale. The aim was to characterize the microbial diversity during an upwelling-driven nutrient enrichment. We did not expect to find bleached corals in SPSPA. The coral holobionts were targeted in this survey to investigate whether there was a correlation between the metagenomic features and seawater parameters (vibrio counts, nutrients, bacterioplankton composition). Sampling was performed before, during and after a turbulence surge. It was a short-lived event, which is locally recurrent. We analyzed 21 metagenomic samples of seawater (n = 12), healthy and bleached corals (n = 9). The findings are summarized in a model of the physico-chemical-biological dynamics in SPSPA, where a cyclic recurrent pattern with extreme regimes of low turbulence-low nutrients (LLR) and high turbulence-high nutrients (HHR) is hypothesized to contribute to structure the marine ecosystem in that barren archipelago.
In brief, sampling was performed by SCUBA diving at the Sub-caulerpa zone (mesophotic), according to the zonation of (Edwards and Lubbock, 1983; Moreira et al., 2014). The survey took place along the NW side of the archipelago from the inlet (ca 4500 m2) to Belmonte islet's contiguous vertical wall. The satellite view and topograghy of the inlet are shown in Supplementary Figures 1C, 2B, respectively. The turbulence surge occurred along the lunar phase from crescent (14/Sep/2010) to full moon (22/Sep/2010). The peak of the surge overlapped with cloudiness, wind and rain. Rain and swash flushed guano, a possible additional source of nutrients (Gagnon et al., 2013), from the cays into the bay. Samples were obtained from the onset (LLR, t1 = 14/Sep/2010), while enhancing (t2 = 15; t3 = 18/Sep/2010, HHR) and almost to recovery of LLR condition, or recovery for short (t4 = 22/set/2010). During climax (20–21/Sep/2010) the strong vortex precluded diving (Supplementary Video 1). Henceforth samples will be referred as 14, 15, 18, and 22. In total, 12 colony fragments (10 × 10 cm) of M. decactis (healthy: n = 8; bleached: n = 4) were collected with hammer and chisel. On days 14 and 15 bleached corals were not found. Coral samples processed for metagenomics were: (i) healthy corals (Mad): 14 (n = 1), 15 (n = 2), 18 (n = 1), and 22 (n = 1); and (ii) bleached corals (MadBle): 18 (n = 2) and 22 (n = 2). Seawater was sampled from the water column immediately above the corals (< 1 m) (4 samples: 14, 15, 18, and 22; 20 L/sample; 3 sterivex/sample). All samples were taken immediately to the Scientific Staion (SS) Laboratory, 20 m from the pier (Supplementary Figure 1D; view of the pier and the SS from the water at LLR). Seawater was filtered. Filters and coral samples were preserved in liquid nitrogen until DNA extraction (no longer than 3 months after).
Is a colonial zooxanthellate scleractinian coral. It has a variable bathymetric distribution, from 3 to 30 and up to 100 m (Neves and Johnsson, 2009). It is widespread in Brazil (N to SE), in Caribe, Gulf of Mexico and locally found in the Southeastern Atlantic (West Africa) (World Register of marine Species—WORMS: www.marinespecies.org). Free-living colonies of M. decactis display an unique formation off southern Brazil, at Galé Island. Spheroid shape, a.k.a. circumrotatory colonies, form the first corallith site discovered in the subtropical South Atlantic Ocean (SAO), at 6–15 m depth over 3400 m2 (coralreeefs-2012). In São Paulo (SE Brazil) it's a major contributor of reef structures, where bleaching has been seriously affecting its populations (Migotto, 1997), adding interest to the study of this coral species. In SPSPA, its one of the two scleractinian species locally found, mostly at the mesophotic zone. Healthy, bleached and with scars left by fish predation (Stegastes sanctipauli, Pomacentridae; Halichoeres radiatus, Labridae) in SPSPA are shown in Supplementary Figure 3.
Environmental parameters were analyzed by standard oceanographic methods with at least three replicates for each parameter and determined by Moreira et al. (2014). Temperature was recorded in situ with a HOBO UA-002-64/date Logger and UEMIS dive computer from 5, 15, 33, 45, and 65 m depth (n = 5 for each depth), during September/2010 and June/2011 (published in Crespo et al., 2014). Environmental data from (Moreira et al., 2014) and temperature from (Crespo et al., 2014) are summarized in Supplementary Figure 4, for aid in data interpretation.
Corals' DNA extraction was performed as in Trindade-Silva et al. (2012). Seawater was sequentially pre-filtered (100 and 20 μm) by gravity and then filtered on the Sterivex (0.22 μm) by positive pressure using Niskin system (2 L/Sterivex). The microbes collected at Sterivex filters were preserved with SET buffer (20% sucrose, 50 mM EDTA and 0.5 mM Tris–HCl). Metagenomic DNA extraction was performed using lysozyme (1 mg/mL) for 1 h at 37°C. Then, proteinase K (0.2 mg/mL) and sodium dodecyl sulfate (SDS) (1% v/v) were added and incubated (55°C; 60 min) under agitation. The lysate was rinsed into SET buffer (1 mL). Organic extraction was performed with one volume of phenol:chloroform:isoamyl alcohol (25:24:1). DNA precipitation was performed with ethanol (2,5 volumes) and amnonium acetate (0.7 M) at −20°C overnight. After centrifugation the pellet was washed twice with ethanol (70%) and air-dried. Elution was done in TE buffer (1X). Three libraries were prepared for each Sterivex and coral sample and pyrosequenced subsequently.
Metagenomes were obtained by pyrosequencing technology using a 454 GS Junior instrument (Roche) (Margulies et al., 2005). Shotgun libraries were generated with 500 ng of whole metagenome samples, sheared into fragments by nebulization. End-repair and adaptor ligation were performed using GS FLX Titanium kit (Roche). Quality control and quantification were performed with Agilent 2100 Bioanalyzer (Agilent Technologies) and TBS 380 Fluorometer (Turner Biosystems), respectively. After the libraries construction, approximately 106 molecules/metagenome were denatured and amplified by emulsion PCR.
Raw sequences were submitted to quality control using PRINSEQ Standalone Lite (version 0.20.4; available at http://sourceforge.net/projects/prinseq/files/). We analyzed 21 metagenomic samples of seawater (n = 12) and corals (n = 9) from 4 days (t1 = 14, t2 = 15, t3 = 18, t4 = 22, of set/2010) along the enrichment process (t4−t1 = 8 days). Annotation was performed by Meta-Genome Rapid Annotation using Subsystems Technology (MG-RAST) server (Meyer et al., 2008) version 3.0, using (SEED) Subsystems Technology and the GenBank database for functional and organismal classifications, respectively. For this purpose, all BLAST queries were conducted with a maximum cutoff E-value 0.00001, a minimum identity of 60%, and a minimum alignment length of 20 measured in aa for protein and bp for RNA databases.
Dinucleotide odds ratio and Karlin distances (δ) between the metagenomes, based on the dinucleotide relative abundances differences (according to Karlin et al., 1997), were calculated using Perl scripts as in Willner et al. (2009). The values in the Karlin matrix were multiplied by a 1000 for easier comparison. Tetranucleotide frequencies were calculated using a Perl script as in Albertsen et al. (2013). The divergence between the observed and expected tetranucleotide frequencies was transferred into z-scores and pairwise comparison of the metagenomic sequences was performed by computing the Pearson's correlation coefficients of the z-scores, both through Python scripts, according to (Teeling et al., 2004). Seawater metagenomes (Sw-14, -15, -18, and -22) were compared among each other, as well as coral metagenomes (Mad14, Mad15, Mad18, MadBle18, Mad22, MadBle22).
