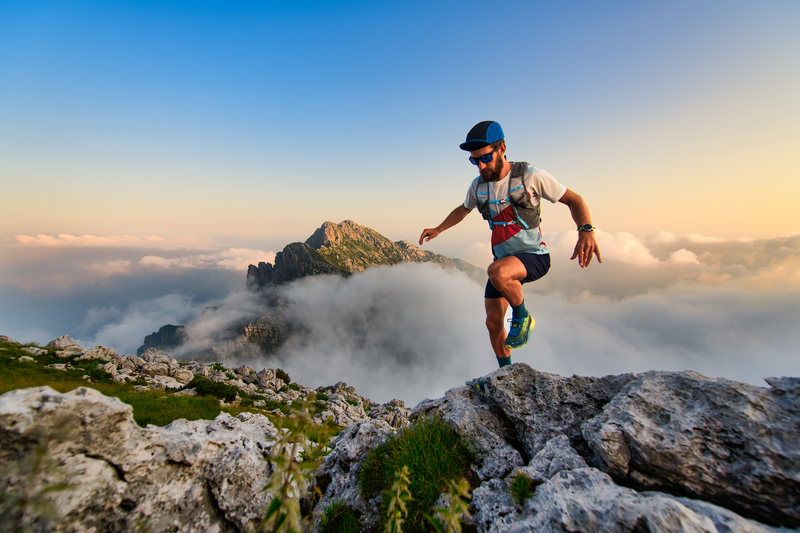
95% of researchers rate our articles as excellent or good
Learn more about the work of our research integrity team to safeguard the quality of each article we publish.
Find out more
ORIGINAL RESEARCH article
Front. Med.
Sec. Ophthalmology
Volume 12 - 2025 | doi: 10.3389/fmed.2025.1559435
This article is part of the Research Topic Myopia in Childhood and Adolescence View all 10 articles
The final, formatted version of the article will be published soon.
You have multiple emails registered with Frontiers:
Please enter your email address:
If you already have an account, please login
You don't have a Frontiers account ? You can register here
The primary objective of this study is to develop a predictive model utilizing fundamental clinical and ocular measurements to predict the effect of overnight orthokeratology on myopia control. Accordingly, this study aims to assist ophthalmologists in selecting adolescent myopia control methods.: This retrospective study used one-year follow-up data of 225 myopia children treated with orthokeratology. Using the random sampling method, 225 samples were randomly divided into a training set (n = 180) and a test set (n = 45). LASSO regression identified predictive factors correlated with controlling myopia. The final features are input into the machine learning model for prediction model construction to predict 1-year axial length elongation. The prediction performance was evaluated according to the accuracy and AUC of the training set and the test set. DCA was used to assess the clinical benefits of the model.Five features (age, diopter, flat keratometry, corneal higher-order aberrations (6mm), and intraocular trefoil (6mm) were used to build the machine learning model (P<0.01). Based on the accuracy, ROC, and DCA curves, the prediction performance and clinical practicability of five prediction models: KNN, SVM, RF, Extra Trees, and XGBoost were compared. In the DCA, all machine learning models consistently achieved greater net benefits within the clinical threshold range. SVM demonstrated the highest predictive quality with an AUC of 0.877 in the training and 0.828 in the external validation set.We developed and validated several prediction models for individualized prediction of myopia control efficacy treated with overnight orthokeratology through machine learning, using easily obtained clinical and corneal topography features. This cost effective strategy helps ophthalmologists predict the effect of using orthokeratology in children, and make timely adjustments to myopia control methods. The differential features selected by this model can also provide insights for optimizing lens design.
Keywords: machine learning, Myopia, Orthokeratology, Axial length, High-order aberration
Received: 12 Jan 2025; Accepted: 04 Apr 2025.
Copyright: © 2025 Zhang, Gao, Wang, Zhang, Zhu and Zhao. This is an open-access article distributed under the terms of the Creative Commons Attribution License (CC BY). The use, distribution or reproduction in other forums is permitted, provided the original author(s) or licensor are credited and that the original publication in this journal is cited, in accordance with accepted academic practice. No use, distribution or reproduction is permitted which does not comply with these terms.
* Correspondence:
Qi Zhao, Second Affiliated Hospital of Dalian Medical University, Dalian, China
Disclaimer: All claims expressed in this article are solely those of the authors and do not necessarily represent those of their affiliated organizations, or those of the publisher, the editors and the reviewers. Any product that may be evaluated in this article or claim that may be made by its manufacturer is not guaranteed or endorsed by the publisher.
Research integrity at Frontiers
Learn more about the work of our research integrity team to safeguard the quality of each article we publish.