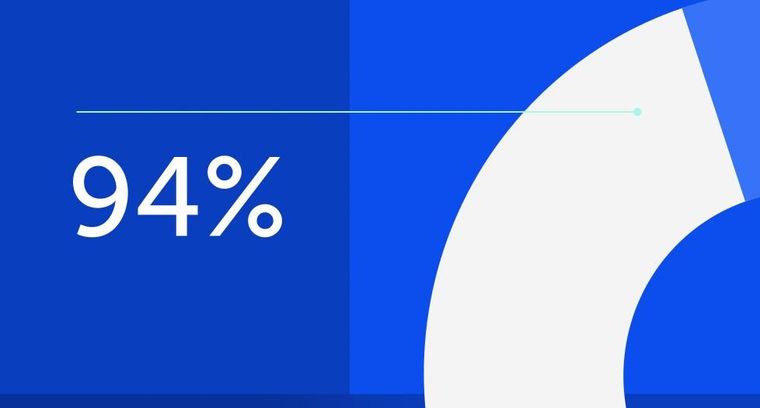
94% of researchers rate our articles as excellent or good
Learn more about the work of our research integrity team to safeguard the quality of each article we publish.
Find out more
ORIGINAL RESEARCH article
Front. Med.
Sec. Precision Medicine
Volume 12 - 2025 | doi: 10.3389/fmed.2025.1599206
This article is part of the Research TopicBridging Tradition and Future: Cutting-edge Exploration and Application of Artificial Intelligence in Comprehensive Diagnosis and Treatment of Lung DiseasesView all 7 articles
The final, formatted version of the article will be published soon.
Select one of your emails
You have multiple emails registered with Frontiers:
Notify me on publication
Please enter your email address:
If you already have an account, please login
You don't have a Frontiers account ? You can register here
Objective: This study aimed to evaluate the predictive performance of integrated clinical and CTbased radiomic models for assessing targeted therapy efficacy in advanced lung adenocarcinoma patients with EGFR(epidermal growth factor receptor) mutations.: This retrospective study included 106 EGFR-mutated advanced lung adenocarcinoma patients treated with targeted therapies at the Second Hospital of Jilin University (2020-2023). Patients were classified as responders (PR) or non-responders (SD/PD) based on RECIST(Response Evaluation Criteria in Solid Tumors) 1.1 criteria, then randomly divided into training (n=74) and validation (n=32) cohorts at a 7:3 ratio. We segmented tumor regions on pre-and post-treatment CT scans using ITK-SNAP, then extracted radiomic features and applied mRMR-LASSO(Minimum Redundancy Maximum Relevance-Least Absolute Shrinkage and Selection Operator). A delta-radiomics model was developed by quantifying feature changes between treatment phases. Significant clinical predictors identified by logistic regression were integrated with radiomic features to build a combined model. Performance was assessed via AUC, sensitivity, specificity, accuracy, positive predictive value (PPV), negative predictive value (NPV), DeLong's test, calibration curves, and decision curve analysis. Results: In the pre-treatment radiomics model, the AUC, accuracy, sensitivity, specificity, PPV, and NPV of the training cohorts were 0.751, 0.690, 0.737, 0.639, 0.683, and 0.697; in validation cohorts, these values were 0.726, 0.656, 0.778, 0.500, 0.667, and 0.636. In the delta-radiomics model, the AUC, accuracy, sensitivity, specificity, PPV, and NPV of the training cohorts were 0.906, 0.865, 0.868, 0.861, 0.868, and 0.861, versus 0.825, 0.719, 0.722, 0.714, 0.765, and 0.667 in validation. For the clinical model, the AUC, accuracy, sensitivity, specificity, PPV, and NPV of the training cohorts were 0.828, 0.729, 0.737, 0.722, 0.737, and 0.722, compared to 0.766, 0.750, 0.722, 0.786, 0.812, and 0.688 in validation. In the combined model,the AUC, accuracy, sensitivity, specificity, PPV, and NPV of the training cohorts were 0.977, 0.946, 0.947, 0.944, 0.947, and 0.944, while in the validation cohorts, these values were 0.913, 0.781, 0.778, 0.786, 0.824, and 0.733.The combined model integrating delta-radiomics with clinical predictors demonstrates superior predictive performance for evaluating targeted therapy efficacy in EGFR-mutated advanced lung adenocarcinoma, significantly outperforming conventional radiomics models relying exclusively on pre-treatment imaging data.
Keywords: lung adenocarcinoma1, EGFR, targeted therapy2, radiomics3, Delta-radiomics4, Nomogram5
Received: 24 Mar 2025; Accepted: 17 Apr 2025.
Copyright: © 2025 Wu, Hua, Chen, Lei, Zhang and Zhang. This is an open-access article distributed under the terms of the Creative Commons Attribution License (CC BY). The use, distribution or reproduction in other forums is permitted, provided the original author(s) or licensor are credited and that the original publication in this journal is cited, in accordance with accepted academic practice. No use, distribution or reproduction is permitted which does not comply with these terms.
* Correspondence: Peng Zhang, Department of Radiology, The Second Hospital of Jilin University,, Changchun, Hebei Province, China
Disclaimer: All claims expressed in this article are solely those of the authors and do not necessarily represent those of their affiliated organizations, or those of the publisher, the editors and the reviewers. Any product that may be evaluated in this article or claim that may be made by its manufacturer is not guaranteed or endorsed by the publisher.
Supplementary Material
Research integrity at Frontiers
Learn more about the work of our research integrity team to safeguard the quality of each article we publish.