The 21 metagenomic libraries were searched for phages using the PHAge Search Tool (PHAST), available at http://phast.wishartlab.com (Zhou et al., 2011). Briefly, pyfasta 0.5.2 (available at http://pypi.python.org/pypi/pyfasta/) was used to split metagenomes into smaller subsets without splitting individual fasta entries, after which PHAST was used to phage search. The tool provides an ensemble of ORF prediction and translation (via GLIMMER; Salzberg et al., 1998), protein identification (via BLASTP; Altschul et al., 1997), phage sequence identification (via BLAST matching to a specific database), tRNA identification (using tRNAscan-SE; Lowe and Eddy, 1997), attachment site recognition (with ARAGORN; Laslett and Canback, 2004) and gene clustering density measurements using density-based spatial clustering of applications with noise (DBSCAN; Ester et al., 1996), and sequence annotation text mining. PHAST's database encloses protein sequences from two sources: the NCBI phage database and the prophage database (Srividhya et al., 2006). Specific keywords (e.g., “protease,” “integrase,” and “tail fiber”) are used for screening. Matched phage or phage-like sequences with E < 0.0001 are saved as hits and their positions tracked for subsequent evaluation for local phage density by DBSCAN. Phage schemes shown in Supplementary Figures 11–18 were generated with PHAST.
The 21 metagenomic libraries were compared to the virulence factor database (VFDB) (Chen et al., 2005) (http://www.mgc.ac.cn/VFs/) using BLASTX (E < 0.0001).
Statistical analysis was conducted using R Version 3.1.3 (Team, 2012) with a suite of packages. The comparison of the correlation coefficients of the z-scores obtained for the tetranucleotide frequencies was visualized through heatmaps using rpy2 and gplots (Gautier, 2008). An exploratory analysis aiming to correlate samples with nutrients' concentrations and metabolisms, according to the level 1 SEED classification, was performed by means of a principal component analysis (PCA) using the rda function of Vegan package (Oksanen et al., 2013). Abundance plots were drawn using the ggplot2 and reshape packages (Wickham, 2007; Wickham and Chang, 2009). The cluster analysis was performed with the APE package (Paradis et al., 2004) using Pearson correlation and ward distance.
The metagenomic data that we generated are available in the MG-RAST v3 server (http://metagenomics.anl.gov/metagenomics.cgi) under the unique identifiers: 4461594.3, 4463932.3, 4463930.3, 4463939.3, 4463927.3, 4461593.3, 4463933.3, 4463931.3, 4463928.3, 4463926.3, 4463925.3, 4468639.3, 4486661.3, 4486665.3, 4486669.3, 4486662.3, 4486667.3, 4486668.3, 4486664.3, 4486663.3, and 4486666.3; and in the Brazilian Marine Biodiversity database (BaMBa) (pmeirelles.18.1).
Ministério do Meio Ambiente (MMA), Instituto Chico Mendes de Conservação da Biodiversidade (ICMBio) Number 10112-2.
In this study 21 metagenomic libraries were obtained from seawater (n = 12) and corals (n = 9) with a total of 446,129 sequences (2.13 × 108 bp) (Table 1). Through annotation-independent analysis, samples Sw14, -15, and -22 were distinguished from -18 (Table 1). Average Karlin distances for pairs of seawater samples Sw14, -15, -18 and -22 defined three categories based on δ ranges. (i) δ < 11 grouped pairs 14-14 and 22-22, indicating high degree of genetic similarity; (ii) 11 < δ < 30 grouped pairs 15–15, 18–18, 14–15, 14–22, and 15–22, indicating intermediate genetic similarity, and (iii) δ> 30 grouped pairs 14–18, 15–18, and 18–22, indicating low genetic similarity. The most dissimilar samples (δ = 50.6) were Sw14 (LLR) and Sw18 (HHR) (Supplementary Figure 5). Similarly, average Karlin distances for coral samples Mad14, -15, -18, and -22 defined three categories. (i) δ < 11 grouped pairs 14–22, 14–15, 15–22, and 22–22; (ii) 11 < δ < 20 grouped pairs 15–15; and (iii) δ> 20 grouped pairs 14–18, 15–18, 18–22, and 18–18. Samples Mad18 were the most dissimilar in comparison with -14, -15, -22 and also among each other (Supplementary Figure 6). The same pattern was revealed by the analysis that resulted from the tetranucleotide frequencies estimated for seawater and coral metgenomes. Samples Sw18 (HHR) were the most dissimilar amongst seawater metagenomes (Supplementary Figure 7A) and metagenomes from HHR bleached corals (MadBle18) were the most dissimilar amongst the holobionts' metagenomes (Supplementary Figure 7B).
To analyze the overall relationships among the most abundant taxa we performed a clustering analysis (Figure 1). The hierarchical clustering of the 21 metagenomes corroborated the binning based in sequence composition (di- and tetranucleotides frequencies). Two major branches split seawater and coral metagenomes. Two seawater branches were defined, with samples 14 (and Sw15-1) representing the LLR. Samples 15 (Sw15-2 and 4), 18 and 22 reflected the turbulence surge (Figure 1). Coral metagenomes were split into healthy and bleached (with only two exceptions: MadBle18-1 grouped into the healthy corals branch and Mad22 into the bleached corals branch). Healthy corals also showed a trend to group according to the enrichment gradient. The healthy corals branch split Mad14 and Mad15-1 from the remainders Mad15-2, Mad18, and Mad22.
Figure 1. Hierarchical clustering of the metagenomes using Order level of the taxonomical identification by MG-Rast. The Pearson correlation and ward distance were used to create the dendrogram. The metagenomes from seawater, healthy and bleached M. decactis are indicated by the colors blue, brown, and red, respectively; and by the sampling day (of September/2010). Exceptions to the clustering of corals according to health status are detached (MadBle18-1 from bleached coral clustered within the healthy branch and Mad22 from healthy coral clustered within the bleached branch). The relative abundance of the 10 most abundant orders is presented at right.
Bacteria was the most abundant domain in all samples with overall average relative abundance of 97.0 ± 1.8% (Mean ± SD), followed by 1.11 ± 0.69% Eukarya (Supplementary Figure 8). Archaeal sequences accounted for ~0.4% of the overall sequences. The main groups were Euryarchaota (~58%) and Thaumarchaeota (21%). Viruses represented ~0.4% of sequences. The most abundant order was Caudovirales (~52%). The overall relative abundance of the most prevalent bacterial phyla were ~61% Proteobacteria, 32% Cyanobacteria followed by 2.4% Bacteroidetes (100% Flavobacteriales), 1.6% Firmicutes, and 1.3% Actinobacteria (Figure 1).
The taxonomic classification of seawater samples revealed a clear difference between LLR and the remainder groups (Sw15, -18, -22). In all samples the dominant groups were Cyanobacteria (C) and Proteobacteria (P). At LLR (Sw14), these groups were equally abundant in seawater (C:P ~1). Along the turbulence gradient, Proteobacteria members increased and Cyanobacteria decreased to a vanishingly small proportion (Sw18, HHR; C:P < < 0.05), with a subsequent recovery (Sw22; C:P > 0.5). The highest proportion of Proteobacteria occurred at HHR (92%) (Figure 2A). At LLR Alphaproteobacteria presented the highest relative abundance (Alpha 28.3% and Gamma 25.1%; ANOVA, P < 0.05), whereas Gammaproteobacteria was dominant in all the following samples (Sw15, -18, and -22: Gamma 29.4% and Alpha 24.8%; P < 0.05) (Supplementary Figure 9). Proteobacterial groups whose relative abundances enhanced during enrichment were Alteromonas, Vibrio and Pseudomonas (Gamma); Ruegeria, Roseobacter, and Candidatus Pelagibacter (Alpha) (Figure 2B). Cyanobacteria and unclassified Alphaproteobacteria (mostly SAR11) decreased correspondingly (Figure 1). Oceanospirillales was absent in the top ten rank at LLR, but appeared in Sw15-4 (2.5%), in HHR (5.6–7.3%), and Sw22 (2.5–2.7%). Archaeal groups also shifted dominance. The most abundant phyla in Sw14-15 was Euryarchaeeota, whereas in Sw18-22 it was Thaumarchaeota (Figure 2C).
