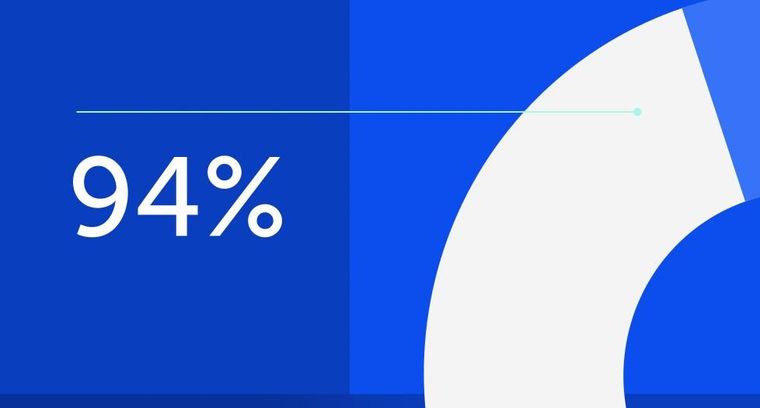
94% of researchers rate our articles as excellent or good
Learn more about the work of our research integrity team to safeguard the quality of each article we publish.
Find out more
ORIGINAL RESEARCH article
Front. Med.
Sec. Precision Medicine
Volume 12 - 2025 | doi: 10.3389/fmed.2025.1569464
This article is part of the Research TopicIntegrating AI and Machine Learning in Advancing Patient Care: Bridging Innovations in Mental Health and Cognitive NeuroscienceView all 3 articles
The final, formatted version of the article will be published soon.
Select one of your emails
You have multiple emails registered with Frontiers:
Notify me on publication
Please enter your email address:
If you already have an account, please login
You don't have a Frontiers account ? You can register here
Symptoms of autism spectrum disorder (ASD) range from mild to severe and are evident in early childhood. Children with ASD have difficulties with social interaction, language development, and behavioral regulation. ASD is a mental condition characterized by challenges in communication, restricted behaviors, difficulties with speech, nonverbal interaction, and distinctive facial features in children. The early diagnosis of ASD depends on identifying anomalies in facial function, which may be minimal or missing in the first stages of the disorder. Due to the unique behavioral patterns shown by children with ASD, facial expression analysis has become an effective method for the early identification of ASD. Hence, utilizing deep learning (DL) methodologies presents an excellent opportunity for improving diagnostic precision and efficacy. This study examines the effectiveness of DL algorithms in differentiating persons with ASD from those without, using a comprehensive dataset that includes images of children and ASD-related diagnostic categories. In this research, ResNet50, Inception-V3, and VGG-19 models were used to identify autism based on the facial traits of children. The assessment of these models used a dataset obtained from Kaggle, consisting of 2,940 face images. The suggested Inception-V3 model surpassed current transfer learning algorithms, achieving a 98% accuracy rate. Regarding performance assessment, the suggested technique demonstrated advantages over the latest models. Our methodology enables healthcare physicians to verify the first screening for ASDs in children.
Keywords: Transfer Learning, Deep leaning, diagnosis, Disability, Mental Health
Received: 31 Jan 2025; Accepted: 16 Apr 2025.
Copyright: © 2025 Al-Nefaie, Aldhyani, Ahmad and Alzahrani. This is an open-access article distributed under the terms of the Creative Commons Attribution License (CC BY). The use, distribution or reproduction in other forums is permitted, provided the original author(s) or licensor are credited and that the original publication in this journal is cited, in accordance with accepted academic practice. No use, distribution or reproduction is permitted which does not comply with these terms.
* Correspondence: Sultan Ahmad, Department of Computer Science, College of Computer Engineering and Sciences, Prince Sattam Bin Abdulaziz University, Alkharj, Saudi Arabia
Disclaimer: All claims expressed in this article are solely those of the authors and do not necessarily represent those of their affiliated organizations, or those of the publisher, the editors and the reviewers. Any product that may be evaluated in this article or claim that may be made by its manufacturer is not guaranteed or endorsed by the publisher.
Supplementary Material
Research integrity at Frontiers
Learn more about the work of our research integrity team to safeguard the quality of each article we publish.