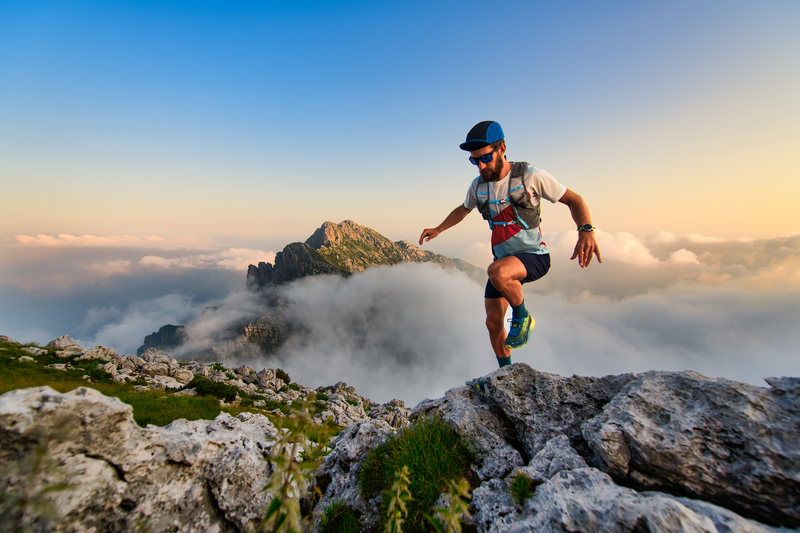
94% of researchers rate our articles as excellent or good
Learn more about the work of our research integrity team to safeguard the quality of each article we publish.
Find out more
ORIGINAL RESEARCH article
Front. Med.
Sec. Pathology
Volume 12 - 2025 | doi: 10.3389/fmed.2025.1555907
The final, formatted version of the article will be published soon.
You have multiple emails registered with Frontiers:
Please enter your email address:
If you already have an account, please login
You don't have a Frontiers account ? You can register here
Background: Recent advances in machine learning are transforming medical image analysis, particularly in cancer detection and classification. Techniques such as deep learning, especially convolutional neural networks (CNNs) and vision transformers (ViTs), are now enabling the precise analysis of complex histopathological images, automating detection, and enhancing classification accuracy across various cancer types. This study focuses on osteosarcoma (OS), the most common bone cancer in children and adolescents, which affects the long bones of the arms and legs. Early and accurate detection of OS is essential for improving patient outcomes and reducing mortality. However, the increasing prevalence of cancer and the demand for personalized treatments create challenges in achieving precise diagnoses and customized therapies.We propose a novel hybrid model that combines convolutional neural networks (CNN) and vision transformers (ViT) to improve diagnostic accuracy for OS using hematoxylin and eosin (H&E) stained histopathological images. The CNN model extracts local features, while the ViT captures global patterns from histopathological images. These features are combined and classified using a Multi-Layer Perceptron (MLP) into four categories: non-tumor (NT), non-viable tumor (NVT), viable tumor (VT), and non-viable ratio (NVR).Results: Using the Cancer Imaging Archive (TCIA) dataset, the model achieved an accuracy of 99.08%, precision of 99.10%, recall of 99.28%, and an F1-score of 99.23%. This is the first successful four-class classification using this dataset, setting a new benchmark in OS research and offering promising potential for future diagnostic advancements.
Keywords: Osteosarcoma, vision Transformer, histopathology, feature extraction, Classification
Received: 05 Jan 2025; Accepted: 24 Mar 2025.
Copyright: © 2025 Borji, Kronreif, Angermayr and Hatamikia. This is an open-access article distributed under the terms of the Creative Commons Attribution License (CC BY). The use, distribution or reproduction in other forums is permitted, provided the original author(s) or licensor are credited and that the original publication in this journal is cited, in accordance with accepted academic practice. No use, distribution or reproduction is permitted which does not comply with these terms.
* Correspondence:
Sepideh Hatamikia, Research Center for Clinical AI-Research in Omics and Medical Data Science (CAROM), Department of Medicine, Danube Private University (DPU), Krems, Austria
Disclaimer: All claims expressed in this article are solely those of the authors and do not necessarily represent those of their affiliated organizations, or those of the publisher, the editors and the reviewers. Any product that may be evaluated in this article or claim that may be made by its manufacturer is not guaranteed or endorsed by the publisher.
Research integrity at Frontiers
Learn more about the work of our research integrity team to safeguard the quality of each article we publish.