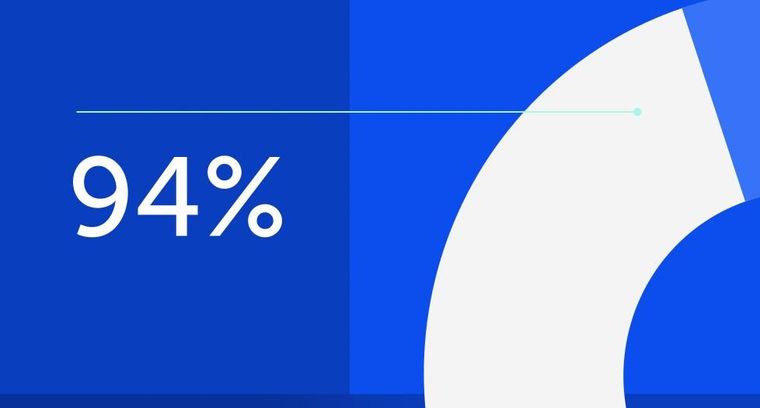
94% of researchers rate our articles as excellent or good
Learn more about the work of our research integrity team to safeguard the quality of each article we publish.
Find out more
REVIEW article
Front. Med.
Sec. Hepatobiliary Diseases
Volume 12 - 2025 | doi: 10.3389/fmed.2025.1591523
This article is part of the Research TopicDigital Technologies in Hepatology: Diagnosis, Treatment, and Epidemiological InsightsView all 6 articles
The final, formatted version of the article will be published soon.
Select one of your emails
You have multiple emails registered with Frontiers:
Notify me on publication
Please enter your email address:
If you already have an account, please login
You don't have a Frontiers account ? You can register here
Liver diseases, including hepatitis, non-alcoholic fatty liver disease (NAFLD), cirrhosis, and hepatocellular carcinoma (HCC), remain a major global health concern, with early and accurate diagnosis being essential for effective management. Imaging modalities such as ultrasound (US), computed tomography (CT), and magnetic resonance imaging (MRI) play a crucial role in non-invasive diagnosis, but their sensitivity and diagnostic accuracy can be limited. Recent advancements in artificial intelligence (AI) have improved imaging-based liver disease assessment by enhancing pattern recognition, automating fibrosis and steatosis quantification, and aiding in HCC detection. AI-driven imaging techniques have shown promise in fibrosis staging through US, CT, MRI, and elastography, reducing the reliance on invasive liver biopsy. For liver steatosis, AI-assisted imaging methods have improved sensitivity and grading consistency, while in HCC detection and characterization, AI models have enhanced lesion identification, classification, and risk stratification across imaging modalities. The growing integration of AI into liver imaging is reshaping diagnostic workflows and has the potential to improve accuracy, efficiency, and clinical decision-making. This review provides an overview of AI applications in liver imaging, focusing on their clinical utility and implications for the future of liver disease diagnosis.
Keywords: Liver Diseases, artificial intelligence, imaging, diagnosis, clinical applications
Received: 11 Mar 2025; Accepted: 08 Apr 2025.
Copyright: © 2025 Yin, Zhang, Du, Zhu, Zhu and Yue. This is an open-access article distributed under the terms of the Creative Commons Attribution License (CC BY). The use, distribution or reproduction in other forums is permitted, provided the original author(s) or licensor are credited and that the original publication in this journal is cited, in accordance with accepted academic practice. No use, distribution or reproduction is permitted which does not comply with these terms.
* Correspondence: Hua Zhu, Yancheng Third People's Hospital, Yancheng, China
Disclaimer: All claims expressed in this article are solely those of the authors and do not necessarily represent those of their affiliated organizations, or those of the publisher, the editors and the reviewers. Any product that may be evaluated in this article or claim that may be made by its manufacturer is not guaranteed or endorsed by the publisher.
Supplementary Material
Research integrity at Frontiers
Learn more about the work of our research integrity team to safeguard the quality of each article we publish.