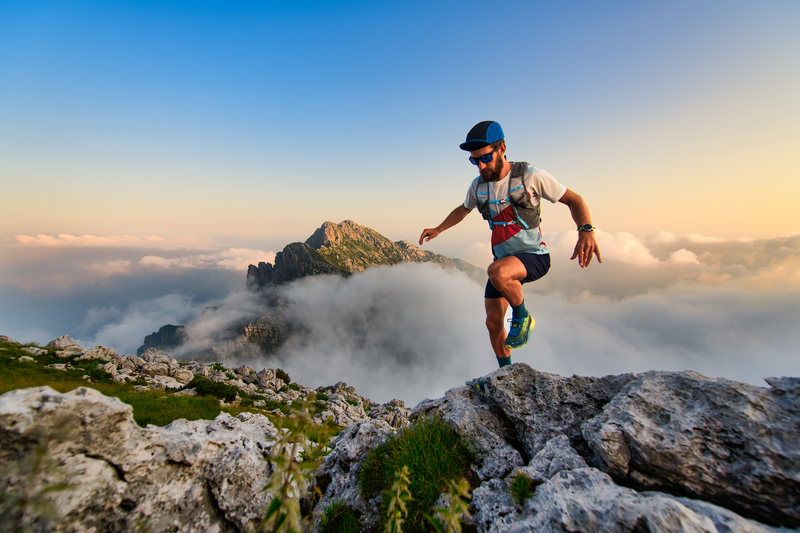
95% of researchers rate our articles as excellent or good
Learn more about the work of our research integrity team to safeguard the quality of each article we publish.
Find out more
ORIGINAL RESEARCH article
Front. Med.
Sec. Precision Medicine
Volume 12 - 2025 | doi: 10.3389/fmed.2025.1582560
This article is part of the Research Topic Revolutionizing Cancer Care: AI and Technological Advances in Breast and Gynecological Oncology View all articles
The final, formatted version of the article will be published soon.
You have multiple emails registered with Frontiers:
Please enter your email address:
If you already have an account, please login
You don't have a Frontiers account ? You can register here
Objective: This study aimed to develop a comprehensive nomogram model by integrating clinical pathological and full-field digital mammography (FFDM) radiomic features to predict the efficacy of neoadjuvant chemotherapy (NAC) in breast cancer patients, thereby providing personalized treatment recommendations. Methods: A retrospective analysis was conducted on the clinical and imaging data of 227 breast cancer patients from 2016 to 2023 at the Second Affiliated Hospital of Harbin Medical University. The patients were divided into a training set (n=159) and a test set (n=68) with a 7:3 ratio. The region of interest (ROI) was manually segmented on FFDM images, and features were extracted and gradually selected. The rad-score was calculated for each patient. Five machine learning classifiers were used to build radiomics models, and the optimal model was selected. Univariate and multivariate regression analyses were performed to identify independent risk factors for predicting the efficacy of NAC in breast cancer patients. A nomogram prediction model was further developed by combining the independent risk factors and rad-score, and probability-basedrisk stratification was applied. An independent cohort was collected from an external hospital to evaluate the performance of the model.The radiomics model based on support vector machine (SVM) demonstrated the best predictive performance. FFDM tumor density and HER-2 status were identified as independent risk factors for achieving pathologic complete response (PCR) after NAC (P<0.05). The nomogram prediction model, developed by combining the independent risk factors and rad-score, outperformed other models, with areas under the curve (AUC) of 0.91 and 0.85 for the training and test sets, respectively. Based on the optimal cutoff points of 103.42 from the nomogram model, patients were classified into high-risk probability and low-risk probability groups. When the nomogram model was applied to an independent cohort of 18 47 patients, only one four patients was misclassified as achieving PCR after NAChad incorrect diagnoses. The nomogram model demonstrated stable and accurate predictive performance.The nomogram prediction model, developed by integrating clinical pathological and radiomic features, demonstrated significant performance in predicting the efficacy of NAC in breast cancer, providing valuable reference for clinical personalized prediction planning.
Keywords: breast cancer, Radiomics, Neoadjuvant chemotherapy, machine learning, Full-field digital mammography, nomogram
Received: 24 Feb 2025; Accepted: 02 Apr 2025.
Copyright: © 2025 Ruan, Liu, Jin, zhao, Zhang, Cheng, Wang, Cao, Yan, Cai, Li and GAO. This is an open-access article distributed under the terms of the Creative Commons Attribution License (CC BY). The use, distribution or reproduction in other forums is permitted, provided the original author(s) or licensor are credited and that the original publication in this journal is cited, in accordance with accepted academic practice. No use, distribution or reproduction is permitted which does not comply with these terms.
* Correspondence:
BO GAO, Departments of Radiology, Second Affiliated Hospital, Harbin Medical University, Harbin, China
Disclaimer: All claims expressed in this article are solely those of the authors and do not necessarily represent those of their affiliated organizations, or those of the publisher, the editors and the reviewers. Any product that may be evaluated in this article or claim that may be made by its manufacturer is not guaranteed or endorsed by the publisher.
Research integrity at Frontiers
Learn more about the work of our research integrity team to safeguard the quality of each article we publish.