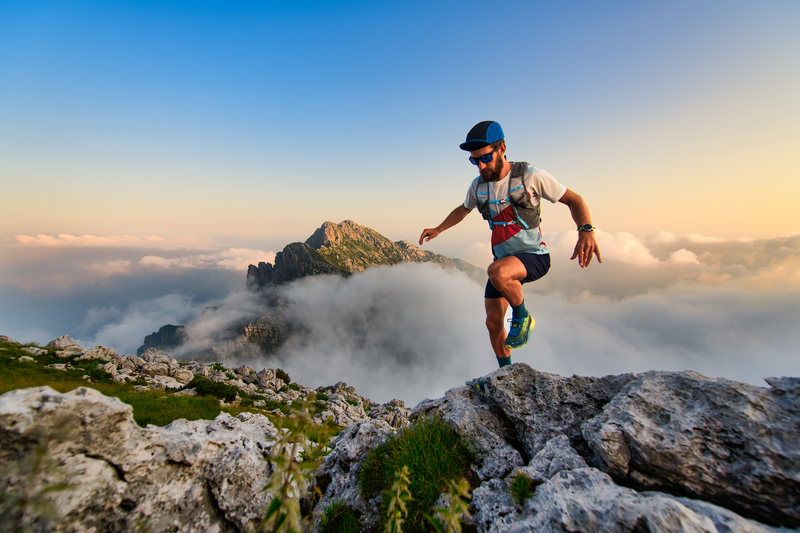
94% of researchers rate our articles as excellent or good
Learn more about the work of our research integrity team to safeguard the quality of each article we publish.
Find out more
ORIGINAL RESEARCH article
Front. Med.
Sec. Pathology
Volume 12 - 2025 | doi: 10.3389/fmed.2025.1557449
This article is part of the Research Topic Artificial Intelligence-Assisted Medical Imaging Solutions for Integrating Pathology and Radiology Automated Systems - Volume II View all 15 articles
The final, formatted version of the article will be published soon.
You have multiple emails registered with Frontiers:
Please enter your email address:
If you already have an account, please login
You don't have a Frontiers account ? You can register here
Medical image segmentation is essential for analyzing medical data, improving diagnostics, treatment planning, and research. However, current methods struggle with different imaging types, poor generalization, and rare structure detection. To address these issues, we propose MedFusion-TransNet, a novel multi-modal fusion approach utilizing transformer-based architectures. By integrating multi-scale feature encoding, attention mechanisms, and dynamic optimization, our method significantly enhances segmentation precision. Our method uses the Context-Aware Segmentation Network (CASNet) and Dynamic Region-Guided Optimization (DRGO) to enhance segmentation by focusing on key anatomical areas. These innovations tackle challenges like imbalanced datasets, boundary delineation, and multi-modal complexity. Validation on benchmark datasets demonstrates substantial improvements in accuracy, robustness, and boundary precision, marking a significant step forward in segmentation technologies. MedFusion-TransNet offers a transformative tool for advancing the quality and reliability of medical image analysis across diverse clinical applications.
Keywords: Medical image segmentation, Multi-modal fusion, transformer architecture, Dynamic optimization, Boundary precision
Received: 08 Jan 2025; Accepted: 03 Mar 2025.
Copyright: © 2025 Sun. This is an open-access article distributed under the terms of the Creative Commons Attribution License (CC BY). The use, distribution or reproduction in other forums is permitted, provided the original author(s) or licensor are credited and that the original publication in this journal is cited, in accordance with accepted academic practice. No use, distribution or reproduction is permitted which does not comply with these terms.
* Correspondence:
Jianfei Sun, Henglongjiang Puri High School, Heilongjiang, China
Disclaimer: All claims expressed in this article are solely those of the authors and do not necessarily represent those of their affiliated organizations, or those of the publisher, the editors and the reviewers. Any product that may be evaluated in this article or claim that may be made by its manufacturer is not guaranteed or endorsed by the publisher.
Research integrity at Frontiers
Learn more about the work of our research integrity team to safeguard the quality of each article we publish.