Detecting rare gene transfer events in bacterial populations
- 1 Department of Ecology and Evolutionary Biology, Yale University, New Haven, CT, USA
- 2 GenØk-Centre for Biosafety, The Science Park, Tromsø, Norway
- 3 Department of Pharmacy, Faculty of Health Sciences, University of Tromsø, Tromsø, Norway
Experimental approaches to identify horizontal gene transfer (HGT) events of non-mobile DNA in bacteria have typically relied on detection of the initial transformants or their immediate offspring. However, rare HGT events occurring in large and structured populations are unlikely to be detected in a short time frame. Population genetic modeling of the growth dynamics of bacterial genotypes is therefore necessary to account for natural selection and genetic drift during the time lag and to predict realistic time frames for detection with a given sampling design. Here we draw on statistical approaches to population genetic theory to construct a cohesive probabilistic framework for investigation of HGT of exogenous DNA into bacteria. In particular, the stochastic timing of rare HGT events is accounted for. Integrating over all possible event timings, we provide an equation for the probability of detection, given that HGT actually occurred. Furthermore, we identify the key variables determining the probability of detecting HGT events in four different case scenarios that are representative of bacterial populations in various environments. Our theoretical analysis provides insight into the temporal aspects of dissemination of genetic material, such as antibiotic resistance genes or transgenes present in genetically modified organisms. Due to the long time scales involved and the exponential growth of bacteria with differing fitness, quantitative analyses incorporating bacterial generation time, and levels of selection, such as the one presented here, will be a necessary component of any future experimental design and analysis of HGT as it occurs in natural settings.
Introduction
Bacteria in natural populations are known to import and integrate exogenous genetic material of diverse, often unidentified, origins (Eisen, 2000; Ochman et al., 2000; Lawrence, 2002; Nakamura et al., 2004; Didelot and Maiden, 2010). Bacterial genomes can be exposed not only to the multitude of sources of exogenous DNA present in their natural environments (Levy-Booth et al., 2007; Nielsen et al., 2007; Pontiroli et al., 2007; Pietramellara et al., 2009; Rizzi et al., 2012), but also to introduced sources of novel DNA such as the fraction of recombinant DNA present in genetically modified organisms (GMOs). Such exposure can potentially lead to horizontal gene transfer (HGT) events of GMO recombinant DNA, dependent on the multitude of parameters that govern HGT processes in various environments (Bertolla and Simonet, 1999; Bensasson et al., 2004). However, for long-term persistence of infrequently acquired genetic material in new bacterial hosts, a conferred selective advantage is considered necessary (Feil and Spratt, 2001; Berg and Kurland, 2002; Johnsen et al., 2009; Kuo and Ochman, 2010). Experimental investigations have shown that most HGT events that integrate into the bacterial chromosome are deleterious (Elena et al., 1998; Remold and Lenski, 2004). Thus, in terms of the persistence of its signature and its effects on fitness, HGT processes resemble routine mutational processes that take place at similarly low frequencies in bacteria and that are eventually lost from the population (Kimura and Ohta, 1969; Jorgensen and Kurland, 1987; Lawrence et al., 2001; Mira et al., 2001; Johnsen et al., 2011). However, rare HGT events and mutations can be positively selected under particular conditions and are the sources of bacterial adaptation and evolution (Imhof and Schlötterer, 2001; Townsend et al., 2003; Orr, 2005; Barret et al., 2006). HGT is particularly well known for playing a central role in the evolution of resistance to antibacterial agents (Bergstrom et al., 2000; Heinemann and Traavik, 2004; Aminov and Mackie, 2007; Aminov, 2010, 2011).
The detection of HGT events in a given bacterial genome can be performed retrospectively through bioinformatics-based comparative analyses (Ochman et al., 2000; Spratt et al., 2001; Nakamura et al., 2004; Didelot and Maiden, 2010). Alternatively, events may be detected via focused experimental efforts on defined bacterial populations under controlled conditions in the laboratory or monitoring efforts on subsamples taken from bacterial populations present in various environments, e.g., from soil, water, wounds, or gastrointestinal tracts (GITs; Nielsen and Townsend, 2004; Thomas and Nielsen, 2005; Pontiroli et al., 2009; Aminov, 2011). The latter approach can enable the identification of HGT events as they occur in the context of complex interactions of diverse bacterial communities. Its main limitation is sensitivity due to restricted sampling capacity of large bacterial populations, other methodological limitations, and cost of analysis. Representative analysis of HGT events in bacterial communities also depends on knowledge of the structure and population dynamics of the population and the sequence of the DNA transferred. Detection strategies frequently rely on hidden or implicit assumptions regarding the distribution and proportion of the individual cells in the sampled larger bacterial population that would carry the transferred DNA sequences (Keese, 2008; Heinemann et al., 2011).
Large-scale cultivation of genetically modified plants (GM-plants) result in multitudinous opportunities for bacterial exposure to recombinant DNA and therefore opportunities for unintended horizontal dissemination of transgenes (EFSA, 2004, 2009; Nielsen et al., 2005; Levy-Booth et al., 2007; Wögerbauer, 2007; Pietramellara et al., 2009; Brigulla and Wackernagel, 2010). In laboratory-settings, experimental studies of single bacterial species have demonstrated that bacteria can take up DNA fragments from plants and integrate them into bacterial genomes under highly optimized conditions (e.g., Gebhard and Smalla, 1998; De Vries et al., 2001; Kay et al., 2002; Ceccherini et al., 2003). In contrast, in natural settings, sampling-based studies of agricultural soils, run-off water, and GIT contents have found spread of transgenes from GM-plants, but negative or inconclusive evidence for HGT (Gebhard and Smalla, 1999; Netherwood et al., 2004; Mohr and Tebbe, 2007; Demanèche et al., 2008; Douville et al., 2009).
Most research on HGT from GM-plants to bacteria has been performed via an assay after a limited time period following transgene exposure, perhaps in part because only limited explicit considerations of the population dynamics of HGT events have been presented to guide sampling design and data analysis (Heinemann and Traavik, 2004; Nielsen and Townsend, 2004; Nielsen et al., 2005). Given the low mechanistic probability of occurrence, horizontally transferred non-mobile DNA will initially be present at an exceedingly low frequency in the overall population. It may therefore take months, years, or even longer for the few initial transformants to divide and numerically out-compete non-transformed cells of the population to reach frequencies that can be efficiently detected by sampling efforts. The generation time of bacterial populations is therefore of high importance for detection efforts. Cell division time varies with species and environments and can be as short as <1 h in nutrient rich environments such as the GIT and up to several weeks in nutrient limited environments such as soil.
The time lag between initial occurrence and potential detection will be present even though the relevant HGT events lead to positive selection of transformants (Nielsen and Townsend, 2001, 2004; Heinemann and Traavik, 2004; Pettersen et al., 2005). Quantifying this time lag and determining the relationship between HGT frequencies and probability of detection requires mathematical models with dependency on several key parameters: HGT frequencies, changes in relative fitness of the transformants, bacterial population sizes, and generation times in nature. A few studies have accordingly begun to characterize the effects of natural selection and the probability of fixation of HGT events in bacterial populations (Nielsen and Townsend, 2001, 2004; Pettersen et al., 2005).
Here we integrate previous theory into a cohesive probabilistic framework that addresses current methodological shortcomings in the detection of HGT events and guides experimental design of future sampling of bacterial populations. Our analysis yields a simple formulation for the probability of detection given that a HGT actually occurred, and facilitates computation of the statistical power of an experimental sampling design.
We apply the model to four different scenarios that are relevant for experimental monitoring of complex bacterial communities, accounting for both the adaptive dynamics of natural selection and the unknown timing of HGT events. In scenarios 1 and 2, the effects of variable DNA exposure are considered (i.e., exposed sub-population versus the total population of bacteria). Sampling occurs at the end of the DNA exposure period. In scenarios 3 and 4, the sampling is delayed until sometime after the DNA exposure of the bacterial recipients has ended. The total population size (N) and the strength of selection (m) varies in the scenarios (between N = 106–1012, and m = 10−10–1). The m parameter represents the relative cost or advantage conferred by the HGT event to the transformant bacterium compared to untransformed members of the same population. In nature, m values would range from the reciprocal of the population size (weak positive selection) to near infinity (strong positive selection). The latter would for instance be caused by antibiotic treatment leading to death of all susceptible non-transformed cells. However, for most traits much lower values of m are expected. The m value of a given trait is not a constant and will depend on the environmental conditions. For instance, an antibiotic resistance trait can be highly advantageous in the presence of antibiotics (high positive m) but confer a fitness cost in the absence of antibiotics (negative m value; c.f. Johnsen et al., 2011).
Modeling
Immediately following an HGT event into a large bacterial population, the lineage of bacterial cells carrying the novel transferred gene is highly vulnerable to extinction due to natural stochasticity in cell survival over the first generations (Fisher, 1922, 1930; Haldane, 1927; Johnson and Gerrish, 2002; Pettersen et al., 2005). Subsequently, after the transformant population has established at higher numbers, it can be assumed to follow a fairly deterministic path, given continued directional selection. For a selected variant in transit to fixation with Malthusian relative fitness m per generation over tg generations, the current frequency p of a mutant starting at frequency p0 can be modeled deterministically as

