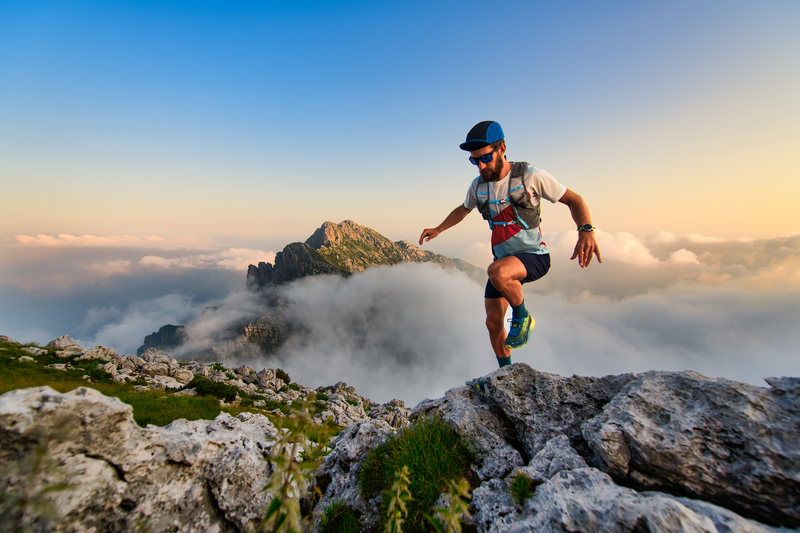
95% of researchers rate our articles as excellent or good
Learn more about the work of our research integrity team to safeguard the quality of each article we publish.
Find out more
ORIGINAL RESEARCH article
Front. Med.
Sec. Obstetrics and Gynecology
Volume 12 - 2025 | doi: 10.3389/fmed.2025.1562818
This article is part of the Research Topic Revolutionizing Cancer Care: AI and Technological Advances in Breast and Gynecological Oncology View all articles
The final, formatted version of the article will be published soon.
You have multiple emails registered with Frontiers:
Please enter your email address:
If you already have an account, please login
You don't have a Frontiers account ? You can register here
Objective: This study conducts a bibliometric analysis of artificial intelligence (AI) applications in cervical cancer to provide a comprehensive overview of the research landscape and current advancements.Methods: Relevant publications on cervical cancer and AI were retrieved from the Web of Science Core Collection. Bibliometric analysis was performed using CiteSpace and VOSviewer to assess publication trends, authorship, country and institutional contributions, journal sources, and keyword co-occurrence patterns.Results: From 1996 to 2024, our analysis of 770 publications on cervical cancer and AI showed a surge in research, with 86% published in the last five years. China (315 pubs, 32%) and the US (155 pubs, 16%) were the top contributors. Key institutions were the Chinese Academy of Sciences, Southern Medical University, and Huazhong University of Science and Technology. Research hotspots included disease prediction, image analysis, and machine learning in cervical cancer. Schiffman led in publications (12) and citations (207). China had the highest citations (3,819). Top journals were "Diagnostics," "Scientific Reports," and "Frontiers in Oncology." Keywords like "machine learning" and "deep learning" indicated current research trends. This study maps the field's growth, highlighting key contributors and topics.This bibliometric analysis provides valuable insights into research trends and hotspots, guiding future studies and fostering collaboration to enhance AI applications in cervical cancer.
Keywords: Qiang Huang, Male, Attending Physician, Master's Degree *Corresponding Author: Yanli Mo, Female, Attending Physician, Master's Degree Cervical Cancer, artificial intelligence, bibliometric analysis, machine learning, AI 1.Introduction
Received: 18 Jan 2025; Accepted: 24 Mar 2025.
Copyright: © 2025 Huang, Su, Li, Lin, Cheng, Chen and Mo. This is an open-access article distributed under the terms of the Creative Commons Attribution License (CC BY). The use, distribution or reproduction in other forums is permitted, provided the original author(s) or licensor are credited and that the original publication in this journal is cited, in accordance with accepted academic practice. No use, distribution or reproduction is permitted which does not comply with these terms.
* Correspondence:
Yanli Mo, Department of Pulmonary Oncology, Affiliated Hospital of Guangdong Medical University, Zhanjiang, China
Disclaimer: All claims expressed in this article are solely those of the authors and do not necessarily represent those of their affiliated organizations, or those of the publisher, the editors and the reviewers. Any product that may be evaluated in this article or claim that may be made by its manufacturer is not guaranteed or endorsed by the publisher.
Research integrity at Frontiers
Learn more about the work of our research integrity team to safeguard the quality of each article we publish.