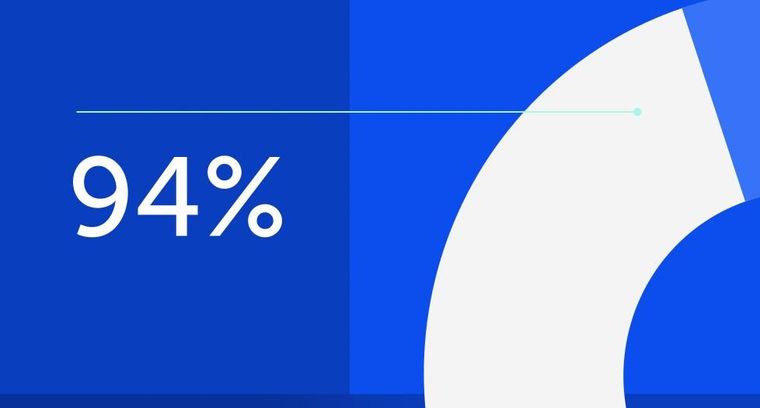
94% of researchers rate our articles as excellent or good
Learn more about the work of our research integrity team to safeguard the quality of each article we publish.
Find out more
ORIGINAL RESEARCH article
Front. Med.
Sec. Intensive Care Medicine and Anesthesiology
Volume 12 - 2025 | doi: 10.3389/fmed.2025.1570928
The final, formatted version of the article will be published soon.
Select one of your emails
You have multiple emails registered with Frontiers:
Notify me on publication
Please enter your email address:
If you already have an account, please login
You don't have a Frontiers account ? You can register here
Background: At present, there are no specialized models for predicting mortality risk in patients with alcoholic cirrhosis complicated by severe acute kidney injury (AKI) in the ICU. This study aims to develop and validate machine learning models to predict the mortality risk of this population during hospitalization. Methods: A retrospective analysis was conducted on 856 adult patients with alcoholic cirrhosis complicated by severe AKI, utilizing data from the MIMIC-IV database. Within the dataset, 627 patients from the period 2008 to 2016 were designated as the training cohort, whereas 229 patients from 2017 to 2019 comprised the temporal external validation cohort. Feature selection was conducted utilizing LASSO regression, which was subsequently followed by the development of eight distinct machine learning models. The performance of these models in the temporal external validation cohort was rigorously assessed using the area under the receiver operating characteristic curve (AUROC) to determine the optimal model. The model was interpreted using the SHAP method, and nomograms were subsequently constructed. A comprehensive evaluation was performed from the perspectives of discrimination (assessed via AUROC and AUPRC), calibration (using calibration curves), and clinical utility (evaluated through DCA curves). Results: LASSO regression identified nine key features: total bilirubin, acute respiratory failure, vasopressin, septic shock, oliguria, AKI stage, lactate, fresh frozen plasma transfusion, and norepinephrine. In the temporal external validation cohort, the Lasso-LR model achieved the highest AUROC value of 0.809, establishing it as the optimal model. We developed both a static nomogram and a web-based dynamic nomogram (https://zhangjingyu123456.shinyapps.io/dynnomapp/) for visualization purposes. In the nomogram model, the AUROC for the training cohort and temporal external validation cohort were 0.836 (95% CI: 0.802-0.870) and 0.809 (95% CI: 0.754-0.865), respectively. The calibration slope and Brier score for the training cohort were 1.000 and 0.146, respectively; for the temporal external validation cohort, these values were 0.808 and 0.177, respectively. The DCA curves indicate that the model has certain clinical application value. Conclusions: The Lasso-LR model exhibits robust predictive capability for in-hospital mortality among patients with alcoholic cirrhosis complicated by AKI, offering valuable prognostic insights and individualized treatment decision support for healthcare professionals.
Keywords: Alcoholic cirrhosis, Acute Kidney Injury, In-hospital mortality, machine learning, predictive model
Received: 04 Feb 2025; Accepted: 16 Apr 2025.
Copyright: © 2025 Sun, Liu, Min, Zhong, Zhang and Du. This is an open-access article distributed under the terms of the Creative Commons Attribution License (CC BY). The use, distribution or reproduction in other forums is permitted, provided the original author(s) or licensor are credited and that the original publication in this journal is cited, in accordance with accepted academic practice. No use, distribution or reproduction is permitted which does not comply with these terms.
* Correspondence: Zhian Du, First Affiliated Hospital of Jinzhou Medical University, Jinzhou, Liaoning Province, China
Disclaimer: All claims expressed in this article are solely those of the authors and do not necessarily represent those of their affiliated organizations, or those of the publisher, the editors and the reviewers. Any product that may be evaluated in this article or claim that may be made by its manufacturer is not guaranteed or endorsed by the publisher.
Supplementary Material
Research integrity at Frontiers
Learn more about the work of our research integrity team to safeguard the quality of each article we publish.