(Hartl and Clark, 1997; Nielsen and Townsend, 2004). With a single HGT event, the frequency of the transformant in a haploid population becomes 1/N where N is the overall number of bacterial cells in the population of interest. Thus, for the frequency of a transformant (p) subsequent to a HGT event,

(Nielsen and Townsend, 2004). Solved for p, Eq. 2 yields

Because HGT events are relatively rare and presumably independent, we assume that the time delay until a HGT occurs is exponentially distributed, parameterized by a rate that incorporates the number of bacteria exposed, x, the rate of HGT per exposed bacterium, r, and the time, tx, during which exposure may occur (See Nielsen and Townsend, 2001 for a detailed description of these factors). Accordingly, the time to the next HGT, Tx, would be distributed as

The probability of fixation of a new variant gene in a haploid population has been characterized as

(Kimura, 1957, 1962; Moran, 1961; Gillespie, 1974; see Patwa and Wahl, 2008, for a review of alternate cases).
Following the exponentially distributed occurrence rate (Eq. 4), filtered by the fixation process (Eq. 5), the timing until the occurrence, T, of the first HGT that is to be eventually fixed in the population, would be distributed as

Given that an HGT occurs that is on its way to fixation, what is the probability that such a transfer will be detected? This probability depends in part on the sample size of the monitoring effort, n. Here, n is treated as the number of bacteria in the environment sampled in a perfect assay for possession of the HGT event. If the frequency of the primary transformant and its offspring in the population at a given time is p, then the probability of detection is

Because the frequency p of a HGT event/transformant that is under strong positive selection deterministically increases with time until it is fixed in the population (Figure 1), the probability of detection depends on the amount of time t since the first HGT event occurred, which depends on the time of first exposure to DNA of concern, T. The later the samples are taken, the greater the probability that a selected HGT event on its way to fixation will be detected.
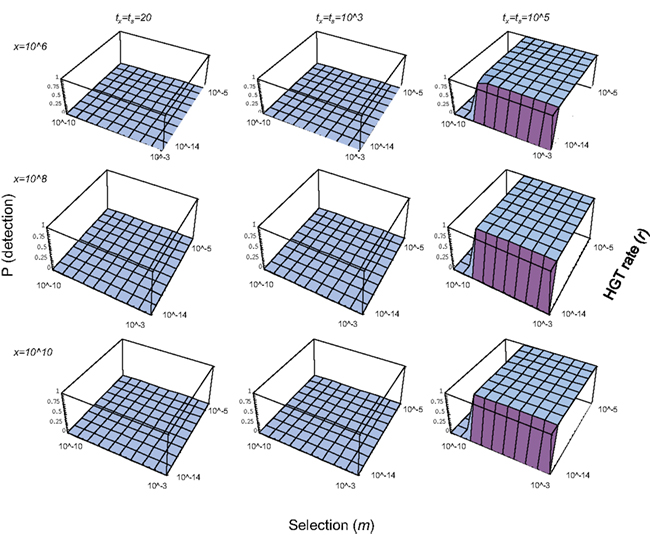
Figure 1. Scenario 1: HGT in large populations, no sampling delay; weak positive selection. Probabilities of detection of transformants in a large bacterial population N = 1012 with HGT rates ranging from r = 10−14 to 10−5, and weak positive selection of transformants ranging from m = 10−10 to 10−3. The proportion of DNA exposed bacteria is low, medium and high (x = 106, 108, and 1010, respectively, out of the 1012 total bacterial population, from top to bottom), and the time period of DNA exposure is the same as the time to sampling: tx = ts = 20, 103, and 105, from left to right. Sample size n = 10,000 bacteria.
The probability of detection for a HGT event on its way to fixation with selection coefficient m at time tg after the original transfer event is derived by substituting Eq. 3 for p into Eq. 7 (c.f. Nielsen and Townsend, 2004). Assuming the value of Eq. 3 is very small (i.e., population size is large and selection coefficient is sufficiently small), a useful approximation for the probability of detection of a HGT event on its way to fixation is
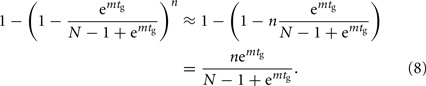
However, for practical implementation, the probability term from Eq. 3 may not be known to be small. Furthermore, the unknown timing of the successful HGT is a key factor in the probability of detection. Therefore it would be best to integrate over all possible timings in order to calculate a representative probability of detection of HGT events. Noting that in this case tg = ts − t, this integration, from Eqs 4, 5, and 8, is
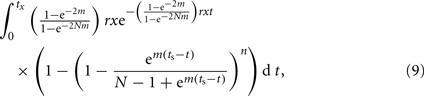
or, moving factors that do not depend upon time t out of the integral,

Equation 10 yields a prediction of the probability of occurrence and detection of a HGT event, and may be parameterized across a range of rates of HGT.
For experimental design purposes (or for prediction for policy purposes), it may be important to calculate not just the full probability of detection, but also the restricted, higher probability of detection given that a successful HGT has occurred. This calculation can be achieved by dividing the result of Eq. 10 by the probability of any successful HGT event over the time tx,

The approximation is valid provided N is large compared to m. Setting this approximation aside for generality, the larger probability of detection given that a successful HGT has occurred is then