Figure 2. Relative abundances of Cyanobacteria, Proteobacteria, and Archaea. (A) Cyanobacteria and Proteobacteria relative abundances in samples Sw14, -15, -18, and -22. The ratio C:P is shown above bars. (B) Relative abundances of GammaProteobacteria (Alteromonas, Vibrio, Pseudomonas) and AlphaProteobacteria (Candidatus pelagibacter, Ruegeria, and Roseobacter) in samples Sw14, -15, -18, and -22. (C) Relative abundances of Euryarchaeota, Thaumarchaeota and Crenarchaeota in pooled samples seawater Sw14–15, Sw18–22; and M. decactis healthy indicated as Healthy coral (14–15) and (18–22); and diseased indicated as Diseased Coral (18–22).
The overall most abundant subsystems were Protein Metabolism (9.8 ± 1.0%), Amino Acids and Derivatives (9.4 ± 0.7%), Carbohydrates (9.4 ± 0.7%), Cofactors, Vitamins, Prosthetic Groups, Pigments (7.2 ± 0.7%), and RNA Metabolism (5.2 ± 0.7%) (Supplementary Figure 10). The most abundant gene per metagenome was the TonB-dependent receptor, of the subsystem Iron Acquisition and Metabolism, in samples Sw18 (HHR) (Supplementary Table 1). A phage protein encoding gene was the most abundant gene in five samples: Sw22-1,2 (recovery) and Sw14-1,2,3 (LLR). Phage detection through specific databases (using PHAST) revealed that the most probable hosts were cyanobacteria for Sw14 and -22 (LLR and recovery, respectively), and Proteobacteria for the remainders (Sw15 and HHR), with only two exceptions (Table 2; Supplementary Figures 11–18). The range of unknown proteins was 17.9–42.4% (Sw18-2 and Sw14-1, respectively).
To investigate the metabolic profile of planktonic dwelling microbes and to correlate differences with the enrichment gradient, we performed a PCA analysis (Figures 3A,B). Clustering of samples Sw14 (LLR) was not explained by nutrients concentrations, contrarily to the remainder samples (Figure 3A). Basic cell functions such as Carbohydrates and Respiration explained the clustering of samples Sw14 (LLR), whether enrichment samples Sw15 and Sw18 (HHR) clustered in response to Virulence, Disease, and Defence; Membrane Transport and Nitrogen Metabolism subsystems (Figure 3B). Samples Sw15 were grouped by the concentration of the nutrients organic phosphorous, ammonia, orthophosphate, and nitrite. The former three showed the highest concentration at Sw15 (Figure 3A, Supplementary Figure 4). HHR samples (Sw18) were coupled by the heterotrophic-characteristic metabolisms, Membrane Transport and Virulence, Disease and Defense. Accordingly, their most abundant gene was the TonB-dependent receptor (Virulence, Disease and Defense). Recovery samples (Sw22) were coupled by the concentration of nitrate (the highest, Figure 3A, Supplementary Figure 4) and Nitrogen Metabolism (Sw22-1; Figure 3B), correspondingly.
Figure 3. Principal component analysis (PCA) diagrams. (A) Diagram generated for the bacterioplankton samples and nutrients in seawater. (B) Diagram generated for the bacterioplankton samples and metabolisms in seawater.
Bacteria was the most abundant identified Domain in all samples with overall relative abundance of 57.15%, followed by Eukarya (39.63%). Archaeal sequences represented 1.92% and viruses 0.1%. Within the domain Eukarya, 33.59% of the sequences were Cnidaria (Supplementary Figure 19A). Main overall archaeal phyla were Euryarchaeota (45.61%), Thaumarchaeota (42.11%), and Crenarchaeota (10.96%). (Figure 2C). There were marked differences in comparison with seawater metagenomes (Figure 1). The most abundant bacterial phyla were Proteobacteria (~48% of the counts), Firmicutes (17%), Actinobacteria (10%), Bacteroidetes (6%), and Cyanobacteria (4%). The most abundant proteobacterial orders were Rhizobiales (16.29%), Rhodobacterales (12.44%); Burkholderiales (10.77%), Pseudomonadales (4.85%), Pasteurellales (4.82%), Alteromonadales (4.68%), Desulfuromonadales (4.38%), Enterobacteriales (4.12%), Myxococcales (4.01%), and Chromatiales (3.46%). Cyanobacteria was mostly represented by Chroococcales (50.23%), Oscillatoriales (24.35%), Nostocales (20.03%), and Prochlorales (5.24%). Interestingly, Rhodobacterales, Rhizobiales, Actinomycetales, Burkholderiales, and Clostridiales were abundant in healthy and diseased M. decactis.
At HHR bleached corals (MadBle18) showed more sequences affiliated to Proteobacteria than the healthy corals (Mad18) (Supplementary Figure 19B). The presence of Pasteurellales among the ten most abundant orders in five out of nine metagenomes (in Mad15, -18, and, -22 with 11–14% of counts) appeared to be a diagnostic feature (Figure 1). Pasteurellales was found in low relative abundance (< 1%) in the remainder corals and in seawater metagenomes. Thaumarchaeota affiliates in all M. decatis samples (Mad14, -15, -18, and -22) approximated Sw18-22 abundance levels (Figure 2C). Cnidaria and Nematoda metagenomic sequences were more abundant in healthy and bleached corals, respectively (Supplementary Figure 8).
The overall most abundant subsystems were Carbohydrates (16.6 ± 2.8%), Amino Acids and Derivatives (14.3 ± 1.2%), Protein Metabolism (9.66 ± 1.6%), Cofactors, Vitamins, Prosthetic groups, Pigments (8.9 ± 1.6%), and DNA Metabolism (6.6 ± 2.4%) (Supplementary Figure 20).
To investigate major differences in metabolisms the corals' metagenomes were pooled (according to Figure 1 and Supplementary Figure 7B) in samples (i) healthy 14–15 (Mad14 and Mad15-1,2), (ii) healthy 18–22 (Mad18 and Mad22), and (iii) diseased 18–22 (MadBle18-1,2 and MadBle22-1,2). Seawater samples were included as reference (Sw14–15, Sw18–22). Prevalence of motility and chemotaxis in seawater compared to corals was the main difference observed between type samples. DNA metabolism prevailed in corals Mad18–22 (healthy and bleached) relative to the remainder samples (Supplementary Figure 20). DNA metabolism was the fourth most represented in corals. The overall most abundant gene (EC 2.1.1.72) in corals was affiliated to this subsystem, which was the most abundant gene in three metagenomes of the Mad18–22 group, and in none of the Mad14–15 group (Supplementary Table 1). The Iron Acquisition subsystem was investigated further by pooling coral samples according to health status in (i) healthy and (ii) diseased. The relative abundance range was lower in healthy corals (5.56–9.09%) than in diseased corals (5.15–16.36%) (P < 0.05). Iron Acquisition in Vibrio was the most abundant function in overall samples, with a relative abundance range of 18.2–83.3% (MadBle22-1 and Mad22, respectively). Healthy corals presented higher relative abundances (60–83%) than diseased corals (18.2–34.4%) for this function. Six functions were represented only in diseased corals: Campylobacter Iron Metabolism (6.3–40.0%); Heme, Hemin Uptake and Utilization Systems in GramPositives (0–20.0%); Siderophore Pyoverdine (0–18.2%), Transport of Iron (0–14.9%); Iron Acquisition in Streptococcus (0–10.3%) and Iron(III) Dicitrate Transport System (0–3.1%). Heme, Hemin Uptake and Utilization Systems in Gram-Negatives was overrepresented in diseased (16.2%) compared to healthy corals (7.3%) (P < 0.05).
