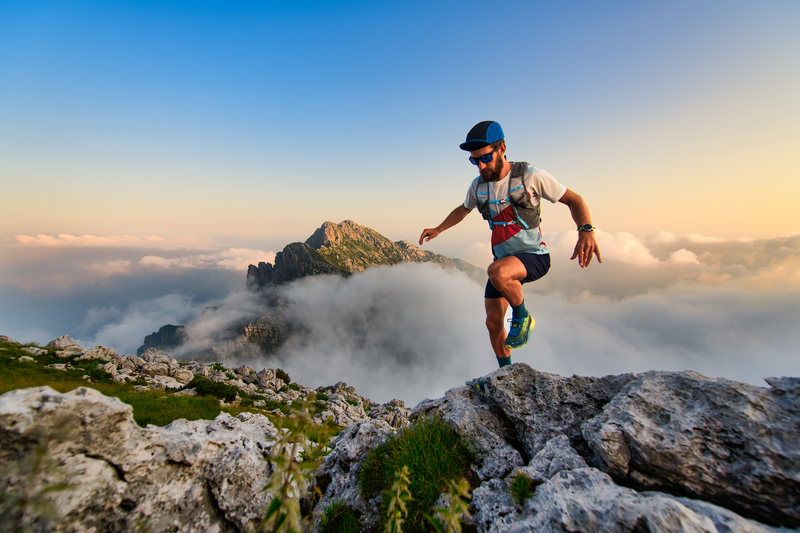
94% of researchers rate our articles as excellent or good
Learn more about the work of our research integrity team to safeguard the quality of each article we publish.
Find out more
ORIGINAL RESEARCH article
Front. Med.
Sec. Ophthalmology
Volume 12 - 2025 | doi: 10.3389/fmed.2025.1551557
This article is part of the Research Topic Efficient Artificial Intelligence in Ophthalmic Imaging – Volume II View all 3 articles
The final, formatted version of the article will be published soon.
You have multiple emails registered with Frontiers:
Please enter your email address:
If you already have an account, please login
You don't have a Frontiers account ? You can register here
Over 64 million people worldwide are affected by heart failure (HF), a condition that significantly raises mortality and medical expenses. In this study, we explore the potential of retinal optical coherence tomography (OCT) features as non-invasive biomarkers for the classification of heart failure subtypes: left ventricular heart failure (LVHF), congestive heart failure (CHF), and unspecified heart failure (UHF). By analyzing retinal measurements from the left eye, right eye, and both eyes, we aim to investigate the relationship between ocular indicators and heart failure using machine learning (ML) techniques. We conducted nine classification experiments to compare normal individuals against LVHF, CHF, and UHF patients, using retinal OCT features from each eye separately and in combination. Our analysis revealed that retinal thickness metrics, particularly ISOS-RPE and macular thickness in various regions, were significantly reduced in heart failure patients. Logistic regression, CatBoost, and XGBoost models demonstrated robust performance, with notable accuracy and area under the curve (AUC) scores, especially in classifying CHF and UHF. Feature importance analysis highlighted key retinal parameters, such as inner segment-outer segment to retinal pigment epithelium (ISOS-RPE) and inner nuclear layer to the external limiting membrane (INL-ELM) thickness, as crucial indicators for heart failure detection. The integration of explainable artificial intelligence further enhanced model interpretability, shedding light on the biological mechanisms linking retinal changes to heart failure pathology. Our findings suggest that retinal OCT features, particularly when derived from both eyes, have significant potential as non-invasive tools for early detection and classification of heart failure. These insights may aid in developing wearable, portable diagnostic systems, providing scalable solutions for personalized healthcare, and improving clinical outcomes for heart failure patients.
Keywords: Cardiovascular Diseases, Heart Failure, machine learning, deep learning, Explainable AI, UK Biobank
Received: 06 Jan 2025; Accepted: 03 Mar 2025.
Copyright: © 2025 Alyounis, ghosh, raja, alskafi, Yousefi and Khandoker. This is an open-access article distributed under the terms of the Creative Commons Attribution License (CC BY). The use, distribution or reproduction in other forums is permitted, provided the original author(s) or licensor are credited and that the original publication in this journal is cited, in accordance with accepted academic practice. No use, distribution or reproduction is permitted which does not comply with these terms.
* Correspondence:
Sona Alyounis, Khalifa University, Abu Dhabi, United Arab Emirates
Disclaimer: All claims expressed in this article are solely those of the authors and do not necessarily represent those of their affiliated organizations, or those of the publisher, the editors and the reviewers. Any product that may be evaluated in this article or claim that may be made by its manufacturer is not guaranteed or endorsed by the publisher.
Research integrity at Frontiers
Learn more about the work of our research integrity team to safeguard the quality of each article we publish.