Materials and Methods
We applied this model to estimate the probability of successfully finding HGT events (e.g., antibiotic resistance genes or transgenes) in bacterial populations under different scenarios representative of various environmental conditions. The total population sizes ranged from 106 (small) to 1012 (large). We assume only a fraction of the bacterial population that was sampled was exposed to novel genetic material (0.0001–1% in large populations, and 0.1–10% in small populations); resulting in HGT rates (and hence, transformant rates) ranging from 10−14 to 10−5. Moreover, as explained above, we considered only transformants that have relative fitness gains, as expressed by a positive selection coefficient, including weak positive selection (m = 10−10–10−3) or strong positive selection (m = 10−3–1). Our analysis excludes secondary transmissions, a process that may need explicit consideration in cases of plasmid transfer (Landis et al., 2000).
Four different environmental scenarios were examined that broadly represent the population dynamics of HGT events in bacterial populations. The scenarios encompassed: (i) large and small bacterial populations, (ii) strong and weak selection of the HGT events (transformants), and (iii) immediate or delayed sampling, i.e., if the sampling of the larger bacterial population was performed at the end of the DNA exposure, or delayed in time until long after the DNA exposure had ended. Within these scenarios, we varied the HGT rate, the selection coefficient, the ratio of exposed to total population of bacteria, the time period of exposure, and the time until the bacteria was sampled in the field (Table 1).
Since approx. 10,000 bacteria represents the upper limit of the number of individual isolates that can be practically assayed in a research laboratory (Nielsen and Townsend, 2004), we assume this sample size (n = 10,000) for all our scenario calculations, even though effective sample sizes in actual studies to date have been smaller. In scenarios where several samples were taken from a field at several time points, sample size will be proportionally reduced at each sampling point. This ensures comparability among experimental designs.
In scenarios 1 and 2, the focus is on the effects of variable DNA exposure level (exposed sub-population versus the total population of bacteria) and on the strength of selection. We keep the time span of exposure equal to the time span before sampling, i.e., sampling occurs at the end of the DNA exposure (i.e., tx = ts). In scenarios 3 and 4, the sampling is delayed for considerable amounts of time after the DNA exposure of the bacterial recipients has ended (i.e., tx < ts).
All calculations were performed and graphics were drawn in the Mathematica 4.1 software (Wolfram Research, IL, USA). The Mathematica notebook containing these calculations is available in the Appendix.
Results
Scenario 1. Detection of Bacterial Transformants in Large Populations
Scenario 1 represents a large bacterial population (e.g., abundant members of the soil bacterial community or the GIT of an animal population). Of the total population, only a sub-fraction of 0.0001, 0.01, and 1% is actually exposed to DNA (e.g., due to limited release/exposure of DNA from the defined source and or DNA degradation in soil or the GIT; Nielsen et al., 2007; Nordgård et al., 2007; Rizzi et al., 2012). Those bacteria exposed can acquire DNA at rates ranging from extremely low (below what is usually experimentally measurable in the laboratory) to very high. Detection is likely only when sampling was performed after a long period of exposure (Figures 1 and 2). The strength of directional selection is of considerable importance. However the determining factor is time of sampling after onset of exposure. To achieve a 90% or greater probability of detection, given that transfer has occurred, requires a selection coefficient greater than m = 10−4 (Figure 1). This observation suggests a time interval from the onset of a DNA exposure, until detection is possible, of 11 years in the GIT to up to 3,000 years in soil and (given 105 bacterial generations, with generation times of 1 h to 2 weeks, respectively). Figures 1 and 2 illustrate that an increase in the proportion of exposed bacteria is of little importance when compared to prolonging the time period of DNA exposure and sampling. Given enough time, even weakly but positively selected HGT events (e.g., antibiotic resistance gene or transgene) resulting from DNA exposure to only a small fraction of the total population and with a low HGT rate, is likely to establish in the bacterial population.
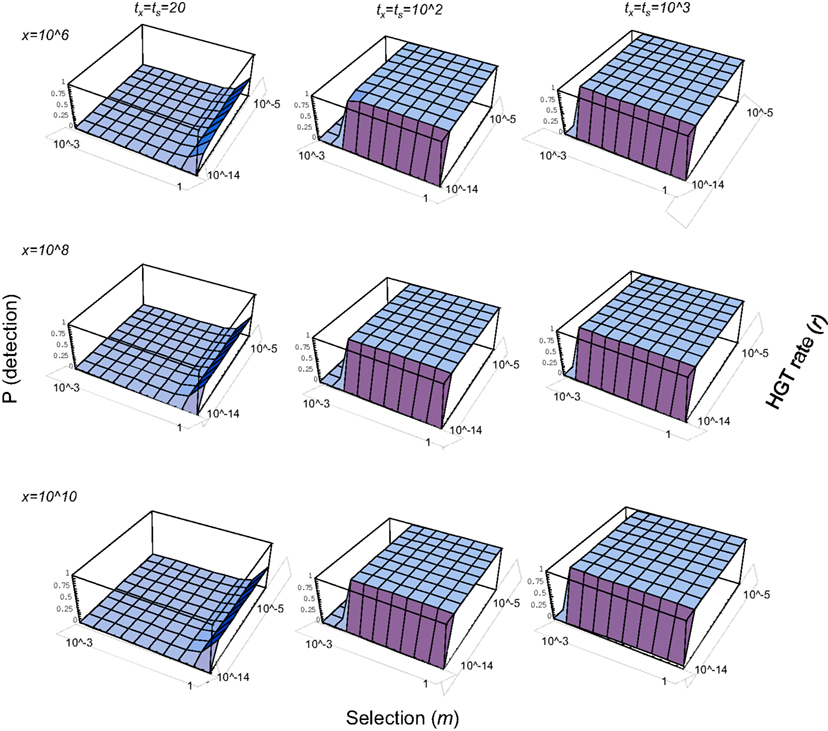
Figure 2. Scenario 1: HGT in large populations, no sampling delay; strong positive selection. Probability of detection of transformants in a large bacterial population, N = 1012, with a HGT rate ranging from r = 10−14 to 10−5, and strong positive selection on transformants ranging from m = 10−3 to 1. The number of bacteria exposed to DNA is low, medium, and high (x = 106, 108, and 1010, respectively, out of the 1012 total bacterial population, from top to bottom), and the time period of DNA exposure is the same as time to sampling: tx = ts = 20, 102, and 103, from left to right. Sample size n = 10,000 bacteria.
Scenario 2. Detection of Bacterial Transformants in Small Populations
Scenario 2 considers a small bacterial population. Such a scenario can be representative of fluctuating colonization or infection patterns such as microcolonies on plant, skin, or soil surfaces (e.g., Kinkel et al., 1995; Morris et al., 1997; Monier and Lindow, 2004), or alternatively situations where only a subset of the species/strains in the overall DNA exposed microbial community are capable of acquiring DNA. In this scenario, a sub-fraction of 0.001, 0.1, and 10% of the overall capable population is exposed to DNA and can acquire DNA at rates ranging from extremely low to high frequencies (r = 10−14–10−5).
When selection is weak, the HGT events and, hence, resulting transformants, are likely to be detected only after relatively long exposure times, i.e., more than 1,000 generations, and only when the fraction of exposed bacteria is about 0.1% or higher (Figure 3). In situations where positive selection is stronger, the HGT event is detectable in the short-term, i.e., after 20 generations, given that the fraction of bacteria that are exposed is high (Figure 4, bottom panel). A combination of an intermediate fraction of bacteria exposed (0.1%) and an intermediate time for DNA exposure (1,000 generations) gives a relatively high probability of detecting HGT events (Figure 4, middle panel). Even in cases where the fraction of bacteria exposed to the DNA is very low, long-term exposure (tx > 105 generations) and strong positive selection will lead to establishment of transformants, i.e., at detectable levels (Figure 4, upper panel). Our longest generation time examined, 105, represents a continual exposure period of 10 years (with a bacterial division time of 1 h or less) to more than 3,000 years (with a bacterial division time of 11 days or more).
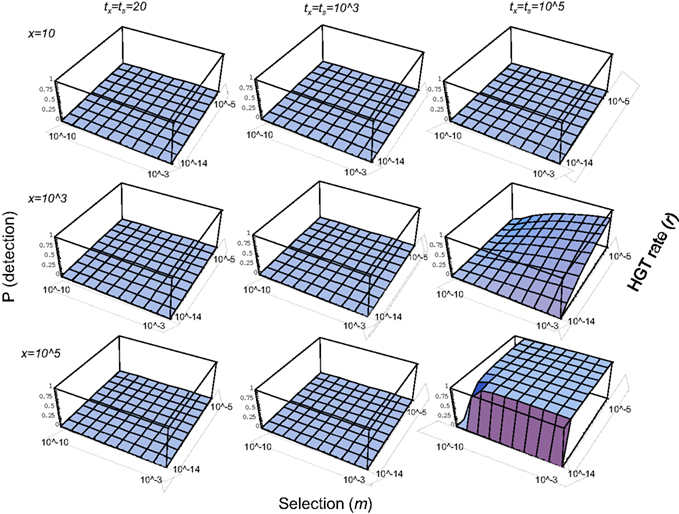
Figure 3. Scenario 2: HGT in small populations, no sampling delay; weak positive selection. Probability of detection of transformants in a small bacterial population, N = 106, with HGT rates ranging from r = 10−14 to 10−5, and weak positive selection on transformants ranging from m = 10−10 to 10−3. The number of bacteria exposed to the DNA is low, medium, and high (x = 10, 103, and 105, respectively, out of the 106 total bacterial population, from top to bottom), and the time period of exposure is the same as the time to sampling; tx = ts = 20, 103, and 105, from left to right. Sample size n = 10,000 bacteria.
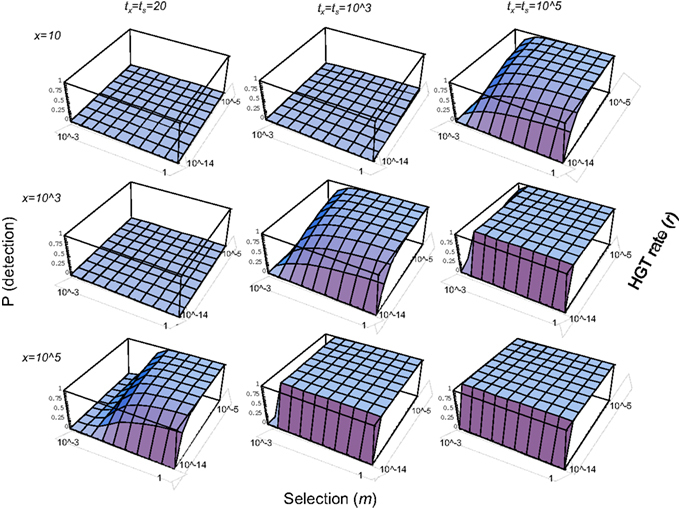
Figure 4. Scenario 2: HGT in small populations, no sampling delay; strong positive selection. Probability of detection of transformants in a small bacterial population, N = 106, with HGT rates ranging from r = 10−14 to 10−5, and strong positive selection on transformants ranging from m = 10−3 to 1. The number of bacteria exposed to DNA is low, medium, and high (x = 10, 103, and 105, respectively, out of the 106 total bacterial population, from top to bottom), and the time period of exposure is the same as the time to sampling: tx = ts = 20, 103, and 105, from left to right. Sample size n = 10,000 bacteria.
The Effect of Delayed Sampling after Short-Term Transient Exposure to DNA
In the following scenarios (three and four, weak and strong selection, respectively), the bacteria are exposed to DNA for only a short period of time (20–100 generations, e.g., representing a time period of a less than a day to a few years, depending on bacterial growth rate). In all cases, the bacterial population is sampled after exposure. That is, a time delay before sampling is introduced after the end of the exposure period that provides additional time for directional selection (of a range of intensities) to act on the transformed cells. These scenarios illustrate situations where the sampled microbial community (e.g., agricultural soil, GIT) is only temporarily exposed to the DNA source in question (e.g., soil or GIT bacteria by seasonal crop cultivation or consumption patterns). We examined large populations (N = 1012) only, and applied different parameter values for the weak and strong selection scenarios. For the weak positive selection scenario, the exposure time was 100 generations, the exposed population was of size n = 108 and the time lag before sampling ranged from 104 to 105 generations). For the strong selection scenario, the DNA exposure time was extremely short (20 bacterial generations), the exposed population was 106, and the time lag before sampling ranged from none (i.e., sampling at the end of exposure), 30 bacterial generations, or 80 bacterial generations later.