The shift from autotroph:heterotroph-balance to offset was further investigated using virulence factors (VFs) as indicators of heterotrophy and risk or threat for corals. In total there were 21,230 significant similarities against the VFDB (Table 3). When normalized to library size, virulence genes were overrepresented in samples Sw 15, -18 (16.0–24.3) and, to a lesser extent in Sw22 (16.6–16.8) (recovery), compared to LLR (Sw14; 12.7–14.2); and in bleached (MadBle; 0.8–8.5) when compared to healthy corals (Mad; 0.6–3.1). We further investigated the iron uptake system, which is a nonspecific virulence system related to competition skills, and thus suitable to reflect the overall heterotrophic community. Iron related virulence genes comprised ~9% (n = 1817) of the total hits to the VFDB. Sw18 samples (HHR) presented the lower percentage of iron related genes relative to the total virulence hits per metagenome (P < 0.05), suggesting that other than iron uptake genes were most representative of the surplus heterotrophs. The VFDB lacks genes related to iron from vibrios (which was overly represented in healthy corals), but encompasses genes related to iron from Haemophilus (Pasteurellales). Among all virulence genes related to iron, ~33% (n = 606) fell into this category. V. cholerae related virulence genes, which confer infective skills, comprised ~6% (n = 1298) of the total virulence hits. The percentage range of these genes relative to total VFs per metagenome in seawater samples Sw14, -15, and -22 was 4.4–6.5% and in Sw18 (HHR) was 6.6–7.7%. Similarly, in healthy corals this range was 0.1–6.9%, and higher in diseased corals: 4.7–8.1% (Table 3). Heterotrophic populations that overgrew in response to turbulence-nutrient pulses were better represented by pathogenic (e.g., V. cholerae-related) than by non-specific (e.g., iron acquisition) VFs.
Table 3. Abundances of virulence factor genes from microbes in the bacterioplankton and M. decactis in SPSPA.
Microbial assemblages during LLR (Sw14) were comparable to those previously described for the surface layers in the western SAO (South Atlantic Gyral - SATL) (Alves Junior et al., 2015) and within the WTRA (Heywood et al., 2006; Schattenhofer et al., 2009), where the dominant groups (= 50%) detected were also Prochlorales and unclassified Alphaproteobacteria or SAR11 and related. Following LLR the microbial assemblages observed increasingly differed from previous studies focusing the surface layers of those most neighboring locations (Heywood et al., 2006; Schattenhofer et al., 2009; Swan et al., 2011). Alteromonadales appeared as the second most abundant group after Prochlorales, prevailing over unclassified Alphaproteobacteria, and Vibrionales emerged as a new group with >5% relative abundance (Sw15). Comparable Alteromonadales relative abundances, combined with lower abundances of unclassified Alphaproteobacteria (SAR11) were previously reported for the sub-superficial chlorophyll maximum (SCM) layer at higher depths (48–82 m) in the SAO, where, instead of Vibrionales, Pseudomonadales, and Mamiellales emerged as differing groups compared to the surface layers (Alves Junior et al., 2015). At HHR (Sw18), other Gammaproteobacteria appeared with > 5% relative abundance, i.e., Pseudomonadales and Oceanospirillales, whereas Thaumarchaota reached Euryarchaeota relative abundance levels. Pseudomonadales, Oceanospirillales, and Thaumarchaeota characterized deep waters (236–1200 m) in the SAO, and in which water masses Prochlorales was not amongst the 10 most abundant orders (AlvesJr-14). (Schattenhofer et al., 2009) reported a Gammaproteobacteria bloom in the North Atlantic Drift Province (NADR), with a maximum relative abundance of >50% of all picoplankton in surface waters, compared to the average values of 2–5% for all the other Atlantic provinces. Only a minor fraction was identified (Alteromonas/Colwellia and Pseudoalteromonas: 2–5%; Vibrio: 1%, and Oceanospirillum: 4%). The Gammaproteobacteria bloom was attributed to the end of the spring phytoplankton bloom, indicated by declining chlorophyll values. Massive growth of Bacteroidetes was concomitant and deep water Archaea presence at surface was not observed. Gammaproteobacteria have the potential to respond to sudden nutrient pulses released from phytoplankton (Cottrell and Kirchman, 2000). Members of Alteromonas, Pseudoalteromonas, and Vibrio are well known to rapidly respond to excess nutrient supply (Bano and Hollibaugh, 2002; Beardsley et al., 2003; Allers et al., 2007, 2008). Thaumarchaeota are typically more abundant at depths of ≥100 m, as oposed to Euryarchaeota, which is known for decreasing abundance below 100 m (Delong, 1992; Zhang et al., 2009; Santoro et al., 2010; Tseng et al., 2015). In SPSPA, concomitant with Thaumarchaeota increase, ammonia levels decreased, possibly due to its ammonia-oxidizing ability (Francis et al., 2005); and Flavobacteriales (Bacteroidetes) relative abundance decreased. Next (Sw22), the five most abundant groups at LLR recovered relative abundances almost to LLR (Sw14) levels (Prochlorococcus, unclassified Alphaproteobacteria—SAR11, Rhodobacterales, Rhizobiales, and Chroococcales), but Alteromonadales, Pseudomonadales, Vibrionales, and Oceanospirillales remained amongst the 10 most abundant groups, as well as Thaumarchaeota remained abundant, which is a distinctive assemblage for those geographical coordinates (Schattenhofer et al., 2009; Swan et al., 2011; Alves Junior et al., 2015). Shifts in planktonic assemblages at the mesophotic zone in SPSPA were possibly driven by the turbulence surge, meaning that microbes from progressively deeper layers could hitchhike with the vertical flux along the surge. The upwelling is also supported by the enrichment and by the wide variation of water temperatures registered for the sampling depth (Supplementary Figure 4).
