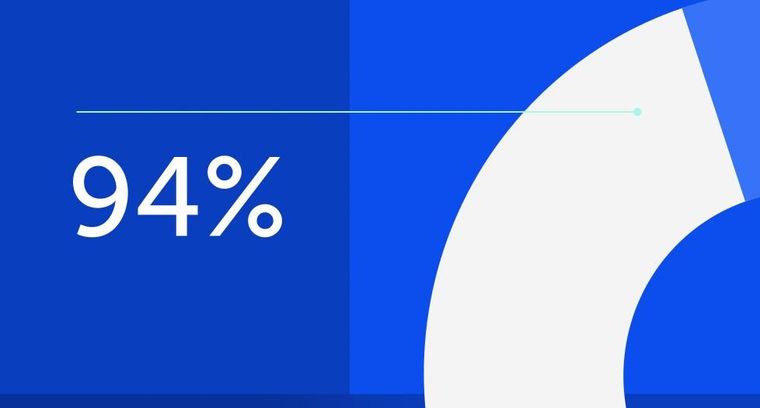
94% of researchers rate our articles as excellent or good
Learn more about the work of our research integrity team to safeguard the quality of each article we publish.
Find out more
ORIGINAL RESEARCH article
Front. Med.
Sec. Precision Medicine
Volume 12 - 2025 | doi: 10.3389/fmed.2025.1577474
This article is part of the Research TopicAI Innovations in Neuroimaging: Transforming Brain AnalysisView all articles
The final, formatted version of the article will be published soon.
Select one of your emails
You have multiple emails registered with Frontiers:
Notify me on publication
Please enter your email address:
If you already have an account, please login
You don't have a Frontiers account ? You can register here
Affecting millions of individuals worldwide, epilepsy is a neurological condition marked by repeated convulsions. Monitoring brain activity and identifying seizures depends much on electroencephalography (EEG). An essential step that may help clinicians identify and treat epileptic seizures is the differentiation between epileptic and non-epileptic signals by use of epileptic seizure detection categorization. In this work, we investigated Machine learning algorithms including Random Forest, Gradient Boosting, and K-Nearest Neighbors, alongside advanced DL architectures such as Long Short-Term Memory networks and Long-term Recurrent Convolutional Networks for detecting epileptic seizures in terms of difficulties and procedures evolved depending on EEG data.The EEG data classification by applying ML and DL framework to improve the accuracy of seizure detection. The EEG dataset consisted of 102 patients (55 seizure and 47 non-seizure cases), and the data underwent comprehensive preprocessing, including noise removal, frequency band extraction, and data balancing using SMOTE to address class imbalance. Key features, including delta, theta, alpha, beta, and gamma bands, as well as spectral entropy, were extracted to aid in the classification process. A comparative analysis was conducted, resulting in high classification accuracy, with the Random Forest model achieving the best results at 99.9% accuracy. The study demonstrates the potential of EEG data for reliable seizure detection while emphasizing the need for further development of more practical and non-invasive monitoring systems for real-world applications.
Keywords: Electroencephalography, EEG data classification, Seizure detection, Epilepsy, SMOTE
Received: 15 Feb 2025; Accepted: 14 Apr 2025.
Copyright: © 2025 Al-Adhaileh, Ahmad, Alharbi, ALARFAJ, Dhopeshwarkar and Aldhyani. This is an open-access article distributed under the terms of the Creative Commons Attribution License (CC BY). The use, distribution or reproduction in other forums is permitted, provided the original author(s) or licensor are credited and that the original publication in this journal is cited, in accordance with accepted academic practice. No use, distribution or reproduction is permitted which does not comply with these terms.
* Correspondence: Theyazn H.H Aldhyani, King Faisal University, Al-Ahsa, Saudi Arabia
Disclaimer: All claims expressed in this article are solely those of the authors and do not necessarily represent those of their affiliated organizations, or those of the publisher, the editors and the reviewers. Any product that may be evaluated in this article or claim that may be made by its manufacturer is not guaranteed or endorsed by the publisher.
Supplementary Material
Research integrity at Frontiers
Learn more about the work of our research integrity team to safeguard the quality of each article we publish.