Scenario 3. Delayed Sampling – Large Populations and Weak Positive Selection
In situations where potential transformants experience only weak positive selection (10−10–10−3), with 0.01% of the bacterial population exposed to DNA, and an exposure time of 100 generations, no HGT events could be detected either at the end of the exposure or after a delayed sampling (104 generations after DNA exposure; Figure 5, left). However, a further 5- to 10-fold increase in the time delay before sampling (to 5 × 104 and 105 generations) yielded increasing probabilities of detecting the HGT events (Figure 5, middle and right). Thus, theoretically, in environmental situations where the bacterial generation time is very short (e.g., in a mammalian gut system), HGT events arising from limited, transient DNA exposure can be detected, providing they are positively selected and have had the necessary time to increase in relative numbers within the overall population. However, even the most rapidly dividing bacterial populations would need more than 10 years to comprise 5 × 104 and 105 generations (the 10-year figure would assume a bacterial division time of 30 min). Supposing this scenario represented an environment with intermittent antibiotic treatments, the effect of the length of the time period before transformants become detectable would be sensitive to any inconstancy of selection. The time period before the transformant population either increases in proportion to detectability or is lost from the population would therefore be different, and typically longer, in a situation with more variable selection dynamics.
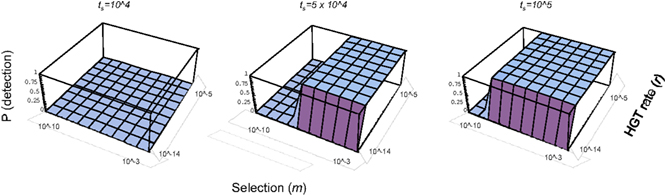
Figure 5. Scenario 3: HGT in large populations with delayed sampling; weak positive selection. Probability of detection of transformants in a large bacterial population, N = 1012, with a HGT rate ranging from r = 10−14 to 10−5 experiencing a short exposure of tx = 100 generations, with delayed sampling. The proportion of the bacteria exposed to DNA is medium (0.01%) and the selection on transformants is weak, ranging from m = 10−10 to 10−3. The time of sampling, encompassing the time of DNA exposure tx, is, from left to right, ts = 104, 5 × 104, and 105 generations. Sample size n = 10,000 bacteria.
Scenario 4. Delayed Sampling – Large Populations and Strong Selection
Under strong positive selection, even HGT events occurring as a consequence of exposure of a very low overall proportion of the population (here 0.0001%) over a short period of time (t = 20 generations) can be detected (Figure 6). The time of sampling nevertheless remains a significant factor in the probability of detection. Sampling at the end of the exposure (T = 20) yields a low probability of detection (Figure 6, left). In contrast, introducing a time lag before sampling, here 30 and 80 generations after the end of exposure (T = 50 and 100, respectively), results in a sharp increase in the likelihood of detection (Figure 6, middle and right). The starkness of this result may at first seem surprising; however positive selection that increases the frequency of the transformed bacteria is the main characteristic that makes detection possible; other factors in this scenario are of negligible importance. Strong directional selection makes such HGT events less affected by genetic drift and the impact of the events therefore closer reflect the distribution of their initial occurrence, similarly to the fate of strongly beneficial mutations (Barret et al., 2006).
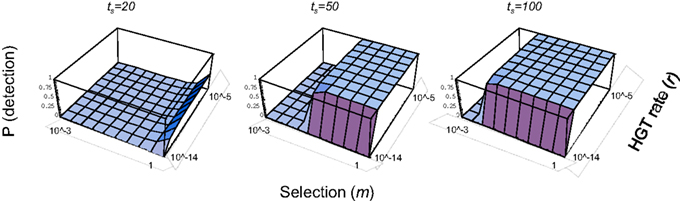
Figure 6. Scenario 4: HGT in large populations with delayed sampling; strong positive selection. Probability of detection of bacterial transformants in a large population N = 1012 with a HGT ranging from r = 10−14 to 10−5, experiencing a short DNA exposure time tx = 20, with sampling at the end of exposure or delayed. The proportion of the bacteria exposed is low (0.0001%). The selection on transformant bacteria is strong, ranging from m = 10−3 to 1. The time of sampling after the onset of DNA exposure is ts = 20, 50, and 100 generations. Sample size n = 10,000 bacteria.
Application to GMP–HGT Monitoring Studies
Application of the model presented here to sampling parameters derived from published field monitoring studies of bacterial populations exposed to GMPs shows limited potential to recover HGT events, had they occurred (Figure 7). Only transgenes conferring strong positive selection coefficients (approx. m = 0.09 or higher) are likely to be detected with high probability by sampling of microbial communities with limited exposure to the GM-plant material (over 365 generations, representing 1 year of exposure). In contrast, HGT of transgenes conferring 10-fold weaker positive selection coefficients (m = 0.009) can be detected with reasonable probability after limited sampling of microbial communities given a 10-fold longer exposure.
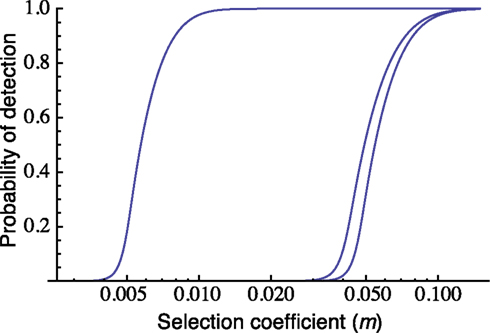
Figure 7. Calculation of the probability of detecting HGT in the field, parameterized with sample sizes from published studies, for selection coefficients ranging from m = 0 to 0.1. Graphs from right to left: a sample size of 600 (Paget et al., 1998), and a sample size of 4000 (Gebhard and Smalla, 1999). The two right graphs assume sampling after ts = 365 generations. The graph on the left side represents a sample size n = 192 after ts = 3,650 generations (Demanèche et al., 2008). In all cases, A HGT rate of r = 10−8 per generation and a population size of N = 1010 bacteria was assumed.
The 10-year long exposure period in a field-based monitoring study by Demanèche et al. (2008) represented the longest exposure period before HGT assay to date. Demanèche and colleagues in France examined samples from agricultural sites that had been cultivated with a GM maize variety over a 10 years time period. In addition, soil samples taken from a conventional field and pasture were included (three sample types) in the analyses. The GM maize variety (event Bt-176) harbored the blaTEM-116 allele, conferring bacterial resistance to certain beta-lactam antibiotics. The outcome of the study was that a range of TEM-type alleles could be found in soil samples taken both from GM and non-GM, conventional sites. The study recovered up to 2.5 × 105 culturable bacteria per gram soil sample and reported up to 6.5% of the ampicillin resistance among the culturable fraction.
A total of 576 resistant colonies were further analyzed with PCR targeted toward blaTEM. Of the 576 amplifications performed on samples from the three different field types, 505 were TEM-positive, representing 87.7% of the resistant fraction. DNA of 80 of the 505 TEM-positive samples was sequenced. Of these, 10 were found to represent the blaTEM-116 allele that is also present in the GM maize event Bt-176. However, only 4 out of the 10 positive samples originated from the soils cultivated with the transgene. Thus, the authors concluded that the resistance gene was already prevalent in soil and that there was no evidence for horizontal dissemination from the GM-plant variety over the 10-year time period. In Figure 7, we assume a sample size of 192 (1/3rd of the 576 resistant samples analyzed by PCR) and a bacterial generation time of one per day (3,650 generations). It is noted that bacterial generation time will vary according to species and strain, as well to seasonal and tempo-spatial effects in soil. Application of our model to the study of Demanèche et al. (2008) suggests that HGT events with m values as low as 0.009 can be detected with at least 90% probability. At these weak levels of selection, the key factor determining the detection probability is the number of bacterial generations, in this case assumed to be 1 per day. Increasing the sample size above n = 192 would have little effect on the probability of detection.
Discussion
We have presented a probabilistic framework for detection of initially rare HGT events/transformants by sampling of larger bacterial populations. This result expands on earlier studies (Nielsen and Townsend, 2001, 2004; Pettersen et al., 2005) to derive a quantitative approach for analysis of the time scale over which HGT events take place and can be detected. Our population genetic framework facilitates practical implementation as well as a more detailed examination of the relative role of the key factors determining the fate of horizontally acquired genes in bacterial populations. The utility of the quantitative approach presented here is, although dependent on some knowledge of the rates of the relevant processes, independent of the specific mechanism of HGT (e.g., transduction, conjugation, transformation). The model is therefore equally applicable to understanding HGT processes between bacterial species/strains/cells as it is applicable to HGT events occurring between unrelated species. Quantitative adjustments to the DNA exposure and HGT rate can accommodate diverse mechanisms. Furthermore, the model identifies parameter values that should guide further hypothesis formation and experimental design.
