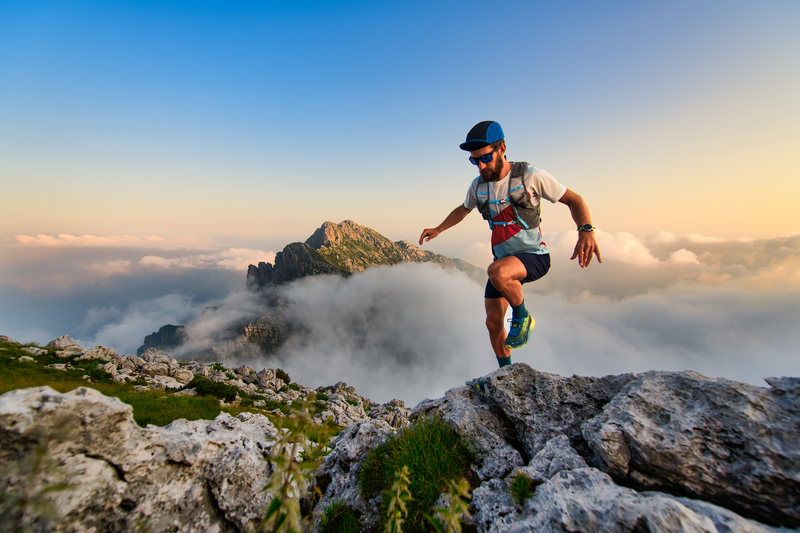
95% of researchers rate our articles as excellent or good
Learn more about the work of our research integrity team to safeguard the quality of each article we publish.
Find out more
ORIGINAL RESEARCH article
Front. Med.
Sec. Precision Medicine
Volume 12 - 2025 | doi: 10.3389/fmed.2025.1564446
This article is part of the Research Topic Unveiling Complex Medical Interdependencies Through High-Order Correlation Mining View all 9 articles
The final, formatted version of the article will be published soon.
You have multiple emails registered with Frontiers:
Please enter your email address:
If you already have an account, please login
You don't have a Frontiers account ? You can register here
Background: Transperineal ultrasound (TPUS) is widely utilized for the evaluation of female stress urinary incontinence (SUI). However, the diagnostic accuracy of parameters related to urethral mobility and morphology remains limited and requires further optimization.Objective: This study aims to develop and validate an optimized deep learning (DL) model based on TPUS images to improve the precision and reliability of female SUI diagnosis.Methods: This retrospective study analyzed TPUS images from 464 women, including 200 patients with SUI and 264 controls, collected between 2020 and 2024. Three DL models were trained on resting-state and Valsalva-state images using an 8:2 training-to-testing split. Model performance was assessed using diagnostic metrics, including area under the curve (AUC), accuracy, sensitivity, and specificity. A TPUS-index model, constructed using measurement parameters assessing urethral mobility, was used for comparison. Finally, the best-performing DL model was selected to evaluate its diagnostic advantages over traditional methods.Results: Among the three developed DL models, DenseNet-121 demonstrated the highest diagnostic performance, achieving an AUC of 0.869, an accuracy of 0.87, a sensitivity of 0.872, a specificity of 0.761, a negative predictive value of 0.788, and a positive predictive value of 0.853.When compared to the TPUS-index model, the DenseNet-121 model exhibited significantly superior diagnostic performance in both the training set (z = -2.088, p = 0.018 ) and the testing set (z = -1.997, p = 0.046 ).This study demonstrates the potential of DL models, particularly DenseNet-121, to enhance the diagnosis of female SUI using TPUS images, providing a reliable and consistent diagnostic tool for clinical practice.
Keywords: Stress urinary incontinence, deep learning, Transperineal ultrasound, Diagnostic accuracy, Densenet-121
Received: 21 Jan 2025; Accepted: 07 Apr 2025.
Copyright: © 2025 Chen, Chen, Nan, Sun, Ma and Yu. This is an open-access article distributed under the terms of the Creative Commons Attribution License (CC BY). The use, distribution or reproduction in other forums is permitted, provided the original author(s) or licensor are credited and that the original publication in this journal is cited, in accordance with accepted academic practice. No use, distribution or reproduction is permitted which does not comply with these terms.
* Correspondence:
Shanshan Yu, Department of Ultrasound, The Second Affiliated Hospital of Xi'an Jiaotong University, Xi'an, China
Disclaimer: All claims expressed in this article are solely those of the authors and do not necessarily represent those of their affiliated organizations, or those of the publisher, the editors and the reviewers. Any product that may be evaluated in this article or claim that may be made by its manufacturer is not guaranteed or endorsed by the publisher.
Research integrity at Frontiers
Learn more about the work of our research integrity team to safeguard the quality of each article we publish.