Although sequence similarities to genes do not represent levels of gene expression, metagenomes have been shown to be strong predictors of the biogeochemical conditions driving the microbial community (Dinsdale et al., 2008). According to the lines of evidence garnered the microbiome of the mesophotic waters in SPSPA undergoes cyclic transient shifts in relation to turbulence-nutrients regimes. A microbial succession resulting from the interplay between physical and chemical factors is a plausible scenario. Two extreme turbulence-nutrient regimes can be clearly distinguished and alternate with intermediate conditions determining microbial assemblages: (i) When turbulence is low (LLR) at least 50% of the microbiome is composed of Prochlorococcus, followed by unclassified Alphaproteobacteria (SAR11 and related), which are small sized cells, highly adapted to oligotrophic conditions and starvation. Rhodobacterlaes, Rhizobiales, and Chroococcales are typical. In this environment phage genes are the most abundant in seawater, mostly from Prochlorococcus and Synechococcus, following the hosts' abundances. The viral shunt is probably less active toward relatively scarce cells. The proportion of unknown genes is the highest; (ii) Episodic surges promote vertical mixing from the immediate lower water mass to the mixed layer. Waves also wash guano from the cays flushing phosphates and ammonium into the inlet. Heterotrophs (Alteromonadales, Vibrionales) respond quickly and surpass autotrophs, motility, and chemotaxis related genes stand out; (iii) Ongoing eddies and intensified high-energy waves promote entrainment of deep water organisms such as Thaumarchaeota and nutrients (nitrite, nitrate) (HHR). Eventual cloudiness, winds and rain cope with turbulence, irradiance is intermittent and turbidity is enhanced. Heterotrophy predominates with dominance of Alteromonadales, Pseudomonadales, and Oceanospirillales. Gammaproteobacterial groups approximate 50% of the microbial assemblage, resembling the end of the spring phytoplankton bloom in higher latitudes (e.g., NADR). The gene pool in surface waters reflects the shift with membrane transport and virulence-related genes (e.g., TonB-dependent receptor, V. cholerae virulence genes) surpassing cyanobacterial phages and basic metabolisms genes. Phages targeting heterotrophs are active. The proportion of unknown genes is the lowest; (iv) Turbulence alleviates (e.g., after moon changes toward new). Larger cell sized heterotrophs begin to decline as viral lysis and predation by grazers overrides growth, which is constrained by the paucity of limiting nutrients (e.g., phosphorus). The microbial loop is most prominent at this stage. Autotrophs respond to irradiance and retake growth (if rain, wind, and cloudiness mitigate this response is accelerated). Nitrogen metabolism is intensive. A reversal to autotrophy:heterotrophy equilibrium is triggered (Figure 4). The short-lived but recurrent turbulence-nutrient pulses might be responsible for structuring the marine ecosystem in a bottom-up manner in SPSPA. These pulses might be indispensable to warrant the energy and carbon flow to the higher trophic levels concurring to the observed pelagic fishes biomass around the barren islets (Luiz and Edwards, 2011). On a stable LLR the growth of phytoplankton is largely supported by regenerated nutrients, so only a small proportion of primary production is available to higher trophic levels or for export to the deep sea (Cullen et al., 2002; Karl, 2014). Turbulence is physically forcing the co-ocurrence of nutrients and light in SPSPA, on the other hand, the fact that nutrient resupply is short-lived might concur to retain the local mesotrophic condition. Bacterioplankton shifts were shown to be transient, following the cyclic nutrient-turbulence pulses and other physical parameters (rain, cloudiness, winds, turbidity).
Figure 4. Cartoon model of the main processes in SPSPA Cyclic turbulence-nutrient pulses determine transient shifts in the bacterioplankton. Circular central blue arrow indicates the cyclic nature of the events. Left panel: during LLR calm tides, weak winds, absence of clouds, and clear waters correlate with lower levels (downwards red arrows) of nitrogen (N) and phosphorous (P), and equivalence between autotrophy and heterotropy, represented by the ratio cyanobacteria:heterotrophs (C:H) equalizing 1. Cyanobacterial phages (green poligons) reflect the abundance of the hosts. Right panel: during HHR harsh conditions are determined by violent turbulence resulting in upwelling and enrichment, represented by high levels (upwards red arrows) of N and P, and a shift to heterotrophy dominance, represented by a low ratio cyanobacteria:heterotrophs (C:H < < 1). Intense winds, cloudiness and rain, that washes the rocks covered with guano, contribute to enrichment and the shift to heterotrophy. Proteobacterial phages (red poligons) reflect the abundance of the hosts as well as Iron acquisition (red circle) and virulence factor (red crosses) genes. Vibrios abundance (blue elipses) reflect seawater parameters. Episodic surges frequently correlate with full moon. When turbulence pulses mitigate and weather assuages a recovery takes place in seawater, both in terms of nutrients concentrations and microbial assemblages (e.g., after moon changes toward new).
Some bacterial taxa prevalent in bleached corals (Rhodobacterales, Rhizobiales, and Clostridiales) have been previously associated with opportunistic diseases (Frias-Lopez et al., 2002; Rosenberg et al., 2007; Sekar et al., 2008; Sunagawa et al., 2009; Mouchka et al., 2010). On the other hand, a study of the corals microbiome, aiming at distinguishing the core, the symbiotic and the whole community microbiome, suggested that Rhodobacterales pertains to the latter. Conversely, Rhizobiales members were suggested to belong to the symbiotic coral microbiome. Actinomycetales and Burkholderiales (both also prevalent in all coral samples) were characterized as part of the coral core microbiome (D Ainsworth et al., 2015). Pasteurellales was one of the most abundant bacteria in the coral metagenomes, contrasting to its dwindling relative abundance in seawater. Pasteurellales members can cause disease in a wide range of domestic and wild animals (Wilson and Ho, 2013). They are commonly found in fish tissues (Birkbeck et al., 2002). Reef fishes (Chaetodontidae) have been characterized as major vectors of coral diseases (Raymundo et al., 2009) and damselfish (Stegastes spp.) was shown to increase the prevalence of the coral Black Band Disease (BBD) (Casey et al., 2014). It is plausible that Pasteurellales are frequently transmitted to corals through fishes, possibly by fish bites, since M. decactis is frequently predated by S. sanctipauli, H. radiatus and other fishes in SPSPA (Supplementary Figure 3). This hypothesis explains the uneven distribution of Pasteurellales between healthy and diseased corals, as well as the disconnection to seawater parameters. Bleached corals were distinguished by enhanced Iron Acquisition metabolism. Six functions within this subsystem were represented only in bleached corals. HHR bleached corals samples (MadBle18) were the most dissimilar in terms of sequence composition (di- and tetranucletides frequencies), higher counts of Proteobacteria (including Vibrionales), and higher relative abundance of hits to the VFDB, including V. cholerae-related VFs. This dissimilarity, including the HHR healthy coral (Mad18), indicates that the healthy coral holobiont might be less sensitive to transient seawater-related perturbations than the diseased animals. The distinguishing characteristics of HHR bleached corals agree with the bacterioplankton and seawater features during HHR, reported both in the present and former study (Supplementary Figure 4; Moreira et al., 2014): sequence composition, higher relative abundance of motility and chemotaxis, and of membrane transport and virulence genes (e.g., Ton-B dependent receptor of the Iron Acquisition metabolism, V. cholerae-related VFs), higher vibrio counts and nutrients in seawater. Taken together, the datasets suggest coupling between the benthic and pelagic compartments, as previously reported (Chimetto Tonon et al., 2015).
Owing to the remote nature of this site, we do not have complete data sets. Resampling will be needed to strengthen the link between turbulence-upwelling and the shifts in microbial assemblages.
This work analyzed shifts in microbial composition related to physical forcings (turbulence-upwelling and storms) in SPSPA. LLR is characterized by the equilibrium between autotrophy-heterotrophy and microbial assemblages that resemble those of surface tropical waters previously characterized in the SAO. At HHR microbial communities shift to heterotrophic and deep-sea characteristic organisms (Thaumarchaota). HHR diseased corals are distinguished by sequence composition and enhanced VFs hits, suggesting some level of coupling between planktonic and coral microbial communities.
The authors declare that the research was conducted in the absence of any commercial or financial relationships that could be construed as a potential conflict of interest.
This work was supported by CNPq, CAPES and FAPERJ. Comissão Interministerial para os Recursos do Mar partially supported the expedition. The authors are grateful to the Brazilian Navy for maintenance of the Scientific Station and training; to Ericka Coni for joining the expedition and participating in sampling; to the Transmar III crew, Douglas Abrantes and Camilo Ferreira for general logistical support, and to Prof. Rob Edwards (San Diego State University) for the valuable ideas and helpful discussion.