Selection
The design of sampling approaches aimed at detecting rare HGT processes is deeply challenging, because the fundamental task is to detect a very low probability event with a very small sample size in a very large population. Field studies over limited time periods are correspondingly not likely to identify rare HGT events in large and complex bacterial communities (Figure 7). The detection of HGT events is therefore most often feasible only if the few initial transformants have a growth advantage so they increase their relative proportion in the overall population. However, there are methodological challenges to the implementation of defined selective conditions at the DNA exposure stage when rare transformants arise.
In laboratory systems, the use of antibiotics at concentrations below the minimal inhibitory concentrations (MIC) can possibly apply such directional selection, and hence enrichment of rare transformants present in large, complex microbial communities. However, it is a non-trivial problem to experimentally achieve sub-lethal concentrations of antibiotics that confer directional selection of rare transformants without simultaneously limiting the viability of the overall bacterial population.
In field systems, directional selection and enrichment of initially rare transformants will depend on the prevailing environmental conditions. There are usually few opportunities to introduce directional selection with controlled selection coefficients.
As exemplified in this study, directional selection typically dominates determination of the probability of detection. Strong sampling designs would therefore avoid focus on the detection of the initial HGT events (and associated HGT frequencies), but rather attempt to detect positively selected descendants of the primary transformants. A shift in focus to the detection of descendants precludes precise determination of HGT frequencies. However, frequencies are poor predictors of the short and long-term (evolutionary) impact of HGT events. As long as such events occur repeatedly, other factors will determine the biological impact of these events (Pettersen et al., 2005).
Our calculations are based on a fixed selection coefficient m. However, the strength of selection will frequently fluctuate over space and time due to environmental variables, as well as variability among bacterial genotypes attributable to gene-by-environment interactions (Kimura, 1954; Barker and Butcher, 1966). Thus, selection coefficients will be inexact and will rarely be amenable to robust quantification over variable environments. Furthermore, the genome of a given bacterial transformant will be exposed to other HGT events and mutational processes that may change the initial beneficial fitness effects of a given HGT event (Lenski et al., 1991; Gerrish, 2001; Heffernan and Wahl, 2002; Rozen et al., 2002; Barret et al., 2006; Johnsen et al., 2011).
In practice, host and environmental variation prevents precise and meaningful quantification of m values. Theoretical modeling approaches, however, offer the opportunity to examine the effects of broad ranges of m, therefore providing opportunities to identify threshold values and to predict the dynamics of rare HGT events in larger bacterial populations.
Fixation
Our approach quantifies detection of HGTs that are on their way to fixation (Kimura, 1962), whereas the biological importance of HGT events arises at population proportions much less than one. For instance, the prevalence of a pathogenic strain carrying an HGT event encoding antibiotic resistance is of highest interest when its relative proportion among sensitive strains is <0.1–0.3; as higher proportions will lead to changes in clinical prescription guidance for first line antibiotic therapy (Daneman et al., 2008). Random or seasonal variations in local population sizes may also cause particular genotypes (e.g., transformants) to fluctuate at low frequencies above or below detection for long periods of time (Gerrish and Lenski, 1998). Genetic drift and uneven survival rates in structured bacterial populations are important in determining the fate of transformants (Heffernan and Wahl, 2002; Pettersen et al., 2005). The event of key importance is therefore when the transformant proportion rises to the point where subsequent evolution is largely deterministic based on the current level of directional selection (Rouzine et al., 2001).
The probability of fixation of a transformant by genetic drift alone is governed by the inverse of population size. Given geographically dispersed and large population sizes, the fixation of a horizontally acquired gene/transgene in a bacterial population has been viewed as unlikely (Berg and Kurland, 2002). However, see also views by Majewski and Cohan (1999), Cohan (2002, 2005), and Novozhilov et al. (2005). The likelihood of fixation of a neutral HGT event may differ from the likelihood of fixation of a neutral mutation; this is because mutations occur routinely and repeatedly in large bacterial populations, whereas HGT events may be much more tempo-spatially variable.
Spatial Considerations
Although our model accounts for the effects of natural selection over time, it contains no inherent spatial component. Samples should be collected with consideration that rare horizontal transfers are not expected to occur and be distributed evenly in large, structured bacterial populations. Similarly, antibiotic resistance genes or transgenes are likely to be initially present only in a limited number of patches (e.g., patients/hospitals, or soil sites/fields); representing metapopulations of the larger global population (Maynard Smith et al., 2000). Initial frequencies will match their occurrence, but subsequent frequencies will correspond to the outcome of spatially variable directional selection and genetic drift. Migration between patches may also be of variable intensity and directionality. Uneven distribution patterns need to be considered in the sampling design.
Field Monitoring
The analyses of published GMP field monitoring studies (Figure 7) indicated that detection of HGT events could only be achieved under circumstances of strong positive selection of the hypothesized transformants. Selection coefficients as high as m = 0.05, by evolutionary genetic standards, represent an extraordinary adaptive event. In the laboratory, selection coefficients as small as m = 0.01 can be measured, and over evolutionary time, selection coefficients as small as the inverse of the effective population size (here, this would be as small as 10−10 bacteria per gram sample) are of importance in determining the genome composition of organisms. The retrospective analyses of these studies also suggest that increasing the sample size massively does little to increase the probability of detecting a HGT event that has occurred. However, increasing the delay between exposure and testing permits detection of HGT events characterized by much lower selection coefficients.
Most of the field sampling-based HGT studies published so far have been based on a number of implicit assumptions on the characteristics of the biological system investigated. A more formal theoretical analysis of the population genetic aspects of the system investigated will contribute to make these assumptions explicit; and therefore provide improved clarity and robustness to future experimental design. The model presented here aims to provide guidance on future field-based sampling incorporating key population genetic factors. The multiple levels of, and importance of population genetic considerations in understanding horizontal gene flow have recently been reviewed by Baquero and Coque, 2011, and references within) and Zur Wiesch et al. (2011).
Clinical Settings
The Research topic for this particular issue of the journal is on resistance genes in the open environment, not in clinical settings. The practical scenarios examined in this study are therefore taken from non-clinical environments. However, the general insight of the presented study is also conceptually relevant to the general aspects of the population genetics of horizontal gene flow in clinical environments. This generality arises because, as we indicate in the Section “Discussion,” the model design does not rely on a given DNA transfer mechanism or particular environmental conditions. Our model examines the relationship between the four essential components determining the fate of initially rare HGT events in larger populations: (exposed) population size, HGT rates, bacterial generation time and selective advantage. These four population parameters are essential to the fate of HGT events occurring both in clinical settings among pathogens, as well as in non-clinical settings among non-pathogens. Despite the general insight to clinical scenarios that our model might provide, certain characteristics of the lifestyle of clinical pathogenic populations render specific calculations based on our model to be inappropriate. These characteristics include:
1. Exceptionally strong selective environments are caused by the use of high doses of antibiotics for treatment of bacterial infections. An acquisition of a resistance gene under antibiotic treatment is exceptionally advantageous, as, 100% of the susceptible population is likely to die. Thus, the relative growth advantage is immense, leading to very rapid population expansion of the transformant population and the absence of competitors. Such strong positive selection is not comparable to the much weaker levels of positive selection for most other traits in non-clinical environments. Moreover, clinical antibiotic usage is also highly time-limited, producing strong fluctuations in the selection for a given resistance trait over time, that would require dynamic epidemiological modeling. We assume constant selection over time in our model.
2. The infectious lifestyle of some pathogens leads to exceptionally rapid changes in their population sizes (during infections) followed by strong bottlenecks (during transmission). Thus, depending on the pathogen in question, the transformed cells may or may not be competing with non-transformed members of their populations. Thus, the fitness effects may have a different context in clinical environments depending on the characteristics of the infectious pathogen in question.
The infection pattern of the pathogen in question will also determine its initial population size N, a value that would be very low for a strict pathogen (e.g., tuberculosis) but perhaps initially somewhat larger for opportunistic pathogens (e.g., Clostridium difficile). However, our model assumes a more stable environment with a constant large population size, and is based on a competitive growth advantage of the transformant (relative to non-transformed members of the same populations; present in the same environment). This growth advantage will materialize as higher cell division rates for the transformant; the rate difference expressed through the m (Malthusian fitness parameter) value. Thus, a materialized growth advantage requires the presence of a much larger non-transformed population.
In summary, our model is not designed to capture the intense short-term positive selection, population expansions (infections), coupled with bottlenecks (insufficient antibiotic treatment, and or transmission of a few bacteria to the next patient) that lead to different ranges of population genetic parameters and other model assumptions. From our point of view, such characteristics cannot be included in our model without addressing the etiology of infections and resistance patterns of individual pathogenic strains. Such developments are of high interest for further work.
From the application of our model in the examination of various environmental scenarios, cases and literature examples, it can be concluded that some interspecies HGT is likely to occur over time and spatial scales not amenable to direct experimental observation. The model suggests sampling-based detection of the descendants (offspring) of the initial transformants is achievable; emphasizing that the probability of detection can only correspond to a calculable level of selection, and that a powerful experimental design requires a delayed sampling strategy.
The recent publications by Gallet et al. (2012) and Toprak et al. (2012) present innovative laboratory approaches for quantification of weak positive selection, or for selection of initially rare but positively selected bacterial phenotypes.
Conflict of Interest Statement
The authors declare that the research was conducted in the absence of any commercial or financial relationships that could be construed as a potential conflict of interest.
Acknowledgments
Thomas Bøhn and Kaare Magne Nielsen acknowledge financial support from Genøk, the Research Council of Norway and the University of Tromsø. Kaare Magne Nielsen acknowledges EU COST Action network FP0905, and the AGES, FEAR project, Austria.
References
Aminov, R. I. (2010). A brief history of the antibiotic era: lessons learned and challenges for the future. Front. Microbiol. 1:134.
Aminov, R. I. (2011). Horizontal gene exchange in environmental microbiota. Front. Microbiol. 2:158.
Aminov, R. I., and Mackie, R. I. (2007). Evolution and ecology of antibiotic resistance genes. FEMS Microbiol. Lett. 271, 147–161.
Baquero, F., and Coque, T. M. (2011). Multilevel population genetics in antibiotic resistance. FEMS Microbiol. Rev. 35, 705–706.
Barker, J. S. F., and Butcher, J. C. (1966). A simulation study of quasi-fixation of genes due to random fluctuation of selection intensities. Genetics 53, 261–268.
Barret, R. D. H., M’Gonigle, L. K., and Otto, S. P. (2006). The distribution of beneficial mutant effects under strong selection. Genetics 174, 2071–2079.
Bensasson, D., Boore, J. L., and Nielsen, K. M. (2004). Genes without frontiers. Heredity 92, 483–489.
Berg, O. C., and Kurland, G. C. (2002). Evolution of microbial genomes: sequence acquisition, and loss. Mol. Biol. Evol. 19, 2265–2276.
Bergstrom, C. T., Lipsitch, M., and Levin, B. R. (2000). Natural selection, infectious transfer and the existence conditions of bacterial plasmids. Genetics 155, 505–519.
Bertolla, F., and Simonet, P. (1999). Horizontal gene transfers in the environment: natural transformation as a putative process for gene transfers between transgenic plants and micro-organisms. Res. Microbiol. 150, 5409–5420.
Brigulla, M., and Wackernagel, W. (2010). Molecular aspects of gene transfer and foreign DNA acquisitions in prokaryotes with regard to safety issues. Appl. Microbiol. Biotechnol. 86, 1027–1041.
Ceccherini, M. T., Poté, J., Kay, E., Tran Van, V., Maréchal, J., Pietramellara, G., Nannipieri, P., Vogel, T. M., and Simonet, P. (2003). Degradation and transformability of DNA from transgenic plants. Appl. Environ. Microbiol. 69, 673–678.
Cohan, F. M. (2002). “Clonal structure,” in Encyclopedia of Evolution, ed. M. Pagel (New York: Oxford University Press), 159–163.
Cohan, F. M. (2005). “Periodic selection and ecological diversity in bacteria,” in Selective Sweeps, ed. D. Nurminsky (Georgetown: Landes Bioscience), 78–93.
Daneman, N., Low, D. E., McGeer, A., Green, K. A., and Fisman, D. N. (2008). At the threshold, defining clinically meaningful resistance thresholds for antibiotic choice in community-acquired pneumonia. Clin. Infect. Dis. 46, 1131–1138.
De Vries, J., Meier, P., and Wackernagel, W. (2001). The natural transformation of Pseudomonas stutzeri and Acinetobacter sp. by kanamycin resistance genes (nptII) present in plasmids and the genome of transgenic plants depends on homologous sequences in the recipient cells. FEMS Microbiol. Lett. 195, 211–215.
Demanèche, S., Sanguin, H., Poté, J., Navarro, E., Bernillon, D., Mavingui, P., Wildi, W., Vogel, T. M., and Simonet, P. (2008). Antibiotic resistant soil bacteria in transgenic plant fields. Proc. Natl. Acad. Sci. U.S.A. 105, 3957–3962.
Didelot, X., and Maiden, M. C. (2010). Impact of recombination on bacterial evolution. Trends Microbiol. 18, 315–322.
Douville, M., Gagné, F., Andre, C., and Blaise, C. (2009). Occurrence of the transgenic corn cry1Ab gene in freshwater mussels (Elliptio complanata) near corn fields: evidence of exposure by bacterial ingestion. Ecotoxicol. Environ. Saf. 72, 17–25.
EFSA. (2004). Opinion of the scientific panel on genetically modified organisms on the use of antibiotic resistance genes as marker genes in genetically modified plants. EFSA J. 48, 1–18.
EFSA. (2009). Use of antibiotic resistance genes as marker genes in genetically modified plants. Scientific opinion of the panel on genetically modified organisms (GMO) and the panel on biological hazards (BIOHAZ). EFSA J. 1034, 1–82.
Eisen, J. (2000). Horizontal gene transfer among microbial genomes: new insights from complete genome analysis. Curr. Opin. Genet. Dev. 10, 606–611.
Elena, S. F., Ekunwe, L., Hajela, N., Oden, S. A., and Lenski, R. E. (1998). Distribution of fitness effects caused by random insertion mutations in Escherichia coli. Genetica 102–103, 349–358.
Feil, E. J., and Spratt, B. G. (2001). Recombination and the population structure of bacterial pathogens. Annu. Rev. Microbiol. 55, 561–590.
Gallet, R., Cooper, T. F., Elena, S. F., and Lenormand, T. (2012). Measuring selection coefficients below 10−3: method, questions, and prospects. Genetics 190, 175–186.
Gebhard, F., and Smalla, K. (1998). Transformation of Acinetobacter sp. strain BD413 by transgenic sugar beet DNA. Appl. Environ. Microbiol. 64, 1550–1554.
Gebhard, F., and Smalla, K. (1999). Monitoring field releases of genetically modified sugar beets for persistence of transgenic plant DNA and horizontal gene transfer. FEMS Microbiol. Ecol. 28, 261–272.
Gerrish, P., and Lenski, R. E. (1998). The fate of competing beneficial mutations in an asexual population. Genetica 102/103, 127–144.
Gillespie, J. H. (1974). Natural selection for within-generation variance in offspring number. Genetics 76, 601–606.
Haldane, J. B. S. (1927). A mathematical theory of natural and artificial selection. Part V. Selection and mutation. Proc. Camb. Philos. Soc. 28, 838–844.
Hartl, D. L., and Clark, A. G. (1997). Principles of Population Genetics, 3rd Edn. Sunderland: Sinauer Associates Inc.
Heffernan, J. M., and Wahl, L. M. (2002). The effects of genetic drift in experimental evolution. Theor. Popul. Biol. 62, 349–356.
