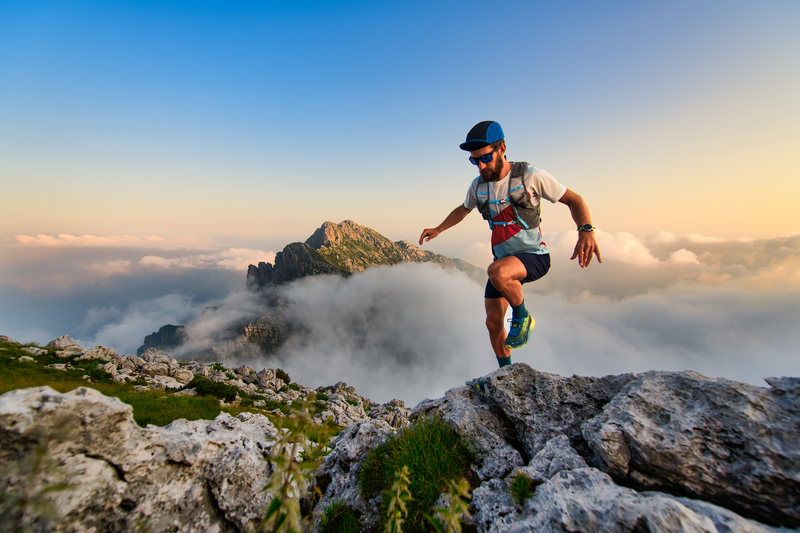
95% of researchers rate our articles as excellent or good
Learn more about the work of our research integrity team to safeguard the quality of each article we publish.
Find out more
ORIGINAL RESEARCH article
Front. Med.
Sec. Pulmonary Medicine
Volume 12 - 2025 | doi: 10.3389/fmed.2025.1590824
This article is part of the Research Topic Application of Multimodal Data and Artificial Intelligence in Pulmonary Diseases View all 3 articles
The final, formatted version of the article will be published soon.
You have multiple emails registered with Frontiers:
Please enter your email address:
If you already have an account, please login
You don't have a Frontiers account ? You can register here
Pulmonary diseases, such as pneumonia and lung abscess, can trigger sepsis, while sepsisinduced immune dysfunction exacerbates Pulmonary tissue damage, creating a vicious cycle.Therefore, designing a safe and effective clinical treatment planning method for sepsis is critically significant. In recent years, deep reinforcement learning (DRL), as one of the artificial intelligence technologies, has achieved remarkable results in the field of sepsis treatment.However, DRL models may be attacked due to their sensitive training data and their high commercial value, especially with the increasing number of DRL models being released on the Internet. Consequently, protecting the "privacy" of DRL models and training data has become an urgent problem. To address this issue, we propose a differential privacy-based DRL model for sepsis treatment. Furthermore, we investigate the impact of differential privacy mechanisms on the performance of the DRL model. Experimental results demonstrate that integrating differential privacy into DRL models enables clinicians to design sepsis treatment plans while protecting patient privacy, thereby mitigating lung tissue damage and dysfunction caused by sepsis.
Keywords: Pulmonary diseases, Sepsis treatment, artificial intelligence, deep reinforcement learning, Differential privacy, clinical treatment plan
Received: 10 Mar 2025; Accepted: 02 Apr 2025.
Copyright: © 2025 Wang, Yang, Wang and Sun. This is an open-access article distributed under the terms of the Creative Commons Attribution License (CC BY). The use, distribution or reproduction in other forums is permitted, provided the original author(s) or licensor are credited and that the original publication in this journal is cited, in accordance with accepted academic practice. No use, distribution or reproduction is permitted which does not comply with these terms.
* Correspondence:
Rongdao Sun, Department of Neurology, Haikou Affiliated Hospital of Central South University Xiangya School of Medicine, Haikou, China
Disclaimer: All claims expressed in this article are solely those of the authors and do not necessarily represent those of their affiliated organizations, or those of the publisher, the editors and the reviewers. Any product that may be evaluated in this article or claim that may be made by its manufacturer is not guaranteed or endorsed by the publisher.
Research integrity at Frontiers
Learn more about the work of our research integrity team to safeguard the quality of each article we publish.