The Supplementary Material for this article can be found online at: http://journal.frontiersin.org/article/10.3389/fmicb.2015.01038
EUC, Atlantic Equatorial Undercurrent; BLAST, Basic Local Alignment Search Tool; chla, chlorophyll a; CFU, colony forming unit; DMSP, dimethylsulfoniopropionate; HOT, Hawaii Ocean Time-series; HHR, high turbulence-high nutrients regime; LLR, low turbulence-low nutrients regime; MAR, Mid-Atlantic Ridge; NCBI, National Center for Biotechnology Information; NADR, North Atlantic Drift Province; PHAST, PHAge Search Tool; SeaWiFS, Sea-viewing Wide Field-of-view Sensor; SATL, South Atlantic Gyral; SAO, South Atlantic Ocean; SPSPA, St Peter and St Paul Archipelago; FZ, St Paul Fault Zone; SEC, South Equatorial Current; SCM, sub-superficial chlorophyll maximum; VFs, virulence factors; VFDB, Virulence Factor Data Base; WTRA, Western Tropical Atlantic Province; WORMS, World Register of marine Species.
Alves Junior N Meirelles, P. M., de Oliveira Santos, E., Dutilh, B., Silva, G. G., Paranhos, R., et al. (2015). Microbial community diversity and physical-chemical features of the Southwestern Atlantic Ocean. Arch. Microbiol. 197, 165–179. doi: 10.1007/s00203-014-1035-6
Albertsen, M., Hugenholtz, P., Skarshewski, A., Nielsen, K. R. L., Tyson, G. W., and Nielsen, P. H. (2013). Genome sequences of rare, uncultured bacteria obtained by differential coverage binning of multiple metagenomes. Nat. Biotechnol. 31, 533–538. doi: 10.1038/nbt.2579
Allers, E., Gómez-Consarnau, L., Pinhassi, J., Gasol, J. M., Simek, K., and Pernthaler, J. (2007). Response of Alteromonadaceae and Rhodobacteriaceae to glucose and phosphorus manipulation in marine mesocosms. Environ. Microbiol. 9, 2417–2429. doi: 10.1111/j.1462-2920.2007.01360.x
Allers, E., Niesner, C., Wild, C., and Pernthaler, J. (2008). Microbes enriched in seawater after addition of coral mucus. Appl. Environ. Microbiol. 74, 3274–3278. doi: 10.1128/AEM.01870-07
Altschul, S. F., Madden, T. L., Schäffer, A. A., Zhang, J., Zhang, Z., Miller, W., et al. (1997). Gapped BLAST and PSI-BLAST: a new generation of protein database search programs. Nucleic Acids Res. 25, 3389–3402. doi: 10.1093/nar/25.17.3389
Bano, N., and Hollibaugh, J. T. (2002). Phylogenetic composition of bacterioplankton assemblages from the Arctic Ocean. Appl. Environ. Microbiol. 68, 505–518. doi: 10.1128/AEM.68.2.505-518.2002
Beardsley, C., Pernthaler, J., Wosniok, W., and Amann, R. (2003). Are readily culturable bacteria in coastal North Sea waters suppressed by selective grazing mortality? Appl. Environ. Microbiol. 69, 2624–2630. doi: 10.1128/AEM.69.5.2624-2630.2003
Birkbeck, T., Laidler, L., Grant, A., and Cox, D. (2002). Pasteurella skyensis sp. nov., isolated from Atlantic salmon (Salmo salar L.). Int. J. Syst. Evol. Microbiol. 52, 699–704. doi: 10.1099/00207713-52-3-699
Campos, T. F. C., Bezerra, F. H. R., Srivastava, N. K., Vieira, M. M., and Vita-Finzi, C. (2010). Holocene tectonic uplift of the St Peter and St Paul Rocks (Equatorial Atlantic) consistent with emplacement by extrusion. Mar. Geol. 271, 177–186. doi: 10.1016/j.margeo.2010.02.013
Casey, J. M., Ainsworth, T. D., Choat, J. H., and Connolly, S. R. (2014). Farming behaviour of reef fishes increases the prevalence of coral disease associated microbes and black band disease. Proc. Biol. Sci. 281, 20141032. doi: 10.1098/rspb.2014.1032
Chen, L., Yang, J., Yu, J., Yao, Z., Sun, L., Shen, Y., et al. (2005). VFDB: a reference database for bacterial virulence factors. Nucleic Acids Res. 33, D325–D328. doi: 10.1093/nar/gki008
Chimetto Tonon, L. A., Silva, B. S. O., Moreira, A. P. B., Valle, C. Jr. Nelson, A., Cavalcanti, G., et al. (2015). Diversity and ecological structure of vibrios in benthic and pelagic habitats along a latitudinal gradient in the Southwest Atlantic Ocean. PeerJ 3:e741. doi: 10.7717/peerj.741
Costello, E. K., Lauber, C. L., Hamady, M., Fierer, N., Gordon, J. I., and Knight, R. (2009). Bacterial community variation in human body habitats across space and time. Science 326, 1694–1697. doi: 10.1126/science.1177486
Cottrell, M. T., and Kirchman, D. L. (2000). Natural assemblages of marine proteobacteria and members of the Cytophaga-Flavobacter cluster consuming low-and high-molecular-weight dissolved organic matter. Appl. Environ. Microbiol. 66, 1692–1697. doi: 10.1128/AEM.66.4.1692-1697.2000
Crespo, T. M., Da Gama Bahia, R., Maneveldt, G. W., and Amado Filho, G. M. (2014). Floristic composition of crustose coralline algae from the St. Peter and St. Paul Archipelago, a summit of the Mid-Atlantic Ridge. Phytotaxa 190, 17–37. doi: 10.11646/phytotaxa.190.1.4
Cullen, J. J., Franks, P. J., Karl, D. M., and Longhurst, A. (2002). “Physical influences on marine ecosystem dynamics”, in The Sea eds A. R. Robinson, J. J. Mccarthy, and B. J. Rothschild (New York, NY: John Wiley & Sons Inc.), 297–336.
D Ainsworth, T., Krause, L., Bridge, T., Torda, G., Raina, J.-B., Zakrzewski, M., et al. (2015). The coral core microbiome identifies rare bacterial taxa as ubiquitous endosymbionts. ISME J. 9, 2261–2274. doi: 10.1038/ismej.2015.39
Delong, E. F. (1992). Archaea in coastal marine environments. Proc. Natl. Acad. Sci. U.S.A. 89, 5685–5689. doi: 10.1073/pnas.89.12.5685
Dinsdale, E. A., Pantos, O., Smriga, S., Edwards, R. A., Angly, F., Wegley, L., et al. (2008). Microbial ecology of four coral atolls in the Northern Line Islands. PLoS ONE 3:e1584. doi: 10.1371/journal.pone.0001584
Edwards, A., and Lubbock, R. (1983). The ecology of Saint Paul's Rocks (Equatorial Atlantic). J. Zool. 200, 51–69. doi: 10.1111/j.1469-7998.1983.tb06108.x
Ester, M., Kriegel, H.-P., Sander, J. R., and Xu, X. (1996). “A density-based algorithm for discovering clusters in large spatial databases with noise”, in KDD Proceedings, Vol. 96 (Menlo Park, CA: AAAI Press), 226–231.
