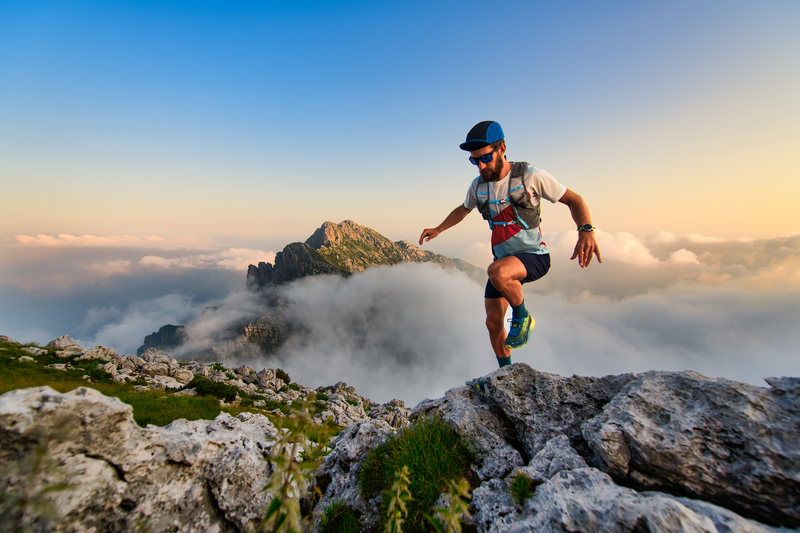
94% of researchers rate our articles as excellent or good
Learn more about the work of our research integrity team to safeguard the quality of each article we publish.
Find out more
ORIGINAL RESEARCH article
Front. Med.
Sec. Precision Medicine
Volume 12 - 2025 | doi: 10.3389/fmed.2025.1549491
This article is part of the Research Topic Unveiling Complex Medical Interdependencies Through High-Order Correlation Mining View all 6 articles
The final, formatted version of the article will be published soon.
You have multiple emails registered with Frontiers:
Please enter your email address:
If you already have an account, please login
You don't have a Frontiers account ? You can register here
Recognizing epilepsy through neurophysiological signals, such as the electroencephalogram (EEG), could provide a reliable method for epilepsy detection. Existing methods primarily extract effective features by capturing the time-frequency relationships of EEG signals but overlook the correlations between EEG signals. Intuitively, certain channel signals exhibit weaker correlations with other channels compared to the normal state. Based on this insight, we propose an EEGbased epilepsy detection method with graph correlation analysis (EEG-GCA), by detecting abnormal channels and segments based on the analysis of inter-channel correlations. Specifically, we employ a graph neural network (GNN) with weight sharing to capture target channel information and aggregate information from neighboring channels. Subsequently, Kullback-Leibler (KL) divergence regularization is used to align the distributions of target channel information and neighbor channel information. Finally, in the testing phase, anomalies in channels and segments are detected by measuring the correlation between the two views. The proposed method is the only one in the field that does not require access to seizure data during the training phase. It introduces a new state-of-the-art method in the field and outperforms all relevant supervised methods. Experimental results have shown that EEG-GCA can indeed accurately estimate epilepsy detection.
Keywords: Electroencephalogram, Graph neural networks, Correlation analysis, anomaly detection, Abnormal EEG Channels Detection
Received: 21 Dec 2024; Accepted: 25 Feb 2025.
Copyright: © 2025 Tian and Zhang. This is an open-access article distributed under the terms of the Creative Commons Attribution License (CC BY). The use, distribution or reproduction in other forums is permitted, provided the original author(s) or licensor are credited and that the original publication in this journal is cited, in accordance with accepted academic practice. No use, distribution or reproduction is permitted which does not comply with these terms.
* Correspondence:
Chongrui Tian, Harbin University of Science and Technology, Harbin, China
Disclaimer: All claims expressed in this article are solely those of the authors and do not necessarily represent those of their affiliated organizations, or those of the publisher, the editors and the reviewers. Any product that may be evaluated in this article or claim that may be made by its manufacturer is not guaranteed or endorsed by the publisher.
Research integrity at Frontiers
Learn more about the work of our research integrity team to safeguard the quality of each article we publish.