Heinemann, J. A., Kurenbach, B., and Bleyendaal, N. (2011). Evaluation of horizontal gene transfer monitoring experiments conducted in New Zealand between 2004 and 2009. J. Org. Syst. 6, 3–19.
Heinemann, J. A., and Traavik, T. (2004). Problems in monitoring horizontal gene transfer in field trials of transgenic plants. Nat. Biotechnol. 22, 1105–1109.
Imhof, M., and Schlötterer, C. (2001). Fitness effects of advantageous mutations in evolving Escherichia coli populations. Proc. Natl. Acad. Sci. U.S.A. 98, 1113–1117.
Johnsen, P. J., Townsend, J., Bøhn, T., Simonsen, G. S., Sundsfjord, A., and Nielsen, K. M. (2009). Factors affecting the reversal of antimicrobial resistance. Lancet Infect. Dis. 9, 357–364.
Johnsen, P. J., Townsend, J. P., Bøhn, T., Simonsen, G. S., Sundsfjord, A., and Nielsen, K. M. (2011). Retrospective evidence for a biological cost of vancomycin resistance in the absence of glycopeptide selective pressures. J. Antimicrob. Chemother. 66, 608–610.
Johnson, T., and Gerrish, P. J. (2002). The fixation probability of a beneficial allele in a population dividing by binary fission. Genetica 115, 283–287.
Jorgensen, F., and Kurland, C. G. (1987). Death rates of bacterial mutants. FEMS Microbiol. Lett. 40, 43–46.
Kay, E., Vogel, T. M., Bertolla, F., Nalin, R., and Simonet, P. (2002). In situ transfer of antibiotic resistance genes from transgenic (transplastomic) tobacco plants to bacteria. Appl. Environ. Microbiol. 68, 3345–3351.
Keese, P. (2008). Risks from GMOs due to horizontal gene transfer. Environ. Biosafety Res. 7, 123–149.
Kimura, M. (1954). Process leading to quasi-fixation of genes in natural populations due to random fluctuation of selection intensities. Genetics 39, 280–295.
Kimura, M. (1962). On the probability of fixation of mutant genes in a population. Genetics 47, 713–719.
Kimura, M., and Ohta, T. (1969). Average number of generations until extinction of an individual mutant gene in a finite population. Genetics 61, 763–771.
Kinkel, L. L., Wilson, M., and Lindow, S. E. (1995). Effects of scales on estimates of epiphytic bacterial populations. Microb. Ecol. 29, 283–297.
Kuo, C. H., and Ochman, H. (2010). The extinction dynamics of bacterial pseudogenes. PLoS Genet. 6, e1001050.
Landis, W. G., Lenart, L. A., and Spromberg, J. A. (2000). Dynamics of horizontal gene transfer and the ecological risk assessment of genetically engineered organisms. Hum. Ecol. Risk Assess. 6, 875–899.
Lawrence, J. G. (2002). Gene transfer in bacteria: speciation without species? Theor. Popul. Biol. 61, 449–460.
Lawrence, J. G., Hendrix, R. W., and Casjens, S. (2001). Where are the pseudogenes in bacterial genomes? Trends Microbiol. 9, 535–540.
Lenski, R. E., Rose, M. R., Simpson, S. C., and Tadler, S. C. (1991). Long-term experimental evolution in Escherichia coli. 1. Adaptation and divergence during 2 000 generations. Am. Nat. 138, 1315–1341.
Levy-Booth, D. J., Campbell, R. G., Gulden, R. H., Hart, M. M., Powell, J. R., Klironomos, J. N., Pauls, K. P., Swantonb, C. J., Trevors, J. T., and Dunfield, K. E. (2007). Cycling of extracellular DNA in the soil environment. Soil Biol. Biochem. 39, 2977–2991.
Majewski, J., and Cohan, F. M. (1999). Adapt globally, act locally: the effect of selective sweeps on bacterial sequence diversity. Genetics 152, 1459–1474.
Maynard Smith, J., Feil, E. J., and Smith, N. H. (2000). Population structure and evolutionary dynamics of pathogenic bacteria. Bioessays 22, 1115–1122.
Mira, A., Ochman, H., and Moran, N. A. (2001). Deletion bias and the evolution of bacterial genomes. Trends Genet. 17, 589–596.
Mohr, K., and Tebbe, C. (2007). Field study results on the probability and risk of a horizontal gene transfer from transgenic herbicide-resistant oilseed rape pollen to gut bacteria of bees. Appl. Microbiol. Biotechnol. 75, 573–582.
Monier, J. M., and Lindow, S. E. (2004). Frequency, size, localization of bacterial aggregates on bean leaf surfaces. Appl. Environ. Microbiol. 70, 346–355.
Moran, P. A. P. (1961). The survival of a mutant under general conditions. Proc. Camb. Philos. Soc. 57, 304–314.
Morris, C. E., Monier, J. M., and Jacques, M. A. (1997). Methods for observing microbial biofilms directly on leaf surfaces and recovering them for isolation of culturable microorganisms. Appl. Environ. Microbiol. 63, 1570–1576.
Nakamura, Y., Itoh, T., Matsuda, H., and Gojobori, T. (2004). Biased biological functions of horizontally transferred genes in prokaryotic genomes. Nat. Genet. 36, 760–766.
Netherwood, T., Martín-Orúe, S. M., O’Donnell, A. G., Gockling, S., Graham, J., Mathers, J. C., and Gilbert, H. J. (2004). Assessing the survival of transgenic plant DNA in the human gastrointestinal tract. Nat Biotechnol. 22, 204–209.
Nielsen, K. M., Berdal, K. G., Kruse, H., Sundsfjord, A., Mikalsen, A., Yazdankhah, S., and Nes, I. (2005). An Assessment of Potential Long-Term Health Effects Caused by Antibiotic Resistance Marker Genes in Genetically Modified Organisms Based on Antibiotic Usage and Resistance Patterns in Norway. VKM-Report, Norwegian Scientific Committee for Food Safety, Oslo.
Nielsen, K. M., Johnsen, P. J., Bensasson, D., and Daffonchio, D. (2007). Release and persistence of extracellular DNA in the open environment. Environ. Biosafety Res. 6, 37–53.
Nielsen, K. M., and Townsend, J. P. (2001). “Environmental exposure, horizontal transfer and selection of transgenes in bacterial populations,” in Enhancing Biocontrol Agents and Handling Risks, Vol. 339, eds M. Vurro, J. Gressel, T. Butt, G. E. Harman, A. Pilgeram, R. J. St. Leger, and D. L. Nuss (Amsterdam: NATO Science Series, IOS Press), 145–158.
Nielsen, K. M., and Townsend, J. P. (2004). Monitoring and modeling horizontal gene transfer. Nat. Biotechnol. 22, 1110–1114.
Nordgård, L., Nguyen, T., Midtvedt, T., Benno, Y., Traavik, T., and Nielsen, K. M. (2007). Lack of detectable uptake of DNA by bacterial gut isolates grown in vitro and by Acinetobacter baylyi colonizing rodents in situ. Environ. Biosafety Res. 6, 149–160.
Novozhilov, A. S., Karev, G. P., and Koonin, E. V. (2005). Mathematical modelling of evolution of horizontally transferred genes. Mol. Biol. Evol. 22, 1721–1732.
Ochman, H., Lawrence, J. G., and Groisman, E. A. (2000). Lateral gene transfer and the nature of bacterial innovation. Nature 405, 299–304.
Paget, E., Lebrun, M., Freyssinet, G., and Simonet, P. (1998). The fate of recombinant plant DNA in soil. Eur. J. Soil Biol. 34, 81–88.
Patwa, Z., and Wahl, L. M. (2008). The fixation probability of beneficial mutations. J. R. Soc. Interface 5, 1279–1289.
Pettersen, A. K., Primicero, R., Bøhn, T., and Nielsen, K. M. (2005). Modeling suggests frequency estimates are not informative for predicting the long-term effect of horizontal gene transfer in bacteria. Environ. Biosafety Res. 4, 222–233.
Pietramellara, G., Ascher, J., Borgogni, F., Ceccherini, M.-T., Guerri, G., and Nannipieri, P. (2009). Extracellular DNA in soil and sediment: fate and ecological relevance. Biol. Fertil. Soils 45, 219–235.
Pontiroli, A., Rizzi, A., Simonet, P., Daffonchio, D., Vogel, T. M., and Monier, J. M. (2009). Visual evidence of horizontal gene transfer between plant and bacteria in the phytosphere of transplastomic tobacco. Appl. Environ. Microbiol. 75, 3314–3322.
Pontiroli, A., Simonet, P., Frostegard, A., Vogel, T. M., and Monier, J. M. (2007). Fate of transgenic plant DNA in the environment. Environ. Biosafety Res. 6, 15–35.
Remold, S. K., and Lenski, R. E. (2004). Pervasive joint influence of epistasis and plasticity on mutational effects in Escherichia coli. Nat. Genet. 36, 423–426.
Rizzi, A., Raddadi, N., Sorlini, C., Nordgård, L., Nielsen, K. M., and Daffonchio, D. (2012). The stability and degradation of dietary DNA in the gastrointestinal tract of mammals – implications for horizontal gene transfer and the biosafety of GMOs. Crit. Rev. Food Sci. Nutr. 52, 142–161.
Rouzine, I. M., Rodrigo, A., and Coffin, J. M. (2001). Transition between stochastic evolution and deterministic evolution in the presence of selection: general theory and application to virology. Microbiol. Mol. Biol. Rev. 65, 151–185.
Rozen, D. E., de Visser, J. A., and Gerrish, P. J. (2002). Fitness effects of fixed beneficial mutations in microbial populations. Curr. Biol. 12, 1040–1045.
Spratt, B. G., Hanage, W. P., and Feil, E. J. (2001). The relative contribution of recombination and point mutation to the diversification of bacterial clones. Curr. Opin. Microbiol. 4, 602–606.
Thomas, C. M., and Nielsen, K. M. (2005). Mechanisms and barriers to horizontal gene transfer between bacteria. Nat. Rev. Microbiol. 3, 711–721.
Toprak, E., Veres, A., Michel, J. B., Chait, R., Hartl, D. L., and Kishony R. (2012). Evolutionary paths to antibiotic resistance under dynamically sustained drug selection. Nat. Genet. 44, 101–105.
Townsend, J. P., Nielsen, K. M., Fisher, D., and Hartl, D. L. (2003). Horizontal acquisition of divergent chromosomal DNA in bacteria: effects of mutator phenotypes. Genetics 164, 13–21.
Wögerbauer, M. (2007). “Risk assessment of antibiotic resistance marker genes in genetically modified organisms,” in BMGFJ – Forschungsberichte der Sektion IV, Band 5/2007, Vienna, Austria.
Zur Wiesch, P. A., Kouyos, R., Engelstadter, J., Regoes, R. R., and Bonhoeffer, S. (2011). Population biological principles of drug-resistance evolution in infectious diseases. Lancet Infect. Dis. 11, 236–247.
Appendix