Falkowski, P. G., Fenchel, T., and Delong, E. F. (2008). The microbial engines that drive Earth's biogeochemical cycles. Science 320, 1034–1039. doi: 10.1126/science.1153213
Fierer, N., and Jackson, R. B. (2006). The diversity and biogeography of soil bacterial communities. Proc. Natl. Acad. Sci. U.S.A. 103, 626–631. doi: 10.1073/pnas.0507535103
Francis, C. A., Roberts, K. J., Beman, J. M., Santoro, A. E., and Oakley, B. B. (2005). Ubiquity and diversity of ammonia-oxidizing archaea in water columns and sediments of the ocean. Proc. Natl. Acad. Sci. U.S.A. 102, 14683–14688. doi: 10.1073/pnas.0506625102
Frias-Lopez, J., Zerkle, A. L., Bonheyo, G. T., and Fouke, B. W. (2002). Partitioning of bacterial communities between seawater and healthy, black band diseased, and dead coral surfaces. Appl. Environ. Microbiol. 68, 2214–2228. doi: 10.1128/AEM.68.5.2214-2228.2002
Fuhrman, J. A. (2009). Microbial community structure and its functional implications. Nature 459, 193–199. doi: 10.1038/nature08058
Gagnon, K., Rothäusler, E., SyrjäNen, A., Yli-Renko, M., and Jormalainen, V. (2013). Seabird guano fertilizes Baltic Sea littoral food webs. PLoS ONE 8:e61284. doi: 10.1371/journal.pone.0061284
Garrett, C., and Munk, W. (1972). Oceanic mixing by breaking internal waves. Deep Sea Res. Oceanogr. Abstr. 19, 823–832. doi: 10.1016/0011-7471(72)90001-0
Gautier, L. (2008). rpy2: A Simple and Efficient Access to R from Python. Available online at: http://rpy.sourceforge.net/rpy2.html
Gregg, M., D'Asaro, E., Shay, T., and Larson, N. (1986). Observations of persistent mixing and near-inertial internal waves. J. Phys. Oceanogr. 16, 856–885.
Hayward, T. L. (1987). The nutrient distribution and primary production in the central North Pacific. Deep-Sea Res. II 34, 1593–1627. doi: 10.1016/0198-0149(87)90111-7
Hayward, T. L. (1991). Primary production in the North Pacific Central Gyre: a controversy with important implications. Trends Ecol. Evol. 6, 281–284. doi: 10.1016/0169-5347(91)90005-I
Heywood, J., Zubkov, M., Tarran, G., Fuchs, B., and Holligan, P. (2006). Prokaryoplankton standing stocks in oligotrophic gyre and equatorial provinces of the Atlantic Ocean: evaluation of inter-annual variability. Deep Sea Res. II. 53, 1530–1547. doi: 10.1016/j.dsr2.2006.05.005
Karl, D. M. (1999). A sea of change: biogeochemical variability in the North Pacific Subtropical Gyre. Ecosystems 2, 181–214. doi: 10.1007/s100219900068
Karl, D. M. (2002). Nutrient dynamics in the deep blue sea. Trends Microbiol. 10, 410–418. doi: 10.1016/S0966-842X(02)02430-7
Karl, D. M. (2014). Microbially mediated transformations of phosphorus in the sea: new views of an old cycle. Ann. Rev. Mar. Sci. 6, 279–337. doi: 10.1146/annurev-marine-010213-135046
Karlin, S., Mrázek, J., and Campbell, A. M. (1997). Compositional biases of bacterial genomes and evolutionary implications. J. Bacteriol. 179, 3899–3913.
Laslett, D., and Canback, B. (2004). ARAGORN, a program to detect tRNA genes and tmRNA genes in nucleotide sequences. Nucleic Acids Res. 32, 11–16. doi: 10.1093/nar/gkh152
Longhurst, A., Sathyendranath, S., Platt, T., and Caverhill, C. (1995). An estimate of global primary production in the ocean from satellite radiometer data. J. Plankton Res. 17, 1245–1271. doi: 10.1093/plankt/17.6.1245
Lowe, T. M., and Eddy, S. R. (1997). tRNAscan-SE: a program for improved detection of transfer RNA genes in genomic sequence. Nucleic Acids Res. 25, 0955–0964. doi: 10.1093/nar/25.5.0955
Luiz, O. J., and Edwards, A. J. (2011). Extinction of a shark population in the Archipelago of Saint Paul's Rocks (equatorial Atlantic) inferred from the historical record. Biol. Conserv. 144, 2873–2881. doi: 10.1016/j.biocon.2011.08.004
Lyman, T. (1859). On a new species of coral (Astraea decactis). Proc. Boston Soc. Nat. Hist. 6, 260–263.
Macedo, S. J., Montes, M. J. F., and Costa, K. M. P. (2009). “Hidrologia”, in O Arquipélago de São Pedro e São Paulo: 10 anos de Estação Científica, ed F. H. V. Hazin (Brasília: SECIRM), 348.
Macedo-Soares, L., Brandão, M., Koettker, A., Menezes, B., Stumpf, L., and Freire, A. (2009). O Zooplâncton no Arquipélago de São Pedro e São Paulo. Ilhas Oceânicas Brasileiras: da Pesquisa ao Manejo, Vol 2. Brasília: Ministério do Meio Ambiente, IBAMA.
Margulies, M., Egholm, M., Altman, W. E., Attiya, S., Bader, J. S., Bemben, L. A., et al. (2005). Genome sequencing in microfabricated high-density picolitre reactors. Nature 437, 376–380. doi: 10.1038/nature03959
Meyer, F., Paarmann, D., D'souza, M., Olson, R., Glass, E., Kubal, M., et al. (2008). The metagenomics RAST serverĆ a public resource for the automatic phylogenetic and functional analysis of metagenomes. BMC Bioinformatics 9:386. doi: 10.1186/1471-2105-9-386
Migotto, A. (1997). “Anthozoan bleaching on the southeastern coast of Brazil in the summer of 1994”, in 6th International Conference of Coelenterate Biology, (Leeuwenhorst), 329–335.
Moreira, A. P. B., Tonon, L. A. C., Pereira Cdo, V., Alves, N. Jr. Amado-Filho, G. M., et al. (2014). Culturable heterotrophic bacteria associated with healthy and bleached scleractinian madracis decactis and the fireworm hermodice carunculata from the remote St. Peter and St. Paul Archipelago, Brazil. Curr. Microbiol. 68, 38–46. doi: 10.1007/s00284-013-0435-1
Mouchka, M. E., Hewson, I., and Harvell, C. D. (2010). Coral-associated bacterial assemblages: current knowledge and the potential for climate-driven impacts. Integr. Comp. Biol. 50, 662–674. doi: 10.1093/icb/icq061
Neves, E., and Johnsson, R. (2009). Taxonomic revision of the southwestern Atlantic Madracis and the description of Madracis fragilis n. sp. (Scleractinia: Pocilloporidae), a new coral species from Brazil. Sci. Mar. 73, 739–746. doi: 10.3989/scimar.2009.73n4735
Oksanen, J., Blanchet, F. G., Kindt, R., Legendre, P., Minchin, P. R., O'Hara, R., et al. (2013). Package ‘Vegan’. Community Ecology Package, Version 2.
