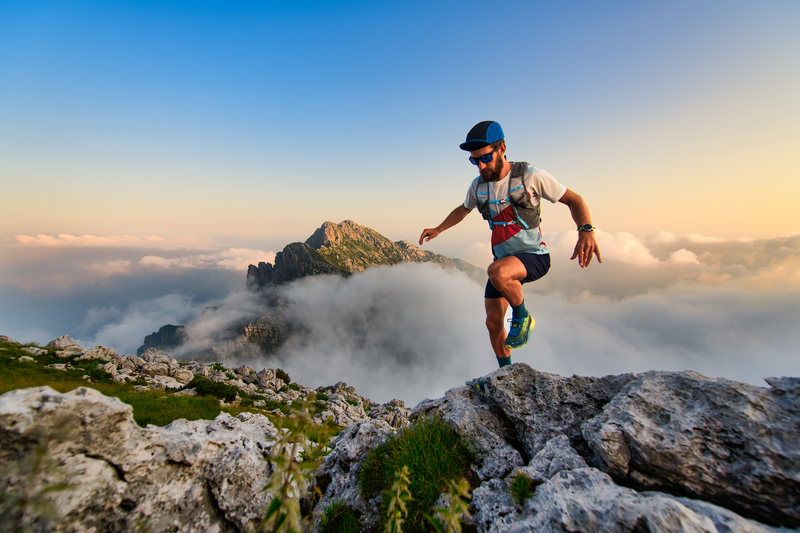
95% of researchers rate our articles as excellent or good
Learn more about the work of our research integrity team to safeguard the quality of each article we publish.
Find out more
ORIGINAL RESEARCH article
Front. Med.
Sec. Ophthalmology
Volume 12 - 2025 | doi: 10.3389/fmed.2025.1573329
This article is part of the Research Topic Imaging in the Diagnosis and Treatment of Eye Diseases View all 18 articles
The final, formatted version of the article will be published soon.
You have multiple emails registered with Frontiers:
Please enter your email address:
If you already have an account, please login
You don't have a Frontiers account ? You can register here
Machine learning technology has demonstrated significant potential in glaucoma research, particularly in early diagnosis, predicting disease progression, evaluating treatment responses, and developing personalized treatment strategies. The application of machine learning not only enhances the understanding of the pathological mechanism of glaucoma and optimizes the diagnostic process but also provides patients with accurate medical services. This study aimed to describe the current state of research, highlight directions for further development, and identify potential trends for improvement. This review was conducted following the scoping review of the Preferred Reporting Items for Systematic Reviews and Meta-Analyses (PRISMA) extension to showcase advancements in the application of machine learning in glaucoma research and treatment. We employed a comprehensive search strategy to retrieve literature from the Web of Science Core Collection database, ultimately including 3,581 articles in the analysis. Through data analysis, we identified current research hotspots, noted differences in researchers' attitudes and opinions, and predicted potential future development trends. We divided the research topics into six categories, clearly identifying "eye diseases", "retinal fundus imaging" and "risk factors" as the key terms for the development of this field. These findings signify the promising prospects of machine learning, particularly when integrated with multimodal technologies and large language models, to enhance the diagnosis and treatment of glaucoma.
Keywords: machine learning, Glaucoma diagnosis, deep learning, Multimodal Imaging, ophthalmic research
Received: 08 Feb 2025; Accepted: 03 Apr 2025.
Copyright: © 2025 Fan, Zhang, Tian, Tian, Si and Li. This is an open-access article distributed under the terms of the Creative Commons Attribution License (CC BY). The use, distribution or reproduction in other forums is permitted, provided the original author(s) or licensor are credited and that the original publication in this journal is cited, in accordance with accepted academic practice. No use, distribution or reproduction is permitted which does not comply with these terms.
* Correspondence:
Ting Fan, School of Intelligent Medicine, China Medical University, Shenyang, 110122, Liaoning Province, China
Disclaimer: All claims expressed in this article are solely those of the authors and do not necessarily represent those of their affiliated organizations, or those of the publisher, the editors and the reviewers. Any product that may be evaluated in this article or claim that may be made by its manufacturer is not guaranteed or endorsed by the publisher.
Research integrity at Frontiers
Learn more about the work of our research integrity team to safeguard the quality of each article we publish.