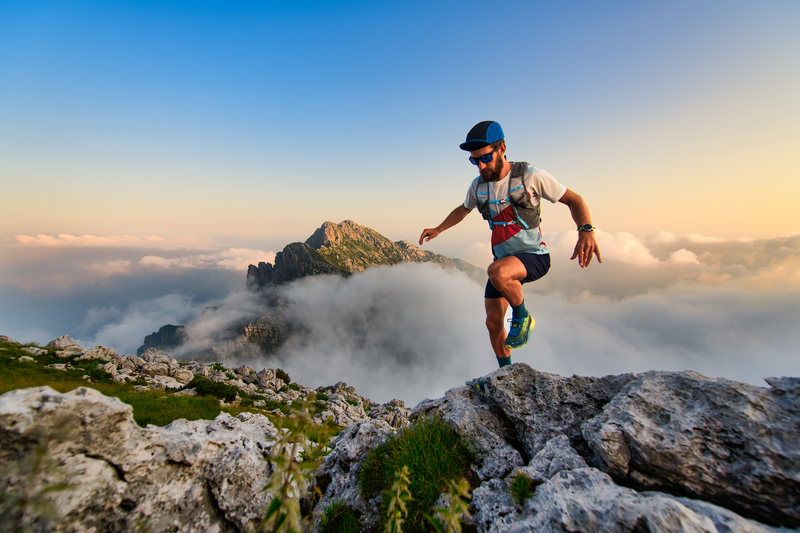
94% of researchers rate our articles as excellent or good
Learn more about the work of our research integrity team to safeguard the quality of each article we publish.
Find out more
ORIGINAL RESEARCH article
Front. Med.
Sec. Precision Medicine
Volume 12 - 2025 | doi: 10.3389/fmed.2025.1548726
The final, formatted version of the article will be published soon.
You have multiple emails registered with Frontiers:
Please enter your email address:
If you already have an account, please login
You don't have a Frontiers account ? You can register here
Background: Breast cancer (BC) is the most prevalent cancer among women and a leading cause of cancer-related deaths worldwide. Emerging evidence suggests that DNA methylation, a well-studied epigenetic modification, regulates various cellular processes critical for cancer development and progression and holds promise as a biomarker for cancer diagnosis and prognosis, potentially enhancing the efficacy of precision therapies.We developed a robust prognostic model for BC based on DNA methylation and clinical data from The Cancer Genome Atlas (TCGA) and Gene Expression Omnibus (GEO). We analyzed the association of the model with clinicopathological features, survival outcomes, and chemotherapy drug sensitivity.Results: A set of 216 differentially methylated CpGs was identified by intersecting three datasets (TCGA, GSE22249, and GSE66695). Using univariate Cox proportional hazard and Lasso Cox regression analyses, we constructed a 14-CpG model significantly associated with progression-free interval (PFI), disease-specific survival (DSS), and overall survival (OS) in BC patients. Kaplan-Meier (KM) survival analysis, receiver operating characteristic (ROC) analysis, and nomogram validation confirmed the clinical value of the signature. The Cox analysis showed a significant association between the signature and PFI and DSS in BC patients. KM analysis effectively distinguished high-risk from low-risk patients, while ROC analysis demonstrated high sensitivity and specificity in predicting BC prognosis.A nomogram based on the signature effectively predicted 5-and 10-year PFI and DSS. Additionally, combining our model with clinical risk factors suggested that patients in the I-II&M+ subgroup could benefit from adjuvant chemotherapy regarding PFI, DSS, and OS. Gene Ontology (GO) functional enrichment and KEGG pathway analyses indicated that the top 3000 differentially expressed genes (DEGs) were enriched in pathways related to DNA replication and repair and cell cycle regulation. Patients in the high-risk group might benefit from drugs targeting DNA replication and repair processes in tumor cells.The 14-CpG model serves as a useful biomarker for predicting prognosis in BC patients. When combined with TNM staging, it offers a potential strategy for individualized clinical decision-making, guiding personalized therapeutic regimen selection for clinicians.
Keywords: breast cancer, Prognostic model, DNA Methylation, biomarker, TCGA, GEO 14-CpG Methylation Signature for BC
Received: 20 Dec 2024; Accepted: 28 Feb 2025.
Copyright: © 2025 Tian, Yu, Qiu, Qiu, Zhuang, Chen, Gu, Hou and Gu. This is an open-access article distributed under the terms of the Creative Commons Attribution License (CC BY). The use, distribution or reproduction in other forums is permitted, provided the original author(s) or licensor are credited and that the original publication in this journal is cited, in accordance with accepted academic practice. No use, distribution or reproduction is permitted which does not comply with these terms.
* Correspondence:
Yi-fan Gu, School of Medicine, Shanghai Jiao Tong University, Shanghai, China
Disclaimer: All claims expressed in this article are solely those of the authors and do not necessarily represent those of their affiliated organizations, or those of the publisher, the editors and the reviewers. Any product that may be evaluated in this article or claim that may be made by its manufacturer is not guaranteed or endorsed by the publisher.
Research integrity at Frontiers
Learn more about the work of our research integrity team to safeguard the quality of each article we publish.