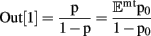

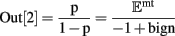




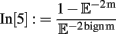
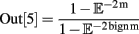

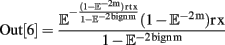


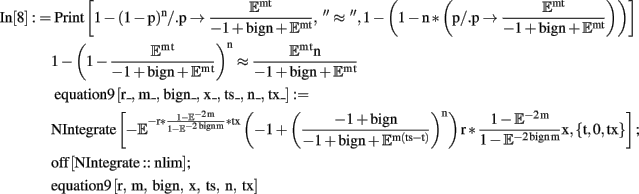





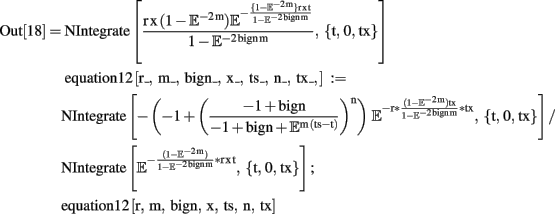



Keywords: lateral or horizontal gene transfer, DNA uptake, modeling, monitoring, sampling, antibiotic resistance, GMO, biosafety
Citation: Townsend JP, Bøhn T and Nielsen KM (2012) Assessing the probability of detection of horizontal gene transfer events in bacterial populations. Front. Microbio. 3:27. doi: 10.3389/fmicb.2012.00027
Received: 12 November 2011; Accepted: 16 January 2012; Published online: 20 February 2012.
Edited by:
Rustam I. Aminov, University of Aberdeen, UKReviewed by:
Morten Otto Alexander Sommer, Technical University of Denmark, DenmarkLilia Macovei, The Forsyth Institute, USA; Douda Bensasson, University of Manchester, UK
Copyright: © 2012 Townsend, Bøhn and Nielsen. This is an open-access article distributed under the terms of the Creative Commons Attribution Non Commercial License, which permits non-commercial use, distribution, and reproduction in other forums, provided the original authors and source are credited.
*Correspondence: Kaare Magne Nielsen, Department of Pharmacy, Research Group in Microbiology, Molecular and Pharmaco-Epidemiology, Breivika, 9037 Tromso, Tromso, Norway e-mail: kaare.nielsen@uit.no