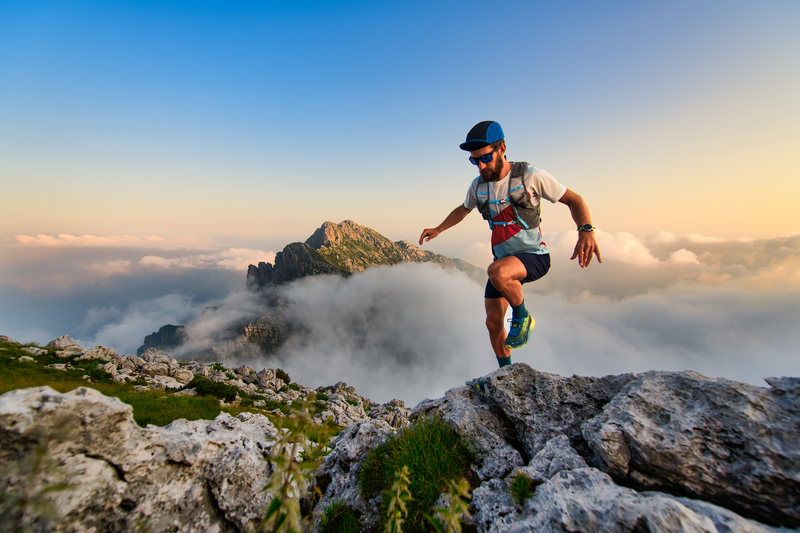
95% of researchers rate our articles as excellent or good
Learn more about the work of our research integrity team to safeguard the quality of each article we publish.
Find out more
ORIGINAL RESEARCH article
Front. Med.
Sec. Nuclear Medicine
Volume 12 - 2025 | doi: 10.3389/fmed.2025.1566555
This article is part of the Research Topic Innovative Approaches in Precision Radiation Oncology View all 6 articles
The final, formatted version of the article will be published soon.
You have multiple emails registered with Frontiers:
Please enter your email address:
If you already have an account, please login
You don't have a Frontiers account ? You can register here
Objective: Computed tomography (CT) imaging of parotid pleomorphic adenoma (PA) has been widely reported, nonetheless few reports have estimated the capsule characteristics of PA at length. This study aimed to establish and validate CT-based intratumoral and peritumoral radiomics models to clarify the characteristics between parotid PA with and without complete capsule.Methods: In total, data of 129 patients with PA were randomly assigned to a training and test set at a ratio of 7:3. Quantitative radiomics features of the intratumoral and peritumoral regions of 2 mm and 5 mm on CT images were extracted, and radiomics models of Tumor, External2, External5, Tumor + External2, and Tumor + External5 were constructed and used to train six different machine learning algorithms. Meanwhile, the prediction performances of different radiomics models (Tumor,External2,External5,Tumor+External2,Tumor+External5) based on single phase (plain, arterial, and venous phase) and multiphase (three-phase combination) were compared. The receiver operating characteristic (ROC) curve analysis and the area under the curve (AUC) were used to evaluate the prediction performance of each model.Results: Among all the established machine learning prediction radiomics models, the model based on a three-phase combination had better prediction performance, and the model using a combination of intratumoral and peritumoral radiomics features achieved a higher AUC than the model with only intratumoral or peritumoral radiomics features, and the Tumor+External2 model based on LR was the optimal model, the AUC of the test set was 0.817[95%CI=0.712, 0.847], and its prediction performance was significantly higher (p < 0.05, DeLong’s test) than that with the Tumor model based on LDA (AUC of 0.772), the External2 model based on LR (AUC of 0.751), and the External5 model based on SVM (AUC of 0.667). And the Tumor+External2 model based on LR had a higher AUC than the Tumor+External5 model based on LDA (AUC = 0.817 vs. 0.796), but no statistically significant difference (P=0.667). Conclusions: The intratumoral and peritumoral radiomics model based on multiphasic CT images could accurately predict capsular characteristics of parotid of PA preoperatively, which may help in making treatment strategies before surgery, as well as avoid intraoperative tumor spillage and residuals.
Keywords: Pleomorphic Adenomas, Radiomics, machine learning, Capsular, computed tomography
Received: 25 Jan 2025; Accepted: 03 Apr 2025.
Copyright: © 2025 Shen, Xiang, Han, Huang and Li. This is an open-access article distributed under the terms of the Creative Commons Attribution License (CC BY). The use, distribution or reproduction in other forums is permitted, provided the original author(s) or licensor are credited and that the original publication in this journal is cited, in accordance with accepted academic practice. No use, distribution or reproduction is permitted which does not comply with these terms.
* Correspondence:
Kui Huang, the Affiliated Stomatology Hospital of Southwest Medical University, Luzhou, China
Yongmei Li, Department of Radiology, The First Affiliated Hospital, Chongqing Medical University, Chongqing, China
Disclaimer: All claims expressed in this article are solely those of the authors and do not necessarily represent those of their affiliated organizations, or those of the publisher, the editors and the reviewers. Any product that may be evaluated in this article or claim that may be made by its manufacturer is not guaranteed or endorsed by the publisher.
Research integrity at Frontiers
Learn more about the work of our research integrity team to safeguard the quality of each article we publish.