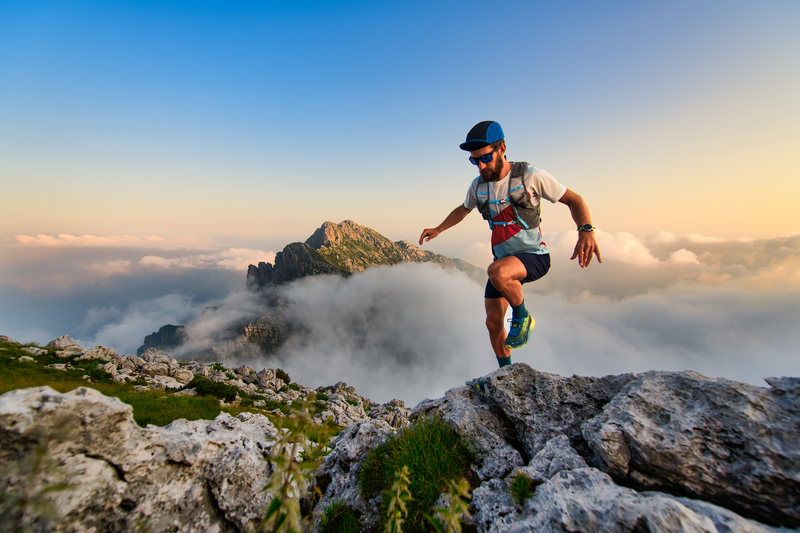
94% of researchers rate our articles as excellent or good
Learn more about the work of our research integrity team to safeguard the quality of each article we publish.
Find out more
ORIGINAL RESEARCH article
Front. Med.
Sec. Geriatric Medicine
Volume 12 - 2025 | doi: 10.3389/fmed.2025.1548034
This article is part of the Research Topic Challenges and Innovations in Healthcare Management and Long-Term Care for an Aging Society View all 3 articles
The final, formatted version of the article will be published soon.
You have multiple emails registered with Frontiers:
Please enter your email address:
If you already have an account, please login
You don't have a Frontiers account ? You can register here
This study aims to empirically examine the impact of healthy lifestyle behaviors on depressive symptoms among older adults in China, utilizing data from the 2020 China Health and Retirement Longitudinal Study (CHARLS 2020). A total of 9,020 valid samples of individuals aged 60 and above were analyzed. Sleep duration, social participation, and physical exercise were employed as independent variables, while depressive symptoms were assessed using the CESD-10 scale. Through the application of an ordered logistic regression model, The results show that there is a significant correlation between healthy sleep patterns, active social participation and regular physical exercise and the possibility of depression in the elderly (p < 0.05). These results suggest that promoting healthy lifestyle interventions could be a key strategy in alleviating the mental health burden in China's aging population.
Keywords: China elderly population, depressive symptoms, Healthy lifestyle behaviors, CHARLS, Mental Health
Received: 19 Dec 2024; Accepted: 12 Feb 2025.
Copyright: © 2025 Jiao, Ge-Zhang and Yang. This is an open-access article distributed under the terms of the Creative Commons Attribution License (CC BY). The use, distribution or reproduction in other forums is permitted, provided the original author(s) or licensor are credited and that the original publication in this journal is cited, in accordance with accepted academic practice. No use, distribution or reproduction is permitted which does not comply with these terms.
* Correspondence:
Jingqi Yang, Jiangxi Provincial People's Hospital, Nanchang, 330006, Jiangxi Province, China
Disclaimer: All claims expressed in this article are solely those of the authors and do not necessarily represent those of their affiliated organizations, or those of the publisher, the editors and the reviewers. Any product that may be evaluated in this article or claim that may be made by its manufacturer is not guaranteed or endorsed by the publisher.
Research integrity at Frontiers
Learn more about the work of our research integrity team to safeguard the quality of each article we publish.