Paradis, E., Claude, J., and Strimmer, K. (2004). APE: analyses of phylogenetics and evolution in R language. Bioinformatics 20, 289–290. doi: 10.1093/bioinformatics/btg412
Philander, S. (1986). Unusual conditions in the tropical Atlantic Ocean in 1984. Nature 322, 236–238. doi: 10.1038/322236a0
Platt, T., Harrison, W. G., Lewis, M. R., Li, W. K., Sathyendranath, S., Smith, R. E., et al. (1989). Biological production of the oceans: the case for a consensus. Mar. Ecol. Prog. Ser. 52, 77–88. doi: 10.3354/meps052077
Raymundo, L. J., Halford, A. R., Maypa, A. P., and Kerr, A. M. (2009). Functionally diverse reef-fish communities ameliorate coral disease. Proc. Natl. Acad. Sci. U.S.A. 106, 17067–17070. doi: 10.1073/pnas.0900365106
Robertson, D. R. (2001). Population maintenance among tropical reef fishes: inferences from small-island endemics. Proc. Natl. Acad. Sci. U.S.A. 98, 5667–5670. doi: 10.1073/pnas.091367798
Rosenberg, E., Koren, O., Reshef, L., Efrony, R., and Zilber-Rosenberg, I. (2007). The role of microorganisms in coral health, disease and evolution. Nat. Rev. Microbiol. 5, 355–362. doi: 10.1038/nrmicro1635
Rusch, D. B., Halpern, A. L., Sutton, G., Heidelberg, K. B., Williamson, S., Yooseph, S., et al. (2007). The Sorcerer II global ocean sampling expedition: northwest Atlantic through eastern tropical Pacific. PLoS Biol. 5:e77. doi: 10.1371/journal.pbio.0050077
Salzberg, S. L., Delcher, A. L., Kasif, S., and White, O. (1998). Microbial gene identification using interpolated Markov models. Nucleic Acids Res. 26, 544–548. doi: 10.1093/nar/26.2.544
Santoro, A. E., Casciotti, K. L., and Francis, C. A. (2010). Activity, abundance and diversity of nitrifying archaea and bacteria in the central California Current. Environ. Microbiol. 12, 1989–2006. doi: 10.1111/j.1462-2920.2010.02205.x
Schattenhofer, M., Fuchs, B. M., Amann, R., Zubkov, M. V., Tarran, G. A., and Pernthaler, J. (2009). Latitudinal distribution of prokaryotic picoplankton populations in the Atlantic Ocean. Environ. Microbiol. 11, 2078–2093. doi: 10.1111/j.1462-2920.2009.01929.x
Sekar, R., Kaczmarsky, L. T., and Richardson, L. L. (2008). Microbial community composition of black band disease on the coral host Siderastrea siderea from three regions of the wider Caribbean. Mar. Ecol. Prog. Ser. 362, 85–98. doi: 10.3354/meps07496
Sherman, J. T., and Pinkel, R. (1991). Estimates of the vertical wavenumber-frequency spectra of vertical shear and strain. J. Phys. Oceanogr. 21, 292–303.
Shushkina, E., Vinogradov, M., Lebedeva, L., and Anokhina, L. (1997). Productivity characteristics of epipelagic communities of the World's Oceans. Oceanol. Russ. Acad. Sci. 37, 346–353.
Srividhya, K., Rao, G. V., Raghavenderan, L., Mehta, P., Prilusky, J., Manicka, S., et al. (2006). “Database and comparative identification of prophages”, in Intelligent Control and Automation, eds D.-S. Huang, K. Li, and G. W. Irwin (Berlin; Heidelberg: Springer), 863–868.
Sunagawa, S., Desantis, T. Z., Piceno, Y. M., Brodie, E. L., Desalvo, M. K., Voolstra, C. R., et al. (2009). Bacterial diversity and white plague disease-associated community changes in the caribbean coral Montastraea faveolata. ISME J. 3, 512–521. doi: 10.1038/ismej.2008.131
Swan, B. K., Martinez-Garcia, M., Preston, C. M., Sczyrba, A., Woyke, T., Lamy, D., et al. (2011). Potential for chemolithoautotrophy among ubiquitous bacteria lineages in the dark ocean. Science 333, 1296–1300. doi: 10.1126/science.1203690
Team, R. D. C. (2012). R: A language and environment for statistical computing. Vienna: R Foundation for Statistical Computing.
Teeling, H., Waldmann, J., Lombardot, T., Bauer, M., and Glöckner, F. O. (2004). TETRA: a web-service and a stand-alone program for the analysis and comparison of tetranucleotide usage patterns in DNA sequences. BMC Bioinformatics 5:163. doi: 10.1186/1471-2105-5-163
Trindade-Silva, A. E., Rua, C., Silva, G. G., Dutilh, B. E., Moreira, A. P. B., Edwards, R. A., et al. (2012). Taxonomic and functional microbial signatures of the endemic marine sponge Arenosclera brasiliensis. PLoS ONE 7:e39905. doi: 10.1371/journal.pone.0039905
Tseng, C.-H., Chiang, P.-W., Lai, H.-C., Shiah, F.-K., Hsu, T.-C., Chen, Y.-L., et al. (2015). Prokaryotic assemblages and metagenomes in pelagic zones of the South China Sea. BMC Genomics 16:219. doi: 10.1186/s12864-015-1434-3
Wickham, H. (2007). Reshaping data with the reshape package. J. Stat. Softw. 21, 1–20. Available online at: http://www.jstatsoft.org/v21/i12/
Wickham, H., and Chang, W. (2009). ggplot2: An implementation of the Grammar of Graphics. R package version 0.8. 3.
Willner, D., Thurber, R. V., and Rohwer, F. (2009). Metagenomic signatures of 86 microbial and viral metagenomes. Environ. Microbiol. 11, 1752–1766. doi: 10.1111/j.1462-2920.2009.01901.x
Wilson, B. A., and Ho, M. (2013). Pasteurella multocida: from zoonosis to cellular microbiology. Clin. Microbiol. Rev. 26, 631–655. doi: 10.1128/CMR.00024-13
Zhang, Y., Sintes, E., Chen, J., Zhang, Y., Dai, M., Jiao, N., et al. (2009). Role of mesoscale cyclonic eddies in the distribution and activity of Archaea and Bacteria in the South China Sea. Aquat. Microb. Ecol. 56, 65. doi: 10.3354/ame01324
Keywords: metagenomics, Atlantic Ocean, oceanic islands, Scleractinia, Madracis decactis
Citation: Moreira APB, Meirelles PM, Santos EO, Amado-Filho GM, Francini-Filho RB, Thompson CC and Thompson FL (2015) Turbulence-driven shifts in holobionts and planktonic microbial assemblages in St. Peter and St. Paul Archipelago, Mid-Atlantic Ridge, Brazil. Front. Microbiol. 6:1038. doi: 10.3389/fmicb.2015.01038
Received: 01 June 2015; Accepted: 11 September 2015;
Published: 02 October 2015.
Edited by:
Maurizio Labbate, University of Technology Sydney, AustraliaReviewed by:
Matthew Schrenk, Michigan State University, USACopyright © 2015 Moreira, Meirelles, Santos, Amado-Filho, Francini-Filho, Thompson and Thompson. This is an open-access article distributed under the terms of the Creative Commons Attribution License (CC BY). The use, distribution or reproduction in other forums is permitted, provided the original author(s) or licensor are credited and that the original publication in this journal is cited, in accordance with accepted academic practice. No use, distribution or reproduction is permitted which does not comply with these terms.
*Correspondence: Fabiano L. Thompson, CCS, BIOMAR, Laboratório de Microbiologia, Institute of Biology, Cidade Universitária, Av. Carlos Chagas F°. S/N, Bloco A (Anexo) A3, sl 102, Rio de Janeiro, RJ, CEP 21941-599, Brazil,ZmFiaWFub3Rob21wc29uMUBnbWFpbC5jb20=
Disclaimer: All claims expressed in this article are solely those of the authors and do not necessarily represent those of their affiliated organizations, or those of the publisher, the editors and the reviewers. Any product that may be evaluated in this article or claim that may be made by its manufacturer is not guaranteed or endorsed by the publisher.
Research integrity at Frontiers
Learn more about the work of our research integrity team to safeguard the quality of each article we publish.